ABSTRACT
Search engines may be thought of as gateways to the entire Internet universe. As a result, they have an impact on the search results that are displayed for each query. People from all areas of life now have greater access to information because of the creation and widespread use of search engines. Most search engines do not favor or bias their results. Generally, search engines aim for impartiality, striving to provide comprehensive results for every query, irrespective of personal bias. The search terms entered by users shape the outcomes, underscoring the pivotal role of user input in this process. Evaluating the neutrality of search results entails acknowledging occasional disregard for user requests. This study investigates how confirmation bias affects users’ search behavior and health literacy when seeking health information online. We conducted research involving 89 internet users tasked with accessing health information online. Participants were provided with biased information related to the search topic to challenge their preconceived beliefs. Subsequent analysis of their search activity logs revealed that individuals inclined towards confirmation bias tended to focus on results aligning with their pre-existing beliefs, enabling faster task completion. However, this approach compromised health literacy, vital for critically evaluating online health information. This highlights the importance of addressing confirmation bias in online health information-seeking behavior to promote informed decision-making and enhance health literacy among internet users. Thus, the study elucidated that the individuals who neglect health literacy hinder their ability to effectively navigate the wealth of health information on the internet. This highlights the importance of cultivating critical thinking skills and unbiased information-seeking behaviors in the digital age.
INTRODUCTION
Search engines may be thought of as gateways to the entire Internet universe. As a result, they have an impact on the search results that are displayed for each query. People from all areas of life now have greater access to information because of the creation and widespread use of search engines. Most search engines do not favor or bias their results. The results must be comprehensive in every facet of the search. They must maintain neutrality when assessing the data provided by their software whether or not it is relevant to the topic. The terms entered by a search engine, on the other hand, determine the results. There are various factors to consider regarding the neutrality of search results. They should be prepared for their requests to be ignored sometimes. Another investigation was conducted as part of this inquiry. The study was conducted, and the findings of two of the most popular search engines were analysed. A search for “abortion,” for example, yields several results spread across multiple pages.[1,2] The legalization of homosexual marriage, as well as the rising use of marijuana for medicinal purposes, are both causing problems. Our research is based on the following principles: to appear as one of the more conservative search engine results or something a little softer. We also give three sentiment-based measures because it is vital to demonstrate the presence of bias and compare opposing viewpoints. Use two separate search engines to accomplish two unique goals.[3,4] A significant amount of research has been conducted on tough problems that are now being explored in depth. In addition to displaying the two search engines’ bias, when it comes to a specific area of dispute, they both exhibit the same type of prejudice, albeit for different reasons. This essay aims to look at online search results and user behaviour. Another type of cognitive bias that affects humans is known as confirmation bias. When people seek knowledge that confirms their existing beliefs, they develop this sort of bias. 89 people utilised the internet platform during the user testing. Many individuals use the internet to research health issues. During the study, we were able to affect the participants’ perceptions by providing them with background information.[5] As a result, we could keep our preconceived notions under control. We then looked at their search history to see if any patterns emerged. Confirmation bias is a prevalent participant error documented in several studies. I completed the assignment quickly because I was interested in the first few pages of results. Despite being aware of confirmation bias and having some awareness of health concerns, individuals chose to reject this viewpoint, according to the data.[6–8] When one studies health information on the internet with skepticism, the need for literacy becomes clear. Many people rely largely on search engines as their major source of information.
Although early search engines like Google exist, the grading techniques used by Alta Vista are not unduly difficult. For example, extra enhancement, such as a higher Google page rank, is there. A scoring method uses click stream data in addition to other factors modifying everything about the scenario’s conditions. Modern search engines are equipped with a wide range of functions. Several elements are considered simultaneously while ranking and retrieving information. The core algorithms employed by search engines to establish rankings which are only utilised as a supplement. Given the variety of information available, the information provided to people may be deemed problematic.
Most people are unaware that the driving force behind search engines is algorithms, which are computer programs. In this sense, the outcomes provided to them were unbiased by their prejudices and assumptions. They are expected to address every aspect of the search. They must retain objectivity throughout the process of evaluating the data offered by the software that they use. Whether or not it is pertinent to the discussion, Search neutrality, on the other hand, is not always the norm.[9,10] This component is essential to the operation of modern search engines. It is critical to consider the use of a search engine. This medium allows site visitors to get news aggregated from several sources. Some Internet sites provide material for Search Engine Results Pages (SERPs). Depending on how the results are organised, certain results may be favoured over others, such as perspective from within the data collection that has been made public.
To put it another way, imagine that the consumer is seeking something in 2016. The presidential election results are given to voters in order, with each outcome ranked from best to worst. It makes no difference to me if the completed collection leans toward a certain political viewpoint, such as a conservative or liberal one, locating instances of bias in a volume of published material. The problem is challenging, and the solution will only get more intricate as time passes. We have an issue with the scenario where rankings are included and the fact that rankings are considered. The spectrum of emotions represented in a work of writing includes both good and negative. Not only are the actual outcomes important, but so is how they shape up in contrast to the distribution.[11–13] According to the most recent studies, increasing the efficacy of the ranking method using a learning-to-rank technique. The articles discovered online relate to a user’s search keywords; as a result, the ranking algorithm may be responsible for the appearance of prejudice. For example, what is known as a “training set” in the context of algorithmic ranking? The clickthrough logs generated by users and, hence, inherently biased or generated artificially are the basic principles of algorithmic learning that the algorithm’s developers emphasized. Human characteristics can influence the system’s learning process and cause bias. The phrase “personalization learning to rank” was coined because of the interaction between these two components. If computer programs are to blame for the prejudice, the outputs of such programs are also to blame.[14] The user’s preferences have a role in choosing which publications are shown. Users who like to acquire their news from liberal sites, for example, will find that she most likely did it because of the previous results she clicked on. It is believed that the software will determine that the user prefers positive information and will utilize it accordingly. It gives visitors access to liberalism-related information. The top K publications all share a left-wing viewpoint. In each of these instances, the outcomes that were presented suggest that those who are concerned about the topic in question may retain an “unjustified bias” against a particular point of view, as the term implies.
