Contents
ABSTRACT
Foresight methods have been used by governments to reduce the margin of error in decision-making, but there is no golden rule for foresight activity; rather, several methods are combined to support decision-making. This article proposes an index number to support Technology Foresight in the field of Pharmacology/Pharmacy. The index number was formed by the relationship between bibliometric and human resources variables. First, Principal Components Analysis was used to reduce the initial bibliometric variables proposed by literature. Finally, Data Envelopment Analysis was used to calculate the number of Decision-Making Units (DMU), which are the most prolific institutions in the study country. The study examined 12 DMUs with 2,744 human resources (100% with academic degree) and 1,515 with research category (55.2%) from these, 217 granted patents (17.1% cited patents) and 1,017 papers (92.3% cited papers) were obtained. A simple but robust index was obtained to support decision-making in Technology Foresight. The results obtained from DMUs affect the Technology Foresight Index due to some institutions with low levels of scientific and technological activity and others with many highly qualified personnel. Technology foresight should be performed periodically by governments to reduce uncertainty in the innovation process and to develop highly competitive technologies. In this sense, this index is reliable for decision-making in the field of pharmacology/ pharmaceuticals. It proposes a novel index relating bibliometric variables (output indicator) and human resources variables (input indicator) to foresee the scientific and technological development in the field of Pharmacology/Pharmacy at the national level. In addition, this study includes variables representing scientific (paper) and technological (patent) activity, as well as the impact of both at the international level.
INTRODUCTION
Since ancient times, society has made several efforts to know the future to anticipate it and prepare for its advent. This is the main reason why foresight and strategy are related to each other. This relationship is extrapolated to multiple disciplines such as organization, technology, public policies, research, and scientific-technical development and is applicable to decision-making at any entity such as business, academia, and government. Many countries realized that traditional planning methods had been insufficient to identify possible future scenarios and began to accept the idea of anticipating the future through prospective methods. Companies not only needed a glimpse of their future, but they also needed to know the possible technology options that coming up. Likewise, governments needed to foresee trends in different areas of knowledge to guarantee scientific and technological development.
The pioneering countries in using foresight to predict scenarios were the United States (USA) in 1937,[1] China and Japan in the late ‘50s and the early ‘60s.[2] In the ‘80s, France carried out its first exercises,[3] Netherlands[4] and Germany.[5] Finally, in the ‘90s, the United Kingdom,[6] Italy[7] and Austria[8] joined this practice. In Latin America, countries such as Colombia, Chile, Argentina, Venezuela, and Mexico have created initiatives that collect scientific and technological data through science and technology observatories. These countries have led strategic exercises in support of technology foresight using scientometric indicators to determine science and technology policies at the national level.[9,10]
The considerable uncertainty that exists in the management processes for scientific and technological development explains the use of technology foresight. Its implementation as a practice for foreseeing scenarios in current times is justified, among other aspects, by the development of technologies, the globalization of the economy, the overuse of natural resources and the distribution of financial resources. An articulation is required that integrates cross-cutting policies with sectorial ones beyond the scope of science and technology policies to achieve scientific and technological development with social inclusion. Social development, educational, economic, commercial, industrial, labour, and environmental policies are some examples to be considered. All of these should be part of an integrated approach where science, technology and innovation contribute to inclusive development.[11]
LITERATURE REVIEW
Technology Foresight provides a wide variety of methods for its analysis, but none of them is considered as the golden rule; quite the contrary, to perform a good foresight activity, several of these methods must be combined. Popper describes prospective activity in “Foresight Diamond” and includes several methods in each zone.[12] These methods can be randomly combined and some of them can even be useful in more than one stage. This combination of methods depends on the objective pursued, the information sources, and the accumulated experience in the continuous exercises of the prospective activity, as well as the subject matter or purpose to which it is applied. The present study is situated in Evidence zone, where modelling, literature review, patents analysis, time series analysis (Indicators/TSA) and bibliometrics are found. The latter is the tool used in the present research, which proposes an index number consisting of bibliometric variables (output variables) and human resources variables (input variables).
An index number is a statistical measurement of one or more magnitudes to measure their variations on time, i.e., it is the percentage of the quotient between reference period and current period, which makes it possible to compare different variables that may be expressed in different units of measurement.[13] The theory of index numbers has had many applications, among them in economy, where the consumer price index is calculated each year for the preparation of the basic basket of goods and services, considering a reference period.
Some studies use the index numbers theory during Technology Foresight exercises e.g., an environmental study,[14] a production study of multispecies fisheries,[15] a market study related to consumers demand of products;[16,17] even a study that allows measuring the disciplinary development of Library and Information Science.[18] In the case of the pharmaceutical industry, few studies have been published using the index number theory. The Malmquist index has been used to evaluate the evolution of the pharmaceutical industry in several countries: in Japan during the period 1982-2001,[19] in Spain during 1994-2000[20] and in Iran during 2000-2013.[21] None of previous papers use similar indicators to those applied in this study to anticipate the scientific and technological development in the field of Pharmacology/ Pharmacy. The proposed index, called Technology Foresight Index, foresees the development of the pharmaceutical industry, considering the efficiency of a set of Decision-Making Units (DMU). The Technology Foresight Index does not try in any way to evaluate these institutions, nor the pharmaceutical industry, but to support planning for a future near enough to be considered and far enough into the future to make the appropriate decisions in terms of scientific and technological policy. This index helps to reduce uncertainty in innovation processes, reduce the margin of error in decision-making, develop new technologies, provide sources of knowledge, as well as provide an exchange of points of view.
Consequently, the main objective of this study is the design of an index number based on bibliometric variables (output) and human resources variables (input) to support decision-making during the Technology Foresight activity. There is currently no measure to anticipate the development of the pharmaceutical industry, so the originality of this study is to obtain an index to support decision-making in the scientific and technological policy related to this industrial sector.
METHODOLOGY
The main objective of this study is to obtain an index number (Technology Foresight Index) to support decision-making in science and technology within research units. For this purpose, input and output variables are considered, since in this way it is possible to evaluate the balance between the human resources dedicated to the research and development activity (input variables) and the results expected to be obtained in terms of papers and patents (output variables).
The Technology Foresight Index is obtained from the relationship between human resources variables (input) and bibliometric variables (output). The first ones are obtained by a simple questionnaire applied to institutions and the last ones are obtained from Web of Science and their final selection is carried out by Principal Components Analysis (PCA). The validation of the Technology Foresight Index uses the most prolific institutions from the study country (Decision-Making Units) calculated by Data Envelopment Analysis (DEA).
