ABSTRACT
The present study shows the incidence of knowing the impact of social networks of co-authorship in the construction of new research, since they are fundamental for the location of expert authors in the thematic developments of which they are the main referents. In this sense, their location allows the construction of networks that have a significant impact on aspects such as: relational capital, cognitive capital and structural capital. In this way, it makes it possible to understand the incidence of structural gaps as positive or negative determinants when reviewing the influence of their research and the size of their epistemological influence on new researchers and topics associated with the construct of emotional education. In the methodology, the two most recognized databases in the academic community (Scopus and Web of Science) were used from the search parameters of: rank, date of consultation, type of documents, search field, keywords and results together with the review of the manifest variables of: link strength, network intensity, size, structural gaps, diversity index of collaboration and permanence, the impact of existing research. The conclusions show the need to continue delving into the construct of emotional education in the training of teachers and students, as well as in the strengthening of social networks of knowledge that allow further deepening in collaborations to produce new epistemological views from more interdisciplinary approaches with expert and novice researchers who contribute to the transformation of the educational systems of their countries.
INTRODUCTION
The impact of research is sometimes linked to the reputation of the leading author in the development of academic production. This generates positive repercussions in the sense that it leads to future works and alliances of better enhancement that allow the obtaining of budgetary resources and sponsors that contribute to new exercises of a greater magnitude. (Geraldo Campos et al., 2020);[1] However, there may also be negative consequences that limit the generation of social networks of knowledge, since by losing effective recognition, the researcher is affected in his prestige, which leads to not being in force as a reference of the subject in question, at the time of making new collaborative networks of knowledge, as established by Li et al, (2013).[2]
Thus, the scientific social networks of knowledge require studies that allow establishing the impacts that have been generated in relation to the topic, in the sense of expressing what has been investigated, how they have been carried out, in order to discover, in each development, new topics to work on. In this way, emphasis is given to those who have been in charge of offering different perspectives, which constitutes them as active references to be linked with them in projects of greater scope. (Simões et al., 2024).[3] In addition, to know what interactions they have been able to build in their networks and how much they have been able to consolidate their research processes. (Gao et al., 2023).[4]
In this way, it is necessary to review the relevance of social knowledge networks in emotional education, as the following points out Feng et al., (2024)[5] where they express the importance of establishing knowledge networks that are consolidated in relation to education. From education, the need arises to continue thinking about it in order to introduce changes that contribute substantial improvements in its processes. A contribution is, precisely, everything related to emotion, its conceptual, epistemological and research horizons and its link to the learning processes of teachers and students. Thus, working on research that addresses the issue of emotional education and the social networks of knowledge that have been developing can provide essential elements to address these problems. For this reason, authors such as Jaramillo et al., (2023),[6,7] Kaur et al.[8] and Kurata et al., (2023)[9] point out the importance of research on the topic of training teachers and students in emotional education.
This leads to think about the development of this study, which presents three indicators of social capital (relational capital, structural capital and cognitive capital). These lead to review the implications of the relationships that arise from the exercises of the research networks, which can lead to consolidation or reduction, due to factors associated with the main author. In this sense, the main author becomes a reference at a given moment, being responsible for originating high impact productions in other epistemological networks of the knowledge in which he/ she has become an expert.
Theoretical framework
Emotional Education is an educational process that stems from a larger construct that of Emotional Intelligence, developed most strongly in the 1990s in the United States by Mayer et al, (2016).[10] From there, it has been expanding its research horizon, linking in different populations. Groups in Spain such as the University of Malaga De-Torres et al., (2022)[11] and at the University of Barcelona the Grop de Recerca en Orientació Psicopedagógica- GROP for its acronym in Catalan (Pérez-González et al., 2022).[12] The aforementioned authors have conducted their research on the populations of teachers and students of different educational levels focusing on the constructs of emotional intelligence, emotional education and emotional competencies. They highlight the creation of social networks of knowledge and interactions of diverse themes in the construction of training programs. In addition, other authors such as (Filella and Ros-Morente, 2022);[13](Gómez-Leal et al., 2023);[14] (Berastegui-Martínez et al., 2024)[15] have been perfecting the work through the creation and validation of instruments and scales to measure the constructs. This is allowing an approach to a reality that has been gaining strength in educational contexts and their stakeholders (Estrada-Fernández et al., 2023;[16] Xu et al., (2023);[17] Sánchez-López et al., 2022.[18])
On the other hand, the social networks of knowledge have become a permanent exercise to delve into aspects that arise from Emotional Education, which evidences the continuous creation of new research. (Filella and Ros-Morente, (2022);[13] Garcia-Blanc et al., (2022);[19] Pérez-González et al., (2022);[12] Ros-Morente et al., (2022);[20] Rueda et al., (2021);[21] Sánchez-López et al., (2022)[18]). In addition to adding what other researchers have been suggesting about teachers and how they must learn to know their emotions and manage them, especially those who are in the classroom.[22] As well as those who are in training in educational centers that prepare them to become preschool and elementary school teachers, which in Colombia are called Escuelas Normales Superiores, together with the inclusion of the constructs of Neuroscience and emotional education in order to generate emotional competencies from the empirical-analytical approach as proposed by[23,24] the above evidences the interest in producing new knowledge to expand it and establish collaborative social networks.
Relational Capital
According to Geraldo-Campos et al. (2020)[25] relational capital refers to a person’s ability to establish and maintain effective relationships with other individuals or groups in his or her personal or professional environment. Relational capital is composed of intangible resources, such as trust, loyalty, reputation, influence, reciprocity and commitment, valuable aspects for the individual and for the collaborative networks to which he or she belongs. The strengthening of collaborative ties between academic communities allows for a construction over time, through interaction and permanent communication with other researchers in the environment.
Kalhor et al. (2022)[26] discuss the concept of relational capital as a fundamental element in the organization of an organization’s intellectual capital. Zhou et al. (2024)[27] argue that interorganizational relationships can be a source of competitive advantage for network communities, which generates benefits for intellectual production and the strengthening of academic networks. Due to its intangibility, relational capital is unique and difficult to imitate, since it depends on the relationships and reputation that a person or organization has built with other actors in the environment, which can generate a sustainable competitive advantage.
