Contents
ABSTRACT
How do intelligent technology innovation systems develop? How do institutional factors influence the development of innovation systems? This study adopted the National/Sectoral Innovation System as the main framework to study the dynamics of intelligent technology innovation system in Taiwan. Combining scientometric mapping, social network analysis, Multi Criteria Decision-Making (MCDM), and 33 interviews to understand the interactions between key stakeholders and the institutions in the intelligent technology sector in Taiwan. The results show that a relatively large proportions of foreign technologies are widely adopted into the system through international business networks. Regarding commercial applications, firms prefer to introduce foreign technology through international business networks. This illustrates the needs of commercializations of domestic technological research and development. The future policy should promote academia-industry collaborations to enhance the commercializations of scientific research outcomes. This research contributes to enhance the knowledge flow in the innovation system, thus, to upgrade the national capabilities of small emerging economies in East Asia.
INTRODUCTION
In this study, the intelligent technology sector is defined as integrating IoT and AI and creating innovative products and services that can sense, learn, and adapt to the environment and user needs.[1,2] We focus on the case of Taiwan, which has a strong tradition and reputation in the electronics and information technology sector[3,4] but also faces the challenges of industrial upgrading and international competition. We adopt a mixed-methods approach that combines scientometrics, Social Network Analysis (SNA), expert questionnaires, and elite interviews to capture the dynamic evolution and characteristics of Taiwanâs intelligent technology innovation system. The main research question is to explore how the innovation system and competitiveness of the intelligent technology sector evolve.
This primary research question can be broken down into two sub-questions:
How was the intelligent technology innovation system formed and developed?
How do institutional factors influence the development of innovation systems?
To critically answer these questions, this study used the co-authorship data of the Web of Science (WoS), the co-patenting data collected from the Webpat database, and Market Observation Post System (MOPS) business contracts to depict the dynamic evolution of the intelligent technology innovation system during the 2009-2018 and analyze the data to identify the companies that are developing or related to the intelligent technology sector and interviews are conducted from August 2019 to August 2023. We will introduce the development process of analytic framework for analyzing this study in section 2, the application of scientometric analysis and Multiple Criteria Decision-Making (MCDM) in section 3, and analyze the results and discussion in section 4. Finally, section 5 will include the conclusion and future work.
UNDERSTAND THE FORMING OF A NATIONAL INNOVATION SYSTEM FOR INTELLIGENT TECHNOLOGY
The Emergence of Intelligent Technology Innovation System
The rapid development of intelligent technologies, including AI and IoT, has been fueled by the surge in computational power and the onset of the Internet era. This dynamic intelligent technology industry has been a catalyst for transforming emerging technology sectors due to its innovative nature, rapid growth, and sustainability.[5] The increasing computation power and the introduction of the Internet have witnessed a transformative phase with the rise of AI and the commercial applications of big data and cloud technology.[6,7] Propelling intelligent technology into an era of explosive growth can create new industries and disrupt existing ones, leading to profound changes in social structures.[5] Technological advancements significantly influence the competitiveness of research and the development of industries.[8,9] With globalization intensifying industrial competition, innovation is becoming more diverse and complex, fostering close collaboration in technology and product development with various strategic partners. The technological convergence associated with industry transformation provides diverse solutions for addressing complex social and technical challenges.[10,11] It blurs existing industry boundaries, opens new niche markets, and generates numerous competitive opportunities.[12] However, empirical studies on the innovation systems of intelligent technology are limited. As emerging industries rapidly evolve, the intricate relationship between science, technology, and business becomes increasingly vital. In this context, the establishment of robust innovation systems becomes imperative.
The Dynamics of Innovation Systems
In the landscape of the knowledge economy, innovation stands as the linchpin of competitiveness, is considered as a pivotal tool for wealth creation.[13] Understanding the dynamics of innovation systems is crucial for comprehending the evolution and interaction of knowledge across organizations, thereby driving competitiveness in the knowledge economy.[14] These innovation systems operate at various levels, including the National Innovation System (NIS),[15,16] Regional Innovation System (RIS),[17] Sectoral Innovation System (SIS)[18] and Technological Innovation System (TIS).[19] NIS focuses on how government policies shape a countryâs innovation landscape. The proposed concept framework of the National and Sectoral Innovation System, as illustrated in Figure 1, This would be helpful for underscore the knowledge flow among internal participants in the innovation system, especially knowledge transfer among companies and their partners.[20–22]