All variables considered in this study have their theoretical support on Frascati Manual[22] and Santiago Manual.[23] These variables are used to assess science and technology development at national and institutional level or even on discipline performance that’s why these variables are conceptually feasible for the design of the index number. Among these variables are input ones, e.g., human resources with research category and academic degree and output variables, e.g., patents and papers, which include granted patent, cited patent, paper, cited paper, as well as the number of citations for both cases. Patents application was not considered because this variable shows technological activity but not the real capacity for technological development. Monetary budget was also not considered in the study because all institutions define and calculate it differently and this prevents the collection of accurate data. The selection of these variables coincides with other studies where input variables are the number of teaching staff and the number of R&D (research and development) staff, and output variables are papers and patents.[24–27]
The Principal Components Analysis (PCA) technique from XLTAT software[28] was used to reduce the number of bibliometric variables, considering that the main objective is to obtain a simple yet robust index. Two bibliometric strategies were performed for the study country to obtain data for the PCA technique. These strategies were performed in the field of Pharmacology/ Pharmacy from the Derwent Innovation Index database (strategy 1) and the Science Citation Index Expanded database (strategy 2) between 2000-2017.
The results obtained from both search strategies, i.e. the number of papers, cited papers and citations; as well as the number of patents, cited patents and citations for each year of the study (2000-2017) were arranged in a data matrix to be entered into the XLSTAT software. From these data, the correlation matrix was obtained, which showed that the data are appropriate for the application of the PCA technique by Kaiser-Meyer-Olkin Index (0.5). The principal factors were extracted from the correlation matrix by the “Principal Component” method. It could be observed from the Sedimentation plot that only two factors obtained Eigen values greater than one (2.3 and 1.7). This means that only two factors are relevant to summarize the initial variables. Finally, the configuration matrix showed the correlation between the variables and the factors based on factor loadings, which made it possible to select the variables with the highest factor loadings to be included in the Technology Foresight Index. The study retained four of the six variables originally proposed by literature[22,23] The four bibliometric variables finally selected were granted patent, cited patent, paper, cited paper because they had the highest factor loadings in the PCA.
The Technology Foresight Index (TFI) is based on index number theory.[13] This index is a composite index formed by the quotient between the Output Indicators Index (OII) and the Input Indicators Index (III) of each DMU. Mathematical equations of these indexes are shown below:
Where: OII: Output Indicators Index, OUTPUTxj: sum of projections of the output variables of each j institution for each i indicator, Ni: number of output variables.
Where: III: Input Indicators Index, INPUTyj: sum of projections of the input variables of each j institution for each i indicator, Ni: number of input variables.
Finally, the Technology Foresight Index is described below:
Where: TFI: Technology Foresight Index, OUTPUTxji: Output Indicators Index of each j institution for each i indicator, INPUTyji: Input Indicators Index of each j institution for each i indicator, N: total number of variables.
The procedure used to calculate the projection of each variable (output and input variable) is obtained from the sum of the values of each variable. Then, the average value of each variable and its percentage value with respect to the total number of each variable is calculated. The percentage difference of the average value is then calculated, i.e., the percentage value of each variable is subtracted from 100%. Next, the checking of the average value is calculated, where the percentage difference (previous result) is multiplied by the average value of each variable and divided by 100. Finally, the projection for the next year is calculated, i.e., the checking rate (previous result) is added to the average value of each variable.
Table 1 shows an example for the calculation of the granted patents projection of DMU 1.
Granted patent | Calculation and example |
---|---|
Total value | Sum of granted patents=70 |
Average value | Sum of granted patents/number of years=70/10=7 |
Percentage value | Average of granted patents * 100% /Average of total patents=7*100/9=77.8 |
Percentage difference of the average value | 100% – percentage of granted patents=100-77.8=22.22 |
Checking of the average value | Percentage difference of the average value * Average of granted patents / 100=22.22*7/100=1.56 |
Projection to next year | Checking rate of the average value + Average of granted patents=1.56+7=8.56 |
The projection of each study variable is calculated. Then, the sum of the projections of human resources variables (academic degree and research category) and bibliometric variables (patents and papers) are used to calculate the Input Indicators Index and the Output Indicators Index, respectively. The variable projection indicates the growth expectations for each variable i.e., the proportion by which each variable will increase.[18]
Data Envelopment Analysis (DEA) technique is then used to validate the Technology Foresight Index. This method evaluates the efficiency of institutions (DMUs), considering used resources (input variables) and obtained results (output variables).[29] Scientific literature proposes three decision rules for DEA, which are mentioned below:
First rule: n≥2 x (p+q)[30]
Second rule: n≥3x(p+q)[31]
Third rule: n≥(pxq)[32]
Where: p: number of human resources or input variables, q: number of bibliometric or output variables, n: number of institutions or Decision-Making Unit (DMU).
The most prolific institutions (DMUs) obtained from strategies 1 and 2 were selected to validate the Technology Foresight Index. These DMUs are used to obtain specific data for bibliometric variables and human resources variables. Data for human resources variables are obtained by means of a questionnaire applied to each institution (DMU). This questionnaire was sent by e-mail to the Human Resources Department and the Research and Innovation Directorate of each DMU selected for the study. The questionnaire is a data table (Annexure 1) where the total number of human resources with research category (category A, B and C) and academic degree (Doctor of Science and Master of Science) for each year of the study (2009-2018) is compiled. All data collected without exception were considered for the calculation of the human resources variables. On other hand, data for bibliometric variables are obtained using two new search strategies (strategy 3 and 4) in the field of Pharmacology/ Pharmacy from the same databases (Derwent Innovation Index and Science Citation Index Expanded) during 2009-2018.
Each of these variables (bibliometric and human resources) are used to obtain the Output Indicators Index (OII) and the Input Indicators Index (III) respectively, and in turn these indexes are used to obtain the Technology Foresight Index (TFI). Figure 1 below summarizes the workflow used in the study to obtain the Technology Foresight Index.