In this sense, following Cudina et al. (2021)[28] collaborative research favors the rhythm of how research and the different scientific products are carried out and directly influences the impact of this research to the extent that it makes possible an increase in the number of publications, citations and diversification of the public to which the academic products are directed. Accordingly, relational capital is fundamental to achieve these results, since the networking that is built from academic relationships and interdisciplinarity is the fundamental basis for the development of research processes (De Lima et al., 2024)[29] and makes research an open and dynamic practice. Accordingly, the following hypothesis is proposed in this study:
H1: Relational capital positively influences impact
Structural Capital
Structural social capital at a general level is considered an important aspect of the intellectual capital of knowledge which is part of the tangible reserves and productions that evidence the information and production of knowledge (Gao et al., 2022),[30] is also associated with numerous forms of social organization, rules and procedures that contribute to cooperation and collaboration (Zeleke et al., 2023),[31] which has an impact on the production and success of an organization. Structural capital depends on the set of links generated through appropriate network structures and organizations that strengthen relationships (Ma’ruf et al., 2024),[32] as processes of communication and articulation to the structures of effectiveness and efficiency. In the academic context it refers to the way in which the links and connections between authors and research groups are configured. This includes the density of connections, the relationships between authors and the structural integration of the knowledge network (Aranibar Melgar and Travieso Valdés, 2023).[33] The analysis of structural capital in this study allows a better understanding of the relationships between networks and collaborative communities towards the production of knowledge in areas related to emotional education. According to the literature on social networks, several authors have worked on the concept of structural capital and its components. In this particular study, structural capital is made up of size and structural gaps.
Structural holes
Structural holes in accordance with are gaps between groups in the same social network.[34] These gaps are considered useful for people occupying intermediate positions in the network since they make it possible to act as intermediaries between different networks and gain greater access to available information. Structural gaps represent a competitive advantage for individuals and organizations in a social network by acting as a facilitator for the transfer of knowledge between groups and increasing the flow of information and academic performance (Dahiyat et al., 2023).[35]
Size
The size of the social network is referred to according to the number of people that make up an academic network, this is represented in the strength of social ties;[36] these social ties can be measured according to the frequency, duration and diversity of shared interactions. According to this author, weak ties, i.e., those that connects people who do not have constant interaction, are important for the dissemination and mobilization of knowledge. This is because people with weak ties are available for new information, which allows them to serve as bridges between various social groups. Therefore, the size of a person’s social network is as important as the diversity and quality of the ties he or she maintains (H. Li and Sewell, 2021).[37] Thus, in relation to the development of structural capital, the following hypothesis is proposed:
H2: Structural capital positively influences impact
Cognitive Capital
Cognitive capital refers to the scientific productions that researchers develop over time as they interact with others; this process involves sharing a series of knowledge and experiences on a specific topic. Therefore, in this process a series of skills are learned that allow the construction of new knowledge through specialized discourse and the establishment of standards of practice within the collective Faraj et al.,(2015);[38,39] Tyndall et al., (2019)[40] Jalali et al.,(2023);[41] Vicencio-Ríos et al, (2022);[42] Luis-de Cos, et al., (2024).[43] Engaging in a meaningful exchange of knowledge requires at least some level of shared understanding between the parties, such as language appropriate and relevant to the issues at hand, which involves; (Barnett and Park, 2023)[44] the longest experience and permanence in shared practice;[41] Ullah et al., (2022).[45] In this sense, the theory of socialization; (Affonso et al., 2022),[46] serves as a key theoretical basis for understanding the development of cognitive capital through team composition and tenure in publishing. Socialization refers to the process by which people acquire the knowledge, skills and dispositions that make them more or less capable members of society (Alzina and Cassa, 2021);[47,48] Essers et al.,(2022).[49]
Some previous research on the socialization of scientific productions considers that academic communities where this process of knowledge exchange takes place significantly improve cognitive capital and this is due to the processes of scientific dissemination. In the context of a co-authorship network, co-authors may not come from the same discipline. They may develop their cognitive capital from their own disciplines before becoming co-authors. Different disciplines have their own shared visions, traditions, codes, languages, knowledge, interpretations, meaning systems, social networks and collectively owned social capital. Each operates as a research community consisting of a large number of scholars who contribute resources and share social capital; and a scholar may be a member of several research communities at the same time. Thus, it is hypothesized that:
H3: Cognitive capital positively influences impact
Conceptual model
The conceptual model proposed in this study on emotional education is presented below (Figure 1), which shows the correlations between the impact of the research and the latent variables, which are constituted as the three dimensions of social capital: relational capital, structural capital and cognitive capital.[40,43]
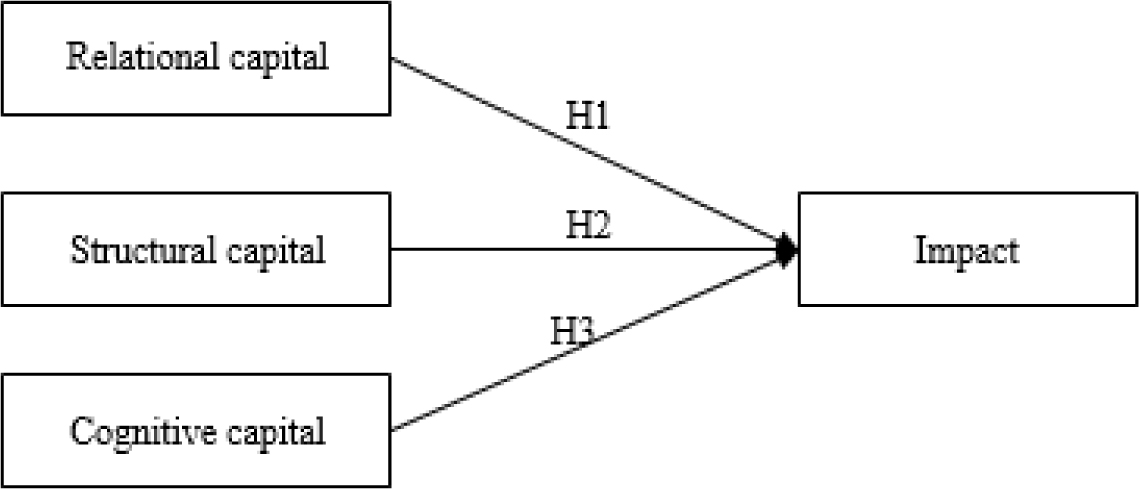
Figure 1:
Conceptual model.
