Contents
ABSTRACT
Speedy technological, social, political, demographic, and urban developments continued to change the scale of the cities worldwide. Therefore, it becomes essential to model urban growth to minimize future uncertainties. Therefore, it is critical to investigate how cities grow and how researchers investigate which factors influence city growth. Using bibliometric methods, this study intends to pinpoint the publishing patterns and expansion potential of Urban Growth Modelling (UGM) works, providing a better understanding and possible future research paths. All published articles on the ‘Urban Growth model’ from Scopus were identified and analyzed using the Bibliometric R-package and VOSviewer software. 218 publications were identified from 1983 to 2023, published in 103 journals, and 25 book chapters contributed by 557 authors, with a 2.7 collaboration Index and 2.56 authors per document. The high-frequency keywords used in recent years are urban growth, land use, remote sensing, urban planning, cellular automaton, urban development, modeling, urbanization, Geographic Information System (GIS), and land use change. Research papers published in Computers, Environment, and Urban Systems are the most cited, with 1047 total citations, h-index 13. The most active country was China, with a total of 38 documents. The most cited paper for UGM research is titled ‘Modelling urban growth in Atlanta using logistic regression’, which received over 378 citations. The study’s findings offer milestones, a starting point for important research productivity data, and an understanding of how UGM research has evolved. This will help to estimate and assess the rate of urbanization, its location, and the consequences of before and after development before it gets stranded in unsuitable and unsustainable pathways.
INTRODUCTION
As per the World Urbanization Prospects (WUP) Report 2018, 41% of the population of lower-middle-income countries lived in cities, expected to rise to 59% by 2050. Although the pace of urbanization in lower-middle-income countries is slower than in developed countries, these countries are expected to have the fastest urban growth between now and 2050. This trend will undoubtedly continue in the future.[1] Speedy technological, social, political, demographic, and urban developments contributed to the changes in the scale of the major cities worldwide. Many have triggered these developments, i.e., the industrial revolution, capital gains, and population growth, which have played an essential part in cities’ urbanization.[2] This unprecedented urbanization is due to the current most significant demographic transition of this century from rural to urban areas and the growth of the peri-urban regions because of economic opportunities and the choice of people to live in nearby urban areas.[3,4] The inevitable outcome of this transition is the spatial extension of towns and cities beyond their city limits and into their peripheries and hinterland to provide settlement to the growing population (Mohammadian, Tavakoli, and Khani, 2017). This rapid urban expansion of urban areas has placed high pressure on urban land resources. It also adversely affects its urban environment at the expense of environmental degradation, loss of vegetation land, climate change, air pollution, terrestrial biodiversity loss, and other eco-environmental impacts.[3,6–13] This massive and complex city system involves many interactions between diverse agents and elements.[14] Therefore, the researchers believe that modeling these factors is critical to better understanding urban expansion’s spatial pattern, mechanism, and effects, which will help frame effective land-use policies.[6,15] Urban growth pattern analysis helps to identify the potential urban growth drivers that lead to the spatial expansion of areas beyond their judicial limits. Urban growth factors vary widely among the empirical studies.[6,16]
Therefore, the urban system requires integrated tools that help to guide and forecast urban growth due to its complex and dynamic nature.[17] Urban growth modeling will become crucial for effective urban area planning and management, maximizing the benefits of urban agglomeration and minimizing the adverse environmental impact.[18–21] While modeling some of these models shows the inability to incorporate socioeconomic variables, not indicate multicollinearity among independent factors, and use only relative weights, enhancing the model’s efficacy, scholars integrated two or more models to generate reliable urban growth models and growth patterns.
Furthermore, some researchers pointed out that bio-inspired algorithms can simulate and predict complex urban growth processes.[22,23] The Cellular Automata (CA) model has been considered the first analytical model as one of the bio-inspired models in urban growth prediction and is used widely in many studies that depend on the evaluation of complex urban development. However, CA models can simulate nominal data, not quantitative driving forces of urban expansion. Suppose it is combined with other spatial-quantitative models like the Markov Chain (MC). In that case, the Multi-Criteria Evaluation technique (MCE), Logistic Regression (LR) Land Transformation Model (LTM), and Agent-Based Modeling (ABM) can make more accurate predictions and modeling can be done. Generally, the MCE techniques have primarily been used to show the ranking order between different spatial factors. However, the value of each spatial element is calculated based on the experience of decision-makers.[24] As a result, the proposed land use pattern based on various combinations of weights will be highly disparate because the dynamic characteristics of spatial factors often fail to be observed. Therefore, the Weight of Evidence (WOE) method has been worked out to overcome the disadvantage of the MCE method because it prominently depends on the nature of data rather than on the subjective decision of individuals.[25]
The ABM can model the actions or decisions of individual agents and model processes based on influencing criteria closely related to a particular agent. Therefore, new factors will be required to load into the system to simulate the environment every time. Also, it works based on the assumption that the agent behavior will remain the same in the prediction year. Hence, it limits the simulation even if the condition changes due to interaction with other social, economic, political, and environmental factors.[26]
Furthermore, over the last 16 years, the SLEUTH (Slope, Land use, Exclusion, Urban, Transportation, and Hill shade), a growth-oriented model, has been successfully applied worldwide to forecast Land Use Land Cover Change (LULC) transition. At the same time, this model does not provide information regarding human behavior and ecological impacts. Also, the model’s drawback is that the simulation operation is constrained by particular variables that cannot be altered or developed.[27] Artificial Neural Networks (ANN), Decision Trees (DT), and Support Vector Machines (SVM) are all examples of Machine Learning (ML) models that are widely used in land-use applications. It can learn and fit the complex nature of components by iteration of training data and target data when the problem comprises input and output data in bulk amounts.[14,28,29] ML models are often combined with CA, LR, and FR models to increase the simulation accuracy. Simulating or modeling urban land expansion cannot be viewed as a linear problem because it involves complex interactions. Hence, various heuristic bio-inspired algorithms, such as the Genetic Algorithm (GA),[30,31] Simulated Annealing (SA),[32] Particle Swarm Optimization (PSO),[33–35] Ant Colony Optimization model (ACO),[36,37] Artificial Fish Swarm Algorithm (AFSA)[38] and Memetic Algorithm (MA),[39] bat-inspired algorithm,[40] Artificial Bee Colony (ABC) and shuffled leaf algorithm has been designed to achieve the optimal pattern of urban land use based on particular objectives and within required constraints. Looking at the facts of multivariable problems and multiple modelling options, it is necessary to showcase the researcher’s trend in the specific area in a classified way so that the researcher will make the mature choice in selecting the appropriate growth model for their studies. With the use of bibliometric methods, it is possible to showcase the research trend.
The study concentrated on presenting the findings on a single platform in light of the extensive research on urban growth modeling conducted worldwide. The main objective of this research is to provide a thorough analysis of the urban growth modeling techniques that have been indexed in the Scopus database from 1983 to 2023 in order to estimate and assess the rate of publication on urban growth modeling and to identify future research directions and techniques for planned and controlled urbanization, its location impact, and the effects of before and after development before it becomes stuck on unsuitable and unsustainable paths. This paper includes a bibliometric analysis of the urban growth modeling topic to explore the study of various urban growth models used over a forty year of span and to characterize the most cited documents, countrywide publications, most cited journal source, keyword occurrence analysis, conceptual structure map analysis of keywords, scientific collaboration of authors, thematic analysis of keywords used in publication in four sub-periods, collaboration analysis between countries. The main contribution of this study is to provide insights into the publication of the latest urban growth models with their pros and cons based on various machine learning techniques indexed in the Scopus database from 1983-2023.