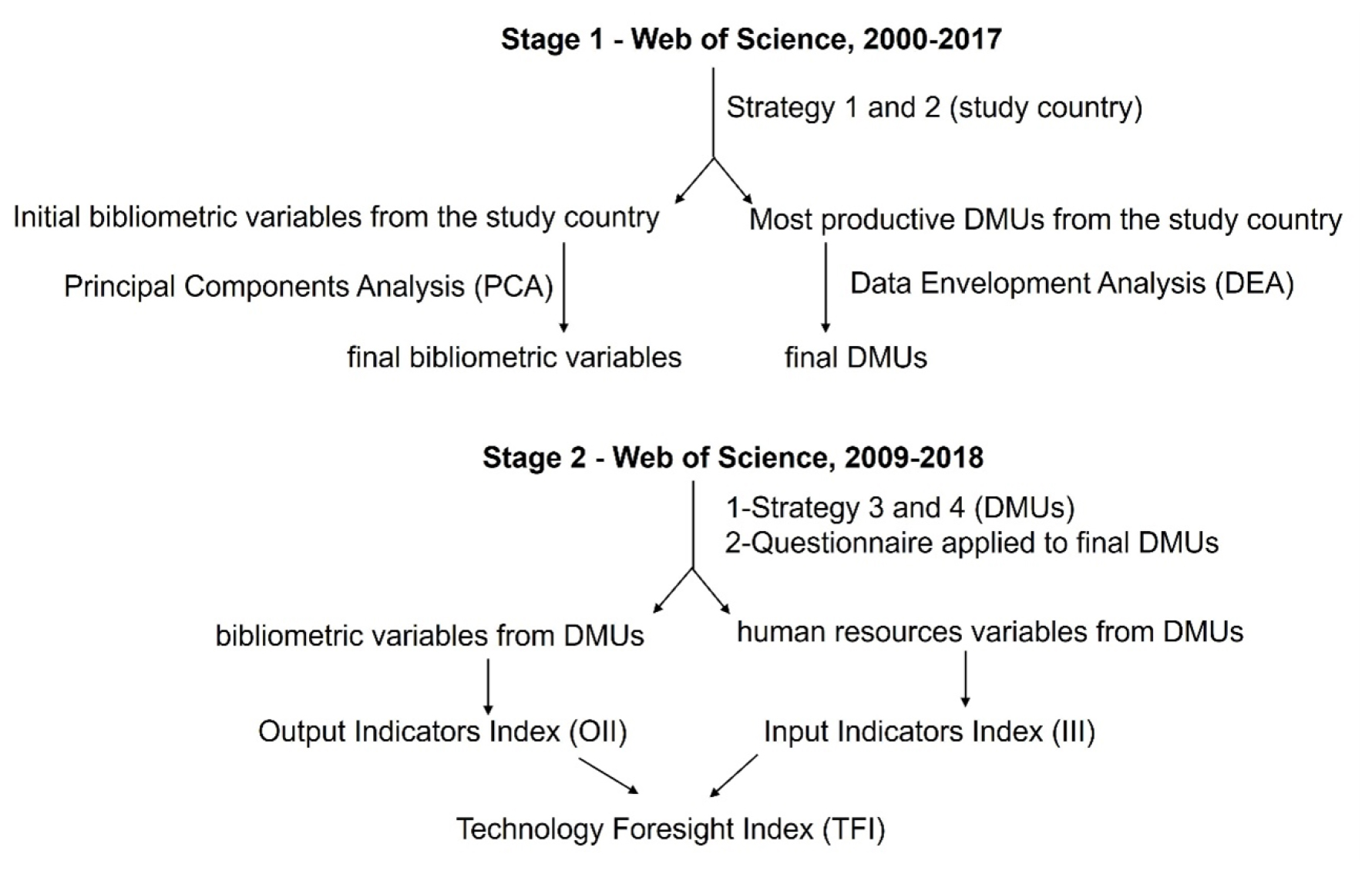
Figure 1:
Work-flow of the study to obtain the Technology Foresight Index (TFI).
Data collection
The search strategies used in the study are described below. All records were downloaded in Excel format to be analysed according to the corresponding phase. It should note that Derwent Innovation Index database creates a unique patent family for each invention to accelerate discovery and analysis.
Search strategy 1
Database: Derwent Innovation Index
Section: Chemical
Class: Pharmaceuticals
Period: 2000-2017
Search strategy 2
Database: Web of Science Core Collection
Web of Science Index: Science Citation Index Expanded
Period: 2000-2017
Document type: article
The strategies 1 and 2 goals are to obtain all data for the PCA technique and to identify the most prolific institutions in the field of Pharmacology/Pharmacy.
Search strategy 3
Database: Derwent Innovation Index
Section: Chemical
Class: Pharmaceuticals
Period: 2009-2018
Search strategy 4
Database: Web of Science Core Collection
Web of Science Index: Science Citation Index Expanded
Period: 2009-2018
Document type: article
The strategies 3 and 4 aim is to obtain DMU data to validate the Technology Foresight Index.
RESULTS
The scientific and technological output of the study country in Web of Science databases between 2000-2017
The total scientific and technological production and the most prolific institutions from the study country were retrieved by search strategy 1 and 2 in Derwent Innovation Index database and Science Citation Index Expanded database, respectively, during the period 2000-2017. Figure 2 shows the distribution of granted patent, cited patent, patent citations, paper, cited paper, and paper citations.