The first association between the variables impact and relational capital serves to expose an H1, which correlates the ability of the researcher to build and maintain relationships in the field of research, maintaining effective relationships between the different actors and the quality and impact of such research. It is possible then, that when you have a high level of relational capital, as a researcher, you may have greater opportunity to collaborate with other researchers and experts, which results in greater impact and quality of research.
A second association is presented between the dependent variable impact and structural capital (Figure 1), which in turn indicates the presence of H2, taking into account that structural capital refers to structural rootedness defined as the density and strength of the links between members of a network.[36] From the above it can be presumed that if a researcher has a high level of structural capital, i.e., strong relationships that can be a source of information and support, the quality and impact of his research will increase, as will his capacity to achieve his objectives and improve his creativity. However, if he/she has insufficient structural capital, the effort will be made to strengthen his/her cognitive and relational capital.
Finally, a third association is presented in the conceptual framework, where impact is correlated with cognitive capital, which yields an H3 that evidences the importance of knowledge as a promising element of success, since it constitutes a competitive advantage in today’s world (Mehralian, et al, 2018).[50] Hence, cognitive capital is usually considered valuable to the extent that it includes not only the learning of skills and knowledge, but also the implementation of specialized discourses and the formation of norms and practices within the research group. Therefore, it can be concluded that the greater the cognitive capital, the greater the impact and quality of scientifically relevant research. (Bornhauser and Rivera, 2023).[51]
METHODOLOGY
Sample
The two most recognized databases in the academic community (Scopus and Web of Science) were used to generate the social capital variables. The linkage was performed through Bibliometrics (Aria and Cuccurullo 2017)[52] and (Robledo et al. 2022;[53] Zuluaga et al. 2022),[54] these two packages allow the union of the main records together with their references. To evaluate the variables of social capital and impact, a query on emotional education was carried out in the Web of Science and Scopus databases (see Table 1). Recent research has highlighted the importance of combining these two databases to carry out efficient scientometric analysis Grisales et al, (2024).[55] Robledo et al., 2022);[56] therefore, the present study follows this recommendation. The results obtained for Web of Science were 310, while for Scopus they were 426, giving a total of 607 unique records. Of these, 181 (29.81%) records in Web of Science were not found in Scopus. This underscores the need to work with multiple databases to achieve greater coverage in scientometric analysis.
Parameters | Web of Science | Scopus |
---|---|---|
Range | 2000-2023 | |
Consultation date | March 25, 2023 | |
Type of documents | Articles, books, book chapters and conferences. | |
Search field | Title, abstract and keywords | |
Words | Emotional education skills teachers training. | |
Results | 310 | 426 |
Total (Wos+Scopus) | 607 |
To obtain the impact and social capital indicators, it was necessary to carry out a preprocessing of the 607 records, which consisted of text mining and web scraping to break down the references and authors. For the process of analyzing the references, the methodology proposed by Tree of Science was used;[56] Zuluaga et al., 2022),[57] while the authors followed the approach of Hurtado-Marin et al. (2021).[58] In the end, 338 data were used to develop the statistical model and validate the hypotheses proposed. The decrease in the amount of data (from 15,645 to 338 researchers) is due to the existence of a high number of researchers with a single participation in an article, which generates a significant bias in the data when performing statistical analyses. These data are also eliminated because they do not provide a sufficiently broad social structure in the personal network to understand the impact on citations. Figure 2 illustrates the general process of data collection and preprocessing.
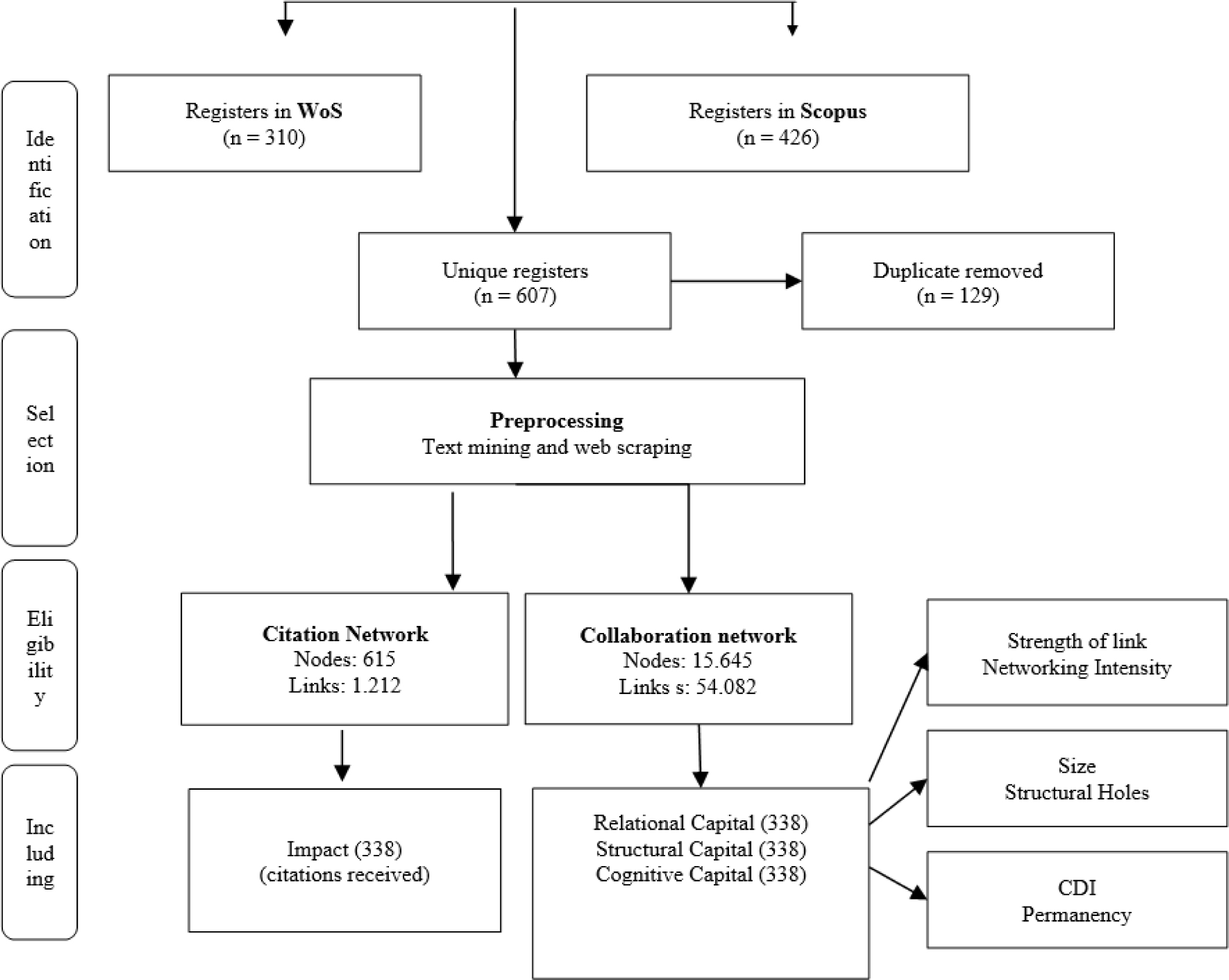
Figure 2:
PRISMA flow of data processing.