DATA AND METHODS
Numerous methods exist for a literature review, including systematic literature review, content analysis, meta-analysis, and bibliometric analysis. To understand the impact of an article, bibliometric analysis includes statistical analysis of published articles and quantification of article citations. The primary requirement for good, high-quality literature is bibliometric analysis. It is a highly effective method for an in-depth literature review and study of academic publications that employ mathematical and statistical techniques. Bibliometric analysis is a numerical examination of various types of literature on a specific topic, excluding all aspects from which one can obtain information, such as leading authors, countries, journals, funding organizations, publishers, publisher city, web of science index, and research area.
Data Source and Research Platform
Scopus database was chosen as it uniquely combined expertly curated abstract and citation databases with enriched data. It also linked the scholarly literature from various disciplines. The search term for literature was “urban growth modeling”, which included many different search paths. Initially, 301 results were discovered. After applying the English language and subject areas filter related to social science, environmental science, earth and planetary science, computer science, engineering, multidisciplinary, and decision science, 218 papers were found pertinent to the above subject areas. Then, this data was converted into different raw data forms, which act as an input in bibliometric analysis. Figure 1 shows the methodology adopted for the study.
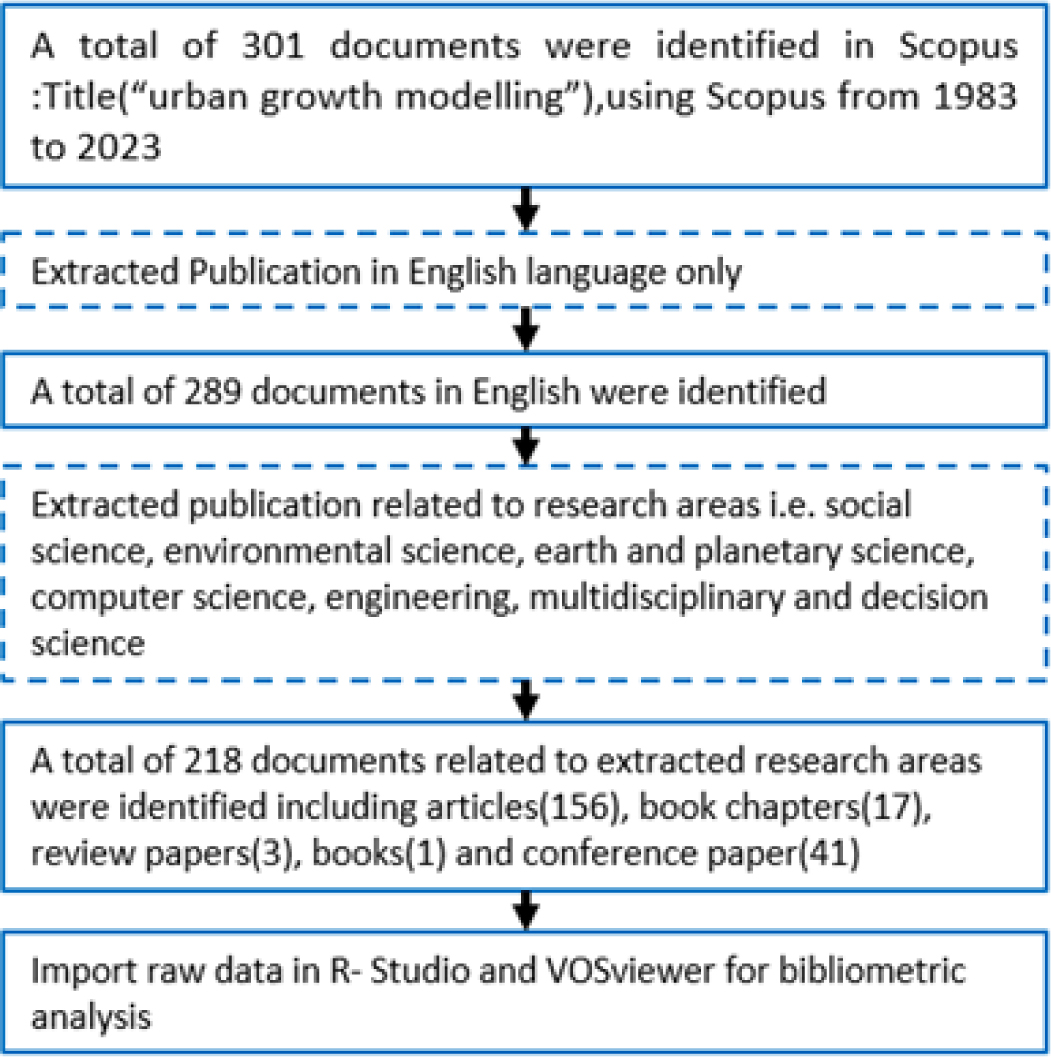
Figure 1:
Methodology of Bibliometric Analysis.
The bibliometric analysis was carried out with the help of Biblioshiny, an online web-based data analysis framework written in R language. With raw data from Scopus or Web of Science, this platform can be used for a variety of bibliometric analyses, such as statistical analysis, data preprocessing, co-occurrence matrix construction, co-citation analysis, coupling analysis, co-word analysis, thematic map evaluation, factorial analysis, historiography, collaboration network, collaboration world map, and cluster analysis.
The bibliometric analysis was done using the R language-based software package Biblioshiny, an online web-based data analysis framework. This platform, with data raw data obtained from Scopus or Web of Science, can be used for many bibliometric processes like statistical analysis, data preprocessing, co-occurrence matrix construction, co-citation analysis, coupling analysis, co-word analysis, and cluster analysis.
DATA ANALYSIS
Characteristics of the Meta Data
The retrieved documents were published between 1983 and 2023 in 128 journals by 557 authors, with a Collaboration Index of 2.70 per document. According to the analysis, the total citation score for all documents was 6,078. The majority of the documents, 156 (71.56%), were articles, followed by conference papers 41 (18.80%) and book chapters 17 (7.79%), as shown in (Table 1). Figure 2 depicts the annual trends in publication and citation times over the study period.