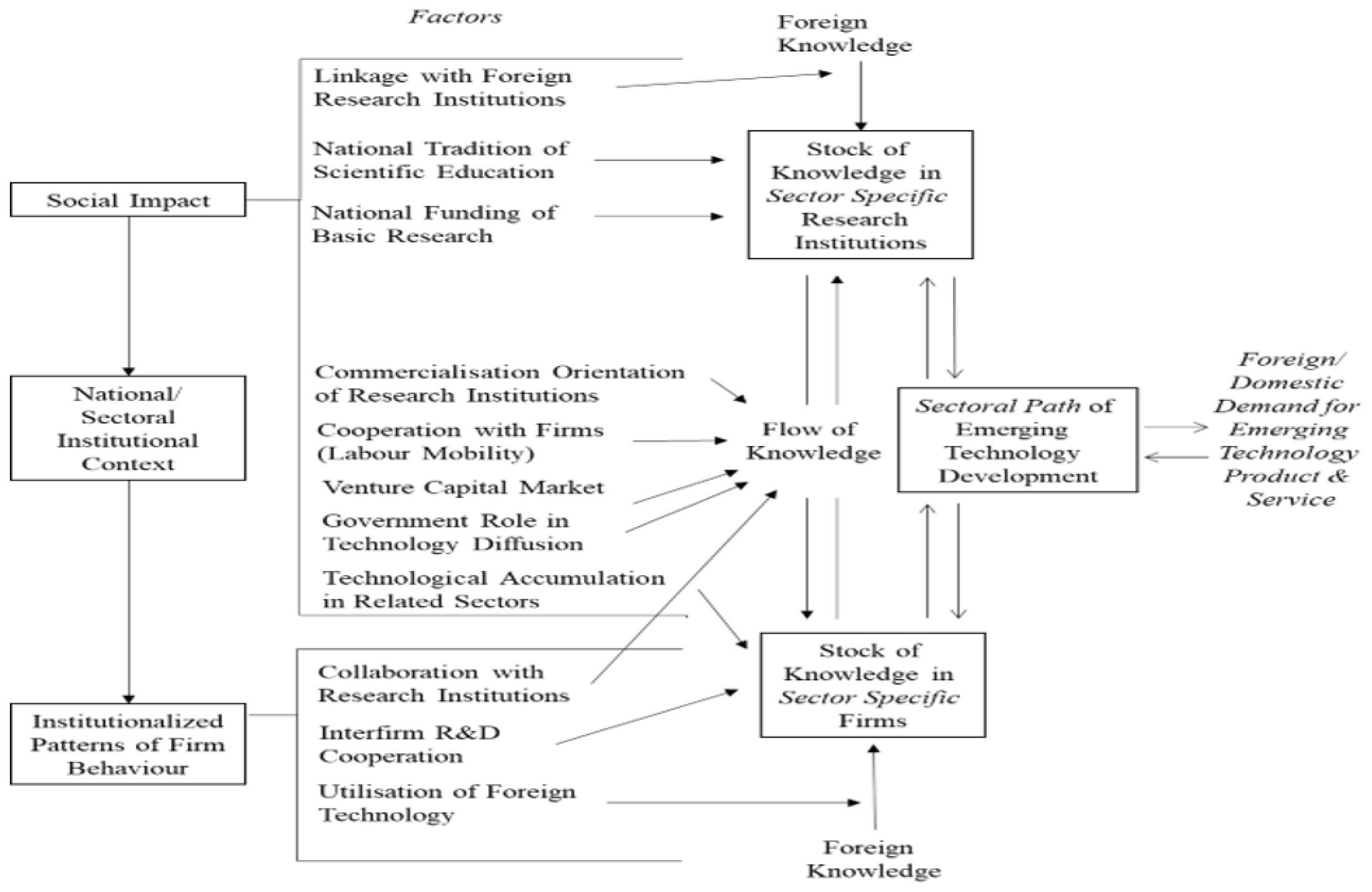
Figure 1:
The National Biotechnology Innovation System (NBIS) Framework (Adopted Chen et al., 2012).
The above-mentioned conceptual framework was first proposed to analyze the development of a national biotechnology innovation system (NBIS)[21–23]. However, the applications of these frameworks on other emerging national/sectoral systems of innovation are still rare. This paper is one of the very few literatures adopting national/sector innovation system amended based on the NBIS framework. Taiwan has a strong tradition and reputation in the electronics and information technology industry.[7,8] Has played a foundational role in emerging technologies such as intelligent technology. However, the literature on innovation systems in this sector still needs to be expanded, particularly concerning the transition from scientific development to commercial application. Addressing these gaps is essential for fostering collaboration between academia and industry as governments formulate policies to support innovation worldwide. This study will use the framework to analyze the flow of knowledge among participants within the innovation system of the intelligent technology sector and explore the critical factors in establishing an innovation system.
Scientometric Mapping and Social Network Analysis in Innovation System Studies
In this study, scientometric mapping and Social Network Analysis (SNA) are adopted to understand the emergence of intelligent technology. These insights strengthen strategic thinking and boost creativity, thus contributing to scienceâs progress.[23] One of the essential milestones in scientometric mapping was the proposal of science overlay maps, which introduced an interactive mapping technique called overlay mapping that can be valuable for understanding and managing technologies.[24] This overlay can represent the contributions of individuals, organizations, communities, or new fields in science and technology.[24,25] These techniques can be used to track how emerging technologies develop in different geographical, social, and cognitive spaces. Due to its nature, scientometric mapping is often used in understanding the dynamics of innovation systems and the emergence of new technology.[9] The innovation systems approach suggests that innovation occurs not only when individual businesses conduct Research and Development (R&D). Instead, it emphasizes that various entities contribute to innovation, each with its own goals. The idea is that when companies collaborate with research centers, it helps spread new ideas and provides a country or economic system with a more competitive edge. Unlike the traditional focus on a single company investing in R&D, this approach looks at multiple actors and networks working together in the innovation process. Recent developments in Network Analysis have made it possible to see these connections as networks that function like knowledge channels.[26]
Applying MCDM to System of Innovation Studies
In industrial development frameworks, addressing the complexities inherent in Multi Criteria Decision-Making (MCDM) requires an objective approach considering the relative weights of assessment criteria.[27] The Analytic Hierarchy Process (AHP) has emerged as a widely used method for determining these weights.[28] This structured approach provides a systematic framework for evaluating and prioritizing diverse criteria crucial for navigating the complexities of industrial development. However, when conducting pairwise comparisons in the analytic hierarchy process, if the number of criteria exceeds three, the general public can often not make consistent pairwise judgments.[29] In this study, the process will be further optimized in section 3. Given the distinct technological specialization patterns observed across different industries,[30] this study explores the formation of intelligent innovation systems. Leveraging the NBIS conceptual framework, this research extends the framework to propose the national system of intelligent technology innovation framework. Subsequently, the AHP methodology is employed to investigate and establish the weighted rankings of all key factors within this framework. This comprehensive exploration aims to illuminate the intricacies of intelligent technology innovation systems.
The Analytic Framework
The intelligent technology sector is rapidly emerging with the rise of AI and IoT. The convergence of various industries has led to an emerging sector. This study discover the paths of scientific, technological, and business development in innovation systems. However, empirical studies on the knowledge flow and actor networks within these emerging industry innovation systems are relatively scarce. Therefore, based on a modified framework from the NBIS, this study focuses on intelligent technology and analyzes the relationships between the actor-network, national/sectoral institutional context, and knowledge base. The COVID-19 pandemic has accelerated digital transformation in industries and the development of intelligent technology, prompting governments worldwide to implement corresponding policies and measures. Hence, the study incorporates the impact of the pandemic and government policies into the research factors, as illustrated in Figure 2.