Operationalization of variables
All variables were determined by extracting the ego network of each researcher. The ego network is the personal network generated through the different co-authorships in the articles. This strategy was chosen because of the researcher’s ability to influence his or her personal network. Other studies have analyzed the total network of scientific collaboration; however, this study proposes a proposal directed towards the elements that a researcher can influence to improve his scientific performance and this is his personal network.
Strength of link
To measure link strength, all personal network link values were summed and divided by the total number of ego network collaborators. This gives an average of the link strength of each researcher.
Networking intensity
Networking intensity explains the extent to which researchers create new links or strengthen old links over a period of time (Robledo, John-Eider, et al., 2022). Therefore, the number of links was identified and divided by the difference between the years of the last publication minus the first publication.
Size of personal social network
The size of the personal social network or ego network is the number of co-authors with whom a researcher has constructed articles throughout his or her career.
Structural holes
Proposed the following formula to identify structural holes:[59]

Where, i is the main node, j and q are other nodes in the social network; pij, piq and pqj represent the ratio of the links from i to j, the connections from i to q and the connections from q to j, respectively.
Diversity Collaboration Index
The Collaborative Diversity Index (CDI) was proposed by Li et al. (2013), is defined as the composition of a team. If the CDI is one, it means that the composition of the team is totally exploratory, that all members are new and there are no papers with duplicate authors. If the CDI is close to zero, it means that there is a total exploitation of the relationships, that there are pairs of the same researchers working on different articles. In other words, they are taking advantage of their work dynamics to produce different articles.
Permanency
It is important to identify the amount of time spent writing articles, since the longer the articles have been written, the more connections they generate or the stronger the existing ones. This variable was generated by identifying the difference between the last year of publication and the first.
Limitations and biases
Although the information search work was done in March 2023 trying to find information related to emotional education and the research done up to that time for the writing of this article, an update was made in the bibliography consulted that allows to broaden the vision of the importance of emotional education and research in emotional education. In addition to this, a search was made mainly in documents in English since it is the language that most science is published at that time from which articles emerged in the search that the search parameters were carried out from 2000 to 2023.
RESULTS
Table 2 presents the results of the multivariate statistical analysis, with which the correlations presented for the seven manifest variables of the present study were evaluated. The columns M (Mean) and S.D. (Standard Deviation) present information on each of the variables, while the VIF column (relationship of variables to each other) presents the correlation of the predictor variables. Columns 1 to 7 represent the values of the correlation coefficients between the different variables. In each of the rows of the table (numbered from 1 to 7) we find the information corresponding to each variable.
Sl. No. | M. | D.E. | VIF | 1 | 2 | 3 | 4 | 5 | 6 | 7 | |
---|---|---|---|---|---|---|---|---|---|---|---|
1 | Strength of link | 1.98 | 1.23 | 3.149 | 1 | ||||||
2 | IntensityNetworking | 11.46 | 15.04 | 1.970 | .42** | 1 | |||||
3 | Size | 28.82 | 44.88 | 2.129 | -.03* | .43** | 1 | ||||
4 | Structural holes | 0.34 | 0.31 | 2.103 | .03* | -.24*** | -.46** | 1 | |||
5 | CDI | 0.62 | 0.24 | 2.898 | -.80** | -.39** | -.04 | .08 | 1 | ||
6 | Permanency | 16.73 | 12.72 | 2.635 | -.04 | .02 | .56** | -.67** | -.03 | 1 | |
7 | Citations | 3.12 | 5.42 | NA | .26** | .21** | .13* | -.21** | -.23** | .25* | 1 |
Note. M and SD are used to represent the mean and standard deviation, respectively.
Values with asterisks indicate the 95% confidence interval.
The confidence interval is a plausible range of population correlations that could have caused the sample correlation (Cumming, 2014).
*indicates p<.05. ** indicates p<.01.
In the table above, the VIF values indicate the degree of multicollinearity between each of the latent variables and the other variables in the model. In this sense, it is evident that in the table, the VIF values vary from 1.970 to 3.149. This indicates that there is some multicollinearity present in the model, but it is not significant. The variable with the highest VIF value is “Link strength” which equals 3.149, indicating that there is some degree of multicollinearity for this variable and it is considered acceptable since its value is below 5. Likewise, the other variables present VIF values below 5 and above 1, indicating that they all present some degree of multicollinearity and are considered acceptable in relation to the other variables in the model. The asterisks present in the values in columns 5 to 9 mean that the correlation coefficient between the variables is significant at or above a confidence level of 95%.
The variables Link Strength and Networking Intensity are directly related to H1 (Relational Capital), the variables Size and Structural Gaps are directly related to H2 (Structural Capital), the variables CDI and Permanence are directly related to H3 (Cognitive Capital) and finally the independent variable “Citations” is presented, which presents the level of impact on scientific production and co-authorship networks in relation to the subject of the statistical study being presented.
Figure 3 analyzes the model proposed in this study in relation to the associations between several variables and how these variables influence the impact; in this case, it highlights the associations of the latent variables, which refer to the three dimensions of social capital and their influence on the impact of the research. According to this model, the relational capital dimension consists of two indicators: strength of the link and intensity of networking. The structural capital dimension contains two indicators: size and structural gaps. The third dimension of cognitive capital comprises two indicators: CDI and permanence. Subsequently, the table includes the dependent variable of research impact evaluated through citation counts.