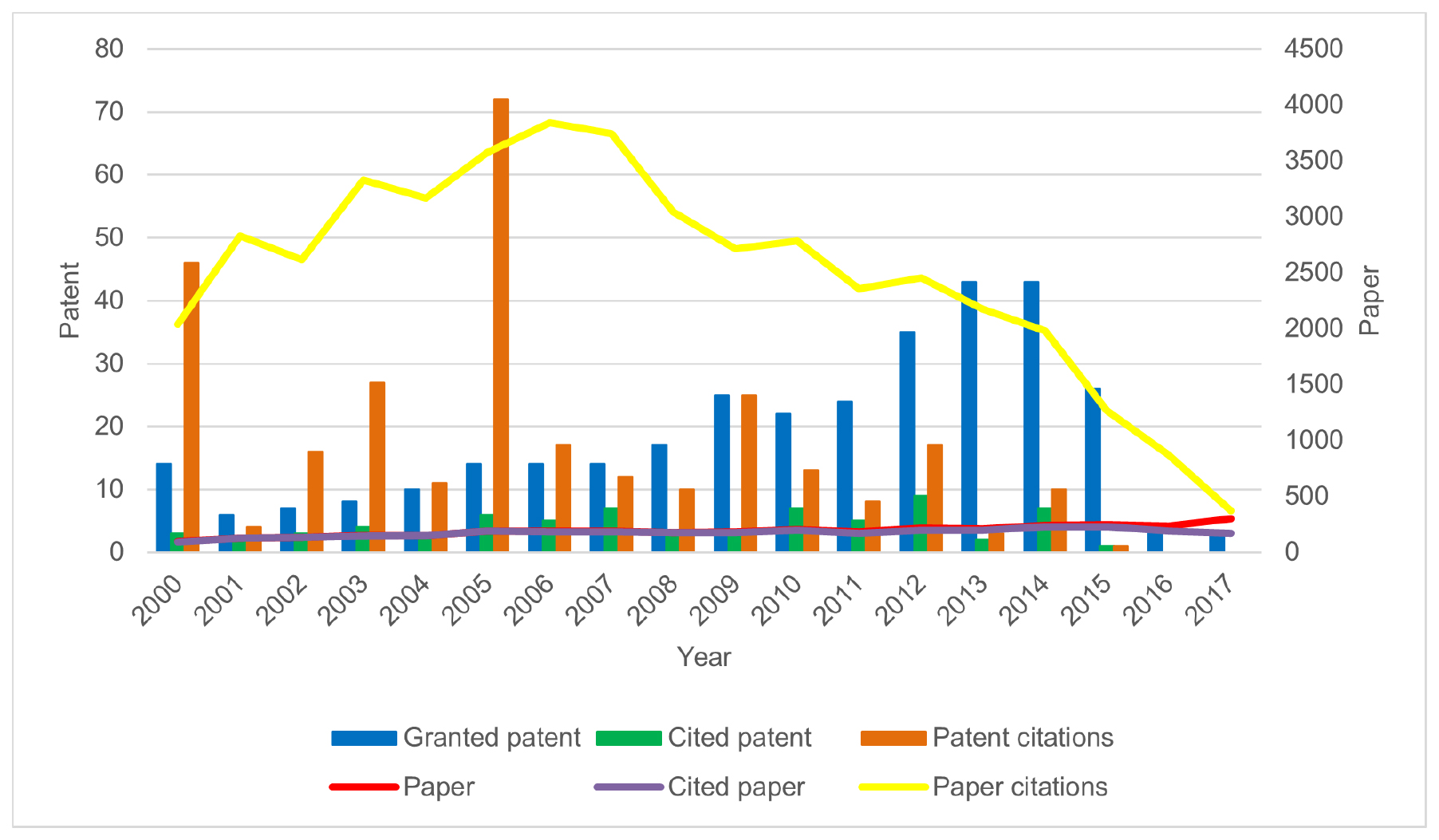
Figure 2:
Distribution of the scientific and technology output of the study country in Web of Science during 2000-2017.
A total of 328 granted patents, 70 cited patents and 292 patent citations from patents were retrieved from Derwent Innovation Index. The annual average values were 18 granted patents of which four were cited patents (22.22%) and 16 patent citations. There was a decrease in these indicators since 2015 (as of July 2018) because a patent to enter the public domain takes approximately 18 months, and to be granted from three to five years, which delays the receipt of citations.
In addition, 3,417 papers, 3,136 cited papers, and 45,145 paper citations from papers were obtained from Science Citation Index Expanded. The average values per year were 190 papers, 174 cited papers (91.57%) and 2,508 paper citations. The decrease in paper citations after 2013 is due to the citation window for the field of Pharmacology/Pharmacy is longer than seven years.[33]
Considering that 18 years (2000-2017) and all scientific (papers) and technological (patents) output related to the field of Pharmacology/Pharmacy coming from research and innovation institutions of an entire country were analysed, it was expected that the total amounts of papers and patents would be higher than the real ones. This situation gives us a preliminary idea about the scarce scientific-technological development in the country’s pharmaceutical industry. However, these results are not conclusive until each DMU is evaluated separately; considering the relationship between the number of human resources involved in the R&D activity and its results in terms of papers and patents (Technology Foresight Index).
Reduction of bibliometric variables and selection of Decision-Making Units
Principal Components Analysis (PCA) was used to reduce the six bibliometric variables. Data were retrieved from the strategy 1 and 2 (for the study country in the field of Pharmacology/ Pharmacy from Derwent Innovation Index database and Science Citation Index Expanded database during 2000-2017). Table 2 shows the configuration matrix obtained from XLTAT software.
Variables | F1 | F2 | Initial communality | Final communality | Specific variance |
---|---|---|---|---|---|
Granted Patent | 0.402 | -0.501 | 0.638 | 0.413 | 0.587 |
Cited patent | -0.126 | -0.896 | 0.598 | 0.819 | 0.181 |
Patent citations | -0.478 | -0.285 | 0.290 | 0.310 | 0.690 |
Paper | 0.897 | 0.034 | 0.883 | 0.806 | 0.194 |
Cited paper | 0.851 | -0.480 | 0.875 | 0.955 | 0.045 |
Paper citations | -0.592 | -0.558 | 0.765 | 0.662 | 0.338 |
The values in bold for each variable represent the factor for which the squared cosine is the largest. |
As shown in Table 2, variables were distributed in two factors. The analysis per variable indicates that granted patent, and cited patent had the largest factor loadings in Factor 2 and, the rest of variables (paper, cited paper and paper citations and patent citations) had the largest factor loadings in Factor 1. Factor loadings indicate the degree of correspondence between the variable and the factor, i.e. high loadings indicate that the variable is representative for the factor. Considering the above and taking into account that the objective of Principal Components Analysis is to reduce the number of bibliometric variables, the following variables are selected for Factor 1: paper and cited paper because they have the highest factor loadings in this factor (0.897 and 0.851, respectively) and the following variables are selected for Factor 2: granted patent and cited patent because, as shown in Table 2, these variables have a high correspondence with this factor (-0.501 and -0.896, respectively).The selected variables allow the evaluation of fundamental aspects for the Technology Foresight Index of each DMU examined, which are: scientific activity (paper), technological activity (patent) and influence in the scientific community (cited paper and cited patent).
This result was verified by the percentage of factor loadings (66.09%) shown in Figure 3 and corroborated that the most representative variables for the design of the Technology Foresight Index were granted patent, cited patent, paper, and cited paper.