Relying on search engine results, may have been modified, to return the materials relevant to the present inquiry. As a result, doing so currently is not appropriate. When people seek contentious matters to debate, bias becomes a greater concern. As a result of this, the findings lacked an objective perspective. This is where most of our attention is focused, particularly on controversial topics.[15,16] The SERPs supplied by Google and Bing are examined in this study. Let’s use Bing’s method to see whether there are any noteworthy discrepancies between our various points of view. We may use opinion mining to determine whether a search engine’s results page may have a bias toward favoring or disfavouring a specific query. The pages of search engine results from at least two different queries were collected and analysed. The search engine at issue is a specific one within the scope of this article, we hope to answer the following questions (RQ):
RQ1: How to acquire search engine results that are not biased in any way. This question aims to assess the level of bias in search engine results and whether search engines are truly neutral in delivering information.
RQ2: How do search engines handle sensitive subject questions? This question addresses the challenges search engines face when dealing with contentious topics and explores strategies for ensuring fairness and objectivity in such cases.
RQ3: How can conservative and liberal ideas be distinguished from one another? This question seeks to understand how search engine results may reflect different ideological perspectives and whether these distinctions are due to algorithmic bias or user behavior.
RQ4: What factors differentiate various search engines in terms of the information they provide? This question investigates the unique features and biases of different search engines and their impact on the diversity of information accessible to users.
RQ5: What are some of the most divisive issues in search engine results? This question aims to identify and analyze the topics or domains where bias and controversy are most prominent, shedding light on the challenges of navigating online information.
If the information gained via the use of search engines has any bias, then it is feasible for us to determine if the bias is due to a bias in the algorithm or a bias in the data that was submitted. The following is a definition of the research question scope based on the preceding two research questions: It is conceivable that unjustified prejudice will be difficult to discern, especially when the rankings garner a lot of attention and thought. As a result, determining the extent of prejudice that occurs is difficult. It is structured by how many ways of thinking make up the final group. There is one more criterion to consider, and that is how well the results rank. To answer the second issue, we will compare the two search engines using both ranking data and sentiment.[17] Due to the controversial nature of some topics, we would like to know if the two search engines have similar or unique points of view on issues.
To be more specific, we provide a method for comparing texts based on sentiment to identify if a search engine’s results include any bias and to compare their unique points of view on disputed issues in a pairwise approach. According to the results of our testing, both search engines produce biased results for the opinion-based metrics we employed in our research. These two search engines were utilised. This problem is also known as “input bias,” which refers to bias incorporated into the corpus. Furthermore, our findings show that Bing and Google have similar viewpoints on the large majority of the subjects that have been discussed. The paper will have the following structure: To begin, we will review past research on search bias that has been conducted. During the second half of our discussion, we will further detail the sentiment-based comparison metrics we utilize. In the next section, we will go through the structure of our SERP dataset crawling and assessment in detail. Following the presentation of the results of our experiments and subsequent discussion of our findings, we conclude our study.
Related Work
The notion of “search neutrality,” as defined by the New York Times, can be summarised as follows: concerns about the possibility of bias in search engine results are best summarised by.[9] The lists of results produced by search algorithms in response to search queries are not created using any objective criterion, and the technology underlying search engines are not unbiased; instead, they are built with features that prioritize some values over others. All these things are done automatically by the most prominent search engines in the results lists they provide in response to user queries. According to the findings of certain research conducted by Robert Epstein and Ronald E. Robertson,[3] search engines influence election outcomes. Manipulation of search engine results may have an impact on election outcomes. The following is an excerpt from the abstract: Five studies conducted in two nations concluded that modifying search engine algorithms had a substantial impact.
Furthermore, such rankings can be camouflaged so that individuals show no symptoms of being aware of the manipulation. Even though their study is based on user research done in various countries, they lack the tools required to detect and assess bias automatically. According to a more recent study conducted by the same group of researchers, it can be difficult to detect bias in election-related search results, which has been proven to significantly influence the decisions made by undecided voters.[4] In contrast to prior research that presented a mechanism for automatically detecting search bias, this study gives an actual analysis.
This blog article reviews a study on Google’s bias in search results, discovering that 40% of the results were skewed left or liberal. Human labelers come from many walks of life and hold a broad spectrum of political views.[18] According to the author of an essay that was prominently covered in The Guardian, search engine algorithms tend to favour content that is either fake or biased in favour of the right. The author expressed this viewpoint in an essay. The core of our work is detecting and analyzing bias in search engine results, with a particular emphasis on well-known news sources such as the New York Times and the BBC. According to a study, people are more likely to read news from websites with which they agree and disagree. This is true even if the language model of the news item is comparable to the individual’s political beliefs.[10] It impacts people who read the news on websites that publish opposing ideas and viewpoints. The researchers reached this conclusion after doing some search engine research. Because more people become interested in the news after being provided with a broader range of outcomes, the results they read in the future will need to be more diversified to hold their attention. Yom-Tov and colleagues identified this during their investigation.