Description | Results | Description | Results |
---|---|---|---|
MAIN INFORMATION ABOUT DATA | DOCUMENT CONTENTS | ||
Timespan | 1983:2023 | Keywords Plus (ID) | 1215 |
Sources (Journals, Books, etc) | 128 | Author’s Keywords (DE) | 537 |
Documents | 218 | AUTHORS | |
Average years from publication | 8.89 | Authors | 557 |
Average citations per documents | 27.88 | Author Appearances | 686 |
Average citations per year per doc | 2.65 | Authors of single-authored documents | 17 |
References | 8891 | Authors of multi-authored documents | 540 |
DOCUMENT TYPES | AUTHORS COLLABORATION | ||
Article | 156 | Single-authored documents | 18 |
Book | 1 | Documents per Author | 0.391 |
Book Chapter | 17 | Authors per Document | 2.56 |
Conference Paper | 41 | Co-Authors per Documents | 3.15 |
Review | 3 | Collaboration Index | 2.7 |
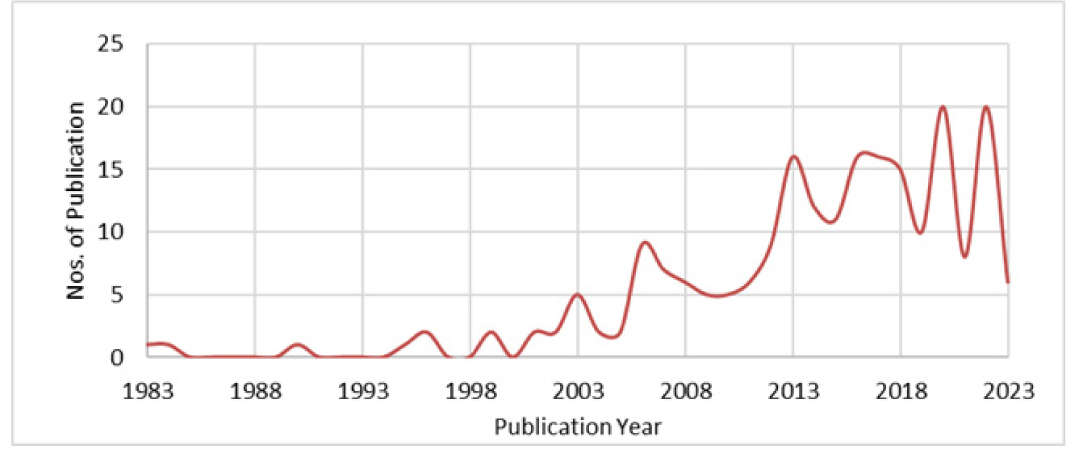
Figure 2:
Annual Trend of Publication on UGM from 1983 to 2023.
Most Cited Documents
The top 10 cited documents are presented in Table 2. The top most cited paper for UGM research is ‘Modeling urban growth in Atlanta using logistic regression’, which received over 378 citations. From this, it was determined that despite the lack of temporal dynamics, logistic regression was spatially explicit, suited for multi-scale research, and, most crucially, allowed for a far better comprehension of the processes influencing the development and growth of the urban spatial pattern,[42] followed by ‘Urban Growth Pattern Modeling: A Case Study of Wuhan City, PR China with 358 citations.[43] The later study proposed a Modelling of urban growth patterns.[44]
Author | Year | Title of Article | TC |
---|---|---|---|
Hu Z et al. | 2007 | Modeling urban growth in Atlanta using logistic regression | 378 |
Cheng J et al. | 2003 | Urban growth pattern modeling: a case study of Wuhan city, PR China | 358 |
Makse Ha et al. | 1995 | Modeling Urban Growth Patterns | 328 |
Weng Q et al. | 2001 | Modeling Urban Growth Effects on Surface Runoff with the Integration of Remote Sensing and GIS | 299 |
Luo J et al. | 2009 | Modeling spatial variations of urban growth patterns in Chinese cities: The case of Nanjing | 236 |
Yang X et al. | 2003 | Modeling urban growth and landscape changes in the Atlanta metropolitan area | 177 |
Feng Y et al. | 2011 | Modeling dynamic urban growth using cellular automata and particle swarm optimization rules | 170 |
Ahmed B et al. | 2012 | Modeling Urban Land Cover Growth Dynamics Using Multi-Temporal Satellite Images: A Case Study of Dhaka, Bangladesh | 159 |
Allen J et al. | 2003 | Modeling and Prediction of Future Urban Growth in the Charleston Region of South Carolina: a GIS-based Integrated Approach | 140 |
Rienow A et al. | 2015 | Supporting SLEUTH – Enhancing a cellular automaton with support vector machines for urban growth modeling | 128 |
Output analysis of top 10 countries wise publication
A total of 45 countries contributed to 218 papers. China tops the list with around 38 publications, followed by The United States of America (USA) with 32 papers and India with 18 papers. These three countries are the most productive countries for research in the field of UGM. Additionally, our results showed that China is ranked first regarding the number of documents and citations (Table 3).
Country | Articles | Freq. | SCP | MCP | TC |
---|---|---|---|---|---|
China | 38 | 21.35% | 24 | 14 | 146 |
USA | 32 | 17.98% | 28 | 4 | 126 |
India | 18 | 10.11% | 15 | 3 | 76 |
Iran | 15 | 8.43% | 11 | 4 | 62 |
Australia | 9 | 5.06% | 2 | 7 | 13 |
Malaysia | 6 | 3.37% | 3 | 3 | 12 |
Germany | 5 | 2.81% | 3 | 2 | 26 |
Japan | 5 | 2.81% | 5 | 0 | 17 |
Turkey | 5 | 2.81% | 4 | 1 | 15 |
United Kingdom | 5 | 2.81% | 2 | 3 | 17 |
Output analysis of the top 10 most cited Journal source
The 218 documents on UGM were published in 103 Journals, and the top 10 most published journals are listed in Table 4. Research papers on urban growth modeling published in Computers, Environment, and Urban Systems was the most significant number of publications 15, followed by ISPRS International Journal of Geo-Information with nine papers, and International Archives of the Photogrammetry, Remote Sensing and Spatial Information Sciences – ISPRS Archives with six papers.
Element | h-index | g-index | TC | NP |
---|---|---|---|---|
Computers, Environment and Urban Systems. | 13 | 15 | 1047 | 15 |
ISPRS International Journal of Geo-Information. | 7 | 9 | 347 | 9 |
International Archives of the Photogrammetry, Remote Sensing and Spatial Information Sciences – ISPRS Archives. | 4 | 5 | 33 | 6 |
International Journal of Geographical Information Science. | 6 | 6 | 397 | 6 |
Geocarto International. | 4 | 5 | 106 | 5 |
Journal of Urban Planning and Development. | 5 | 5 | 81 | 5 |
Landscape and Urban Planning. | 5 | 5 | 895 | 5 |
International Geoscience and Remote Sensing Symposium (IGARASS). | 2 | 3 | 11 | 4 |
Journal of the Indian Society of Remote Sensing. | 3 | 4 | 42 | 4 |
Egyptian Journal of Remote Sensing and Space Science. | 3 | 3 | 81 | 3 |
Keyword Occurrence Analysis
Keyword occurrence analysis identifies the keywords used in the titles of published research papers by authors working in urban growth modeling worldwide. Figure 3 depicts the result of the keywords plus analysis, which unveils the most used keywords in UGM literature, which can enable the identification of research themes and topics that have been heavily studied by researchers and documented in the Scopus database during the past 40 years.
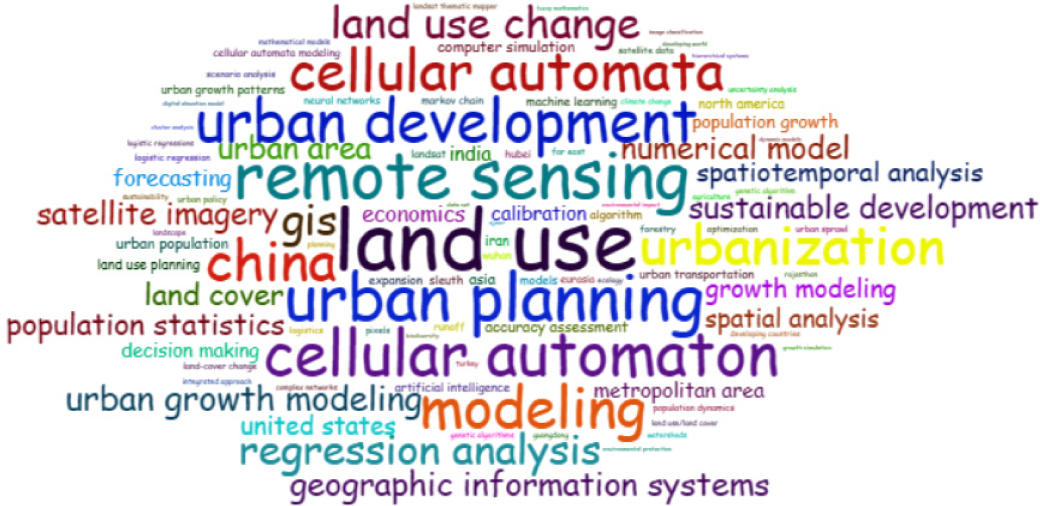
Figure 3:
Word clouds illustrating high-frequency words in the UGM.