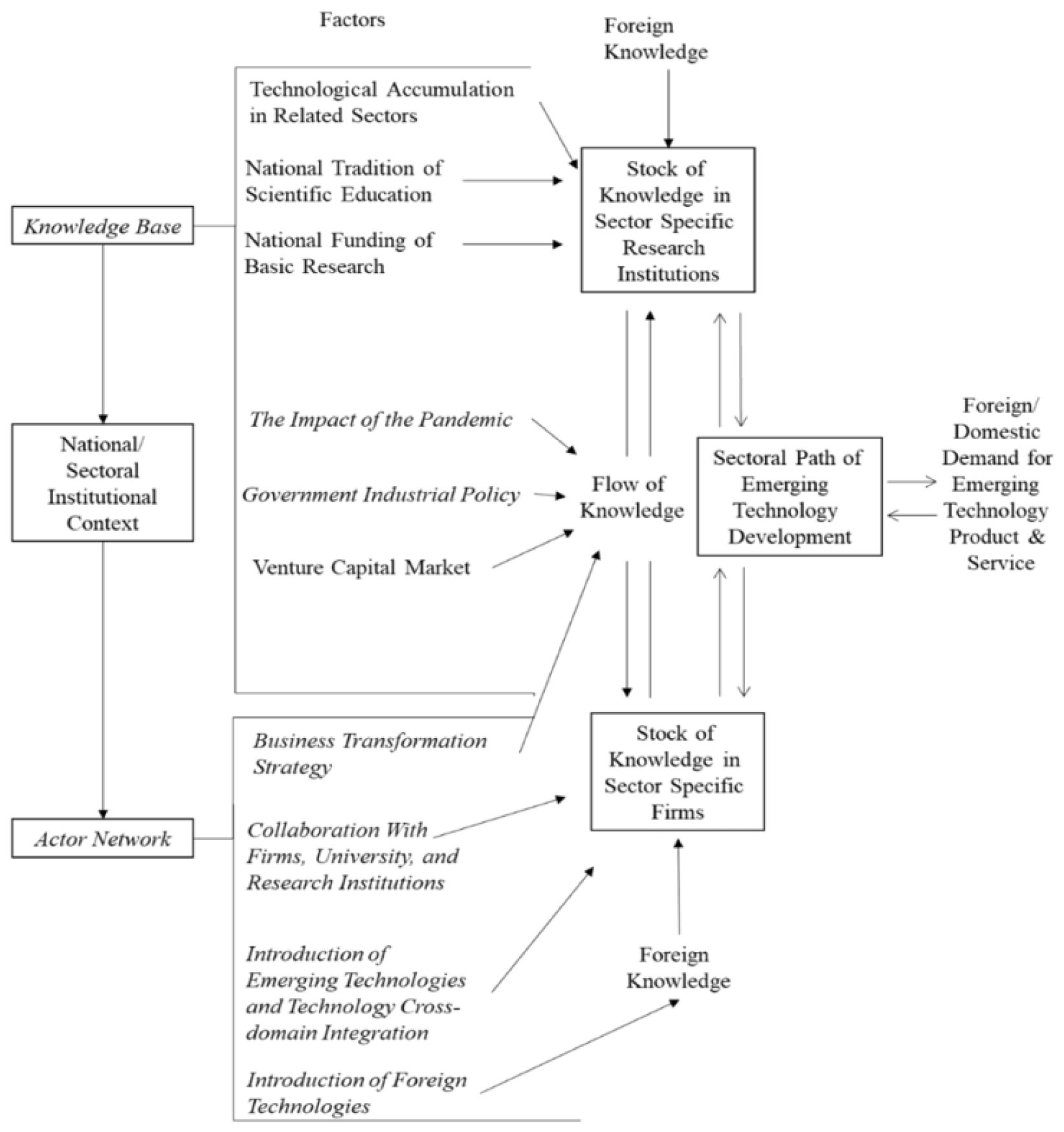
Figure 2:
The National Intelligent Technology Innovation System Framework (Adapted Chen et al., 2012).
Research Framework
Knowledge base encompasses technical accumulation in relevant industries, national science education, and funding for basic research. For example, indicators of technical accumulation could include the number of patents, scientific publications, and the volume of funding allocated to basic research.
The national/sectoral institutional context considers the influence of risk investment markets and government policies. The study acknowledges the pandemicâs substantial impact on market operations and industrial policies. In subsequent sections, specific indicators and criteria for measuring risk investment markets and government policies will be detailed.
The actor-network explores enterprise transformation strategies, emphasizing the accumulation of knowledge between firms and research institutions. The study will provide concrete examples and measurements beyond mere dissemination among firms. Critical factors such as cross-domain integration of emerging technologies and introducing foreign technologies will be explicitly defined. Ultimately, this study uses MCDM expert questionnaires and evaluates the importance of all factors of this framework.
DATA AND METHODS
This study employs a mixed-methods approach, utilizing various methods to analyze the innovation networks of Intelligent Technology in Taiwan. Scientometric mapping and SNA are used to understand the emergence of intelligent technology. Additionally, MCDM with AHP is utilized to explore the relationships among factors influencing knowledge storage systematically, flows, and the knowledge landscape of firms within specific sectors. Elite interviews complement the quantitative data, providing a holistic view of the innovation network of intelligent technology.
Data Collection
This study examines the trend of Intelligent Technology development in Taiwan over the past decades. It explores the formation of its innovation network through Webpat global patent database, WoS scientific papers, and in-person interviews with top management level companies to illustrate the similarities and differences in the dynamic evolution of Intelligent Technology development in Taiwan.
Scientometric Mapping
Scientometric mapping involves the analysis of scientific publications and patent data to understand the emergence and evolution of Intelligent Technology. Scientific publication data were retrieved from the WoS, while patent data were obtained from Webpat and business contracts for Market Observation Post System (MOPS). The primary challenge in scientometric mapping lies in identifying relevant data. Given the complex and evolving nature of emerging technologies like Intelligent Technology, selecting appropriate keywords becomes crucial.[31] A combined keyword search approach, commonly practiced in similar studies, was adopted to address this challenge.[32,33] After conducting an extensive literature review, a set of 12 keywords was selected to capture various aspects of Intelligent Technology, including â(Depthâ OR âDeepâ) learning,â âNatural language processing,â and âMachine learning.â Additionally, 147 Internet of Things (IoT) keywords were identified and categorized into perception, transmission, and application layers. These keywords were validated by experts with over 25 years of experience in intelligent manufacturing. 2009 was an important year in the history of science and technology, with the issuance of 3G licenses in China.[34] The same year, the iPhone officially applied a 3G network and GPS to smartphones.[35] This affected the vigorous development of the IoT industry. Google developed AlphaGo Zero, which became the worldâs most potent Go program at the time, opening the door to the era of artificial intelligence applications; in 2014, the Taiwanese government promoted the âAsian Silicon Valleyâ plan Drawing on the two main axes of âdriving economic growth through innovation and entrepreneurshipâ and âpromoting industrial transformation and upgrading through the Internet of Things,â Taiwan has become an innovation base for AIoT (Artificial Intelligence and the Internet of Things). Promote many manufacturing industries in Taiwan to introduce the Internet of Things for intelligent manufacturing.[36] At the same time, the rise of cloud computing and big data has attracted attention.[6,7] Therefore, to ensure a comprehensive analysis, data were collected from 2009 to 2018 and segmented into two five-year periods: 2009-2013 and 2014-2018. This timeframe allows for an in-depth examination of trends and patterns in the evolution of Intelligent Technology within Taiwanâs innovation landscape.