Subject discovery and sentiment analysis become critical when a query offers information on all elements and views on those aspects. Contrastive Opinion Modeling (COM) is the name that Fang and his colleagues gave to their initial study subject recommendation in the field of opinion mining.[5] According to Fang and his colleagues, COM is responsible for delivering several perspectives on the same query issue throughout a database of text collections. This concept is related to a collection of text collections while a certain query is being run. To establish the extent of disagreement, the authors employ methods other than the Jensen-Shannon divergence, which compares individual topic-opinion distributions.[19] We concluded that while topic discovery is useful when comparing search engines, it is insufficient for establishing whether search engines are trustworthy. We chose emotion over subject information to allow for a comparison of the viewpoints supplied by different search engines and an assessment of the degree to which the texts that were retrieved demonstrated a feeling of fairness. There are multiple inquiry diversification techniques depending on how individuals feel about a particular issue.[1] In addition, a variety of various accuracy levels are used in an assessment of emotion categorization. Naveed and his colleagues’ work, which was completed concurrently with this paper, is mentioned in another piece of literature titled “Feature Sentiment Diversification of User Generated Reviews: The FREuD Approach”.[8]
Furthermore, the findings of Kulshrestha and colleagues were compared to our own in terms of the various types of bias observed in search engines.[7] The first two on the list combine all kinds of prejudice, including bias at the input or source level, bias in ranking systems, and bias at the output level. They provided a variety of criteria to quantify and categorize the many types of bias that may be discovered in tweets about politics. This was done to distinguish the biases from one another. There are four important areas in which our work differs from that of others. We did not conduct a complete analysis using tweets; instead, we relied on news stories that give organized textual information. Instead of utilizing Twitter as our major source of information, we utilized two commercial search engines for the third time and compared the viewpoints offered by each of them rather than simply looking for bias. This was done for various reasons, the most important being that political prejudice cannot be assessed. It is also conceivable that bias was introduced by the inquiry, especially if the investigation favors one side of an issue or perspective over another.[20–22] This, in addition to the skewed results provided by the algorithms used by search engines, contributes to the problem. The user’s previous notions are typically reinforced using terminology that benefits the user. In terms of phenomenology, phrases like “Obama was born in Kenya,” “gun control,” and “gun rights” can indicate a bias toward one side or the other of an issue. When doing our research, we will also consider the likelihood of query bias. Because internet users cannot distinguish between fake and real information, it is becoming increasingly common for them to rely on fraudulent rather than genuine information. This trend is expected to continue. As a result, what is known as a “filter bubble” is becoming a source of concern for the whole community. When a person only reads information that is relevant to them because search engines and recommendation systems have optimized it for them, this is referred to as “user-centric optimization,” and it falls under the topic of web design.
The phenomenon is known as “filter bubbles” and can skew information accessed online. Internet users are vulnerable to a tendency known as “confirmation bias,” which can lead to their receiving erroneous or low-quality information. “Confirmation bias” is defined as “unconsciously favoring information that supports one’s position or belief “.[12] This is what the expression “unconscious information bias” refers to. As a result, the consumer is quite excited about the items. A search on the internet is performed so that user X may assess whether “product Y is good for dieting.” Because Y is a product that significantly influences the consumer, we are doing this to educate them better. As a result, X will focus on data that supports product Y, even if the findings contain information that contradicts product Y. This is because the consumer has a high level of trust in the information offered by product Y. As a result, the user is more inclined to accept misleading information since it is compatible with their previous preconceptions; as a result, the user is more likely to believe false information. People who research on the internet are more likely to succumb to confirmation bias. People risk succumbing to confirmation bias when they perform internet research on topics such as politics, food, clothing, and shelter. Many people’s social lives may be disturbed. A person who, for example, searches the internet for information on a range of health-related issues may be susceptible to confirmation bias.
Despite the extensive use of search engines and their critical role in providing information, there is a research gap in understanding the extent of bias and neutrality in search engine results. While some studies have explored this topic, there remains a need for a comprehensive investigation that considers both the algorithmic and user-driven aspects of bias.[23–26] Additionally, more research needs to delve into the implications of confirmation bias on user behavior when searching for information online. The existing literature largely focuses on political bias, leaving other domains unexplored.
Methods for Personal Analysis of the Relative Importance of Two Searches
The TextBlob handles punctuation automatically, so we don’t need to preprocess the papers before conducting document-level sentiment analysis. TextBlob makes this value available to us. Compared to the sentence-level analysis, this section of the Research is significantly more comprehensive.
Complete concept explanations in phrases
Sentiment analysis is conducted at the sentence level, so a text is not analyzed as a collection of sentences but as individual sentences. To begin, we will utilize TextBlob to identify the polarity value associated with each sentence in the supplied text. To calculate an emotion score for the submitted content, we first added the polarity values of all the phrases and then divided the sum by the total number of sentences. This allowed us to conduct a more in-depth examination of the materials presented. To put it another way, when TextBlob does sentence-level analysis, it examines each phrase as if it were a new document. Even though the scores for documents and sentences are derived in two different ways, you will only need to use one polarity number when comparing the results of two different search engines. This is because you will only be comparing the polarity of each document.
Induction of Behavioral Changes
To build a logically consistent comparison, one must consider the relationship between a text’s semantic orientation. To put it another way, the more liberal the paper’s stance, the more likely it will be a pro-abortion text, and the same is true for the chance that a pro-Brexit article will be a pro-Brexit piece. When comparing documents, the “conservative/liberal” sentiment score of a document may only be used if both the sentiment of the document and information on its problematic issue are considered. Using the transformation technique, it is feasible to draw coherent parallels between various critical situations. As a result, to maintain consistency, we must apply a transformation to one of these texts. We change the polarity value of the Brexit paper by removing one and multiplying the result by one. Our grading system gives more weight to liberal papers, implying that the more significant a document is for the topic of the contentious question, the more liberal the document is. As a result, the polarity of an unfavorable document can be reversed if it supports a liberal perspective on the subject. Because transformation occurs after identifying the polarities of the document, the technique for converting a document or an entire phrase is the same. There is no difference in the method for either.