Based on Keyword occurrence analysis, it is inferred that the researchers had used many keywords most frequently, like “urban growth” 182 times, “land use”:55 times, “remote sensing” 44 times, “urban planning” 43 times, “cellular automaton”:41 times, “urban development”: 39 times, “modeling”: 38 times, “urbanization”: 36 times, “GIS”:29 times, and “landuse change”:29 times, Further, in the current time the keyword urban growth occurs mainly with the Cellular automation, GIS and Urban Planning. This demonstrates the researchers’ interest in utilizing cutting-edge methods and technologies to simulate urban growth for improved land use and urban planning. Even so, there is little interest in using artificial intelligence and machine learning; potentially, this will be the focus of future studies.
Conceptual structure map analysis
Figure 4 shows the topic dendrogram based on factorial analysis of Keyword plus to represent the conceptual structure of the current literature on UGM for capturing an article content with greater depth of understanding the scientific concepts in UGM research over the past 40 years. The analysis of UGM mapping and the conceptual structure between reported keywords were visualized by using three methods: Correspondence Analysis (CA), Multiple Correspondence Analysis (MCA), and Multidimensional Scaling (MDS), as seen in (Figure 4 A, B, and C). The CA analysis shows the red cluster has the most keywords, i.e., growth modeling, urban planning, urban growth, sustainable development, satellite imagery, remote sensing, land use, urban growth modeling, cellular automata, geographic information system, spatiotemporal analysis, numerical model, urbanization, land cover, regression analysis, urban development, urban area and land use change which means the attention of the researchers to the UGM subject theme of the study. MCA analysis also shows that the red cluster has the most keywords. In contrast, MDS shows the blue cluster has the most fundamental words, i.e., growth modeling, land use change, numerical analysis land cover, spatiotemporal analysis, cellular automaton, regression analysis, urban development, urbanization, urban growth, and urban planning.
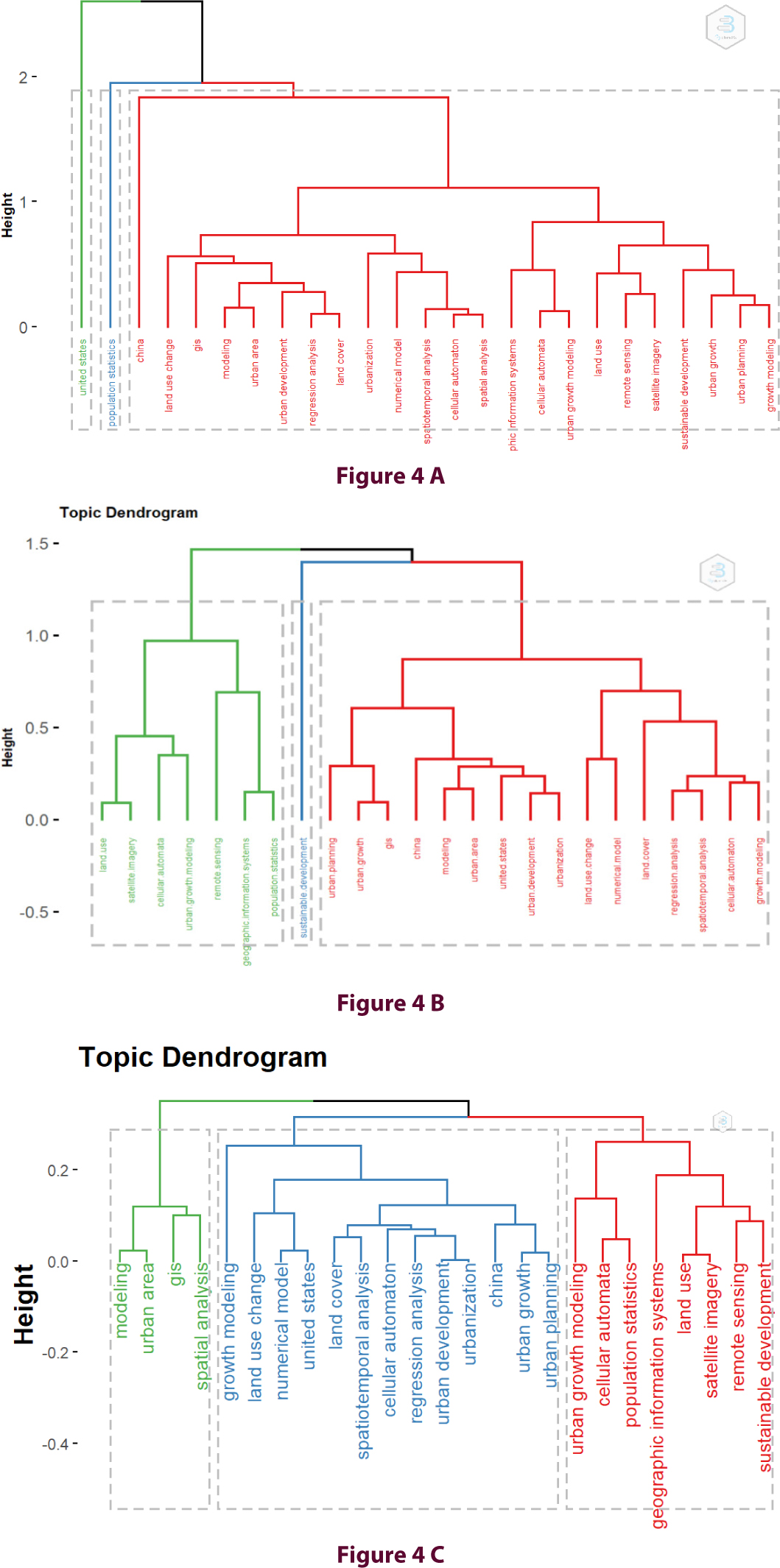
Figure 4:
Conceptual structure map of UGM themes using techniques of Correspondence Analysis (A), multiple Correspondence Analysis (B), and Multidimensional Scaling (C) method for (n=25 Keyword).
Co-authorship analysis between countries
Co-authorship analysis shows the contribution of authors from different countries in published research articles, which helps identify the research interest on the same theme by authors from different countries. The VOSviewer is used to visualize the social networks between co-authors and countries based on the Links (L) and Total Link Strength (TLS) having a link threshold of 4 occurrences. Among the initial 45 countries, only 14 met the thresholds and were grouped into 4 clusters with Links (L=60) and Total Link Strength (TLS=124). The United States was reported with (L=8; TLS=28), followed by China (L=7; TLS=24), Iran (L=6; TLS=11) times, Australia (L=5; TLS=10), United Kingdom (L=6; TLS=7) times, and Netherland (L=5; TLS=7), among others (Figure 5). Based on the Co-authorship analysis, most work is only carried out in developed nations. Still, developing and underdeveloped nations are not part of the network, so it is necessary to network with this kind of nation to create better sustainable urban planning by understanding the best-case scenario of each country and their experience with urban growth.