Social Network Analysis
The section describes the methodology and data collection process for conducting SNA to understand the innovation networks within the intelligent technology sector in Taiwan and explore R&D collaboration relationships between institutions. Thus, providing insights into the interactions within the industryâs innovation system. The data primarily came from WoS for academic data, Webpat for patent data, and MOPS for business data. Ucinet and Vosviewer software packages were utilized to visualize the relationships between R&D institutions and joint patents. Similarly, patent data from Webpat were sorted using Excel and visualized through Ucinet to obtain a network diagram of joint patents. Significant players in the industry were highlighted by identifying institutions with high connectivity in intelligent technology. Additionally, the principal IPC patent codes were used to identify areas for development in intelligent technology. The study then identified the leading actors involved in various R&D processes in intelligent technology, including universities, research institutions, and firms. The elite interviews with these top actors were then conducted in this study.
Multiple Criteria Decision-Making (MCDM)
In this section, we present the design, validation, and analysis of the MCDM questionnaire and the verification of the results. Designing MCDM questionnaires using AHP involves systematically exploring factors influencing knowledge dynamics in intelligent technology sectors. The questionnaire delves into the dynamic interplay of factors within the intelligent technology landscape. Operating within the Technology Innovation System framework, the questionnaire considers three primary dimensions and their criteria. The Knowledge Base dimension focuses on the convergence of intelligent technology, encompassing factors such as Technological Accumulation and National Scientific Education. The National/Sectoral Institutional Context dimension considers factors like The Impact of the Pandemic and Government Industrial Policy. The Actor-Network dimension recognizes knowledge accumulation between firms and research institutions, including factors like Business Transformation Strategy and Introduction of Emerging Technologies. An AHP analysis framework, based on these criteria and dimensions, guides questionnaire creation. When selecting indicators in MCDM, too many indicators will often affect the consistency of the subjectsâ judgments.[29] This study used personal discussions with the subjects to complete the questionnaires to ensure that the subjectsâ logic was consistent. According to the principle, the Consistency Index (C.I.) must be less than or equal to 0.1.[28] If the validation exceeds 0.1, the questionnaire will be considered invalid. Therefore, using C.I. as an index to measure matrix consistency, this study collected 30 questionnaires, of which 22 are below the consistency index requirement. The questionnaire administration targeted professionals from companies, research institutions, and universities with over ten years of experience in the intelligent technology sector. Finally, questionnaire results will be augmented through interviews, ensuring the robustness and reliability of the data collected.
Interview and Documentary Collection
This study adopts a mixed-methods approach, combining quantitative and qualitative methodologies to explore the intricate dynamics of emerging technology innovation networks. Following the quantitative data collection, a thorough search identifies critical firms and organizations driving advancements in intelligent technology industries. The investigative phase involves elite interviews conducted from August 2019 to August 2023. Thirty-three firms and organizations were selected for in-depth interviews, with transcripts transcribed for subsequent analysis. These transcripts are systematically coded, facilitating the comprehensive exploration of qualitative data.
Combining quantitative data and qualitative interview, this study aim to understand the development of intelligent technology sector. Qualitative findings disclose of the industryâs structure and developmental trajectory. This mix method approach integrated quantitative and qualitative methods, aims to provide a comprehensive portrayal of the intelligent technology sector. By validating quantitative results and capturing contextual insights from industry stakeholders, this study contributes to a holistic understanding of the industryâs innovation landscape, enriching insights into its evolution and prospects.
Data Analysis
Analytical techniques such as scientometric mapping, social network analysis, and thematic analysis of interview transcripts examine patterns, trends, and relationships within the data. This analysis aims to explore the form and develop an intelligent technology innovation system. Comparisons are drawn to illustrate the similarities and differences in the evolution of intelligent technology development in Taiwan over time. The results of this analysis contribute to a deeper understanding of the dynamics shaping the intelligent sector industry and inform strategic decision-making for future developments in this field.
Scientometric Mapping
In this study, scientometric mapping facilitate visualizing scientometric indicators. VOSviewer is a free accessible software designed to create and visualize bibliometric maps tailored for constructing large bibliometric maps that are easily interpretable.[37] Its functionality is geared towards analyzing bibliometrics comprehensively, making it particularly beneficial for presenting extensive bibliometric maps. VOSviewer further improves the graphical presentation of these maps by incorporating features such as zooming capability, specialized labeling algorithms, and density calculations. âBubble mapsâ and âHeat mapsâ are commonly used for scientometric mapping.[38] In a bubble map, the size of each bubble is interpreted as a scientometric indicator, such as the total number of citations of the corresponding articles, and the links between each bubble define a different indicator, such as the articleâs co-occurrences.[39] The visualization of bubble position is set to the centrality and density.[40] The heat map is a similar visualization method showing density or other attributes by different colors.
Social Network Analysis
This study analyzes and organizes to understand actorsâ collaboration relationships. Visual representations of the collaborations were created using Ucinet, and different colors were assigned to indicate the collaboration actors. This study uses the bibliometrics and patent data to conduct social network analysis on the co-author network, institution network, and community to investigate the interaction and network relationships among different levels. Finally, network analysis is performed on the co-patentees. We use WoS to collect intelligent technology-related bibliometrics collaboration relationships among authors, affiliated institutions, and organizations. Webpat is used for patent retrieval, the inventor is selected for co-inventor analysis, and the patentee is chosen for co-patenteesâ analysis. We collected the financial annual report from MOPS database.We consolidated this data into Microsoft EXCEL and exported it in the desired file format. This is followed by import to the network analysis tool Ucinet to explore the network relationship for visual analysis.
Multiple Criteria Decision-Making (MCDM)
In this phase of the study, quantitative and qualitative data analysis methods will be employed to derive meaningful insights from the collected data. Once the expert survey questionnaire is complete, it will undergo a dual-layered review. Initially, the quantitative analysis will thoroughly categorize and summarize factors based on an extensive review of relevant literature. This process will be foundational in understanding the factors shaping intelligent technologyâs landscape.