Metric Units That Are Comparable
We compare the documents returned by search engines using three distinct sentiment-based measures. In this case, TextBlob’s emotion polarities are used as the major criterion right away. The following recommendation is to use sentiment data as well as rankings in conjunction with Normalized Discounted Cumulative Gain (NDCG) Senti measure. The average correctness is a final criterion that favors ranked lists with more frequent relevant documents for a contentious issue. To begin, we will compare two search engines using sentiment ratings from TextBlob. It returns sentiment ratings for documents and phrases, which we then use to get the average polarity value for each document. This procedure is described in the preceding section. We are just interested in the document sentiment scores for comparison because we are comparing sentiment analysis scores from two distinct levels and may need to change some of the polarity values. This metric should make it easy to compare the document sets returned by different search engines. To address this issue, we created an improved version of the frequently used NDCG measurement. The NDCG-Senti is a version that combines ranking data with sentiment analysis. The NDCG approach, considered a classic, is the foundation for our ranking-sentiment measure. The relevance scores are substituted in the computation for the sentiment scores obtained by the NDCG-Senti algorithm. Our team developed a modified version of the NDCG scoring algorithm. This technique, in addition to the standard NDCG calculation, considers the gathered document sets from search engines, allowing for a more realistic comparison of the scores. This second suggested measure is obtained from the polarity data in the original papers, which has been converted and normalized before calculation. The TextBlob sentiment ratings are processed and normalized before being employed in the computation to get the NDCG-Senti scores.
Average Precision
We prefer and support the inclusion of liberal texts towards the bottom of the list; the frequency with which they occur in our framework determines accuracy in the manner we’ve explained. We used the definitions of the projected value of the average precision formula provided in[2] to carry out the computation. A sense of justice and equality Search engines are rated primarily using two major criteria in our evaluation method. We conclude that they are equivalent if both produce objective conclusions (i.e., there is no bias) and if the groups of articles that they return for the problematic issue are sentimentally comparable. Because we require a mathematical theory of fairness to analyze search engine results, this may make the procedure more difficult than necessary. Furthermore, a baseline meter for comparing the two should be devised. This will allow the development of a fairness meter. When search engine results are compared to appropriate comparison metrics, such as NDCG-Senti, average accuracy, or average sentiment polarity, it is possible to determine if search engine results depart considerably from a fair collection of content.
TextBlob’s sentiment ratings should be in the [-1,1] range; hence the value of this metric should be adjusted to zero to produce a balanced collection of documents. This procedure enables us to create a fair version of NDCGSenti. It would help if you remembered this because we assign a score of 0 or 1 to each item on a list of 10 papers we analyze. This explains why sentiment scores are produced using normalized sentiment ratings, which can vary from 0 to 1, and why these ratings are utilised to construct sentiment scores. The NDCG-Senti score generated by this technique may be used to judge whether a document collection is fair. This phase used the same 50 document lists that were used in the previous section to establish the fair average accuracy.
Standard Operating Procedures for Normalization
We can eliminate any negative emotion ratings that may have been present in the data by applying the min-max normalization approach to the polarities and mapping them into the range [0, 1]. We calculate the lowest and highest values for each query by using the polarity values gathered for the document set associated with each query. After that, we normalise the polarity values by using the lowest and highest values and obtain non-negative ratings for the submitted articles. To determine the overall correctness of a set of papers, the following information is considered: Both chia and quinoa were coined as search phrases before they were ever used in actual internet searches. This is how search results can be arranged. According to one idea, the healthiest foods today are chia seeds and quinoa. These are foods that are good for your health and should be eaten regularly. Participants were tasked with conducting online research to see how a certain food component influenced weight loss programs.
Examining the techniques, the following describes how the study on online platform users has been carried out, the research process and when the follow-up survey is completed. The participants’ initial step was to register for the event on the Lancers.jp website, which they did online. Participants were then sent to a website where they could take part in the user study. Participants were randomly assigned to one of two conditions, depending on the situation: one with or without the invocation of confirmation. What they attempted to learn, as well as what they already knew. Following that, guests were provided with some event background information. An example of an introduction is as follows: Information about “chia seed.” Consider that you recently went to the doctor at your place of employment for a medical exam. You voiced your discontent with the situation as soon as you became aware of it.
A high triglyceride level in your blood indicated that you were close to being classified as obese. You chose to embark on a weight-loss journey because you didn’t want to spend your twenties the same way you spent your thirties. After a time, one of your company’s health-conscious employees questioned how he had maintained such superb physical condition for so long. That’s when you discovered he kept his physique in such good shape by eating “chia seeds,” a nutrient-dense meal, every day. As a result, he could keep his body in good shape.
On top of that, your buddy provided you with the following information on the topic of debate here: “chia seeds.” In addition to the material just presented, we supplied the following resources as takeaways to those in attendance. It may be used to influence people’s opinions on the search topic and find individuals who share those opinions.[6] Those who were members of the biased group obtained favorable ratings in this situation. There is some uncertainty over the function of chia seeds, often known as quinoa, in the weight loss process (i.e., confirmation bias). We told them to join a group responsible for distributing lies like the ones they’ve manufactured. Ingested omega-3 fatty acids in chia seeds (also known as quinoa), help break down the body’s stored fat. Some of the visuals used to persuade participants that chia seeds may help them lose weight. It was also stated that “non-native celebrities enjoy it, and the general public is embracing it at a rapid speed.”
A variety of data was provided to people in the critical group to persuade them to conduct more research. They were advised that “more than half of all health material on the Internet is incorrect.” Therefore, we exhibited a variety of figures and visuals to encourage visitors to be cautious while performing web searches and to think critically in general. Following an introduction to the exercise, the participants were given a questionnaire to complete. A pre-questionnaire was used to gather background information and viewpoints on the search query. “Chia seeds, often known as quinoa, may surprise you,” we questioned participants who took part in this study. Participants gave their responses to the question. Please rate the following items on a scale of one to seven: 1. a complete lack of information; 2. a lack of comprehension; 3. a lack of understanding; 4. a wealth of knowledge and expertise; or 5. a wealth of knowledge and expertise We asked the participants if quinoa (or chia seeds, for that matter) qualified as a “superfood,” and we got a variety of responses: first, there is no impact; second, there is inadequate efficiency; third, it is effective to a degree; and fourth, it is exceedingly efficient. Before commencing the workouts, participants were asked to complete a preliminary questionnaire. This exercise involves reading the instructions and browsing the internet for pertinent information. Follow the steps in the following paragraphs to find out if chia seeds are helpful for you. Is it possible that quinoa, for example, may help in the fight against obesity? Begin by selecting the “Show Search Results” button below, and you’ll be able to see all your search results.