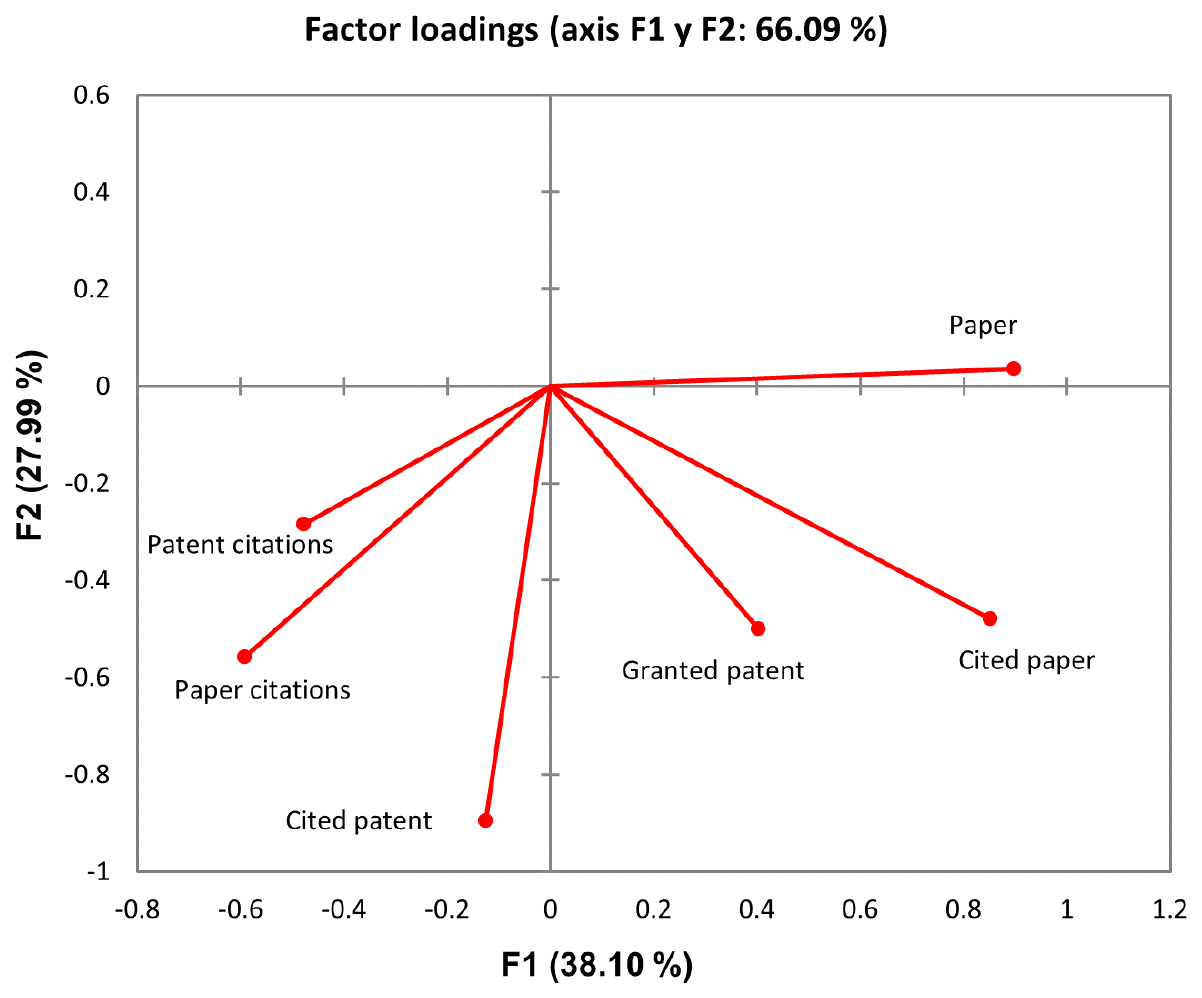
Figure 3:
Graphical representation of factor loadings obtained by Principal Component Analysis.
Once variables have been selected, the number of the DMUs to evaluate the efficiency of the Technology Foresight Index was calculated using Data Envelopment Analysis (DEA). Table 3 shows results.
Decision rules | p | q | n |
---|---|---|---|
n≥ (pxq) | 2 | 2 | 4 |
n≥2x(p+q) | 2 | 2 | 8 |
n≥3x(p+q) | 2 | 2 | 12 |
Data Envelopment Analysis (DEA) proposed that between 4 and 12 DMUs were needed to evaluate the efficiency of the Technology Foresight Index, considering the number of input variables (2) and output variables (2). The number of the Decision-Making Units used in the study (12) complies with the three decision rules proposed for DEA, which allowed a more complete validation of the Technology Foresight Index.
Obtaining the Technology Foresight Index
The Technology Foresight Index (TFI) was obtained by the quotient between the Output Indicators Index (OII) and the Input Indicators Index (III) of each DMU. The former was calculated for each DMU considering the bibliometric variables obtained from strategy 3 (Derwent Innovation Index database) and strategy 4 (Science Citation Index Expanded database) in the field of Pharmacology/Pharmacy during the period 2009-2018. The latter was calculated considering the variables obtained from the questionnaire applied to each DMU.
As shown in Table 4, the Technology Foresight Index was obtained from the quotient between the Output Indicators Index (OII) and the Input Indicators Index (III) divided by the total number of variables.
DMU | Academic degrees | Research categories | Inputyj | III | Patents | Papers | Outputxj | OII | TFI |
---|---|---|---|---|---|---|---|---|---|
1 | 18.58 | 16.06 | 34.64 | 0.173 | 12.11 | 53.53 | 65.64 | 0.328 | 0.47 |
2 | 35.65 | 22.04 | 57.69 | 0.288 | 1.98 | 5.25 | 7.23 | 0.036 | 0.03 |
3 | 104.42 | 38.39 | 142.82 | 0.714 | 2.51 | 50.34 | 52.85 | 0.264 | 0.09 |
4 | 31.12 | 11.95 | 43.07 | 0.215 | 2.05 | 13.16 | 15.22 | 0.076 | 0.09 |
5 | 10.70 | 17.47 | 28.17 | 0.141 | 4.81 | 100.76 | 105.57 | 0.528 | 0.94 |
6 | 18.80 | 15.57 | 34.37 | 0.172 | 0.30 | 32.67 | 32.97 | 0.165 | 0.24 |
7 | 24.92 | 14.20 | 39.12 | 0.196 | 1.60 | 0.30 | 1.90 | 0.010 | 0.01 |
8 | 11.00 | 16.70 | 27.70 | 0.138 | 0.77 | 5.25 | 6.02 | 0.030 | 0.05 |
9 | 23.02 | 14.70 | 37.72 | 0.189 | 1.70 | 12.90 | 14.60 | 0.073 | 0.10 |
10 | 11.10 | 15.58 | 26.68 | 0.133 | 2.67 | 1.95 | 4.61 | 0.023 | 0.04 |
11 | 24.96 | 16.80 | 41.76 | 0.209 | 0.40 | 10.20 | 10.60 | 0.053 | 0.06 |
12 | 35.45 | 20.77 | 56.22 | 0.281 | 0.50 | 6.90 | 7.40 | 0.037 | 0.03 |
study country | 0.18 |
As part of the design of this indicator, a decision rule is established to facilitate its interpretation. Technology Foresight Index is a value estimated between 0 and 1.