The first step involves constructing pairwise comparison matrices based on the results from expert-filled questionnaires, comparing the options within the scoring range. The comparison matrix describes the differences between these options, and the scoring differences take the following form:
fij=fi(oij) for j=1,2,3,4,5, the comparison matrix takes the following form:
This AHP program is used because decision-makers need to select options with specific score differences assigned to consecutive possibilities, forming a score vector. After constructing the pairwise comparison matrix, the eigenvalue and eigenvector are calculated using eigenvector theory to determine the relative weights between factors. An approximation method can be used to find the maximum eigenvalue λmax and the dominant eigenvector Generally, the row vector average normalization method yields optimal results, and its equation is as follows:
The maximum eigenvalue Îmax is then obtained using the formula:
Finally, it is crucial to validate consistency, examining the pairwise comparison matrices constructed by respondents to determine their rationality and whether there is logical consistency before and after. The C.I. must be less than or equal to 0.1 for the questionnaire to be considered adequate. If it exceeds 0.1, it indicates issues with the inter-factor relationships within the hierarchy, necessitating a reanalysis of all questions related to factor relationships.[28]
Thematic Analysis
This study also adopted a thematic analysis approach to explore in depth the wealth of data collected from the individual interviews.
We identified important themes and trends in the intelligent technology sector by systematically coding the interview texts. The thematic analysis aimed to reveal interview participantsâ perceptions, opinions, and experiences about the intelligent technology sector. The collected interviews were classified in detail, with âRâ representing research institutions, âFâ representing firms, and âUâ representing universities. By recognizing themes and patterns, we can gain insights into the sector dynamic changes and the concerns and challenges of relevant stakeholders.[41] In addition, thematic analysis helps to identify possible new trends and potential development directions,[42] providing valuable reference for the future development of the intelligent technology sector. By combining the results of the thematic analysis with findings from quantitative data and other qualitative research methods, we can gain a more comprehensive understanding of the innovation system of the intelligent technology sector and provide powerful insights to guide future research and practice.
DYNAMIC OF THE INTELLIGENT TECHNOLOGY INNOVATION SYSTEM
In this section, we delve into the formation and evolution of the intelligent technology innovation system, focusing on the relationships among stakeholders and actors within the innovation system.
Actor Network
Actor networks facilitate knowledge exchange, collaboration, and resource sharing among diverse stakeholders, playing a crucial role in driving innovation, fostering technology transfer, and accelerating the development of intelligent technology. This section analyzes the dynamics, challenges, and opportunities within the intelligent technology sector across the scientific, technological, and commercial dimensions to explore the innovation process and outcomes comprehensively.
We collected and organized the co-authors of relevant research papers from WoS to investigate the scientific development in intelligent technology. Utilizing VOSviewer for visual analysis, we examined the participation landscape in scientific research as illustrated in Figures 3 and 4.
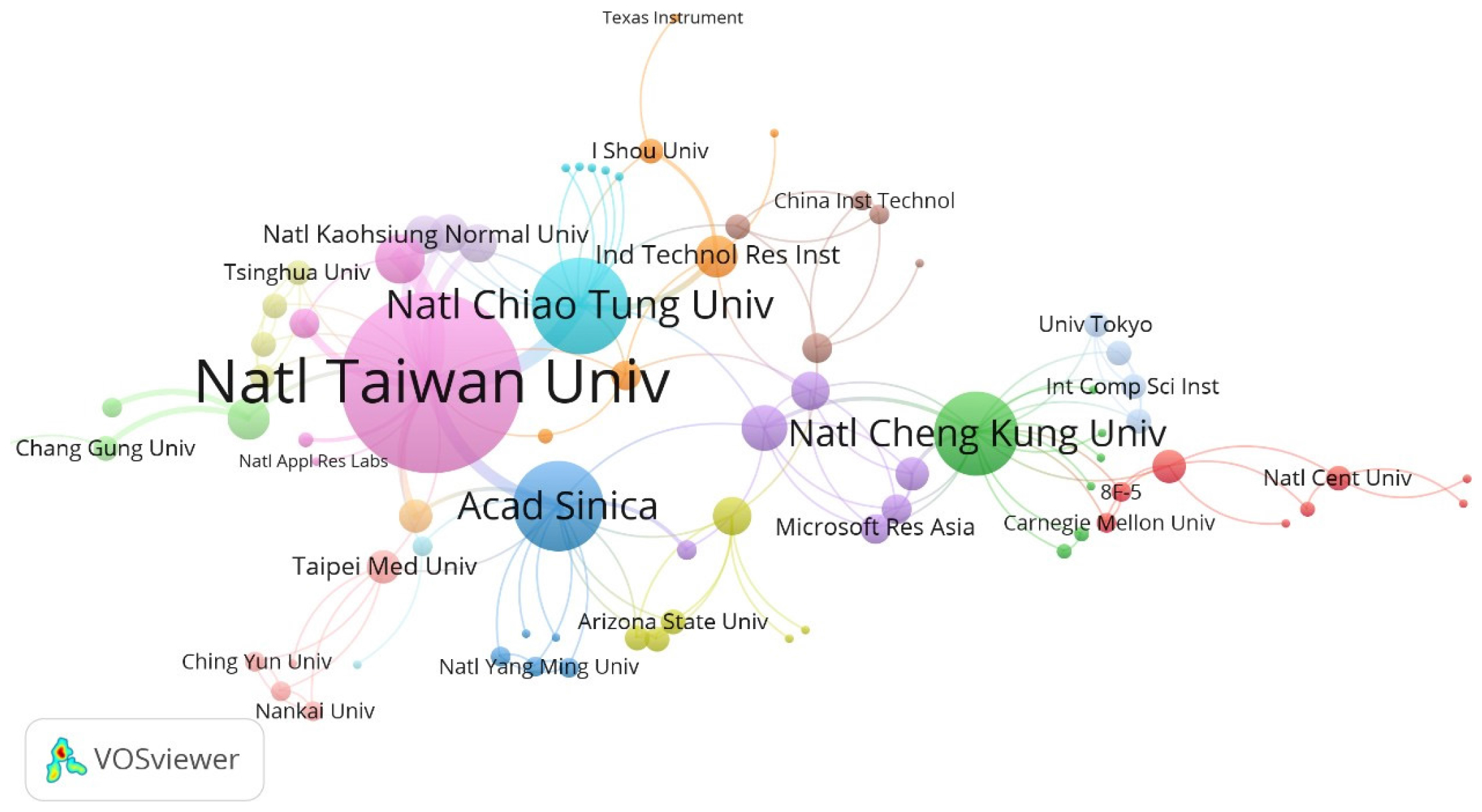
Figure 3:
Co-authorship Network 2009-2013.