RESULTS
This data was collected from participants as they went about their mission so we could observe how many ways they took. It would help them if they took time to read over the pages. The amount of time and the number of times the results were checked throughout the search, the study’s focus was on how much time participants spend looking at SERP results. The amount of time spent on a single page during a single “session” is referred to as session time. As more individuals use search engines, the number of people who click on the results increases. Click-throughs are the search results that each participant chose. These results include the URL, a description of the website’s content, and where the page appears in the search results list. When concluding log data, it should be remembered that these findings were based on past studies on online search behavior.
The Hypothetical Framework
We projected that confirmation bias would be the primary driver of the product’s success, avoiding confirmation bias by surfing the internet for health-related information with less attention than the average internet user. According to this argument, the use of search engines may be related to the problem of confirmation bias. The longer a person remains on a search engine, the more probable they will return to the site. Click-through rates from search engine results pages and average time spent on a website Both page views and overall session time have recently decreased. Furthermore, consumers will notice that the maximum click depth is far lower than what they are used to. Users are unlikely to appreciate metrics such as “availability of facts and proof “ and “mastery of knowledge” offered by “providers” assessment due to confirmation bias. These metrics are used to determine how well a person recalls information.
The inability to use these abilities is due to a lack of analytical capabilities. Preconceived notions can obstruct a person’s ability to think properly. As a result of its potential to teach individuals about bias and health literacy, we found that the internet significantly influences people’s ability to critically examine health-related issues. Confirmation bias is a cognitive bias that makes it harder for people to develop meaningful health literacy while using internet platforms. Despite an increase in page views, the amount of time spent on each session is decreasing.
In contrast to prior research that failed to account for the issue of confirmation bias, it was demonstrated that as the maximum click depth grew, the number of clicks dropped. Previous research did not account for confirmation bias, resulting in contradictory results shown in Table 1. In this part, we’ll review the analytical findings from the search results.
Parameter | High | Low | p-Value | ||||
---|---|---|---|---|---|---|---|
B | C | B | C | Health literacy score | C | I | |
SERP Processing Time (second) | 83.8 (93.5) | 74.0 (79.9) | 79.0 (77.4) | 73.2 (76.7) | 0.49 | 0.99 | 0.36 |
Search session time (second) | 394.8 (404.8) | 359.2 (364.2) | 399.5 (289.8) | 393.3 (378.3) | 0.42 | 0.64 | 0.45 |
Dwell time per webpage (second) | 78.5 (62.9) | 69.4 (74.4) | 62.9 (46.7) | 65.6 (74.6) | 0.39 | 0.24 | 0.63 |
Number of page views | 4.87 (4.86) | 4.52 (2.96) | 5.99 (3.76) | 6.35 (5.50) | 0.34 | 0.99 | 0.39 |
Maximum click depth | 26.7 (38.2) | 29.7 (36.0) | 35.8 (39.2) | 28.3 (32.4) | 0.33 | 0.98 | 0.42 |
Belief change | 0.68 (0.89) | 0.82 (0.92) | 0.90 (0.88) | 2.00 (0.92) | 0.59 | 0.39 | 0.97 |
It was an evaluation that considered the results of the pre-and post-surveys and the observed behaviors, reliable and unreliable information percentage shown in Figure 1. We assumed that “quinoa” and “chia seeds” would be interchangeable. We decided to investigate this further. We used a variance analysis to investigate the relationship between the Health Literacy Score (eHEALs) and whether an internet search revealed a lack of confirmation bias (bias condition). A high or a low eHEALS score will activate the eHEALS factor (high group), but having a low eHEALS score does not automatically imply a poor quality of life (low group).
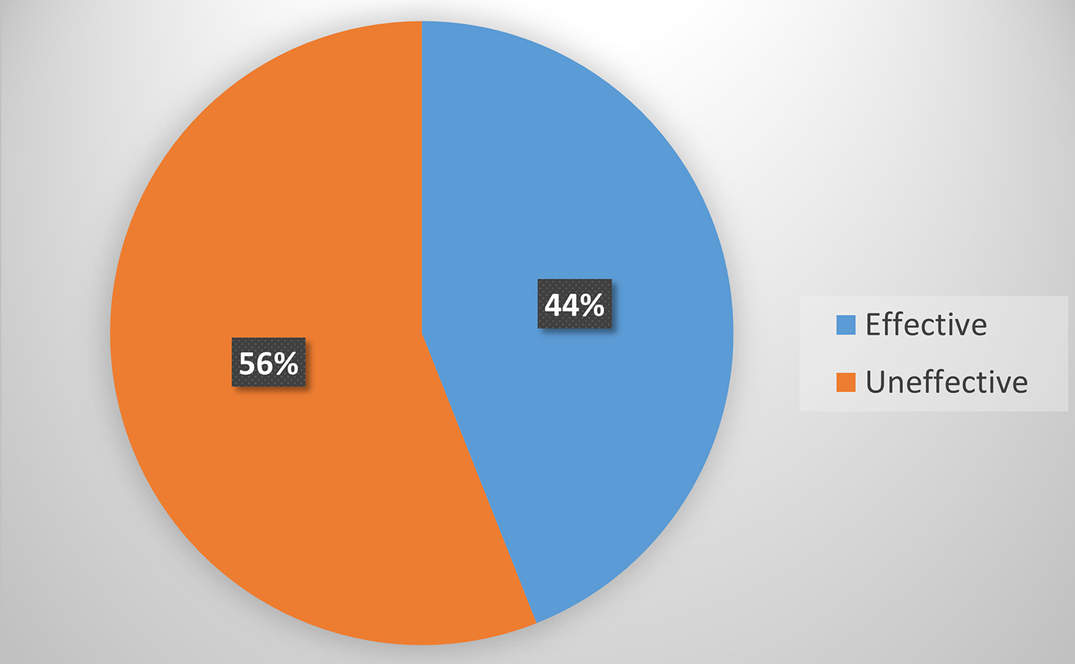
Figure 1:
Count of the Reliable and Unreliable Information.
