ABSTRACT
Inverse optimization is defined as a process of reverse traditional mathematical optimization where parameters of an optimization model are determined such that given decisions will be either approximately or precisely optimal. The concept of inverse optimization was initially introduced and formulated in 2001. Research in this field has both theoretical and practical applications and has been applied in various areas. This study utilizes bibliometric analysis to determine the most impactful studies and researchers in the field of inverse optimization. Our research objective is to examine the current state of inverse optimization studies, based on data from the Web of Science database. Several classifications including leading journals, most frequently cited papers and influential authors are made. The results indicate that the majority of inverse optimization applications have been in the realm of cancer treatment and that the number of studies in this field has decreased during 2019-2020, that might be due to COVID-19 pandemic. Also, the USA and MIT (Massachusetts Institute of Technology) are among the most productive and influential entities in this field.
INTRODUCTION
In recent decades, inverse optimization has garnered significant attention among researchers in the fields of mathematics and operations research. The objective of inverse optimization is to ascertain the parameters of an optimization model, often called the āforward model.ā This process aims to achieve a set of specified decisions that are either approximately or precisely optimal. This concept is further elucidated in Figure 1.
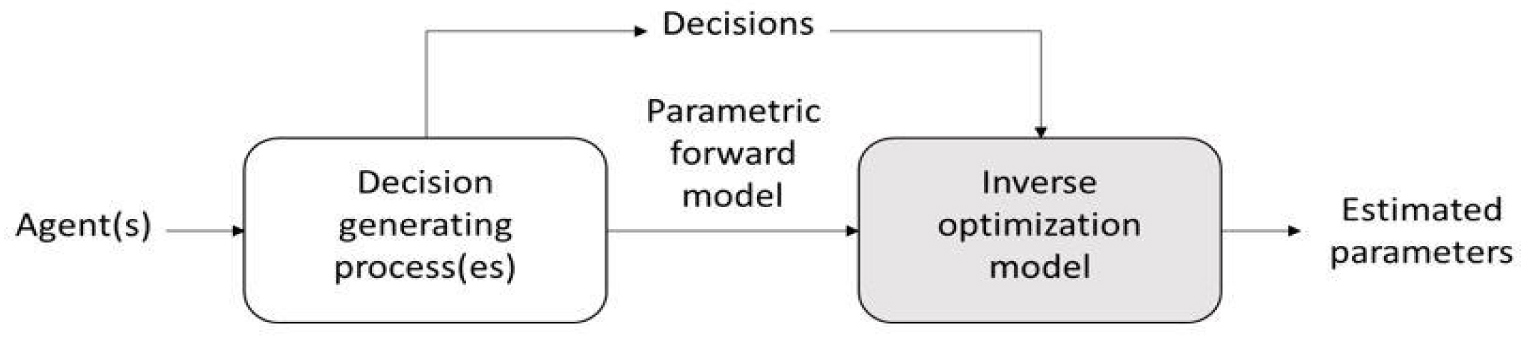
Figure 1:
Methodology of Inverse Optimization.[1]
The initial application of inverse problems in geophysical sciences can be traced back to Tarantola’s groundbreaking work in 1987.[2] Following this, Ahuja made a landmark contribution to the field in 2001, formally introducing the concept of inverse optimization. Ahuja’s model, significant in its approach, aims to minimally alter a predefined cost vector to make a specific observed solution within a feasible space optimal. This research avenue has been explored across various domains, including transportation, healthcare, robust optimization and sustainability, among others. In these contexts, inverse optimization serves to craft decision-making models that render a known set of decisions as optimal or nearly optimal.[3]
The theoretical development of inverse optimization has evolved substantially over time. Traditional methods, as elucidated by Ahuja and others, primarily focused on parameter extraction that ensured a single solutionās optimality. However, recognizing the challenge of achieving absolute optimality in complex scenarios, the field has seen a shift toward generalized models. Expanding the boundaries of the field, Chan introduced a general approach to tackle sub-optimal or infeasible solutions in linear programming, leveraging the concepts of strong duality and dual feasibility.[4] Troutt extended the inverse linear programming model to include multiple feasible solutions, employing the principle of decisional regret to guide the modelās development.[5,6] Ghobadi addressed the uncertainties inherent in observed data through robust inverse optimization, creating models equipped to manage worst-case scenarios.[7] The advancement in methodologies continued with Saez, Keshavarz and Bertsimas utilizing Karush-Kuhn-Tucker (KKT) conditions, the introduction of slack variables and the application of variational inequalities, respectively, to further refine inverse optimization models for diverse data situations.[8ā10]
In a notable advancement, Aswani proposed an innovative loss function to quantify the discrepancy between measured and estimated data, emphasizing the joint estimation of optimization problems and their optimal solutions.[11] Chanās comprehensive framework for inverse linear optimization specifically focuses on cases with a single observed feasible solution, incorporating a fit metric to assess the precision of the inverse solution.[12]
To the best of our knowledge, none have examined the state of the field from a bibliometric perspective. Bibliometric analysis, increasingly popular across various scientific communities, is an essential tool for identifying patterns in scientific contributions.[13] In this study, we aim to offer a comprehensive overview of research in the field of inverse optimization through a bibliometric approach.
To achieve this goal, we use the Web of Science (WoS) database, which has been a significant repository of bibliometric data since its creation by the Thomson Reuters Institute of Scientific Information in 1960. Covering the period from 2010-2020, WoS is one of the largest databases of its kind, providing easy access to bibliometric data.[14] It collects a wide range of bibliographic information, citations and references from scientific publications in various disciplines, dating back to 1945.
This study makes a significant contribution to the literature by providing current insights into the state of inverse optimization, offering a detailed view of recent publications from a bibliometric perspective. This information will be invaluable for researchers, aiding in the improvement of future studies in the field. Additionally, we seek to visualize scholarly networks and developments in inverse optimization to identify potential areas for future research collaboration.
Section 2 presents the research aims and objectives. Section 3 describes the methodology of our study. The results are presented in Section 4. The discussion and concluding remarks are in Sections 5 and 6, respectively.
RESEARCH AIMS AND OBJECTIVES
This study aims to provide a comprehensive bibliometric analysis of the field of inverse optimization. Our goal is to dissect the academic landscape of this domain, identifying key trends, leading contributors and prominent areas of application. By examining the bibliometric data extracted from the Web of Science database, we intend to offer an overview of the field’s developmental trajectory and significant research outputs. Our research objectives are as follows:
To Catalogue the Most Impactful Research and Researchers
Identify and highlight the most influential studies, authors and institutions in the field of inverse optimization, thereby recognizing the pivotal contributions to the domain.