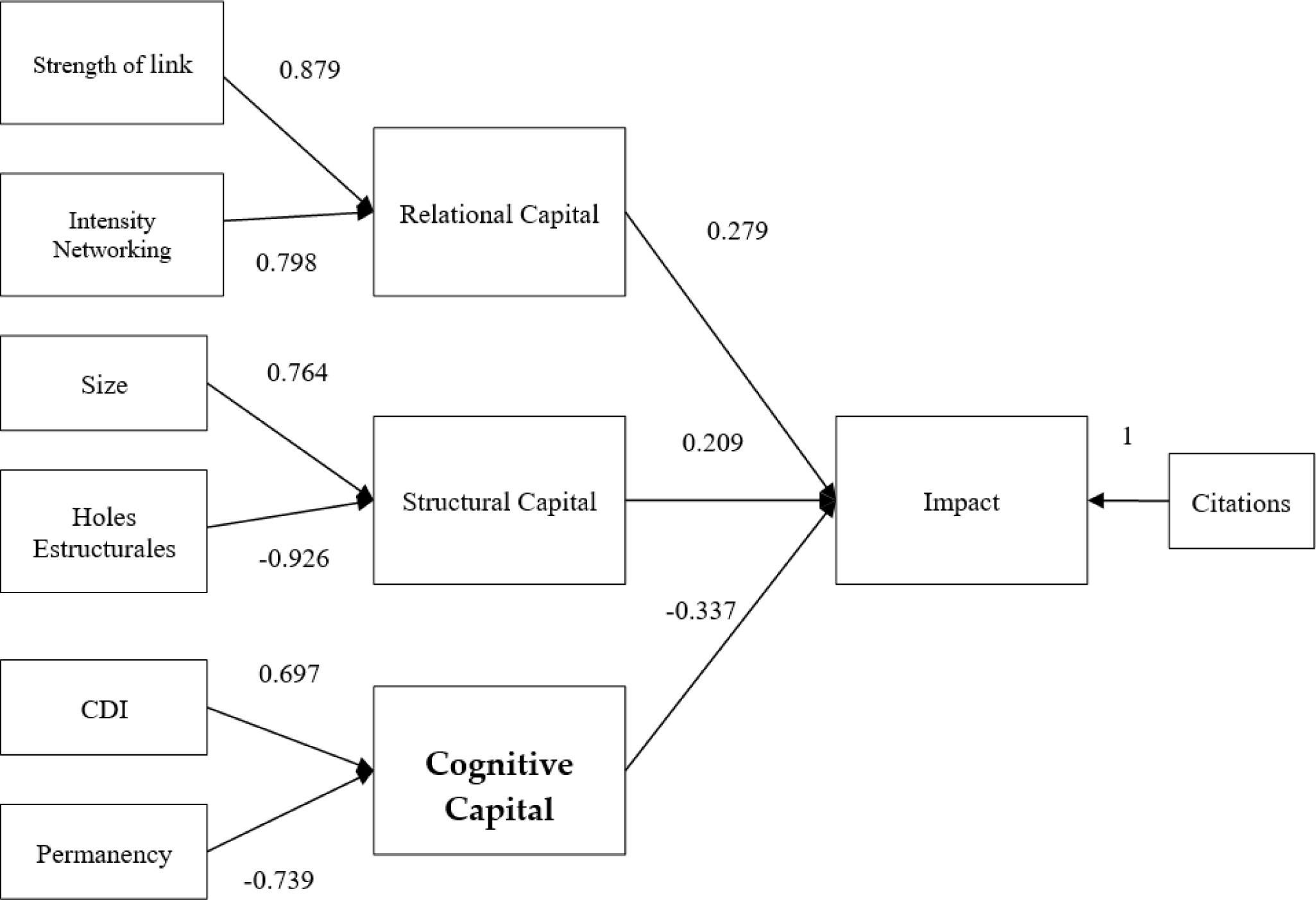
Figure 3:
Analysis of the trajectory of impact variables.
In the data provided, it is observed that the manifest variable link strength has a direct positive relationship with the latent variable relational capital with a path index of 0.879. This suggests that a greater strength of the link between the actors may contribute to a greater formation and development of relational capital, which in turn may increase the impact. The manifest variable networking intensity also has a positive direct relationship with the latent variable relational capital at a path index of 0.798, indicating that greater networking intensity may contribute to greater formation and development of relational capital and, therefore, increase impact. In contrast, the manifest variable size has a direct positive relationship with the latent variable structural capital with a path coefficient of 0.764, indicating that greater size may contribute to greater formation and development of structural capital and, therefore, increase impact.
On the other hand, the manifest variable structural gaps has a direct negative relationship with the latent variable structural capital represented in a path index of -0.926, indicating that a greater amount of structural gaps may decrease the formation and development of structural capital and, therefore, reduce the impact. Regarding the latent variables cognitive capital and impact, it is observed that the manifest variable CDI has a positive direct relationship with the latent variable cognitive capital given at a path coefficient of 0.697, suggesting that more cognitive capital may contribute to greater formation and development of collective cognition. However, it is also observed that the latent variable cognitive capital has a negative direct relationship with the impact according to the path index of -0.337, indicating that greater formation and development of cognitive capital may reduce the impact.
Finally, the manifest variable Permanence has a direct negative relationship with the latent variable cognitive capital path coefficient of -0.739, suggesting that greater actor tenure may reduce the formation and development of cognitive capital. In addition, the latent variable cognitive capital also has a negative direct relationship with impact at a path coefficient of -0.337.
Table 3 shows the effect sizes of all hypotheses: bond strength, networking intensity, size, structural voids, CDI and permanence.
Hypothesis | Factor Loadings | Cohen f2 | Effect size |
---|---|---|---|
Strength of link | 0.879 | 0.014 | Low |
Intensity Networking | 0.798 | 0.014 | Low |
Size | 0.764 | 0.007 | Low |
Structural holes | -0,926 | 0.0007 | Low |
CDI | 0.679 | 3,49 | Low |
Permanency | -0.739 | 0.03 | Medium |
It is evident that link strength, networking intensity, size, structural gaps and CDI have a low effect size, suggesting a weak or insignificant correlation with impact. While permanence reveals a medium effect size, indicating a strong relationship with impact.
DISCUSSION
The present study makes it possible to establish that, based on the review of how the social networks of co-authorship are and are found in reference to the subject of emotional education, which implies knowing the effect of the dimensions of relational capital, structural capital and cognitive capital, which are shown below.