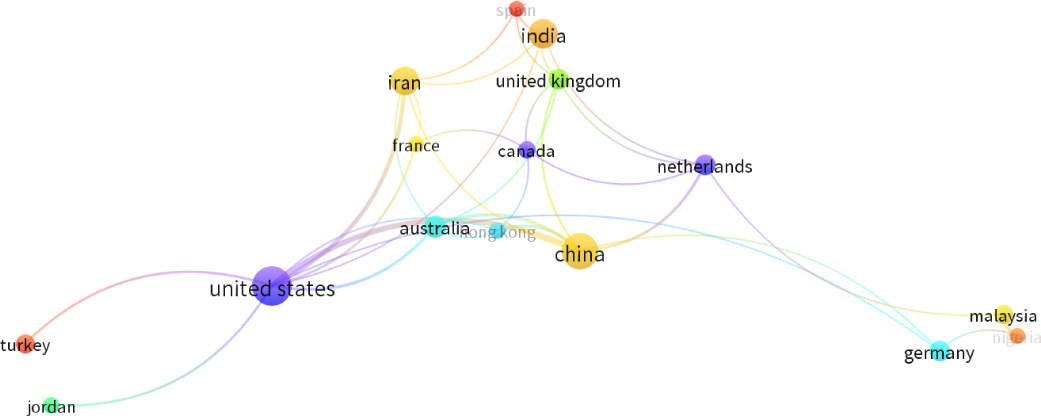
Figure 5:
Co-authorship analysis between countries based on the Links (L).
Thematic analysis
Figure 6 of thematic maps portrays prevailing and emerging themes on UGM-based keyword analysis concerning the thematic evolution area have identified two thematic areas over four sub-periods of time slices (1983-2008; 2009-2014; 2015-2017; 2018-2020, and 2021-2023) as presented in Figure 6 A. Moreover, thirty-three thematic areas were identified over these four sub-periods based on the article title by using thematic evolution of reported topics that caught the reader’s attention and identified the extent to which topics are related (Figure 6B).
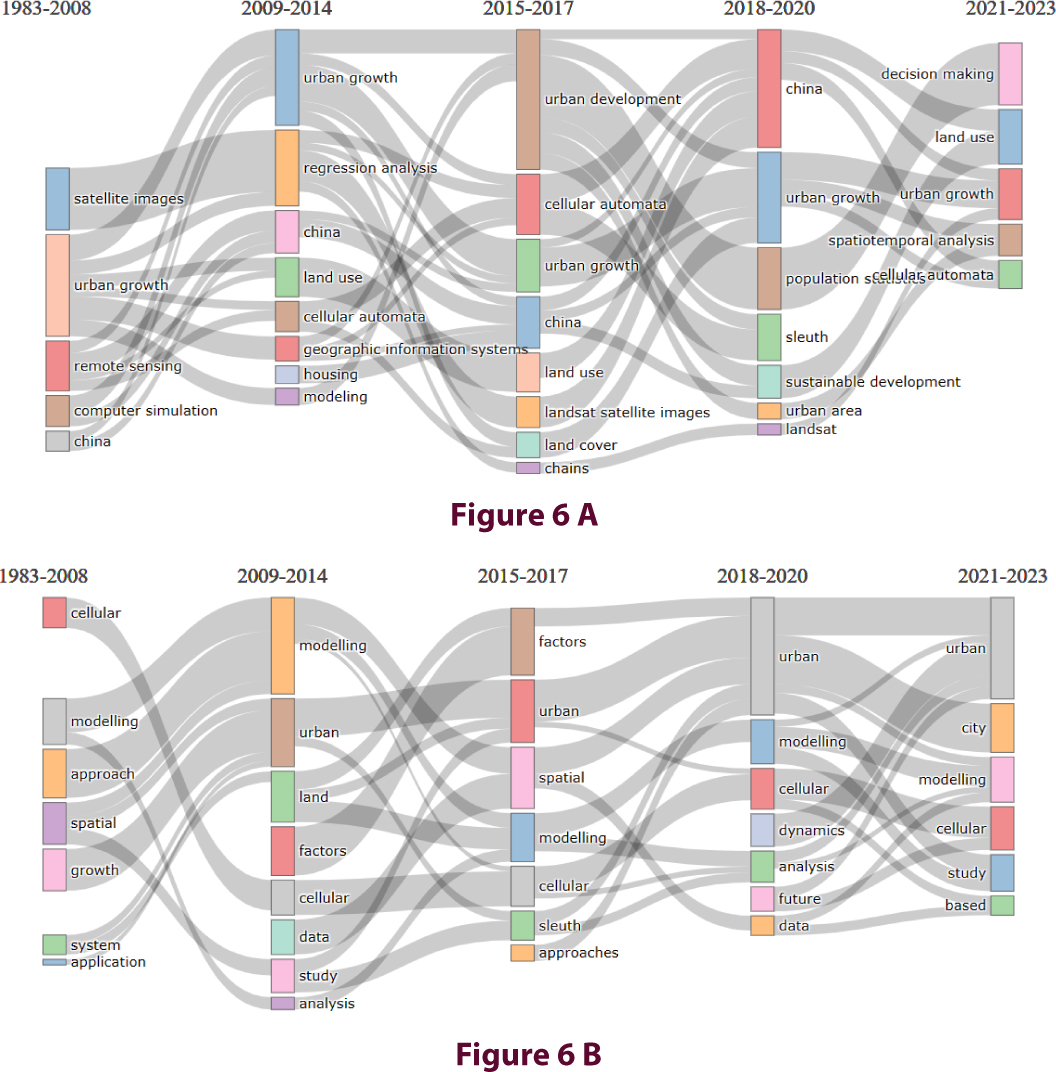
Figure 6:
Representative thematic evolution diagram based on (A) keyword plus and (B) Article Title.
Collaboration analysis between countries on UGM
R software was used to uncover new knowledge and determine the collaboration between researchers within the top 50 countries. The analysis of collaborations between countries shows that (the USA, China, and Germany) are located in clusters four, one, and five, respectively, with betweenness between countries as (235.48, 137.63, and 108.76) as can be seen in Table 5.