As a result, the statistical significance test results may be easily changed using the NDCG-senti and the average accuracy score for each participant. To obtain an overall evaluation for the tasks, an average computation of the emotion scores from all documents is required. We’ll only have one score till then. We shall be able to establish the usual polarity of the reported feelings using this procedure. A score can be made in this manner: the web search response effectiveness percentage is 44 percent which is shown in Figure 2.
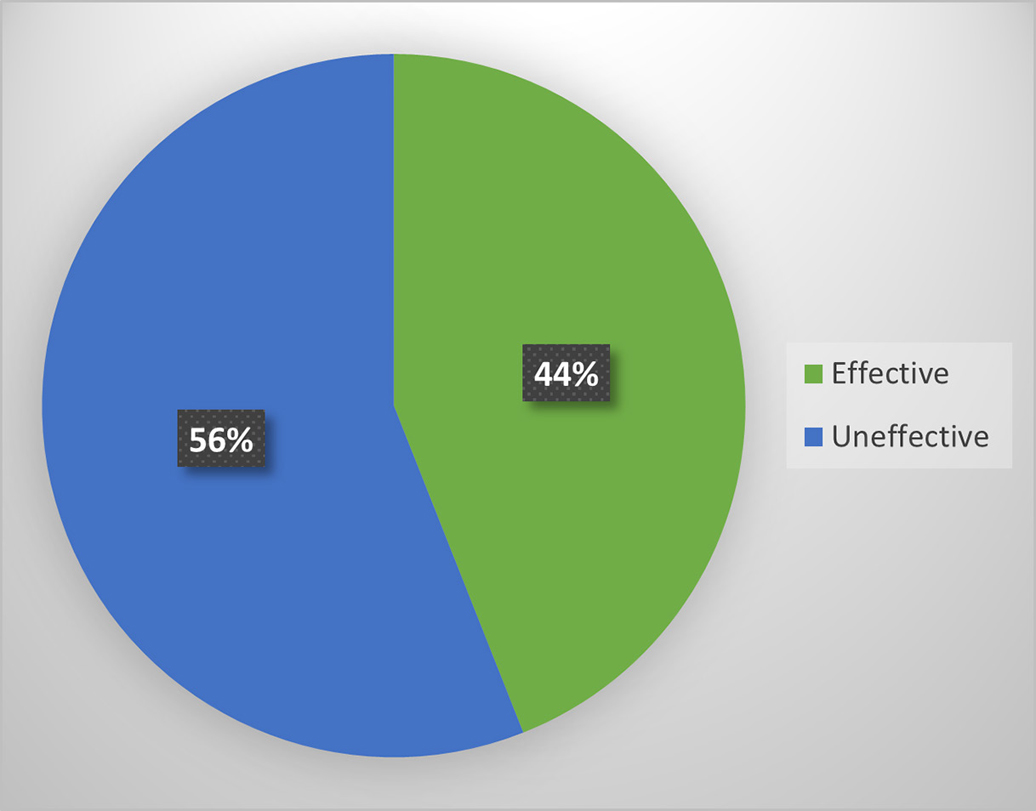
Figure 2:
Web Search Response.
Participants who performed poorly had eHEALS scores lower than the median (21,4), whereas those who performed well had eHEALS scores higher than the median (23,0,0). (The answer was 21.4). Based on their level of confirmation bias, participants were separated into two groups (the non-biased group). Each category has its own set of distinguishing characteristics (the critical group). Because we did not investigate whether the data collected in this experiment was normal, we used the alignment rank transformation to complete our nonparametric analysis of variance. Visitors spent an average of 4.1 min on the search results page. For this study, we tracked how much time each participant spent going through search engine results pages and analyzing the information. eHEALS (p=0.38), the bias condition, and their interaction were not found to be statistically significant in this investigation (.89 for the low group and 0.25 for the high). The low group had a mean score of 72.7, whereas the high group received a score of 63.1; the low group’s score was statistically significant at p =0.25. As seen in the Table 2, 4.2 sec were spent searching. We also timed how long it took everyone to find the object. The total time spent looking must include SERPs and any other sites that may be linked to the search. We had a brief discussion about it earlier. There was also no noticeable difference in the final findings between the interaction and the bias component.
Perspective | High | Low | P-Value | ||||
---|---|---|---|---|---|---|---|
B | C | B | C | eHEALS | Condition | Interaction | |
Existence of Data | 2.91 (0.94) | 3.5 (0. 88) | 2.4 (0.91) | 2.4 (0.91) | 0.49 | 0.94 | 0.76 |
Novelty of Information | 3.6 (0.94) | 3.5 (0.97) | 2.7 (0.98) | 2.9 (0.94) | 0.42 | 0.62 | 0.45 |
Openness of Contents | 4.4 (0.88) | 4.35 (0.74) | 4.2 (0.69) | 4.4 (0.75) | 0.39 | 0.65 | 0.6 |
Reliable Sources | 3.9 (0.85) | 3.8 (0.86) | 3.10 (0.92) | 2.8 (0.87) | 0.34 | 0.78 | 0.39 |
Appearance of the Website | 3.7 (0.99) | 3.4 (0.91) | 3.5 (0.93) | 3.6 (0.92) | 0.33 | 0.48 | 0.42 |
Topic Coverage | 4.4 (0.77) | 4.2 (0.92) | 4.1 (0.78) | 3.9 (0.73) | 0.59 | 0.89 | 0.97 |
While answering how long it takes to see a single web page, we compared each participant’s total time spent with the average time spent accessing the internet. This provided us with a decent estimate of how much time each participant spent on average in Table 2. This is all we can say now. The average was 4.4, with a high of 66.4 and a low of 54.5. In other words, the P-values for eHEALS, the bias condition, and the interaction were all 28. This has already been witnessed by many people. To make fair judgments of each person’s efforts, we needed to know exactly how much time and effort each participant put into acquiring the data. There were considerable variances in the page views and the bias condition factor, but they were not statistically significant. Even though the p-value for the interaction was only 28, the researchers decided that the bias condition was significant 99.9% of the time. One is only allowed to make this many clicks while exploring a single depth level. “Maximum click depth” measures how far down search results pages participants travel to check whether they are paying attention to items lower in the page’s rank. Maximal depth informs us whether participants were paying attention to lower-level material (SERPs).