The effects of relational capital
The effects of relational capital in academic networks in relation to the thematic of emotional education is important to maintain effective relationships of researchers in scientific collaboration networks. According to the data in Table 3, it can be inferred that there is a low Cohen’s f 2 effect of relational capital in relation to the strength of the link between the various researchers working on this topic, which may be of concern for the development of future research, given that the lack of an intense collaborative network reduces the level of opportunities and resources for the development and quality of the scientific collaboration network, which is directly related to the intensity of networking.[51,34] In this sense, the variable allows understanding the dimension and scope of what a network implies, where significant impacts are established at the time of new developments of the subject matter and the assimilation of greater production exercises of expert authors, in projection and novices.
Structural capital effects
The analysis of structural capital in academic networks is essential to understand how relationships develop in an academic community. In this sense, the influence of size and structural gaps are important factors that can influence the structural capital of an academic network Nahapiet and Ghoshal, (1998),[44,60] In relation to size, Cohen’s f 2 for the size of the academic network was found to be 0.007, indicating that size has a moderate influence on the structural capital of the network. That is, as the size of the network increases, more opportunities for collaboration and knowledge sharing can be generated, which can increase structural capital. On the other hand, regarding structural gaps, it was found that Cohen’s f2 for network structural gaps presents a low indicator of 0.0007, indicating that structural gaps have a very low influence on network structural capital. In this sense, structural gaps may allow diversification of information sources, which can be beneficial for the development of an academic network. However, if structural gaps are too large, it may hinder the flow of knowledge and limit the development of structural capital. The above indicates that network size has a moderate influence on structural capital, while structural gaps have a very low influence. These findings can be useful for understanding how relationships develop in an academic network and how collaborative work strategies can be fostered in different research groups and academic institutions.
Cognitive capital effects
According to the results of the Cognitive Capital, it can be observed that the factor load for the variable Permanence is -0.739, influencing negatively between this variable and the Cognitive Capital. On the other hand, the factor load for the CDI variable is 0.679, indicating a strong positive relationship between this variable and Cognitive Capital. In addition, it can be observed that the f2 effect for the permanence variable is medium (0.30), for the CDI variable it is low (3.49). This shows that there is a difference between the variables and Cognitive Capital. It is concluded that these results indicate that permanence is positively related to Cognitive Capital, as is CDI. This study made it possible to create a new way of measuring patterns of co-authorship in research, known as CDI. In terms of knowledge, it contributes a new perspective to the existing literature on this construct. Previous studies were focused on bibliometric analyses, where they focused on research performance in terms of co-authorship among nations, disciplines and institutions. Passos et al. (2022),[4,61] According to these results, the CDI makes it possible to evaluate the positive correlation between an author’s productivity index and the average number of signatures on his or her papers Habid Agagi et al.(2022).[62]
CONCLUSION
The development of this article shows the importance of the intervention of the variables relational capital, structural capital and cognitive capital and their direct relationship with impact. In this sense, new knowledge is established that will allow the creation of scientific collaboration networks for the generation of research that will enrich the construct of emotional education and its direct relationship with teacher training, as well as its application at different educational levels.
On the other hand, one must be aware of the limitations that emerge in a research process, given that sometimes it is not easy to establish scientific collaboration networks that generate the expected impact. In this way, it will be possible to attend to the vicissitudes that arise in the developments, both the connections that are established with the main authors, their permanent associations and the new ones that begin to emerge.
Finally, the subject of emotional education is applicable to any scenario where the human being is in the possibility of permanent interaction with others. In this sense, the context of the school and its members (teachers, students, teachers’ directors and parents) are fundamental participants to generate new knowledge and research networks that make it possible to impact, in a positive way, the developments related to the subject.
We believe that this research will allow us to establish new lines of research in relation to the construct of emotional education. Thinking about its linkage to the educational processes in the classroom, in teacher training in the curricula, may provide significant contributions to teachers and students, as well as to their families.
Cite this article:
Licona MÁA, Cuadros JAC, Arroyave EAZ. Co-Authorship Networks and Impact on Emotional Education Research. J Scientometric Res. 2024;13(3):x-x.
References
- Geraldo Campos LA, Soria Quijaite JJ, Tito Huamaní PL, Modelo SE. basado en valores organizacionales y capital intelectual: un estudio realizado en entidades del sistema financiero peruano. Retos. 2020;10(19):5-28. [Google Scholar]
- Li EY, Liao CH, Yen HR. Co-authorship networks and research impact: A social capital perspective. Research Policy. 2013;42(9):1515-1530. [Google Scholar]
- Simões LRD, Andriola WB, De Queiroz Machado D. Variation in co-authorship networks for publications on student aid, based on the terms used in the research. Education Policy Analysis Archives. 2024:32 [CrossRef] | [Google Scholar]