To Analyze Trends in Research Themes and Applications
Examine the evolution of research themes within inverse optimization, particularly focusing on areas like cancer treatment and other prominent applications.
To Explore Collaborative Networks
Investigate the nature and extent of collaborative research in inverse optimization, including institutional partnerships.
Our research questions are listed below
What are the key trends in Inverse Optimization Research? Which topics have dominated the field?
Who are the leading contributors in the Field? Which authors, institutions and countries have been most influential in shaping the field?
What future directions can be derived from the current state of research? Based on current trends and gaps, what potential research areas appear promising?
By addressing these questions, this research seeks to offer a holistic view of the inverse optimization field, shedding light on its historical progression, current state and potential future trajectory.
Bibliometric Method
The methodology used in bibliometric analysis and data visualization helps researchers to understand different aspects of studies in a field. Machine learning, big data algorithms and ANOVA are some of the approaches used in bibliometric analysis. In some studies like[15] and[16] ANOVA was used. Some others like[17ā21] utilized big data and machine learning or deep learning, respectively. Many studies have also explored the use of big data, such as[22ā24] Additionally, bibliometric and social network analysis has been applied in.[25]
To the best of our knowledge, there has not been a study in inverse optimization that has used these analysis methods. This study will provide valuable insights and contribute to the literature by using bibliometric analysis to assess the current state of inverse optimization research.
The research process of the present study involves the following steps
Data Collection
782 records are extracted from the WoS database using the search query “Inverse Optimization” in the title or abstract without any time period limitations. These records were analyzed based on annual publications, the publication of the most productive countries, institutions, journals and authors. The data were extracted from the WoS database.
Bibliometric Analysis
The descriptive bibliometric analysis was conducted to determine the most effective studies and researchers in the field of interest. Using VOSviewer and Microsoft Excel the strongest collaborative relationships were identified. These tools also used to depict co-citation, co-authorship, co-occurrence and bibliographic coupling networks of countries, institutions, journals, authors and papers.
Conclusions on Academic Research Trend
This part of the analysis reveals the key trends and patterns in the field of Inverse Optimization, identifying the most influential contributors and the evolution of research themes over time.
RESULTS
The subsequent section details the results obtained through the utilization of VOSviewer, a tool for constructing and visualizing bibliometric networks, in conjunction with data sourced from WoS database. This combination allows for an in-depth analysis and visual representation of the research trends, patterns and key contributors in the field of inverse optimization.
Publications Overview
The number of publications is a key metric for gauging the development trend of scientific research.[26] Figure 2 illustrates the types of records in our dataset, revealing an overall upward trajectory in the volume of papers published in the field of Inverse Optimization. However, this growth trend seems to have decelerated post-2018, with an exception in 2021. This observation is consistent with the global decline in non-COVID- related publications during the COVID-19 pandemic.[27] Furthermore, Figure 2 provides an analysis of the distribution of data by publication types, encompassing articles, proceeding papers, editorial materials, meeting abstracts and reviews within the realm of Inverse Optimization.
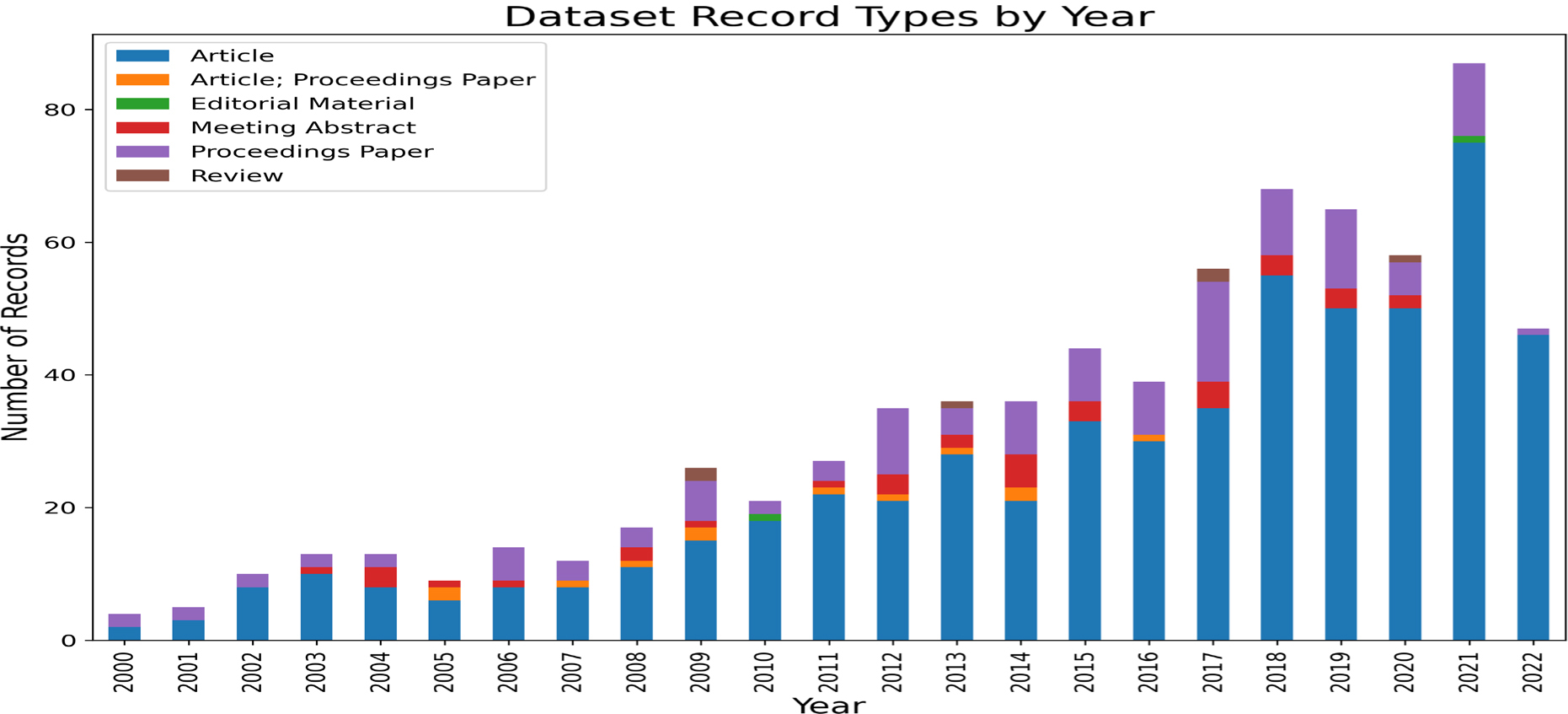
Figure 2:
Annual distribution of publications on “Inverse Optimization” in the WoS database.