Figure 3 and Table 2 reveal a maximum click depth, with one group biased and the other critical. Lower levels of health literacy were associated with a lack of interest in deeper results and analysis of people with lower levels of health literacy. Confirmation bias may improve health literacy just a bit. It is important to keep in mind that if this is the case, the low skewed group will have a greater average maximum click depth than the crucial group (24.7 vs. 17.2). People’s differing perspectives on the problem might account for 4.6% of the difference in the numbers. It was necessary to distinguish between pre-existing and post-existing attitudes to determine how much the experimental search task impacted the participants’ pre-existing beliefs. The Table 2 has a 4.7. A mental state that compels one to conduct extensive research and analysis. Our findings show a link between a person’s search and browsing behavior and their commitment to conscientious information-seeking. We discovered this by looking at people’s search and browsing habits.
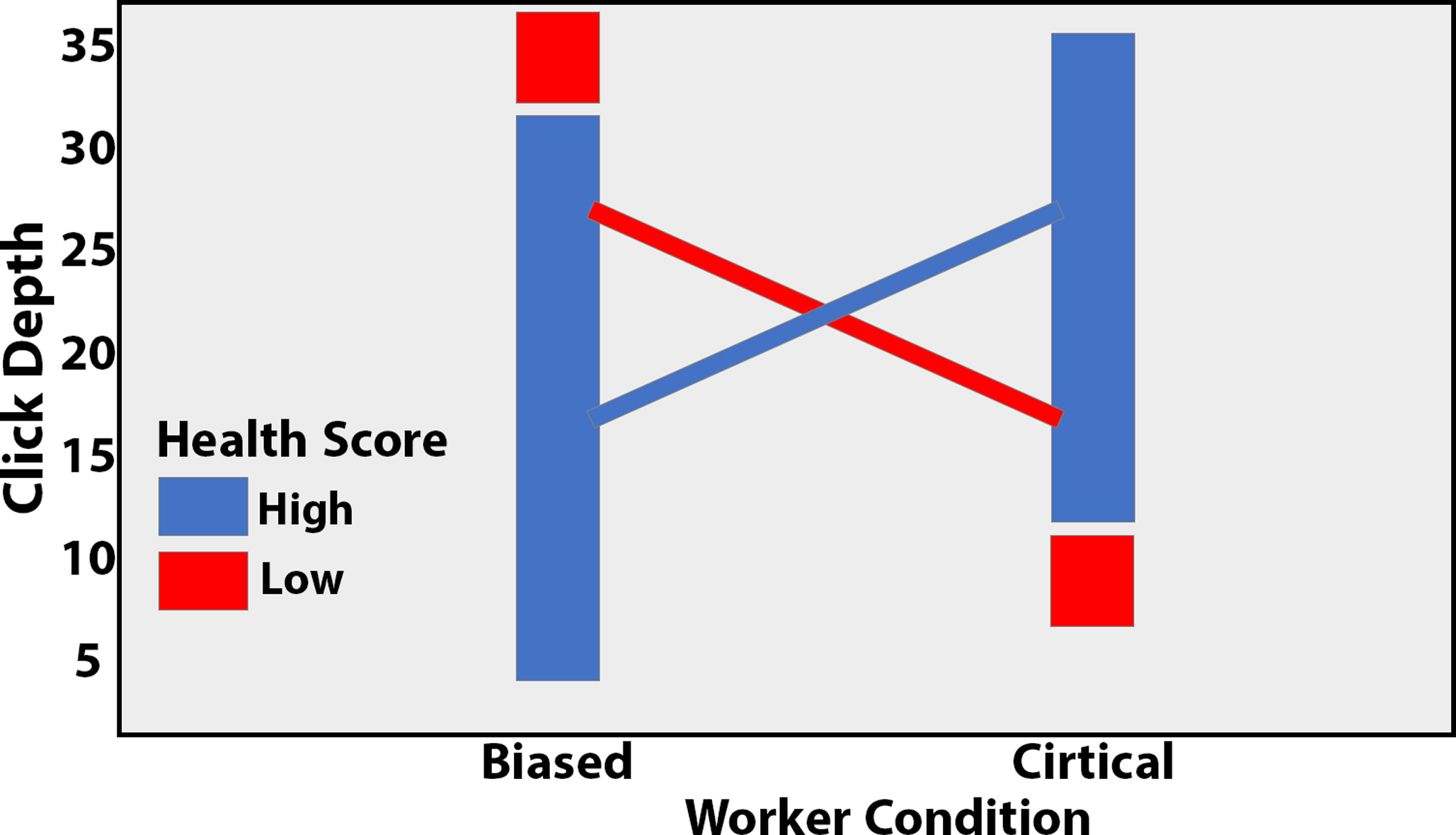
Figure 3:
Error Bars Biased and Critical Click Depth.
In either the bias condition or the interaction, there were no statistically significant changes (the bias condition had a p-value of 93, while the interaction had a p-value of 65). Regarding “the existence of evidence and proof,” there was a considerable difference between those in the high and low groups, regardless of whether there was a confirmation bias. It was discovered that “the freshness of the information” had no statistically significant influence on bias or the interaction between bias and bias. This is correct (the bias condition was 0.53, and the interaction was 0.34). The eHEALS component, on the other hand, demonstrated a statistically significant difference (p less than 001). Participants with a high relevance score were more worried about “information freshness” than those with a low significance score. The eHEALS component, on the other hand, demonstrated a statistically significant difference (p less than 001). A higher score on the importance of avoiding confirmation bias did not affect the outcome.