- Gao J, Nyhan J, Duke-Williams O, Mahony S. Gender influences in Digital Humanities co-authorship networks. Journal of Documentation. 2022;78(7):327-350. [CrossRef] | [Google Scholar]
- Feng J, Gao X, Li H, Liu B, Tang X. A Science Mapping Approach-Based Review of Construction Workers’ Safety-Related Behavior. Buildings. 2024;14(4):1162 [CrossRef] | [Google Scholar]
- Jaramillo AM, Williams HTP, Perra N, Menezes R. The structure of segregation in co-authorship networks and its impact on scientific production. EPJ Data Science. 2023;12(1):1-15. [CrossRef] | [Google Scholar]
- Blaschke S. Publication authorship: A new approach to the bibliometric study of scientific work and beyond. PloS One. 2024;19(4) [CrossRef] | [Google Scholar]
- Kaur G, Kalra D, Kumari I, Gupta S, Saluja S. Exploring the Fusion of Neuroscience and Leadership Through Bibliometric Analysis. 1 C.E:IGI Global. [CrossRef] | [Google Scholar]
- Kurata K, Miyashita S, Sengoku S, Kodama K, Lim YJA. Comparative Analysis of Social Entrepreneurship and Entrepreneurship: An Examination of International Co-Authorship Networks. Sustainability: Science Practice and Policy. 2023;15(22):15873 [CrossRef] | [Google Scholar]
- Mayer JD, Caruso DR, Salovey P. The ability model of emotional intelligence: Principles and updates. Emotion Review: Journal of the International Society for Research on Emotion. 2016;8(4):290-300. [CrossRef] | [Google Scholar]
- De-Torres I, Bustos F, Arango-Lasprilla JC, Fernández-Berrocal P. Training on Emotional Intelligence for Caregivers of Patients with Acquired Brain Injury and Cognitive Impairment: A Quasi-Experimental Study. International Journal of Environmental Research and Public Health. 2022;19(21) [CrossRef] | [Google Scholar]
- Pérez-González JC, Filella G, Soldevila A, Faiad Y, Sanchez-Ruiz M-J. Integrating self-regulated learning and individual differences in the prediction of university academic achievement across a three-year-long degree. Metacognition and Learning. 2022;17(3):1141-1165. [CrossRef] | [Google Scholar]
- Filella G, Ros-Morente A. Happy Software: An interactive program based on an emotion management model for assertive conflict resolution. Frontiers in Psychology. 2022;13:935726 [CrossRef] | [Google Scholar]
- Gómez-Leal R, Gutiérrez-Cobo MJ, Megías-Robles A, Fernández-Berrocal P. The dark triad and subjective well-being: The mediating role of cognitive-emotional regulation strategies. Scandinavian Journal of Psychology. 2023;64(3):368-375. [CrossRef] | [Google Scholar]
- Berastegui-Martínez J, De la Caba-Collado MÁ, Pérez-Escoda N. Intervención en educación emocional. Efectos en la competencia emocional del alumnado de Primaria y Secundaria. Revista Complutense de Educación. 2024;35(1):187-197. [CrossRef] | [Google Scholar]
- Estrada-Fernández C, Ros-Morente A, Alsinet-Mora C. Influence of anger management and emotional skills on self-esteem in pre-adolescents and their relationship with emotional control and psychological well-being. Revista de Psicología y Educación. 2023;18(1):62-70. [CrossRef] | [Google Scholar]
- Xu J, Zhang L, Ji Q, Ji P, Chen Y, Song M, Guo L, et al. Nursing students’ emotional empathy, emotional intelligence and higher education-related stress: a cross-sectional study. BMC Nursing. 2023;22(1):1-9. [CrossRef] | [Google Scholar]
- Sánchez-López MT, Fernández-Berrocal P, Gómez-Leal R, Megías-Robles A. Emotional Intelligence and Risk Behaviour: A Risk Domain-Dependent Relationship. Psicothema. 2022;34(3):402-409. [CrossRef] | [Google Scholar]
- Garcia-Blanc N, Joana A, Gomis-Cañellas R, Ros-Morente A, Filella-Guiu G. El vocabulario emocional y la ira en estudiantes españoles. Revista D’innovació I Recerca E Educació. 2022;15(2):1-17. [CrossRef] | [Google Scholar]
- Ros-Morente A, Coronel M, Filella G. Study of emotional skills in a sample of students and workers. Intangible Capital. 2022;18(3):430 [CrossRef] | [Google Scholar]
- Rueda P, Pérez-Romero N, Victoria Cerezo M, Fernández-Berrocal P. The role of emotional intelligence in adolescent bullying: A systematic review. Psicologí a Educativa. 2021;28(1):53-59. [CrossRef] | [Google Scholar]
- Cárdenas Cuadros JA. El amor en la relación maestro-estudiante y su influencia como posible factor de protección en la salud mental del maestro. Ciencia Y Academia. 2022:2 [CrossRef] | [Google Scholar]
- Albor-Licona MA. FORMABILIDAD Y EDUCABILIDAD PARA LA ENSEÑANZA, APRENDIZAJE Y EVALUACIÓN EN LA EDUCACIÓN. Revista Internacional ReNoSCol. 2021:75-81. [CrossRef] | [Google Scholar]
- Albor-Licona MA. Innovación educativa, camino de permanente reflexión y transformación. Ciencia Y Academia. 2023:4 [CrossRef] | [Google Scholar]
- Geraldo-Campos L, Sánchez A, Esther Perez. Importancia de los estilos de liderazgo: un abordaje de revisión teórica. Apuntes Universitarios. 2020;10:156-174. [CrossRef] | [Google Scholar]
- Kalhor G, Asadi Sarijalou A, Sharifi Sadr N, Bahrak B. A new insight to the analysis of co-authorship in Google Scholar. Applied Network Science. 2022;7(1):1-17. [CrossRef] | [Google Scholar]
- Zhou T, Pan R, Zhang J, Wang H. An attribute-based Node2Vec model for dynamic community detection on co-authorship network. Computational Statistics. 2024:1-28. [CrossRef] | [Google Scholar]
- Cudina JN, Ossa JC, Jacó-Vilela AM. Redes de colaboración científica en la investigación historiográfica de la psicología en América Latina. Revista Interamericana de Psicologia = Interamerican Journal of Psychology. 2021;55(3) [CrossRef] | [Google Scholar]
- De Lima LGA, De Carvalho RB, Do Rocio Fontoura Teixeira M. Internacionalização: Mapeamento de redes de coautoria internacional de bolsistas do Programa de Pós-Doutorado no exterior da CAPES na área de saúde. Revista Ibero-Americana de Estudos em Educação. 2024:e024005-e024005. [CrossRef] | [Google Scholar]
- Gao J, Nyhan J, Duke-Williams O, Mahony S. Exploring international collaboration and language dynamics in Digital Humanities: insights from co-authorship networks in canonical journals. Journal of Documentation. 2023;79(7):240-260. [CrossRef] | [Google Scholar]
- Zeleke BD, Geleto AK, Asefa S, Komicha HH. The role of social capital in addressing seed access constraints and adoption intensity: Evidence from Arsi Highland, Oromia Region, Ethiopia. Heliyon. 2023;9(2) [CrossRef] | [Google Scholar]