Overview of the Keywords
A keyword analysis of the titles and abstracts in our dataset was conducted to identify prevalent research themes in inverse optimization. Figure 3 depicts the network structure of keywords used in these publications, with Figure 3 highlighting that the size of the nodes correlates with keyword usage frequency. Notably, the three most frequent keywords used alongside āInverse Optimizationā are āoptimization,ā āalgorithm,ā and āradiation therapy.ā Moreover, the top five specific keywords, excluding more general terms, include āradiation therapy,ā ābrachytherapy,ā ācancer,ā āIMRTā (Intensity-Modulated Radiation Therapy) and ācombinatorial optimization.ā This suggests a significant focus of inverse optimization within the healthcare sector, particularly in cancer treatment. Additionally, the keyword āinverse problemā appears frequently, reflecting its conceptual overlap yet distinctness from inverse optimization.
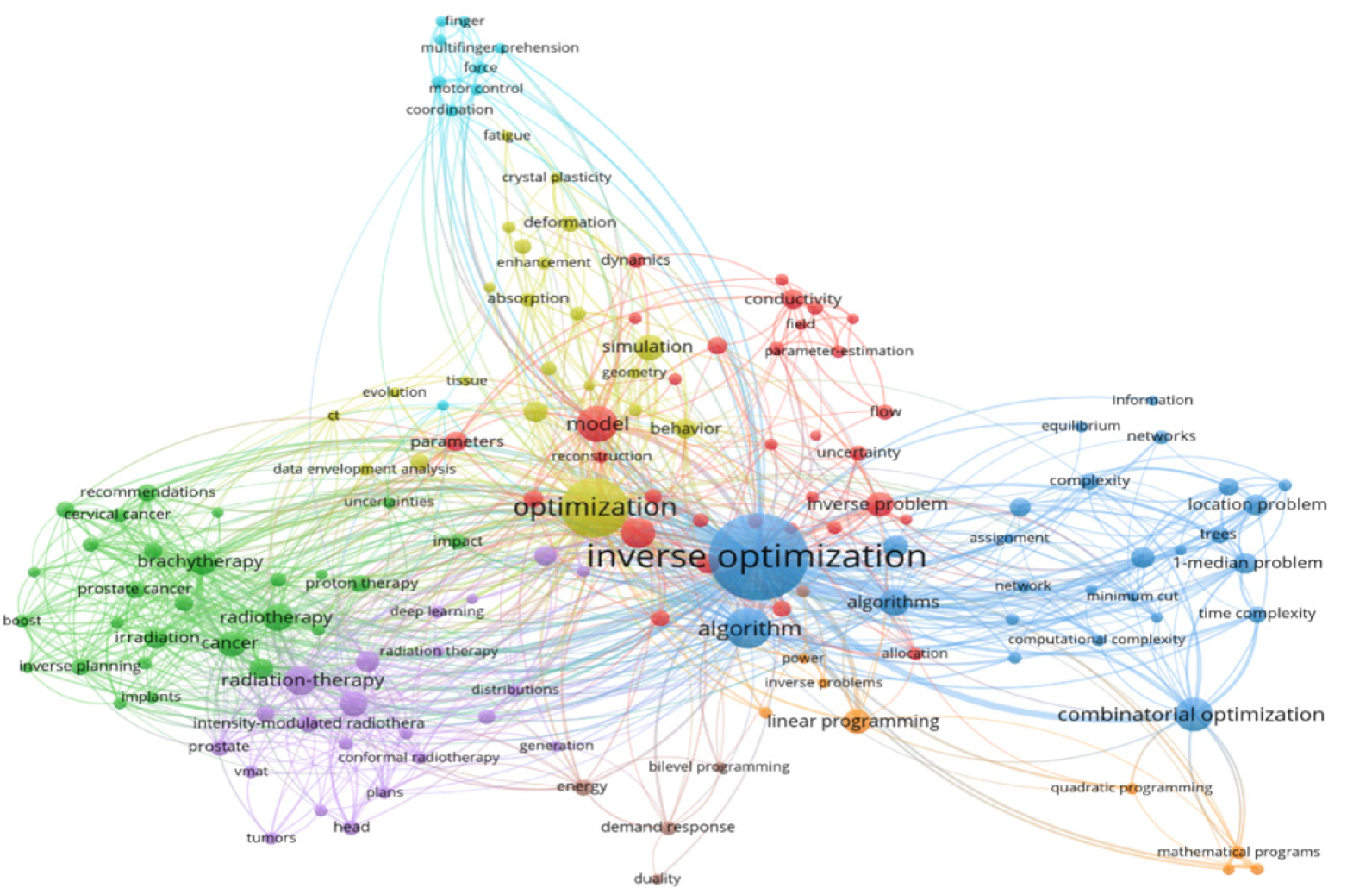
Figure 3:
Term intersection diagram in inverse optimization domain.
As outlined in study,[1] inverse optimization falls within the broader category of āinverse problems,ā which utilize data on the outcomes of a process to infer the causative factors.[28,29] However, inverse optimization is distinct in its approach, focusing on decision-making problems modeled through mathematical programming and utilizing solutions to these problems as data. Consequently, the methodologies and applications of inverse optimization diverge from the general field of inverse problems.
In Figure 3, as per the VOSviewer manual, the link strength denotes the co-occurrence relationship between two keywords. The thickness of each line reflects this strength, with thicker lines indicating a more robust connection between keywords. Furthermore, the size of a node in the graph correlates directly with the frequency of the keyword’s occurrence; larger nodes represent a higher occurrence. Clusters also indicate potential for interdisciplinary collaboration. For instance, if a cluster connects keywords from both healthcare and mathematical modeling, it suggests opportunities for collaboration between these fields.
The analysis of the co-occurring terms with “Inverse Optimization” in Figure 3 predominantly highlights its significant application in healthcare, particularly in cancer treatment through radiation therapy and brachytherapy. Frequent references to “Optimization,” “Algorithm,” and “Combinatorial Optimization”, 134, 63 and 43, respectively, underscore the essential role of algorithmic and optimization methods in this domain. The presence of specific cancer-related terms like “IMRT” and “Prostate Cancer,” along with methodological terms like “Model” and “Simulation,” reflects the field’s interdisciplinary nature and its focus on improving treatment quality and planning. The strong linkages between these terms suggest concentrated research activity and potential collaborative efforts within healthcare optimization, especially in oncology. Also, keywords clustered around “inverse optimization” and “algorithm” represents a research area focusing on the development of algorithms specific to inverse optimization problems.