Furthermore, no statistically significant changes were discovered during the investigation. concerning the bias condition as well as the component eHEALS. In this case, we’re talking about the website’s “presentation” or, to put it another way, its “look.”
CONCLUSION
This study aimed to examine the online behaviors of internet users who rely heavily on search engines. Participants were presented with information that challenged their beliefs on the topic they were searching for, resulting in two distinct groups: one with confirmation bias and one without. We gained insight into their pre-search activities by analyzing their search and browsing histories. The findings revealed that individuals with higher health literacy demonstrated a more substantial confirmation bias and preferred highly ranked search results. These individuals also tended to rank higher on search engine results pages and were better able to understand healthcare practitioners’ recommendations. On the other hand, those with lower health literacy had difficulty applying the information they found due to confirmation bias. This study aims to understand further how confirmation bias influences online behavior.
Cite this article
Mansour RF, Fatouh AH. Measurement of Bias in the Contents of Web Search for Health Information Retrieval. J Scientometric Res. 2023;12(3):621-30.
References
- Aktolga E, Allan J. Sentiment diversification with different biases. 2013:593-602.
- Aslam JA, Yilmaz E, Pavlu V. The maximum entropy method for analyzing retrieval measures. 2005:27-34.
- Epstein R, Robertson RE. The search engine manipulation effect (SEME) and its possible impact on the outcomes of elections. 2015;33(2015):E4512-21.
- Epstein R, Robertson RE, Lazer D, Wilson C. Suppressing the search engine manipulation effect (SEME). 2017;1(2017):42
- Fang Y, Si L, Somasundaram N, Yu Z. Mining contrastive opinions on political texts using cross-perspective topic model. 2012:63-72.
- Koutra D, Bennett PN, Horvitz E. Events and controversies: Influences of a shocking news event on information seeking. 2015:614-24.
- Kulshrestha J, Eslami M, Messias J, Zafar MB, Ghosh S, Gummadi KP, et al. Quantifying search bias: Investigating sources of bias for political searches in social media. 2017:417-32.
- Naveed N, Gottron T, Staab S. Feature sentiment diversification of user generated reviews: The freud approach. 2013
- Aladeen H. Investigating the Impact of Bias in Web Search Algorithms: Implications for Digital Inequality. 2023 [Google Scholar]
- Wessel M, Horych T, Ruas T, Aizawa A, Gipp B, Spinde T, et al. Introducing MBIB-the first media bias identification benchmark task and dataset collection. 2023:2765-74. [Google Scholar]
- Bateman S, Teevan J, White RW. The search dashboard: how reflection and comparison impact search behavior. 2012:1785-1794. [CrossRef] | [Google Scholar]
- Clarke CLA, Agichtein E, Dumais S, White RW. The Influence of Caption Features on Clickthrough Patterns in Web Search. 2007:135-42. [CrossRef] | [Google Scholar]
- Ennals R, Trushkowsky B, Agosta JM. Highlighting Disputed Claims on the Web. 2010:341-50. [CrossRef] | [Google Scholar]
- Joachims T, Granka L, Pan B, Hembrooke H, Radlinski F, Gay G, et al. Evaluating the Accuracy of Implicit Feedback from Clicks and Query Reformulations in Web Search. ACM Trans. Inf. Syst. 2007;25(2):7-es. [CrossRef] | [Google Scholar]
- Kahneman D. Thinking, fast and slow. Macmillan. 2011 [CrossRef] | [Google Scholar]
- Kong HK, Liu Z, Karahalios K. Trust and Recall of Information across Varying Degrees of Title-Visualization Misalignment. 2019;346:13 [CrossRef] | [Google Scholar]
- Kusumi T, Hirayama R, Kashima Y. Risk Perception and Risk Talk: The Case of the Fukushima Daiichi Nuclear Radiation Risk. Risk Analysis. 2017;37(12):2305-20. [CrossRef] | [Google Scholar]
- Le H, Maragh R, Ekdale B, High A, Havens T, Shafiq Z, et al. Measuring Political Personalization of Google News Search. 2019:2957-63. [CrossRef] | [Google Scholar]
- Häußler H, Schultheiß S, Lewandowski D. Is googling risky? A study on risk perception and experiences of adverse consequences in web search. Journal of the Association for Information Science and Technology. 2023 [CrossRef] | [Google Scholar]
- Neumann T, De-Arteaga M, Fazelpour S. Justice in misinformation detection systems: An analysis of algorithms, stakeholders, and potential harms. 2022:1504-15. [CrossRef] | [Google Scholar]
- Gao R, Shah C. Toward creating a fairer ranking in search engine results. Information Processing and Management. 2020;57(1):102138 [CrossRef] | [Google Scholar]
- Kaur P, Pannu HS, Malhi AK. Comparative analysis on cross-modal information retrieval: A review. Computer Science Review. 2021;39:100336 [CrossRef] | [Google Scholar]
- Gomroki G, Behzadi H, Fattahi R, Salehi Fadardi J. Identifying effective cognitive biases in information retrieval. Journal of Information Science. 2023;49(2):348-58. [CrossRef] | [Google Scholar]
- Draws T, Roy N, Inel O, Rieger A, Hada R, Yalcin MO, et al. Viewpoint diversity in search results. 2023:279-97. [CrossRef] | [Google Scholar]
- Krieg K, Parada-Cabaleiro E, Medicus G, Lesota O, Schedl M, Rekabsaz N, et al. Grep-BiasIR: A Dataset for Investigating Gender Representation Bias in Information Retrieval Results. 2023:444-8. [CrossRef] | [Google Scholar]
- Suzuki M, Yamamoto Y. Analysis of the relationship between confirmation bias and web search behavior. 2020:184-91. [CrossRef] | [Google Scholar]