- Ma’ruf A, Yandhi AN, Athief FHN. Bibliometric and visual analysis of research on ethical finance. Multidisciplinary Reviews. 2024;7(5):2024099-2024099. [CrossRef] | [Google Scholar]
- Aranibar Melgar EE, Travieso Valdés D. Gestión del conocimiento, capital intelectual y producción académico-científica en el posgrado: un estudio diagnóstico. Revista Cubana de Educación Superior. 2023;42(1) [CrossRef] | [Google Scholar]
- Burt RS. Structural Holes: The Social Structure of Competition. 1995a [CrossRef] | [Google Scholar]
- Dahiyat SE, Khasawneh SM, Bontis N, Al-Dahiyat M. Intellectual capital stocks and flows: examining the mediating roles of social capital and knowledge transfer. VINE Journal of Information and Knowledge Management Systems. 2023;53(1):11-42. [CrossRef] | [Google Scholar]
- Granovetter M. Economic action and social structure: The problem of embeddedness. The American Journal of Sociology. 1985;91(3):481-510. [CrossRef] | [Google Scholar]
- Li H, Sewell DK. A comparison of estimators for the network autocorrelation model based on observed social networks. Social Networks. 2021;66:202-210. [CrossRef] | [Google Scholar]
- Faraj S, Kudaravalli S, Wasko M. Leading Collaboration in Online Communities. The Mississippi Quarterly. 2015;39(2):393-412. [CrossRef] | [Google Scholar]
- Lenoir R. Bourdieu, diez años después: legitimidad cultural y estratificación social. Cultura Y Representaciones Sociales. 2012 https://www.scielo.org.mx/scielo.php?p id=S2007-81102012000100001andscript=sci_arttext [CrossRef] | [Google Scholar]
- Tyndall DE, Forbes TH, Iii Avery JJ, Powell SB. Fostering scholarship in doctoral education: Using a social capital framework to support PhD student writing groups. Journal of Professional Nursing: Official Journal of the American Association of Colleges of Nursing. 2019;35(4):300-304. [CrossRef] | [Google Scholar]
- Jalali ZS, Introne J, Soundarajan S. Social stratification in networks: insights from co-authorship networks. Journal of the Royal Society, Interface / the Royal Society. 2023 [CrossRef] | [Google Scholar]
- Vicencio-Ríos G, Rubio A, Araya-Castillo L, Moraga-Flores H. Scientometric Analysis of Brand Personality. Sustainability: Science Practice and Policy. 2022;15(1):731 [CrossRef] | [Google Scholar]
- Cos IL, Urrutia-Gutierrez S, Cos GL, Arribas-Galarraga S. Efecto de un programa de educación física emocional sobre la inteligencia emocional de futuros docentes. Journal of Sport and Health Research. 2024;16(Supl. 1):9-20. [CrossRef] | [Google Scholar]
- Barnett GA, Park HW. Co-authorship among the Fellows of the International Communication Association. Scientometrics. 2023;128(6):3401-3418. [CrossRef] | [Google Scholar]
- Ullah M, Shahid A, Din IU, Roman M, Assam M, Fayaz M, Ghadi Y, Aljuaid H, et al. Analyzing Interdisciplinary Research Using Co-Authorship Networks. Complexity. 2022 [CrossRef] | [Google Scholar]
- Affonso F, Santiago M de O, Rodrigues Dias TM. Analysis of the evolution of scientific collaboration networks for the prediction of new co-authorships. Transinformação. 2022;34 [CrossRef] | [Google Scholar]
- Alzina RB, Cassa EL. La evaluación en la educación emocional: Instrumentos y recursos. Aula Abierta. 2021;50(4):757-66. [CrossRef] | [Google Scholar]
- Sansuán RZ. Críticas constructivas a la educación emocional. Teoría de la Educación. Revista Interuniversitaria. 2024;36(1):95-118. [CrossRef] | [Google Scholar]
- Essers D, Grigoli F, Pugacheva E. Network effects and research collaborations: evidence from IMF Working Paper co-authorship. Scientometrics. 2022;127(12):7169-7192. [CrossRef] | [Google Scholar]
- Mehralian G, Nazari J, Ghasemzadeh P. The effects of knowledge creation process on organizational performance using the BSC approach: the mediating role of intellectual capital. Journal of Knowledge Management. 2018:22 [CrossRef] | [Google Scholar]
- Bornhauser N, Rivera JMG. La educación emocional: prácticas y discursos de subjetivación. Teoría de la Educación. Revista Interuniversitaria. 2023;35(1):101-122. [CrossRef] | [Google Scholar]
- Aria M, Corrado C. Bibliometrix: An R-Tool for Comprehensive Science Mapping Analysis. Journal of Informetrics. 2017;11(4):959-75. [CrossRef] | [Google Scholar]
- Robledo S, John-Eider V, Néstor-Darìo D-M, Duque-Uribe V. Networking as an entrepreneurial marketing tool: the link between effectuation and word of mouth. Journal of Research in Marketing and Entrepreneurship. 2022;25(2):270-285. [CrossRef] | [Google Scholar]
- Zuluaga M, Robledo S, Arbelaez-Echeverri O, Osorio-Zuluaga GA, Duque-Méndez N. Tree of Science – ToS: A Web-based Tool for Scientific Literature Recommendation. Search Less, Research More! Issues in Science and Technology Librarianship. 2022:100 [CrossRef] | [Google Scholar]
- Grisales AAM, Robledo S, Zuluaga M. [Retrieved May 20, 2024];Topic Modeling: Perspectives From a Literature Review. (n.d.) [CrossRef] | [Google Scholar]
- Robledo S, Zuluaga M, Valencia L-A, Arbelaez-Echeverri O, Duque P, Alzate-Cardona J-D, et al. Tree of Science with Scopus: A Shiny Application. Issues in Science and Technology Librarianship. 2022:100 [CrossRef] | [Google Scholar]
- Zuluaga M, Robledo S, Arbelaez-Echeverri O, Osorio-Zuluaga G, Duque-Méndez N. “Tree of Science – ToS: A Web-Based Tool for Scientific Literature Recommendation. Search Less, Research More!”. Issues in Science and Technology Librarianship. 2022:100 [CrossRef] | [Google Scholar]
- Hurtado-Marín VA, Agudelo-Giraldo JD, Robledo S, Restrepo-Parra E. Analysis of dynamic networks based on the Ising model for the case of study of co-authorship of scientific articles. Scientific Reports. 2021;11(1):5721 [CrossRef] | [Google Scholar]
- Burt RS. Structural Holes: The Social Structure of Competition. 1995 [CrossRef] | [Google Scholar]
- Nahapiet J, Ghoshal S. Social Capital, Intellectual Capital and the Organizational Advantage. AMRO. 1998;23(2):242-266. [CrossRef] | [Google Scholar]
- De Oliveira Passos M, Gonzalez PL, Tessmann MS, de Abreu Pereira Uhr D. The greatest co-authorships of finance theory literature (1896-2006): scientometrics based on complex networks. Scientometrics. 2022;127(10):5841-5862. [CrossRef] | [Google Scholar]
- HabibAgahi MR, Ali Kermani MA, Maghsoud M. On the Co-authorship network analysis in the Process Mining research Community: A social network analysis perspective. Expert Systems with Applications. 2022;206:117853 [CrossRef] | [Google Scholar]