Country | Cluster | Betweenness | Closeness | PageRank |
---|---|---|---|---|
USA | 4 | 235.48 | 0.0049 | 0.128 |
China | 1 | 137.63 | 0.0048 | 0.110 |
Germany | 5 | 108.76 | 0.0046 | 0.045 |
United Kingdom | 3 | 95.17 | 0.0047 | 0.052 |
Spain | 3 | 65.75 | 0.0044 | 0.039 |
India | 3 | 55.35 | 0.0047 | 0.039 |
Iran | 4 | 42.43 | 0.0047 | 0.045 |
Netherlands | 3 | 36.99 | 0.0046 | 0.034 |
Jordan | 4 | 31.00 | 0.0043 | 0.018 |
France | 4 | 25.39 | 0.0045 | 0.025 |
Canada | 3 | 17.04 | 0.0044 | 0.026 |
Malaysia | 5 | 8.41 | 0.0041 | 0.024 |
Hong Kong | 1 | 6.23 | 0.0045 | 0.027 |
Nigeria | 5 | 5.89 | 0.0041 | 0.031 |
Australia | 1 | 5.49 | 0.0046 | 0.042 |
Japan | 2 | 2.00 | 0.0008 | 0.043 |
RESULTS AND DISCUSSION
Bibliometrics has had a tremendous impact in recent years, whether in governing, legislating, or seeking to comprehend particular scientific subjects better. To conduct the study, the data was retrieved from Scopus. This database provides different h-index ratings for journals needed to track citations and determine the impact of publications.[45] A total of 218 documents have been selected from the Scopus database. The retrieved documents were published from 1983-2023. The study focused on the comprehensive analysis of the global publication output and outlined possible future directions the researchers just venturing into the field of UGM by providing sufficient information on the growth and development of the literature, information on active authors, journals, countries, institutions, as well as complete keyword analysis for terms most frequently used in UGM research.
The findings showed that research surrounding UGM steadily increased and reached its highest peak between 2020 and 2022. The retrieved publications on UGM received many citations, with an average of 27.88 per document, indicating many readers and scholars. The most reported published documents in UGM literature were published as research articles 71.56%, followed by full conference papers 18.80% and book chapters 7.79%. These findings show that researchers in the UGM field prefer publishing their work as research papers rather than other types of documents.
The research papers in ‘Computers, Environment, and Urban Systems’ have been very active in publishing documents on UGM over the past years. The published documents had a relatively high reported scientific contribution and ranking, with around 15 documents receiving 1047 citations. Thus, based on the analysis and evidence reported, researchers seem particularly interested in publishing their research as research papers in these domains. They subsequently refer to these notes for reference in other publications. China tops the list with around 38 publications, followed by The United States of America (USA) with 32 papers and India with 18 papers. The top most cited paper for UGM research is ‘Modeling urban growth in Atlanta using logistic regression’, which received over 378 citations.
The keyword occurrence analysis mainly focused on ‘urban growth’, ‘land use’, ‘remote sensing’, ‘urban planning”, ‘cellular automaton’, ‘urban development’, ‘modeling’, ‘urbanization’, ‘GIS’, and ‘landuse change’ among others. These top 10 keywords can be used to identify future research hotspots for UGM. Conceptual structure map analysis using three models (CA, MCA, and MDS) shows the distribution of the topic. It means the researchers’ attention to the study’s UGM subject theme.
In addition, urban growth and urban development decision-making research has received more attention during the recent time slice about the thematic evolution analysis. Modeling urban growth in Atlanta using Logistic regression article published by Hu Z et al., 2007,[42] and Urban Growth Pattern Modeling: a case study of Wuhan City, PR China, published by Cheng J et al. 2003 attracted the interest of most scientists, and they had the highest citations.[43] These articles originally introduced UGM to the international urban planning community.
Based on the Total Link Strength (TLS), the United States, China, Iran, and Australia showed high occurrence in UGM research. Despite its many advantages, our study has limitations, i.e., it is only based on the Scopus database. In future bibliometric analysis, researchers may consider using more diverse data sources. Future bibliometric studies might consider using other databases, such as Web of Science and Google Scholar, to provide a more comprehensive overview of research productivity in UGM.
IMPLICATIONS
The present paper highlights the trends in Urban Growth modeling based on the researcher’s thoughts on the research direction, tools, techniques, and networking countries. From the study, it is found that urban growth’, ‘land use’, ‘remote sensing’, ‘urban planning”, ‘cellular automaton’, ‘urban development’, ‘modeling’, ‘urbanization’, ‘GIS’, and ‘landuse change are the most frequent word used by the researchers. However, very few have used artificial intelligence and machine learning; potentially, this will be the focus of future studies.
Even though the models are already available based on bottom-up and top-down approaches, problems remain as they found in literature studies. Most studies found the individual influence of growth drivers rather than the combined influence of different growth drivers and their impact on human development. Because, in a massive and complex environment, spatial interactions among growth drivers are required to explore, which is not well explored in available studies.
The spatial structure of an urban system is primarily the result of the spatial interaction of driving forces at local, regional, national, and global levels.[15] However, previous empirical studies have limitations, like driving forces considered for urban expansion are only at a city-scale level without considering the regional aspect. The city’s growth is also influenced by nearby cities, i.e., regional level effect. There is also spatial autocorrelation among independent growth factors. Still, only a few researchers have used the geographically weighted regression method to incorporate that effect. Most urban simulation studies use logistic or binary logistic regression models, which cannot represent a non-linear relationship among independent growth drivers.[6]
Most recent studies on urban growth simulation are limited to six to seven driving growth factors of urbanization. Also, models used for urban growth simulation are processed by considering the probability of only the dynamic nature of one growth factor. However, there is complicated interaction and competition among the urban components in a real urban world. Thus, the occurrence of changes in one driving factor also makes changes in other related growth factors. Hence, capturing this complex and dynamic effect of the real urban world through simulation becomes inevitable by coupling human and natural impact with other factors.[46] Furthermore, very few studies of urban growth simulation have considered the potential of the socioeconomic variables and climatic variables. Because of long-term land-use dynamics, future climate changes will considerably impact the city’s growth, and hence, it is necessary to study the impact of extreme weather events.
Traditional models, such as the CA models, are constrained to incorporate quantitative driving forces. Therefore, combining CA with other quantitative models, such as MC, MCE, LR, LTM, and ABM, is essential. These analytical techniques are unable to model more complex data. Therefore, ML models may be used to simulate potential land-use change. ML models can be combined with CA, LR, and FR models to increase the simulation accuracy.[14,28,29] The land parcel can be considered a small child because its activities are mainly based on nearby nature, and finally, it will mimic some of that. Further researchers can use bio-inspired algorithms to solve complex, high-dimensional, multi-modal problems.
In the end, calibration and validation of the model are essential, and it is found that the scholars have used mainly 12 types of calibration and validation techniques for study areas ranging from 88.5 sq. km to 9.6 million sq. km for Urmia, Iran[49] and mainland China[46] respectively. It’s also found that most researchers have calibrated 10 to 20 years of spatial and non-spatial data and forecasted for 10 to 40 years having a resolution of 30 m by using neighborhood window sizes varying from 3×3 m[10] to 11×11 m.[50] Delicate resolution data and window size 5×5 have given satisfactory results,[12,51] so the research can use such calibration and validation techniques based on the budget, resources, and type of assignments.
Urban growth simulation studies reveal mainly nineteen types of scenarios that researchers may consider for their studies like sustainable development, conservation priorities, perspective of carrying capacity, business as usual, Coalescence, Diffusion, Protection of Cropland, Impact of climate change, Open space conservation, Unconstrained Development Scenario, Constrained Development Scenario, without zoning, Zone based on the type of land use, zone based on administrative division, Zone based on development planning subdivision, zone based on urbanized suitability, Intensified Residential development, encouragement of Industrial and commercial development, Optimum allocation of land use and perspective of land acquisition based on bargaining between farmers and governments. Over time, researchers modeled urban growth using analytical models, machine learning algorithms, and bio-inspired algorithms. Now, the time has come to adopt artificial intelligence to model urban growth for a sustainable future.