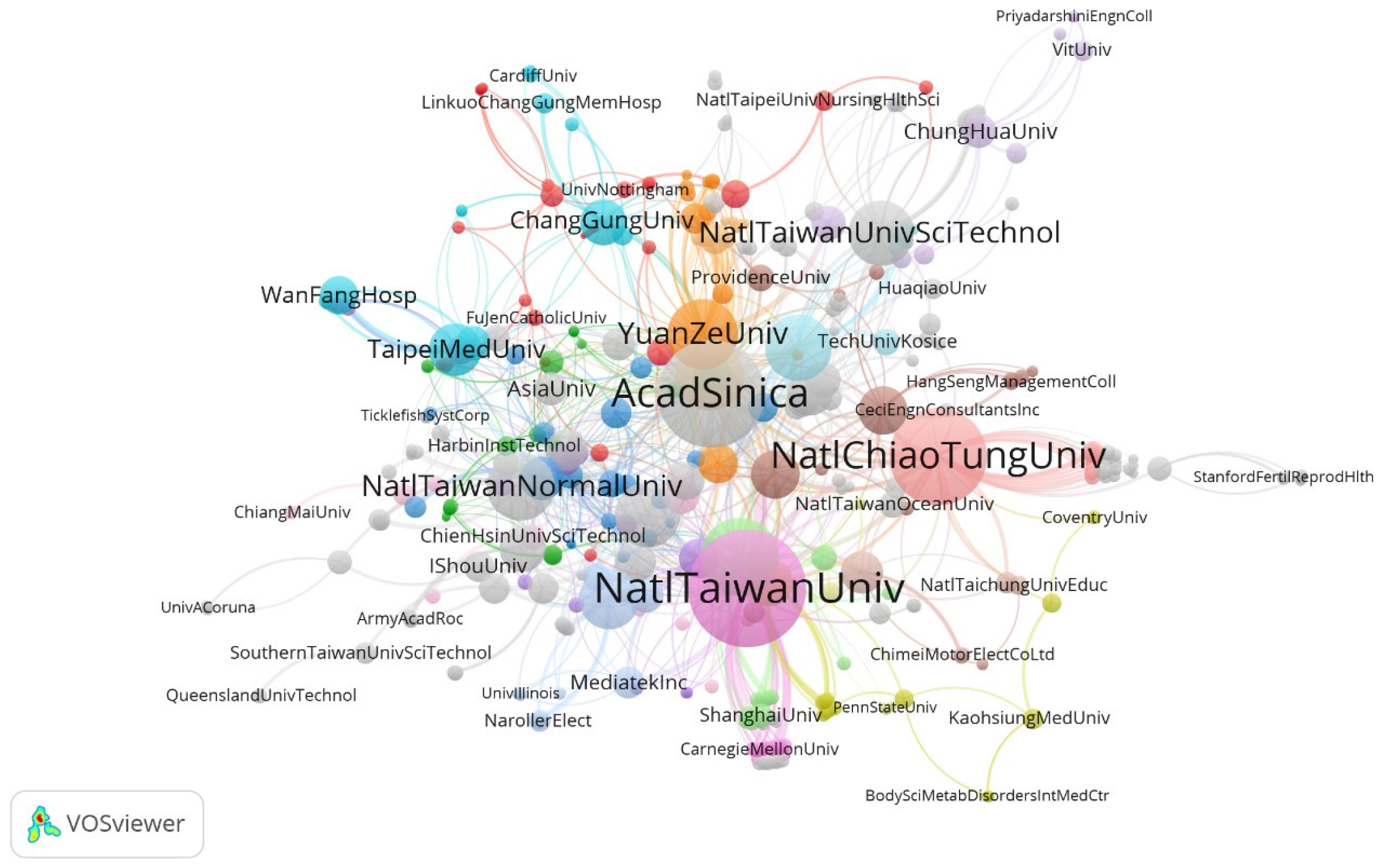
Figure 4:
Co-authorship Network 2014-2018.
Figure 3 reveals that during the early stages of scientific development in the intelligent technology domain, National Taiwan University (NTU) dominates, with a significantly higher number of co- authors than other participants. Besides academic institutions, Academia Sinica is also an essential research institute. Over time, as depicted in Figure 4, scientific research in the intelligent technology domain shows a steady increase. However, despite many participants, firms remain largely absent from scientific research, with collaborations primarily confined to academic institutions. This observation aligns with remarks from interviews:
âOur university typically conducts research in laboratories during the initial stages. We only consider engaging with companies after patent applications are completedâ [(Interview, U2)]
â… with university collaborations focus on proposing projects to the Ministry of Economic Affairs, involving basic research, simulations, or stages where professors have developed. We prioritize collaborations with well-established schools and professors.â [(Interview, R5)]
âCompanies tend to outsource low-cost equipment manufacturing to other firms, representing one scenario of strategic partnershipsâ [(Interview, F4)]
Elite interview results highlight a lesser demand for engagement with firms during the research phase, with interactions typically initiated post-patent application, often requiring considerable preparation time, thereby reducing collaboration opportunities. Research institutions prefer expedited collaboration with established university professors already engaged in research, facilitating project proposal drafting. Firms also prefer strategic alliances with relevant sector players to bolster competitive advantages.
In exploring technological advancements in innovation technology, this study collected relevant patents and co-patents from the Webpat global patent database, organizing them for analysis. The study utilized Ucinet for network visualization to understand the technological research and development participants, as illustrated in Figures 5 and 6.