Overview of the Countries
Table 1 presents the data on countries with the highest frequency of publications in inverse optimization, showcasing both the number of articles and their citation counts for the top 10 countries. As detailed in Table 1, the United States emerges as the leader in publication frequency, followed by China and Canada in second and third positions, respectively.
Country | Documents | Citations | Citations/Documents | Total link strength |
---|---|---|---|---|
USA | 283 | 4264 | 15.07 | 108 |
Peoples R China | 156 | 1262 | 8.09 | 62 |
Canada | 58 | 744 | 12.83 | 35 |
France | 49 | 563 | 11.49 | 33 |
Germany | 49 | 649 | 13.24 | 30 |
Belgium | 19 | 252 | 13.26 | 26 |
Austria | 20 | 610 | 30.50 | 22 |
South Korea | 17 | 163 | 9.59 | 17 |
England | 23 | 398 | 17.30 | 16 |
Spain | 17 | 372 | 21.88 | 16 |
Additionally, we have introduced an index measuring citations per document. According to Table 1, Austria, Spain and England rank highest on this index, indicating their respective impacts in terms of citation frequency relative to their document count.
The data from Table 1 on leading nations in inverse optimization research suggests several implications. The USA is the clear leader, with the highest number of documents, citations and total link strength, indicating its dominant role in the field. China follows, with a significant presence but lower impact in terms of citations per document. Canada, France and Germany also show strong contributions, with notable citation rates that suggest quality research output. Interestingly, Austria, despite a smaller number of documents, shows a high citation per document ratio, indicating highly impactful research. Similarly, Spain and England, while having fewer documents, show high citations rates, pointing to influential contributions in the field. The presence of countries like Belgium and South Korea highlights the global interest and diverse geographical representation in inverse optimization research. Overall, these statistics reveal a field with substantial and varied contributions from several key nations, reflecting both the quantity and quality of research across the globe.
The countries with the highest scientific production often have the highest scientific impact because a larger volume of documents generally leads to a greater likelihood of citations, reflecting a broader influence in the scientific community. However, Spain and Austria stand out for their high citation/document ratio, indicating that although they produce fewer documents compared to leading countries like the USA, their research has a significantly high impact per document. This could be due to the focused quality of research, specific niches of expertise, or higher collaboration rates with internationally renowned researchers, which enhances the visibility and citation rate of their publications. In contrast, countries with higher overall citations might benefit from a larger scientific community and a wider range of research areas, contributing to their total citation count despite a lower citation/document ratio.
Top of Form
Top of Form
Overview of the Institutions
Table 2 presents the institutions that published articles, along with the number of citations received by each institution and the most frequently used author keywords (For the table of the top 30 institutions, see Appendix A.) As evident from Table 2 and Table 5 (Appendix A.), the institution with the highest strength of link is the University of Toronto. The Massachusetts Institute of Technology (MIT) received the most citations (683). It is important to note that the number of citations is heavily influenced by the quality and productivity of scientific publications.
Organization | Documents | Citations | Citations/Documents | Total link strength | Author Keywords |
---|---|---|---|---|---|
University of Toronto. | 21 | 524 | 24.95 | 11 | Inverse optimization; Linear Programming; Treatment Planning; Machine Learning; Knowledge-Based Planning; Radiation Therapy; Clinical Pathway Concordance; Cancer Therapy (e.g., colon cancer, breast cancer, prostate cancer); Multiagent System; Network Analysis. |
Massachusetts General Hospital. | 7 | 50 | 7.14 | 10 | Proton Therapy; Pencil Beam Scanning (PBS); Intensity Modulated Proton Therapy (IMPT); Alternating Direction Method of Multipliers (ADMM); Knowledge-Based Planning; Optimization; Inverse Optimization. |
Emory University | 11 | 77 | 7.00 | 9 | Proton Therapy; Pencil Beam Scanning (PBS); Intensity Modulated Proton Therapy (IMPT); Alternating Direction Method of Multipliers (ADMM); Knowledge-Based Planning; Optimization; Inverse Optimization. |
Harvard Medical School | 6 | 52 | 8.67 | 9 | Proton Therapy; Pencil Beam Scanning (PBS); Intensity Modulated Proton Therapy (IMPT); Alternating Direction Method of Multipliers (ADMM); Minimum Monitor Unit (MU); Knowledge-Based Planning; Inverse Treatment Planning; Brachytherapy. |
MIT | 15 | 683 | 45.53 | 8 | Inverse Optimization; Robust Optimization; Linear Programming; Market Elements (Electricity Pool, Marginal Prices, Market Clearing); Mechanism Design; Auctions (Multiattribute Auctions); Equilibrium; Estimation (Nonparametric Estimation, Utility Estimation); Traffic Assignment; Flow Problems (Maximum-Flow Problem, Minimum-Cut Problem, Minimum-Cost Flow Problem, Minimum Mean-Cycle Problem); Combinatorial Optimization; Computational Complexity; Mixed-Integer Programming. |
Also, common topics are observed among the institutions. For example, both Massachusetts General Hospital and Emory University share several common keywords, including IMPT, ADMM, Knowledge-Based Planning, etc. Upon reviewing related articles, we have observed collaboration between them.[30ā33]
The implications of Table 2 and Table 5 (Appendix A.), highlighting the institutions with the highest overall link strength in inverse optimization research, are multifaceted. The University of Toronto, leading in link strength, suggests a central role in fostering collaborative research networks. MIT’s highest citation count reflects its significant impact and prestige in the field. Notably, the Sahand University of Technology, with the most documents (282, see Appendix A.), indicates a strong focus on inverse optimization research, possibly signifying a specialized or emerging research area within the institution. The high citations per document ratio for the University of Florida (50.33), Graz University of Technology (47.8) and MIT demonstrate not only prolific research output but also high-quality and influential contributions to the field. These tables reveal a diverse set of institutions varying in their research focus, be it in terms of quantity, quality, or collaborative influences. Such diversity underscores the multidisciplinary and global nature of inverse optimization research, highlighting institutions that are key nodes in this expanding research network. These institutions, from different parts of the world, reflect the global interest and varied perspectives contributing to advancements in the field.
Top of Form
Leading Journals in Inverse Optimization
To provide a comprehensive overview of the most influential journals in the field of inverse optimization, we have ranked the top ten journals based on their number of publications. Although the number of publications may not necessarily reflect the quality of a journal, it can provide an indication of the status and prominence of journals in this field.