If the TFI result is lower than 0.5, then the human resources employed in the scientific and technological activity are greater than the obtained results. This reality implies that DMU doesn’t have a good scientific-technological performance, i.e. human resources are focused on non-scientific activities, perhaps they are more targeted towards academic activities.
If the TFI result is greater than 0.5, then the results obtained from the scientific and technological activity are greater than the human resources employed in it. This situation indicates that DMU has a good scientific-technological performance, i.e. the human resources working at DMU take advantage of inputs and have planned research outputs for the projects.
Results showed the Technology Foresight Index for each DMU, and the average value of TFI corresponded to the study country (0.18), only 25% of the DMUs were above this value. The performance of some DMUs affected the Technology Foresight Index for the whole country. In some cases, due to low values of the Output Indicators Index, such as DMU 4 (0.076), DMU 7 (0.010), DMU 8 (0.030), DMU 9 (0.073), DMU 10 (0.023) and, DMU 11 (0.053). All these institutions had very few patents and papers and focused more on academic activity. Other cases like DMU 2 and DMU 12 had the worst scenario because they had low values of the Output Indicators Index (0.036 and 0.037, respectively) and high values of the Input Indicators Index (0.288 and 0.281, respectively). Situations like these affected the Technology Foresight Index of this country.
However, there are two interesting cases to analyse, DMU 1 and DMU 9. Both had similar values of the Input Indicators Index (0.173 and 0.189, respectively), but the former had an Output Indicators Index four times higher than the latter and this condition makes the difference for their Technology Foresight indexes (DMU 1 – 0.47 and DMU 9-0.10).
Finally, a particular case of the study, DMU 3, with a high value of the Output Indicators Index (0.264) but with a very high value of the Input Indicators Index (0.714), which affected its Technology Foresight Index. This case showed the importance of having a balance between input and output indicators inside the institution. Good institutional performance cannot be achieved by increasing research outputs without modulating the training of academic personnel.
DISCUSSION
The Technology Foresight Index is based on input-output relationships, so the solution is not to increase research and innovation outputs, nor to reduce highly qualified human resources of DMUs, but to find a balance between the number of researchers and the results they bring to their institution. Institutional science policies should implement guidelines aimed at projects that include research outputs of both papers and products with innovative character, inventive activity, and industrial applicability. Moreover, there must be an institutional planning related to the growth of human resources dedicated to R&D activity. This important idea coincides with the main conclusion of the Gorbea-Portal’s study[18] where they assert that the disciplinary development of each institution is not determined by its potential resources or its scientific output, but rather by the way it uses the former and the way it produces the latter.
Scientific literature has published some foresight studies related to the pharmaceutical market. One study analysed the future of this industry within 10-15 years, considering the current diseases of population, industry’s own management, international regulatory environment, emerging technologies, the inclusion and exclusion criteria in clinical trials, development of generics,[34] but didn’t include scientific-technological output and human resources necessary for the development of the industry. Another study used an index number (Malmquist index) to quantitatively evaluate the activity of ten Japanese pharmaceutical companies over a 20-year period (1982-2001)[19] but didn’t calculate the projection of the industry into the future.
Other paper[18] proposed an index to determine the projection of the development of a subject that includes bibliometric variables such as document typology, language, indexing in databases and journals; as well as human resources variables such as academic degree, teaching position, research projects, teaching programs and student enrolment, but didn’t include a variable that measures scientific impact, nor other research outputs such as patents that measure the innovation capacity of institutions, since this study evaluates a social science area. This study agrees with Gorbea-Portal and Piña-Pozas on certain points but includes other variables, such as patent and cited items, which are necessary to support decision-making in pharmaceutical industry.
The Technology Foresight Index of the study country is low (0.18), and this shows an unfavourable scenario for its pharmaceutical industry. This scenario is an early warning regarding the science and technology policy implemented for the pharmaceutical industry in that country. The index shows that the human resources in this disciplinary field are not reaching sufficient research and innovation outputs to achieve the optimal development of the industry in the country. This result shows several causal factors:
The country’s science policy prioritizes the scientific and academic training of its professional staff over research outputs.
Few research and innovation projects led by national institutions.
Lack of budget or technological resources for the development of cutting-edge projects capable of boosting the national pharmaceutical industry.
The relationship between industry and academia is not strong enough to detect knowledge niches that could be attractive to business.
The country’s priorities don’t include the development of its own pharmaceutical industry, but rather it is an importer of pharmaceuticals.
Whatever the cause may be, it must be analysed by government and, together with other elements, decisions will be made that can improve this reality in the short, medium, or long term. For this reason, the Technology Foresight Index should be applied annually to achieve a real-time foresight and thus be able to have a criterion each year to support decision-making in terms of Technology Foresight. Systematic monitoring of this index allows continuous follow-up of the results obtained by each decision taken by the government.
In the same way that collaboration among the different sectors of a country leads to its development, collaboration between academia and business is an important factor for achieving a high value in the Technology Foresight Index. Both define the lines of mutual interest to be developed and are the fundamental pieces for the development of the industry. On the one hand, universities have highly qualified and experienced human resources (researchers), although it also has human resources who are not as experienced, but eager for knowledge and intellectual development (post-graduate students). On the other hand, companies have financial resources, cutting-edge technology, the strategy for industrial scale-up, commercial vision, customer needs, contacts with suppliers, and possible sponsorships.
Some authors coincide in this aspect, in their study shows the importance of knowledge transfer channels between these two sectors.[35] Company should participate in scientific congresses to learn about new developments in science and technology, while academia should focus its results beyond research journals. Only collaboration between these two fundamental sectors for society will achieve a cycle of research-innovatio n-development-production-commercialization capable to generate benefits not only for country’s economy, but also to solve the population’s health problems. Of course, this cycle may be capable of generating dividends in terms of exports or decreasing imports, which would serve as monetary budget to invest in other scientific projects, without ceasing to generate profits for the business sector itself.
A final factor is government, which must provide resources to support the development of certain research lines and scientific disciplines.[36,37] The question is to determine which disciplines or research lines should be developed in a country. There are national policies based on international trends to introduce their products into international market and thus guarantee exports of first-rate products. However, there is another policy where priority is given to research project that solve the country’s internal problems. One or other policy has its advantages and disadvantages in relation to segments to be covered by these decisions and depending on the economic and social situation of each nation, but in all cases, an instrument (Technology Foresight Index) is needed to support science and technology policy decisions.