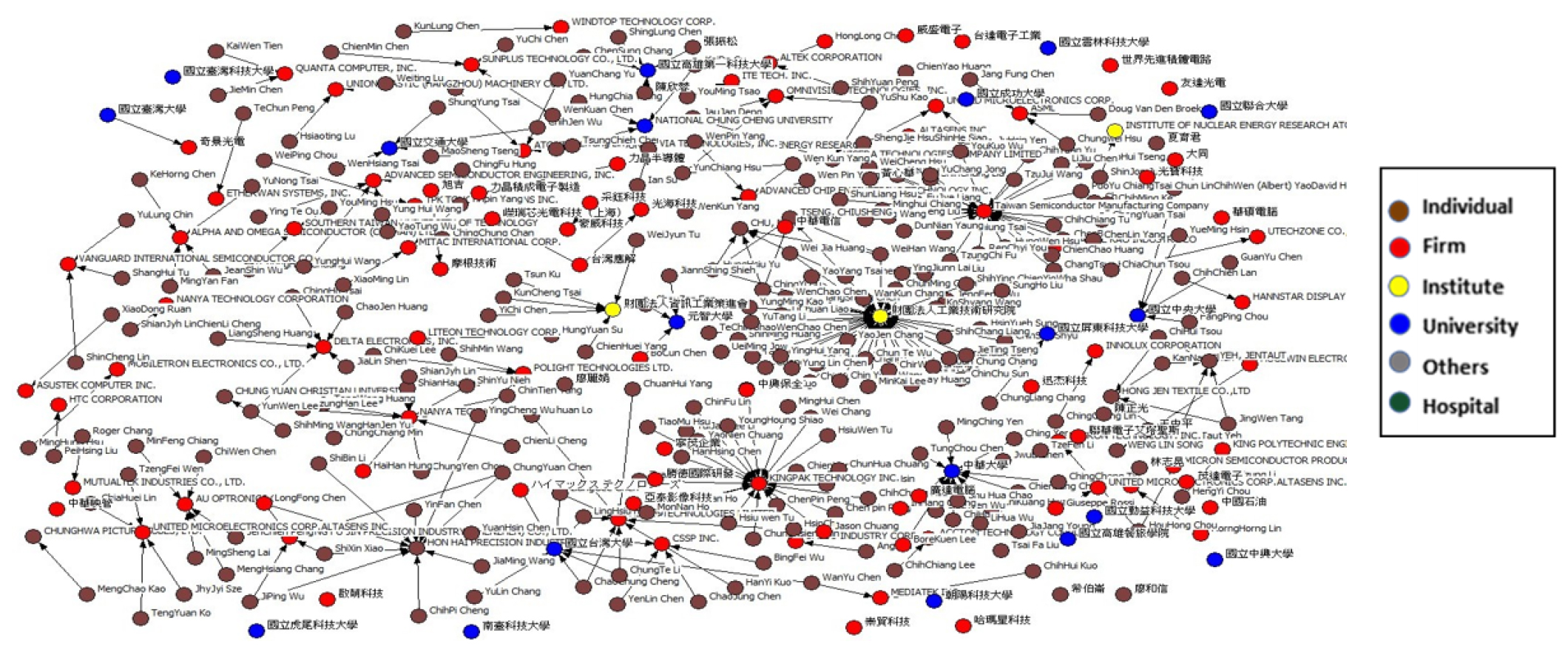
Figure 5:
Co-patenting Network 2009-2013.
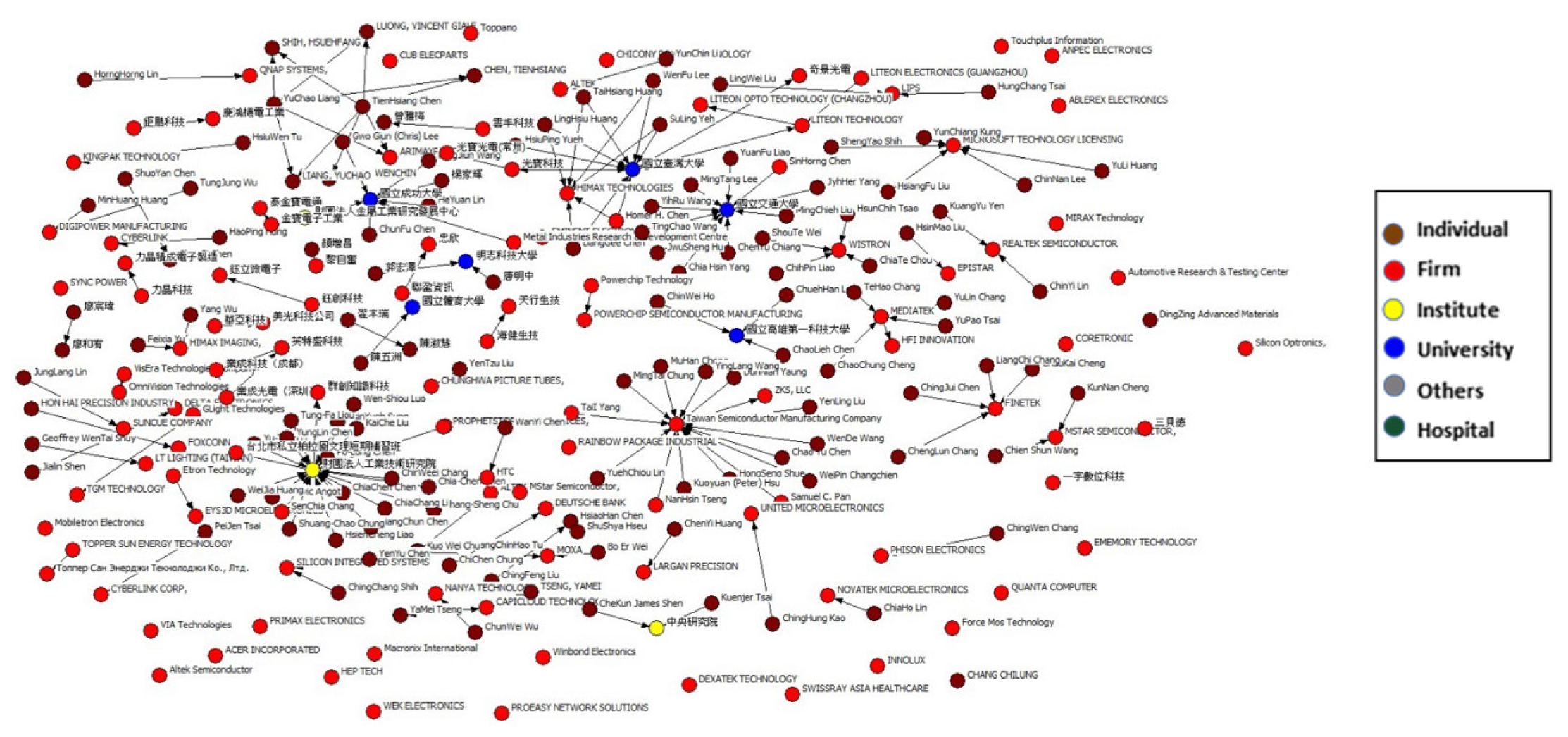
Figure 6:
Co-patenting Network 2014-2018.