According to our ranking, the top three journals in the field of inverse optimization are Medical Physics, Physics in Medicine and Biology and the European Journal of Operational Research (EJOR) (as depicted in Figure 4). As previously discussed, a majority of papers in inverse optimization tend to focus on cancer treatment and this trend is reflected in our journal ranking as well. Among the top three journals, EJOR boasts the highest impact factor, having received a significant number of citations. In addition to EJOR, other journals included in the ranking, such as Operations Research Letters, Operations Research and Journal of Global Optimization, focus on operations research field while other journals focus on fields such as cancer treatment, radiation therapy and healthcare.
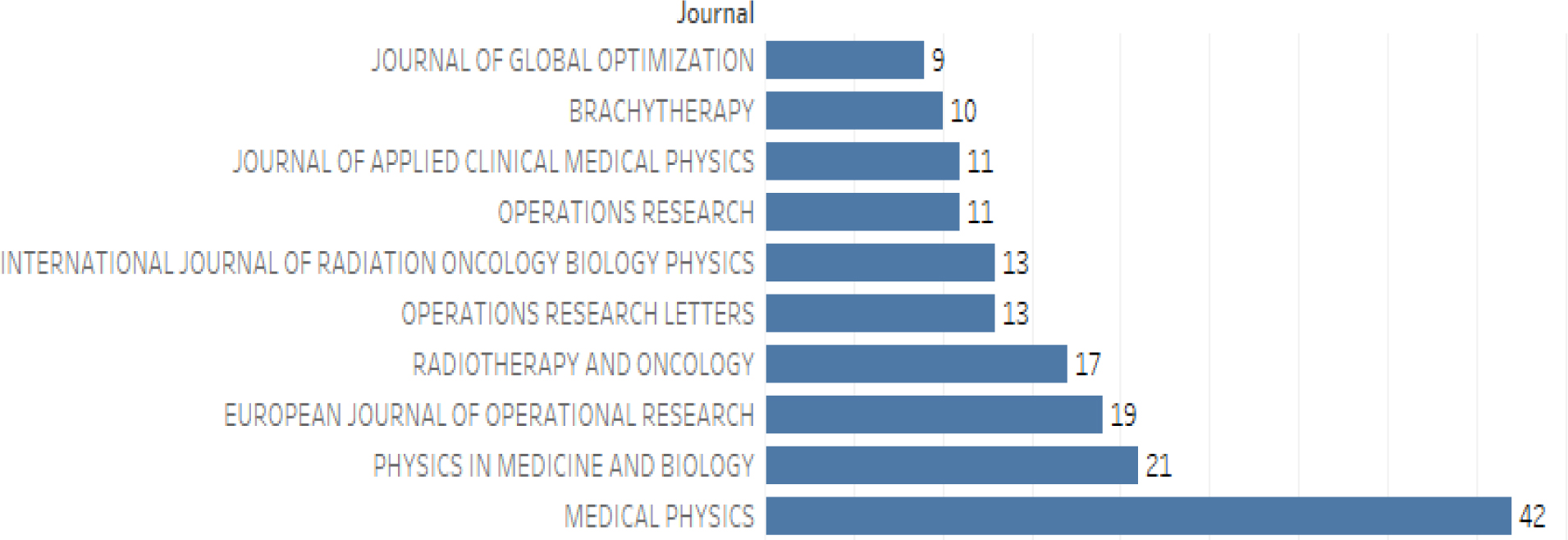
Figure 4:
Publications from the top 10 highest-publishing journals.
Leading journals are shaping the field of inverse optimization by serving as pivotal platforms for interdisciplinary collaboration, particularly between operational research and healthcare. In this regard, we can see those journals such as Brachytherapy, Journal of Applied Clinical Medical Physics, International Journal of Radiation Oncology Biology Physics, Radiation Oncology, Physics in Medicine and Biology and Medical Physics can all be categorized within the medical field. With top-tier journals like Medical Physics and Physics in Medicine and Biology focusing on the medical applications of physics, particularly in cancer treatment, they steer research toward practical and impactful healthcare solutions. Meanwhile, the European Journal of Operational Research and similar publications emphasize the theoretical and methodological rigor, ensuring that the advancements in the field are both scientifically robust and applicable. The frequency of publications in these journals reflects active research directions, with high citation rates underscoring the quality and influence of the research disseminated, thereby setting the tone for current and future research priorities within the field.Top of Form
Most Widely Cited Articles in Inverse Optimization
In Table 3, the top 5 most-cited articles from the period of 2010-2020 are presented, ranked by citation count according to data sourced from WoS (For the table of the top 30 most cited articles, see Table 6 Appendix B). The table includes detailed entries with authors’ full names, journal names and keywords. Notably, the foremost paper in the field of inverse optimization by Ahuja and Orlin, which established and formalized the concept of inverse optimization. This seminal work has been pivotal in the field, reflected by its citation count of 290. The second-most cited article, a review written by Heuberger, has accrued over 200 citations, denoting significant recognition and impact. The articles appear to cover a range of topics, most notably focusing on optimization in different contexts, including combinatorial optimization and optimization in relation to physics, auctions, self-assembly, physiological modeling, material science and renewable energy. The number of citations for these articles ranges from below 100 to nearly 300, indicating their impact and relevance in their respective fields.
Article Title | Times Cited, All Databases | Author Full Names | Journal Abbreviation | Author Keywords |
---|---|---|---|---|
Inverse optimization | 290 | Ahuja, RK; Orlin, JB | OPER RES | |
Inverse combinatorial optimization: A survey on problems, methods and results. | 211 | Heuberger, C | J COMB OPTIM | Inverse optimization; reverse optimization; network flow problems. |
Learning physics-based motion style with nonlinear inverse optimization. | 182 | Liu, CK; Hertzmann, A; Popovic, Z | ACM T GRAPHIC | Character animation; motion style; physics-based animation; inverse optimization. |
An inverse-optimizationbased auction mechanism to support a multiattribute RFQ process. | 160 | Beil, DR; Wein, LM | MANAGE SCI | Inverse optimization; multiattribute auctions; mechanism design. |
Inverse optimization techniques for targeted self-assembly. | 150 | Torquato, Salvatore | SOFT MATTER |
The high citation rates of these articles suggest they address subject matters of substantial importance within their respective fields. The lead article by Ahuja and Orlin is often cited because it laid the foundational framework for inverse optimization, an area of study with wide-ranging applications in logistics, network design and resource allocation. Its prominence is attributed to its introduction of new methodologies that have since become standard in the field. Heubergerās review paper, by systematically summarizing problems, methods and results in inverse combinatorial optimization, has likely become a key reference point for researchers and practitioners alike, explaining its extensive citation count. Review papers typically garner high citations as they provide a synthesis of existing literature and offer direction for future research efforts. The subject matter of these highly cited articles deals with foundational and methodological advances in inverse optimization. Their extensive citation is likely due to the novelty, applicability and the ongoing development in the inverse optimization discipline.