CONCLUSION
The number of research papers on UGM has steadily increased for the past ten years. It examined the material published between 1983 and 2023 and discovered that it was written by 557 authors from 45 countries and published in 128 Scopus-indexed sources. The study offers markers for locating significant UGM research hot spots. The study also provides future researchers additional data on country collaborations based on single and multiple country publications. The analysis of the geographic distribution of UGM research revealed that high-income countries produced more than 50% of the UGM research. Also, very few empirical research studies have been found regarding evaluating urban growth patterns, models of urban growth, and future urban growth scenarios related to cities of Asian developing countries like India, Nepal, and Sri Lanka, except China. In the case of India, the spatial landuse structure is too much different from the developed countries. Because Indian cities mainly consist of mixed landuse, the spatial relations of growth drivers like social capital, migration pattern, landuse policies, lifestyle, topographical and climatic conditions, market forces, industrialization, commuter’s travel pattern, travel cost, and social behavior required to explore. Therefore, this review will help to estimate and assess the rate of urbanization, its location, and the consequences of before and after development before it gets stranded in unsuitable and unsustainable pathways. As a result, additional empirical research analysis that includes low-income and lower-middle-income countries would strengthen the foundation for suggesting and implementing effective policy measures to promote UGM in broader contexts. Several developing countries may be facing significant challenges in the field. Over time, researchers modeled urban growth using analytical models, machine learning algorithms, and bio-inspired algorithms. Now, the time has come to adopt artificial intelligence to model urban growth for a sustainable future.
Cite this article:
Sheladiya KP, Patel CR. Leveraging Urban Growth Models (UGM) for Sustainable Urban Planning and Climate Resilient Cities: A Bibliometric Analysis. J Scientometric Res. 2023;12(3):585-95.
ABBREVIATIONS
UGM | Urban Growth Modelling |
---|---|
WUP | World Urbanization Prospects |
GIS | Geographic Information System |
CA | Cellular Automata |
MC | Markov Chain |
MCE | Multi-Criteria Evaluation |
LR | Logistic Regression |
LTM | Land Transformation Model |
ABM | Agent-Based Model |
WOE | Weight of Evidence |
SLEUTH | Slope, Land use, Exclusion, Urban, Transportation, and Hill shade |
LULC | Land use land cover change |
ANN | Artificial Neural Networks |
DT | Decision Trees |
SVM | Support Vector Machines |
ML | Machine Learning |
GA | Genetic Algorithm |
SA | Simulated Annealing |
PSO | Particle Swarm Optimization |
ACO | Ant Colony Optimization |
AFSA | Artificial Fish Swarm Algorithm |
MA | Memetic Algorithm |
BA | Bat-inspired Algorithm |
ABC | Artificial Bee Colony |
References
- United Nations. World Urbanization Prospects. 2018
- Altuwaijri HA, Alotaibi MH, Almudlaj AM, Almalki FM. Predicting urban growth of Arriyadh city, capital of the Kingdom of Saudi Arabia, using Markov cellular automata in TerrSet geospatial system. Arab J Geosci. 2019;12(4) [Google Scholar]
- Taylor P, Yuan M, Liu Y, He J, Liu D. Cartography and Geographic Information Science Regional land-use allocation using a coupled MAS and GA model : from local simulation to global optimization, a case study in Caidian District, Wuhan, China. 2014:37-41. [Google Scholar]
- Hasnine M, Rukhsana. An Analysis of Urban Sprawl and Prediction of Future Urban Town in Urban Area of Developing Nation: Case Study in India. J Indian Soc Remote Sens. 2020;48(6):909-20. [CrossRef] | [Google Scholar]
- Mohammadian H, Tavakoli J, Khani H. Monitoring land use change and measuring urban sprawl based on its spatial forms The case of Qom city. Egypt J Remote Sens Sp Sci. 2017;20(1):103-16. [CrossRef] | [Google Scholar]
- Li G, Sun S, Fang C. The varying driving forces of urban expansion in China: Insights from a spatial-temporal analysis. Landsc Urban Plan. 2018;174:63-77. Available from [CrossRef] | [Google Scholar]
- Poelmans L, Van Rompaey A. Computers, Environment and Urban Systems Complexity and performance of urban expansion models. Comput Environ Urban Syst. 2010;34(1):17-27. [CrossRef] | [Google Scholar]
- Wang W, Jiao L, Zhang W, Jia Q, Su F, Xu G, et al. Delineating urban growth boundaries under multi-objective and constraints. Sustain Cities Soc. 2020;61:102279 Available from: [CrossRef] | [Google Scholar]
- Zhou L, Dang X, Sun Q, Wang S. Multi-scenario simulation of urban land change in Shanghai by random forest and CA-Markov model. Sustain Cities Soc. 2020;55:102045 [CrossRef] | [Google Scholar]
- Mandal J. Urban Growth Dynamics and Changing Land-Use Land-Cover of Megacity Kolkata and Its Environs. J Indian Soc Remote Sens. 2019;47(10):1707-25. [CrossRef] | [Google Scholar]
- Jiao L, Dong T, Xu G, Zhou Z, Liu J, Liu Y, et al. Geographic micro-process model: Understanding global urban expansion from a process-oriented view. Comput Environ Urban Syst. 2021;87:101603 [CrossRef] | [Google Scholar]
- Shu B, Zhu S, Qu Y, Zhang H, Li X, Carsjens GJ, et al. Modelling multi-regional urban growth with multilevel logistic cellular automata. Comput Environ Urban Syst. 2020:80 [CrossRef] | [Google Scholar]
- Yang J, Gong J, Tang W, Liu C. Patch-based cellular automata model of urban growth simulation: Integrating feedback between quantitative composition and spatial configuration. Comput Environ Urban Syst. 2020;79:101402 [CrossRef] | [Google Scholar]
- Grekousis G. Computers, Environment and Urban Systems Artificial neural networks and deep learning in urban geography : A systematic review and meta-analysis. Comput Environ Urban Syst. 2019;74:244-56. [CrossRef] | [Google Scholar]
- Dadashpoor H, Malekzadeh N. Driving factors of formation, development, and change of spatial structure in metropolitan areas: A systematic review. J Urban Manag. 2020;9(3):286-97. [CrossRef] | [Google Scholar]
- Liu AW and Y. Cellular Automata for Urban Growth Modelling: A Review on Factors defining Cellular Automata for Urban Growth Modelling: A Review on Factors Defining Transition Rules. 2016 [CrossRef] | [Google Scholar]
- Thapa RB, Murayama Y. Computers, Environment and Urban Systems Urban growth modeling of Kathmandu metropolitan region, Nepal. Comput Environ Urban Syst. 2020;35(1):25-34. [CrossRef] | [Google Scholar]
- Li X, Zhou W, Ouyang Z. Forty years of urban expansion in Beijing: What is the relative importance of physical, socioeconomic, and neighborhood factors? Appl Geogr. 2013;38(1):1-10. Available from: [CrossRef] | [Google Scholar]