The economic development of a country depends on many sectors, but specifically pharmaceutical industry is one of the most sensitive in terms of development since it deals with such sensitive issues as health and well-being. Nowadays, either for this reason or for the fact that most research is developed in public institutions with public funds, the economic development of a nation is spoken with specifications such as: social innovation,[38] social technologies,[39] innovation for social inclusion,[40,41] inclusive or democratic innovation.[42] Governments should promote the development of national and independent scientific and technological capabilities as a necessary, although not sufficient condition for achieving economic development with social inclusion.[43]
CONCLUSION
Technology Foresight exercises should be carried out periodically, with the participation of experts and using several methods that support this long and constant process. The development of national industries goes through many challenges from national economy, skilled labour force, acquired or in-house technologies, business – academia relationship, social conditions, as well as government priorities. Bearing this in mind, the main objective of this study is the design of an index number (the Technology Foresight Index) that integrates with current methodologies and supports decision-making in scientific and technological policies.
The Technology Foresight Index can be considered a robust and reliable indicator to be used for decision-making in Technology Foresight exercises at institutional and the national level in any country. The study variables were selected using Principal Component Analysis as a variable reduction technique. This statistical technique determined the variables with the highest factor loadings from a broader set of variables described by the specialized literature. This index is validated by a group of institutions or Decision-Making Units, which are the ones with the highest scientific (papers) and technological (patents) output in that country, i.e., they are the leading institutions regarding the development of new knowledge and products for national pharmaceutical industry. Validation was performed by Data Envelopment Analysis, and the number of DMUs complies with the three decision rules or models suggested for this statistical technique.
In this sense, Technological Foresight index reflects the complex reality of the socio-economic environment in which these DMUs are located, typical of a country with a developing economy. This situation is characterized by some institutions with few scientific and technological output, and other institutions with many highly qualified human resources, affecting the Technology Foresight Index (0.18) at the national level.
This study has a bias related to the input variables, since it was not possible to include the monetary budget used in scientific and technological activity, because not all the DMUs define and calculate it homogeneously. In view of the above explanation, it is suggested that guidelines be implemented to homogenize the control of this variable in all DMUs so that it can be included in the Technology Foresight Index. If monetary budget were known, the Technology Foresight Index could more accurately anticipate the development of the industry.
This study has designed an index number (Technology Foresight Index) that relates both input variables (highly qualified human resources) and output variables (patents and papers) from the most prolific institutions of the country in the field of Pharmacology/ Pharmacy, with the aim of anticipating the development of the pharmaceutical industry. This index allows policymakers to make decisions regarding which lines of research should be prioritized or which should be cancelled due to lack of expected results. Furthermore, it allows monitoring the results obtained by the DMUs that participate in R&D activities, which facilitates the granting of monetary budget for the development of research and innovation projects. Besides, this index makes it possible to anticipate a future close enough to be considered and at the same time far enough away to make decisions, reducing the uncertainty inherent to the development of new products and technologies and therefore the margin of error in the decision-making process. The Technology Foresight Index is applicable to all fields of knowledge, if papers and patents are obtained as research outputs and these outputs are developed by highly qualified personnel. Finally, it would be interesting to apply this indicator in developed economies, where exist a balance between research outputs and professional staff.
Cite this article
Herrera-Vallejera D, Gorbea-Portal S. Technology Foresight Index to Support Science and Technology Policy-Making in the Field of Pharmacology/Pharmacy: A Scientometric Analysis . J Scientometric Res. 2024;13(1):137-47.
ABBREVIATIONS
III | Input Indicators Index |
---|---|
OII | Output Indicators Index |
TFI | Technology Foresight Index |
References
- Inouye A, Süssikind C. Technological Trends and National Policy 1937: The First Modern Technology Assessment. Technology and Culture. 1977;18(4):593-621. [CrossRef] | [Google Scholar]
- Mu RP, Ren ZB, Yuan SD, Qiao Y. Technology foresight towards 2020 in China: the practice and its impacts. Technology Analysis & Strategic Management. 2008;20(3):287-307. [CrossRef] | [Google Scholar]
- Smits R. Aspects of the integration of Science and Technology in French Society In: TA – an Opportunity for Europe, Vol. 2. La Haye: Dutch Government Printing Office. 1986 [CrossRef] | [Google Scholar]
- Van Dijk A, Van Esch R, Hilders M. Technology Foresight Studies in the Netherlands. Science Technology Industry. 1996(17):149-60. [CrossRef] | [Google Scholar]
- Martin B. Foresight in Science and Technology. Technology Analysis & Strategic Management. 1995;7(2):139-78. [CrossRef] | [Google Scholar]
- Britain G. Realising Our Potential: A Strategy for Science, Engineering and Technology (Cm. 2250). 1993 [CrossRef] | [Google Scholar]
- Battezzati L. [National Priorities in Industrial Research: a first report] fr. European Review. 1997;5(2):211-212. [CrossRef] | [Google Scholar]
- Illinois Tool Works Inc (ITW). Technologie Delphi. 1998 [CrossRef] | [Google Scholar]
- De la Vega I. [WORKING PAPER 6 Training module for collection and analysis of research and development and development] es. 2009 [CrossRef] | [Google Scholar]
- De la Vega I. [The use of scientometrics in the construction of techno-scientific policies in Latin America: an uncertain relationship] es. Redes. 2009;15(29):217-40. [CrossRef] | [Google Scholar]
- Sagasti F. [Science, Technology and Innovation: Policies for Latin America] es. Lima: Fondo de Cultura Económica. 2011 [CrossRef] | [Google Scholar]