The table also highlights the interdisciplinary nature of inverse optimization, showing its application from theoretical problems in operations research to practical issues in energy policy planning. The citation counts reflect the academic community’s recognition of the importance of these works. The presence of high citation counts also implies that the concepts developed in these articles are foundational and continue to influence ongoing research across various disciplines. This could be particularly valuable for researchers looking for seminal works in the field or for institutions aiming to develop programs or courses based on the most impactful research.
Also, as demonstrated in Figure 5, approximately 25% of all the papers considered in this field, or around 200 papers, have received no citations. Additionally, only a limited number of articles, less than 25, have received more than 10 citations.
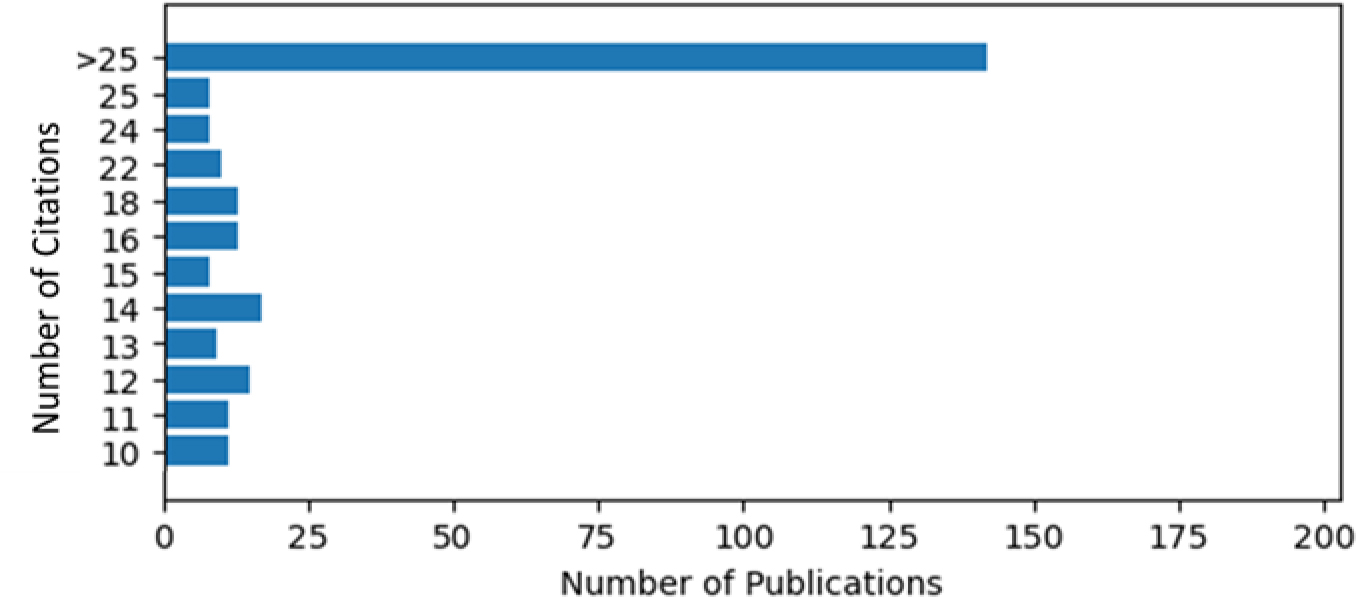
Figure 5:
Citations received by papers.
Figure 5 depicts a skewed distribution of citations among papers in inverse optimization, highlighting a common trend in academic research where a small fraction of work receives the majority of attention. The fact that about 25% of papers have not been cited suggests there could be a large amount of research in the field that is not being utilized or built upon by other academics. This may indicate areas where research topics are less appealing or too niche for the wider research community, or that some papers may lack the novelty or relevance required to attract citations. On the other end, the few papers that have garnered more than 10 citations represent the more influential research within inverse optimization, potentially introducing seminal concepts, innovative methodologies, or comprehensive reviews that resonate with and are used by other researchers. These highly cited articles are likely to be the ones setting the agenda and influencing the direction of future research in the field. The disparity also underscores the importance of effective dissemination and networking within the academic community, as well as the potential impact of publishing in high-visibility journals.Top of Form
Most Prolific and Influential Authors
Table 4 presents the authors with the highest total link strength, accompanied by the number of citations and documents attributed to them, as well as their affiliated institutions and the keywords frequently associated with inverse optimization in their work (For the table of the top 30 the highest overall link strength, see Appendix C.) As depicted in Table 4 and Table 7 (Appendix C), Latash, Mark L. stands out as the author with the highest number of citations. Furthermore, Alizdeh, Behrooz has the largest number of publications on this topic, with 22 documents.
Author | Documents | Citations | Citations/Documents | Total link strength | Institution | Frequent Keywords within Inverse Optimization |
---|---|---|---|---|---|---|
Alizadeh, Behrooz | 22 | 239 | 10.86 | 28 | Sahand University of Technology | Inverse Optimization; Combinatorial Optimization; Location Problem; Time Complexity; p-Median Location; Median Location; Facility Location; Obnoxious Center Location. |
Baroughi, Fahimeh | 14 | 46 | 3.29 | 22 | Sahand University of Technology | Inverse Optimization; Combinatorial Optimization; Location Problem; p-Median; Time Complexity; Median Location; Facility Location Problem. |
Afrashteh, Esmaeil | 8 | 42 | 5.25 | 16 | Sahand University of Technology | Inverse Optimization; Combinatorial Optimization; Time Complexity; Median Location; p-Median Location; Facility Location Problem.Top of Form |
Latash, Mark L. | 13 | 254 | 19.54 | 16 | Pennsylvania State University | Inverse Optimization; Synergy; Finger; Force; Redundancy; Prehension Synergy; Optimization; Uncontrolled Manifold Hypothesis.Top of Form |
Zatsiorsky, Vladimir M. | 12 | 235 | 19.58 | 15 | Pennsylvania State University | Inverse Optimization; Optimization; Grasping; Synergy; Finger; Force; Redundancy; Uniqueness Theorem; Principal Component Analysis.Top of Form |
Additionally, Chan, Timothy C. Y., who is widely recognized as a prominent researcher in this field, holds the third place in terms of citations.