- Aburas MM, Ho YM, Ramli MF, Ash’aari ZH. Improving the capability of an integrated CA-Markov model to simulate spatio-temporal urban growth trends using an Analytical Hierarchy Process and Frequency Ratio. Int J Appl Earth Obs Geoinf. 2017;59:65-78. [CrossRef] | [Google Scholar]
- Feng Y, Liu Y, Batty M. Modeling urban growth with GIS based cellular automata and least squares SVM rules: a case study in Qingpu–Songjiang area of Shanghai, China. Stoch Environ Res Risk Assess. 2016;30(5):1387-400. [CrossRef] | [Google Scholar]
- Bohnet IC, Pert PL. Landscape and Urban Planning Patterns, drivers and impacts of urban growth — A study from Cairns, Queensland, Australia from 1952 to 2031. Landsc Urban Plan. 2010;97(4):239-48. [CrossRef] | [Google Scholar]
- Kar AK. Bio inspired computing – A review of algorithms and scope of applications. Expert Syst Appl. 2016;59:20-32. [CrossRef] | [Google Scholar]
- Neshat M, Sepidnam G, Sargolzaei M, Najaran A. Artificial fish swarm algorithm : a survey of the state- and indicative applications. 2014:965-97. [CrossRef] | [Google Scholar]
- Park S, Jeon S, Kim S, Choi C. Prediction and comparison of urban growth by land suitability index mapping using GIS and RS in South Korea. Landsc Urban Plan. 2011;99(2):104-14. [CrossRef] | [Google Scholar]
- Zheng X, Lv L. A WOE method for urban growth boundary delineation and its applications to land use planning. 2016:8816 [CrossRef] | [Google Scholar]
- Mustafa A, Cools M, Saadi I, Teller J. Coupling agent-based, cellular automata and logistic regression into a hybrid urban expansion model (HUEM). Land use policy. 2017;69:529-40. [CrossRef] | [Google Scholar]
- Rienow A, Goetzke R. Supporting SLEUTH Enhancing a cellular automaton with support vector machines for urban growth modeling. Comput Environ Urban Syst. 2015;49:66-81. [CrossRef] | [Google Scholar]
- Li X, Yeh AG. Neural-network-based cellular automata for simulating multiple land use changes using GIS. 2010:8816 [CrossRef] | [Google Scholar]
- Maithani S. A Neural Network based Urban Growth Model of an Indian City. J Indian Soc Remote Sens. 2009:363-76. [CrossRef] | [Google Scholar]
- Sha H, Tayyebi A, Ahmadlou M. Computers, Environment and Urban Systems Integration of genetic algorithm and multiple kernel support vector regression for modeling urban growth. 2017;65:28-40. [CrossRef] | [Google Scholar]
- Feng Y. Modeling dynamic urban land-use change with geographical cellular automata and generalized pattern search-optimized rules. Int J Geogr Inf Sci. 2017;31(6):1198-219. [CrossRef] | [Google Scholar]
- Mohammadi M, Nastaran M, Sahebgharani A. Development, application, and comparison of hybrid meta-heuristics for urban land-use allocation optimization: Tabu search, genetic, GRASP, and simulated annealing algorithms. Comput Environ Urban Syst. 2016;60:23-36. [CrossRef] | [Google Scholar]
- Eberhart R, Kennedy J. A New Optimizer Using Particle Swarm Theory. Sixth Int Symp Micro Mach Hum Sci. 1995:39-43. [CrossRef] | [Google Scholar]
- Blecic I, Cecchini A, Trunfio GA. Fast and accurate optimization of a GPU-accelerated CA urban model through cooperative coevolutionary particle swarms. Procedia Comput Sci. 2014;29:1631-43. [CrossRef] | [Google Scholar]
- Feng Y, Liu Y, Tong X, Liu M, Deng S. Modeling dynamic urban growth using cellular automata and particle swarm optimization rules. Landsc Urban Plan [Internet]. 2011;102(3):188-96. [CrossRef] | [Google Scholar]
- Ma S, Li X, Cai Y. Computers, Environment and Urban Systems Delimiting the urban growth boundaries with a modified ant colony optimization model. Comput Environ Urban Syst. 2017;62:146-55. [CrossRef] | [Google Scholar]
- Ma S, Liu F, Ma C, Ouyang X. Integrating logistic regression with ant colony optimization for smart urban growth modelling. Front Earth Sci. 2020;14(1):77-89. [CrossRef] | [Google Scholar]
- Huang X, Xu G, Xiao F. Optimization of a novel urban growth simulation model integrating an artificial fish swarm algorithm and cellular automata for a smart city. Sustain. 2021;13(4):1-25. [CrossRef] | [Google Scholar]
- Veerbeek W, Pathirana A, Ashley R, Zevenbergen C. Enhancing the calibration of an urban growth model using a memetic algorithm. Comput Environ Urban Syst. 2015;50:53-65. [CrossRef] | [Google Scholar]
- Cao M, Bennett SJ, Shen Q, Xu R, Cao M, Bennett SJ, et al. A bat-inspired approach to define transition rules for a cellular automaton model used to simulate urban expansion. Int J Geogr Inf Sci. 2016;30(10):1961-79. [CrossRef] | [Google Scholar]
- Sha H. Computers, Environment and Urban Systems Improving spatial accuracy of urban growth simulation models using ensemble forecasting approaches. 2019;76:91-100. [CrossRef] | [Google Scholar]
- Hu Z, Lo CP. Modeling urban growth in Atlanta using logistic regression. Comput Environ Urban Syst. 2007;31(6):667-88. [CrossRef] | [Google Scholar]
- Cheng J, Ottens H, Masser I, Turkstra J. Understanding urban growth: A conceptual model. Int J Urban Sci. 2003;7(1):83-101. [CrossRef] | [Google Scholar]
- Makse HA, Havlin S, Stanley HE. Modelling urban growth patterns. Nature. 1995;377:608-12. [CrossRef] | [Google Scholar]
- Musa IH, Zamit I. Mapping of Cross-Lingual Emotional Topic Model Research Indexed in Scopus databases from 2000-2020. Scientometr Res. 2022;11(3):427-35. [CrossRef] | [Google Scholar]
- Liu X, Liang X, Li X, Xu X, Ou J, Chen Y, et al. Landscape and Urban Planning A future land use simulation model (FLUS) for simulating multiple land use scenarios by coupling human and natural effects. Landsc Urban Plan. 2017;168:94-116. [CrossRef] | [Google Scholar]
- Huang Q, Song W. A land-use spatial optimum allocation model coupling a multi-agent system with the shuffled frog leaping algorithm. Comput Environ Urban Syst [Internet]. 2019;77:101360 Available from: [CrossRef] | [Google Scholar]
- Aburas MM, Ho YM, Ramli MF, Ash’aari ZH. The simulation and prediction of spatio-temporal urban growth trends using cellular automata models: A review. Int J Appl Earth Obs Geoinf [Internet]. 2016;52:380-9. Available from: [CrossRef] | [Google Scholar]
- Naghibi F, Delavar M. Discovery of Transition Rules for Cellular Automata Using Artificial Bee Colony and Particle Swarm Optimization Algorithms in Urban Growth Modeling. ISPRS Int J Geo-Information. 2016;5(12):241 [CrossRef] | [Google Scholar]
- Siddiqui A, Siddiqui A, Maithani S, Jha AK, Kumar P, Srivastav SK, et al. The Egyptian Journal of Remote Sensing and Space Sciences Urban growth dynamics of an Indian metropolitan using CA Markov and Logistic Regression. Egypt J Remote Sens Sp Sci. 2018;21(3):229-36. [CrossRef] | [Google Scholar]
- Berbero Akın A, Clarke KC. Landscape and Urban Planning Cellular automata modeling approaches to forecast urban growth for adana, Turkey: A comparative approach. 2016;153:11-27. [CrossRef] | [Google Scholar]