- Popper R. How are foresight methods selected?. Foresight. 2008;10(6):62-89. [CrossRef] | [Google Scholar]
- Dorin F, Perrotti D, Goldszier P. [Index numbers and their relationship with the economy] es. Santiago de Chile: Economic Commission for Latin America and the Caribbean (ECLAC). 2018 [CrossRef] | [Google Scholar]
- Luyanga S, Miller R, Stage J. Analysis Index number analysis of Namibian water intensity. Ecological Economics. 2006;57(3):374-81. [CrossRef] | [Google Scholar]
- Squires D. [Cited 2022 Sep 24];Index Numbers and Productivity Measurement in Multispecies Fisheries: An Application to the Pacific Coast Trawl Fleet. National Oceanic and Atmospheric Administration & National Marine Fisheries Service; 1988. Report No.: 67. Available fromhttps://spo.nmfs.noaa.gov/content/tr-67-index-numbers-and-productivity-measurement-multispecies-fisheries-application-pacific
[CrossRef] | [Google Scholar] - Kemp MC. Index Numbers and Demand Analysis. The Review of Economic Studies. 1957;24(3):198-202. [CrossRef] | [Google Scholar]
- Bergstrom AR. The Use of Index Numbers in Demand Analysis. The Review of Economic Studies. 1956;23(1):17-26. [CrossRef] | [Google Scholar]
- Gorbea-Portal S, Piña-Pozas M. Proposal of an indicator to measure the performance of the disciplinary development of Library and Information Science in Academic Institutions. Investigación Bibliotecológica. 2013;27(60):153-80. [CrossRef] | [Google Scholar]
- Hashimoto A, Haneda S. Measuring the Change in R&D Efficiency of the Japanese Pharmaceutical Industry. Research Policy. 2008;37(10):1829-36. [CrossRef] | [Google Scholar]
- González E, Gascón F. Sources of productivity growth in the Spanish pharmaceutical industry (1994-2000). Research Policy. 2004;33:735-45. [CrossRef] | [Google Scholar]
- Varmaghani M, Meshkini AH, Farzadfar F, Yousefi M, Yaghoubifard S, Varahrami V, Darzi ER, Anabi M, Kebriaeezadeh A, Zekri HS, et al. Evaluation of productivity in Iranian pharmaceutical companies: A DEA-based Malmquist approach and panel data analysis. Journal of Research in Pharmacy Practice. 2015;4(2):51-6. [CrossRef] | [Google Scholar]
- Organization for Economic Co-operation and Development-OECD. Frascati Manual: Guidelines for Collecting and Reporting Data on Research and Experimental Development, The Measurement of Scientific, Technological and Innovation Activities. Frascati: OECD. 2015 [CrossRef] | [Google Scholar]
- The Network for Science and Technology Indicators -Ibero-American and Inter-American-RICYT. [Santiago Manual: Manual of Science and Technology Internationalization Indicators] es. Santiago de Chile: RICYT. 2007 [CrossRef] | [Google Scholar]
- Wang EC, Huang W. Relative efficiency of R&D activities: a cross-country study for environmental factors in the DEA approach. Research Policy. 2007;36(2):260-73. [CrossRef] | [Google Scholar]
- Meng W, Daqun Z, Li Q, Wenbin L. Two-level DEA approaches in research evaluation. Omega. 2008;36(6):950-957. [CrossRef] | [Google Scholar]
- Groot T, García-Valderrama T. Research quality and efficiency: an analysis of assessment and management issues in Dutch economic and business research programs. Research Policy. 2006;35(9):1362-1376. [CrossRef] | [Google Scholar]
- Thursby J, Kemp S. Growth and productive efficiency of university intellectual property licensing. Research Policy. 2002;31(1):109-124. [CrossRef] | [Google Scholar]
- Addinsoft. XLSTAT. [Cited 2019 September 9]. Available fromhttps://www.xlstat.co m/es/
- Pino-Mejías JL, Solís-Cabrera FM, Delgado-Fernández M, Barea-Barrera R. Assessing the efficiency of research groups using data envelopment analysis (DEA). El profesional de la información. 2010;19(2):160-7. [CrossRef] | [Google Scholar]
- Charnes A, Cooper W, Rhodes E. Measuring the efficiency of decision-making units. European Journal Research. 1978;2(6):429-44. [CrossRef] | [Google Scholar]
- Golany B, Roll Y. An application procedure for DEA. Omega. 1989;17(3):237-50. [CrossRef] | [Google Scholar]
- Murias-Fernández P. [Methodology for the application of data envelopment analysis: evaluation of technical efficiency at the University of Santiago de Compostela] es [dissertation]. Santiago de Compostela: University of Santiago de Compostela. 2004 [CrossRef] | [Google Scholar]
- Fernández-Llimos F. Differences and similarities between Journal Impact Factor and Cite Score. Pharmacy Practice. 2019;16(1):1282 [CrossRef] | [Google Scholar]
- Foster BC. Foresight Scanning: Future Directions of Clinical and Pharmaceutical Research. Journal of Pharmacy and Pharmaceutical Sciences. 2008;11(4):108S-22S. [CrossRef] | [Google Scholar]
- Bekkers R, Bodas-Freitas IM. Analysing preferences for knowledge transfer channels between universities and industry: To what degree do sectors also matter?. Research Policy. 2008;37(10):1837-53. [CrossRef] | [Google Scholar]
- Casas R, Luna M. [Government, academia, and business in Mexico. Towards a new configuration of relations] es. México: UNAM, Plaza y Valdés. 1997 [CrossRef] | [Google Scholar]
- . [Science, Technology, and Innovation Policies in Latin America: between competitiveness and social inclusion] es. [Latin American Perspectives in the Social the Social Study of Science, Technology and Knowledge] es. México: Red Cyted/FCCyT, Siglo XXI. 2014:352-264. [CrossRef] | [Google Scholar]
- . [Science, technology, and innovation for inclusive development in Colombia: Pilot programs developed by Colciencias] es. [Innovation systems for inclusive development: the Latin American experience] es. México: Foro Consultivo Científico y Tecnológico. 2013:125-154. [CrossRef] | [Google Scholar]
- Thomas H, Fresolli M, Becerra L. Science and Technology Policy ex/inclusion: Analyzing opportunities and constraints in Brazil and Argentina. Science and Public Policy. 2012;39(5):579-91. [CrossRef] | [Google Scholar]
- . [Innovation and democratization of knowledge as a contribution to inclusive development] es. [Innovation systems for inclusive development: the Latin American experience] es. México: Foro Consultivo Científico y Tecnológico. 2013:19-34. [CrossRef] | [Google Scholar]
- Vessuri H. Introduction to special section: The use of knowledge for social cohesion and social inclusion. Science and Public Policy. 2012;39(5):545-7. [CrossRef] | [Google Scholar]
- Johnson B, Andersen AD. Learning, Innovation and Inclusive Development. [Cited 2022 Nov 17];New perspectives on economic development strategy and development aid. Globelics Secretariart, Aalborg University Press; 2012 Report No.: 2011/2012. Available fromhttps://vbn.aau.dk/ws/files/70880770/Learning_Innovation_and _Inclusive_Development.pdf
[CrossRef] | [Google Scholar] - Casas R. [Knowledge, technology and development in Latin America] es. Revista Mexicana de Sociología. 2004;66:255-77. [CrossRef] | [Google Scholar]