Table 4 and Table reveal a landscape where a diverse group of authors contributes to the field of inverse optimization, each with varying degrees of influence and specialization. Alizadeh, Behrooz emerges as the most prolific author, suggesting a significant impact on the breadth of the field with the highest number of publications. Latash, Mark L (254). and Chan, Timothy C. Y. (249) stand out for their high citation counts, indicating that their work resonates strongly with the research community and likely drives the field’s progression (See Table Appendix B.). The varying total link strengths point to different degrees of collaborative engagement and influence within the research network.
As it is shown in Tables 2 and 4, the institutions of highly cited authors may not match the highly cited institutions. It may have different reasons, some of them described below.
Mobility of Scholars
Authors may change institutions and their citations often continue to accrue after they have moved. Their new institutions wouldn’t immediately receive the credit for the citations.
Collaborations
Highly cited papers may be the result of collaborations among researchers from multiple institutions.
Aggregated Data
Citation counts for institutions may aggregate the citations of all researchers currently affiliated, regardless of where the work was done, while an author’s citations follow them throughout their career.
Field-Specific Practices
Some fields might be more collaborative or have different practices in citing and authorship that could impact these metrics.
Time Lags in Reporting
There could be delays in updating citation databases with current institutional affiliations.
Institutional Support
Some institutions might have the resources to support highly cited research but may not have the most highly cited individuals if their work is spread across a wide array of researchers.
Additionally, as shown in Table 4 and Figure 3, there is a notable observation that authors who have received a high number of citations in this field do not always align with the use of highly cited keywords. This phenomenon can be attributed to several factors, which are explored further below:
Broad Influence
Authors might be cited for a broad range of work. While their most influential papers may include certain keywords, their overall citation count could be bolstered by a wider variety of publications that span different topics.
Pioneering Work
Highly cited authors may have conducted pioneering work that established new directions for a field. Such work might precede the establishment of the terms that later become highly cited keywords.
Evolving Vocabulary
The vocabulary and popular keywords of a field can evolve over time. Authors may have been highly cited for work usingterminology that was current at the time but has since been superseded or refined.
Citation Context
Citations can occur for various reasons, including criticism, comparison, or historical context, not just for endorsing or building upon the cited work. Therefore, the presence of citations does not always correlate with keyword alignment.
Keyword Generality
Highly cited keywords may be too general or broad and highly cited authors may have focused on more specific or niche topics that do not align with these broad terms.
Collaborative Works
Some authors may have a high citation count due to collaborative projects. The specific keywords might be more closely associated with their co-authors’ direct contributions.
In summary, citation frequency is a complex metric influenced by various factors, including but not limited to the direct content of the publication as signified by its keywords.
DISCUSSION
This paper’s bibliometric analysis of inverse optimization provides a comprehensive overview of the field’s development, key contributors and thematic trends. The results are examined analytically, focusing on notable aspects and their broader implications.
Emphasis on Healthcare Applications
Inverse optimization has shown a significant emphasis on healthcare, especially in cancer treatment methods like radiation therapy and brachytherapy. This trend underscores the field’s practical impact in life-saving treatments. The recurring use of keywords such as “radiation therapy,” “brachytherapy,” “cancer,” and “IMRT” highlights this focus, illustrating the intersection of inverse optimization with critical healthcare applications. This confluence underscores the fieldās theoretical value and tangible benefits in enhancing treatment quality and planning in oncology.
Geographic and Institutional Influence
The analysis reveals a geographical concentration of research activity, predominantly in the USA, followed by China and Canada. Key institutions like the Massachusetts Institute of Technology (MIT) and the University of Toronto are notable for their substantial contributions, suggesting a strong support system within these academic environments. Interestingly, countries like Austria, Spain and England, despite their lower publication volume, demonstrate high impact, as evidenced by their citations per document ratio. This indicates specialized, high-quality research outputs from these nations.
Trends in Publication and Research Focus
There has been a noticeable decline in publication frequency post-2018, with a slight increase observed in 2021. This pattern is likely attributable to the impacts of the COVID-19 pandemic, reflecting the challenges encountered by the research community during global health crises. Additionally, the presence of a substantial proportion of uncited papers indicates potential underexplored areas within the field. In contrast, a small set of highly cited papers highlights influential research, shaping the direction of inverse optimization.
Interdisciplinary Nature and Journal Influence
Publications in prominent journals such as “Medical Physics,” “Physics in Medicine and Biology,” and “European Journal of Operational Research” reflect the interdisciplinary character of inverse optimization. The melding of operational research principles with medical applications in these journals signifies a directed effort towards developing impactful healthcare solutions, maintaining scientific integrity and rigor.
Collaborative and Individual Contributions
The fieldās diversity in authorship, with varying degrees of influence and expertise, illustrates a collaborative nature within inverse optimization. Researchers like Alizadeh, Behrooz and Chan, Timothy C. Y. are noteworthy for their significant contributions to advancing the field. Furthermore, the high citation rates per document for authors such as Burkard, Rainer E., Wang, Lizhi and Park, Jaebum emphasize the quality and relevance of their work.
CONCLUSION
In this study, we have presented a bibliometric overview of the field of inverse optimization. Our findings serve as a useful guide for researchers seeking to identify influential works, including key journals, papers and authors in this domain. While our results generally align with the academic community’s perceptions, some discrepancies in numerical data are present. Notably, there has been a slowdown in the number of publications in this field, except for a spike in 2021. This may be attributed to the challenges in making novel contributions within this complex area. Additionally, the decline in non-COVID-19 research during the pandemic[27] likely influenced this trend. Most publications in inverse optimization are linked to cancer treatment or theoretical developments, with the United States and MIT being particularly prominent and influential.
It is important to acknowledge the limitations of this study, particularly regarding the quality of the journals and papers analyzed. Some authors, though having fewer publications, may contribute works of higher quality. Thus, considering citation counts and expert opinions about these authors would yield a more comprehensive assessment. This consideration is equally relevant to other elements such as institutions, countries and articles. The varying prestige and impact of different journals can influence the outcomes of our analysis. Expanding the scope to include other databases like Scopus could offer a more rounded view. For future research, integrating machine learning algorithms might enhance the depth and breadth of the analysis.
Cite this article
Bigdellou S, Ghaemmaghami A, Aslani S, Yazdi MM. A Bibliometric Analysis of Inverse Optimization Research: Trends, Impact, and Key Contributions. J Scientometric Res. 2024;13(3):x-x.
References
- Chan TCY, Mahmood R, Zhu IY. Inverse optimization: Theory and applications. arXiv Prepr arXiv210903920. 2021 [Google Scholar]
- Tarantola A. Method for data fitting and model parameter estimation. Invers Probl Theory. 1987 [Google Scholar]
- Ahuja RK, Orlin JB. Inverse optimization. Oper Res. 2001;49(5):771-83. [Google Scholar]
- Chan TCY, Craig T, Lee T, Sharpe MB. Generalized inverse multiobjective optimization with application to cancer therapy. Oper Res. 2014;62(3):680-95. [Google Scholar]
- Troutt MD, Pang W-K, Hou S-H. Behavioral estimation of mathematical programming objective function coefficients. Manage Sci. 2006;52(3):422-34. [Google Scholar]
- Troutt MD, Brandyberry AA, Sohn C, Tadisina SK. Linear programming system identification: The general nonnegative parameters case. Eur J Oper Res. 2008;185(1):63-75. [Google Scholar]
- Ghobadi K, Lee T, Mahmoudzadeh H, Terekhov D. Robust inverse optimization. Oper Res Lett. 2018;46(3):339-44. [Google Scholar]
- Saez-Gallego J, Morales JM. Short-term forecasting of price-responsive loads using inverse optimization. IEEE Trans Smart Grid. 2017;9(5):4805-14. [Google Scholar]
- Keshavarz A, Wang Y, Boyd S. Imputing a convex objective function. In: 2011 IEEE international symposium on intelligent control. IEEE. 2011:613-9. [Google Scholar]
- Bertsimas D, Gupta V, Paschalidis IC. Inverse optimization: A new perspective on the Black-Litterman model. Oper Res. 2012;60(6):1389-403. [Google Scholar]
- Aswani A, Kaminsky P, Mintz Y, Flowers E, Fukuoka Y. Behavioral modeling in weight loss interventions. Eur J Oper Res. 2019;272(3):1058-72. [Google Scholar]
- Chan TCY, Lee T, Terekhov D. Inverse optimization: Closed-form solutions, geometry, and goodness of fit. Manage Sci. 2019;65(3):1115-35. [Google Scholar]
- Dhiaf MM, Atayah OF, Nasrallah N, Frederico GF. Thirteen years of Operations Management Research (OMR) journal: a bibliometric analysis and future research directions. Oper Manag Res. 2021;14:235-55. [Google Scholar]
- Donthu N, Kumar S, Pandey N, Soni G. A retrospective overview of Asia Pacific Journal of Marketing and Logistics using a bibliometric analysis. Asia Pacific J Mark Logist. 2020 [Google Scholar]
- Demirkol D, KoƧoglu FÅ, AktaƧ $, Ćigdem E. A Bibliometric Analysis of the Relationship between Diabetes and Artificial Intelligence. J Istanbul Fac Med. 2022:9-10. [Google Scholar]
- Perez-Aranda J, Pelaez-Verdet A. An application of social network mining to scientific data: identifying networks structures and detecting partnerships in metrics and citation patterns. Soc Netw Anal Min. 2021;11(1):1-15. [Google Scholar]
- . Machine learning and big data in the impact literature. A bibliometric review with scientific mapping in web of science. Symmetry (Basel). 2020;12(4):495 [Google Scholar]
- Dhamija P, Bag S. Role of artificial intelligence in operations environment: a review and bibliometric analysis. TQM J. 2020 [Google Scholar]
- Bidwe RV, Mishra S, Patil S, Shaw K, Vora DR, Kotecha K, et al. Deep Learning Approaches for Video Compression: A Bibliometric Analysis. Big Data Cogn Comput. 2022;6(2):44 [Google Scholar]
- Ali L, Alnajjar F, Khan W, Serhani MA, Jassmi H. Bibliometric Analysis and Review of Deep Learning-Based Crack Detection Literature Published between 2010 and 2022. Buildings. 2022;12(4):432 [Google Scholar]
- Nakhodchi S, Dehghantanha A. 15 In: Security of Cyber-Physical Systems. 2020:203-21. [Google Scholar]
- Ardito L, Scuotto V, Del Giudice M, Petruzzelli AM. A bibliometric analysis of research on Big Data analytics for business and management. Manag Decis. 2018 [Google Scholar]
- Liu X, Sun R, Wang S, Wu YJ. The research landscape of big data: a bibliometric analysis. Libr Hi Tech. 2020 [Google Scholar]
- Rialti R, Marzi G, Ciappei C, Busso D. Big data and dynamic capabilities: a bibliometric analysis and systematic literature review. Manag Decis. 2019 [Google Scholar]
- Linden R, Barbosa LF, Digiampietri LA. “Brazilian style science”āan analysis of the difference between Brazilian and international Computer Science departments and graduate programs using social networks analysis and bibliometrics. Soc Netw Anal Min. 2017;7(1):1-19. [Google Scholar]
- Guo Y-M, Huang Z-L, Guo J, Li H, Guo X-R, Nkeli MJ, et al. Bibliometric analysis on smart cities research. Sustainability. 2019;11(13):3606 [Google Scholar]
- Raynaud M, Goutaudier V, Louis K, Al-Awadhi S, Dubourg Q, Truchot A, et al. Impact of the COVID-19 pandemic on publication dynamics and non-COVID-19 research production. BMC Med Res Methodol. 2021;21(1):1-10. [Google Scholar]
- Kaipio J, Somersalo E. Statistical and computational inverse problems. 2006;160 [Google Scholar]
- Tarantola A. Inverse problem theory and methods for model parameter estimation. SIAM. 2005 [Google Scholar]
- Gao H, Clasie B, Liu T, Lin Y. Minimum MU optimization (MMO): an inverse optimization approach for the PBS minimum MU constraint. Phys Med Biol. 2019;64(12):125022 [Google Scholar]
- Sheng K, Sawant A, OāConnor D, Nguyen D, Modiri A, Paganetti H, et al. Inverse Optimization Meets High Performance Computing. In: Medical Physics. Wiley 111 River ST, Hoboken 07030-5774, NJ USA. 2017:3094-5. [Google Scholar]
- Lin Y, Clasie B, Liu T, McDonald M, Langen KM, Gao H, et al. Minimum-mu and sparse-energy-layer (mmsel) constrained inverse optimization method for efficiently deliverable pbs plans. Phys Med Biol. 2019;64(20):205001 [Google Scholar]
- Babier A, Mahmood R, Zhang B, Alves VGL, BarragƔn-Montero AM, Beaudry J, et al. OpenKBP-Opt: an international and reproducible evaluation of 76 knowledge-based planning pipelines. Phys Med Biol. 2022;67(18):185012 [Google Scholar]