Figure 5 reveals that in the patent network, academic representatives include NTU and Chung Hua University (CHU). At the same time, research institutions are represented by the Industrial Technology Research Institute (ITRI) and the Institute for Information sector (III). Firms such as TSMC and Kingpak also feature prominently. This illustrates the active engagement of these stakeholders in patent acquisition within the intelligent technology domain. Moving to later stages, as shown in Figure 6, individual patent applicants decrease while firms increase significantly. This underscores the pivotal role of firms in advancing intelligent technology. However, the overall number of patent R&D is considerably smaller. Despite the expectation that research institutions act as sector intermediaries,[43,44] an intriguing observation emerges in this study: NTU, a university, and TSMC, an enterprise, function as intermediaries, with NTU surpassing ITRI in this capacity. Regarding this phenomenon, interviews provided possible explanations:
âGenerally, companies acquire our technology but do not necessarily obtain ownership. Intellectual property rights may still reside with ITRI unless specified otherwise in contracts.â [(Interview, R1)].
âWe sometimes participate in exhibitions to promote our technology, and at such times, some companies find that our technology aligns well with their companyâs development direction or transformation plans.â [(Interview, U5)].
âWe do not commercialize technology; instead, we hand it over to manufacturersâ [(Interview, U3)].
âThrough associations like the Automation Association, ITRI, or III, we explore suitable partners by understanding their needs and expanding business developmentâ [(Interview, F3)].
Interviews with ITRI revealed that not licensing patents does not imply a lack of intermediary roles. Contractual issues may prevent patent transfers, yet ITRI continues to assist the industry in technological advancement by providing expertise and technical services. ITRI still plays the role of an intermediary. It is impossible to understand this reason only by observing patent data, which illustrates the necessity of elite interviews. Academic institutions also promote their technological research results by participating in exhibitions or competitions to attract potential partners for collaboration and tend to license their technology to firms for commercialization. Interviews with firms revealed that the intermediary effect of research institutions allows manufacturers to develop more needs and expand business through exchanges organized by these institutions, seeking potential partners for collaboration.
In intelligent technology business development, an investigation was conducted into collaborative data among relevant industry companies. Utilizing Ucinet for visualization, institutions were differentiated by shape and companies by color, as depicted in the legend, to understand the dynamics of business collaboration, as shown in Figures 7 and 8.
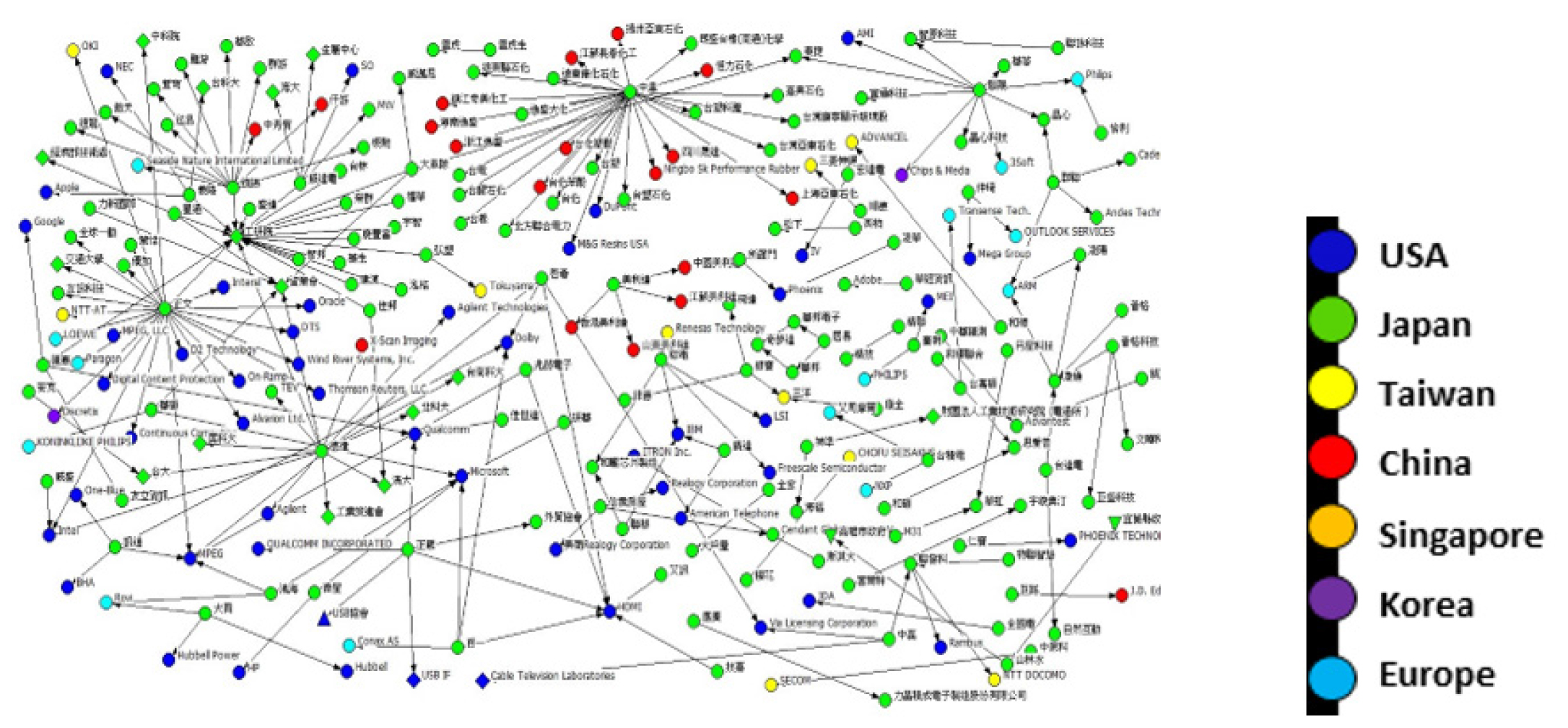
Figure 7:
2009-2013 Business Collaboration Network.
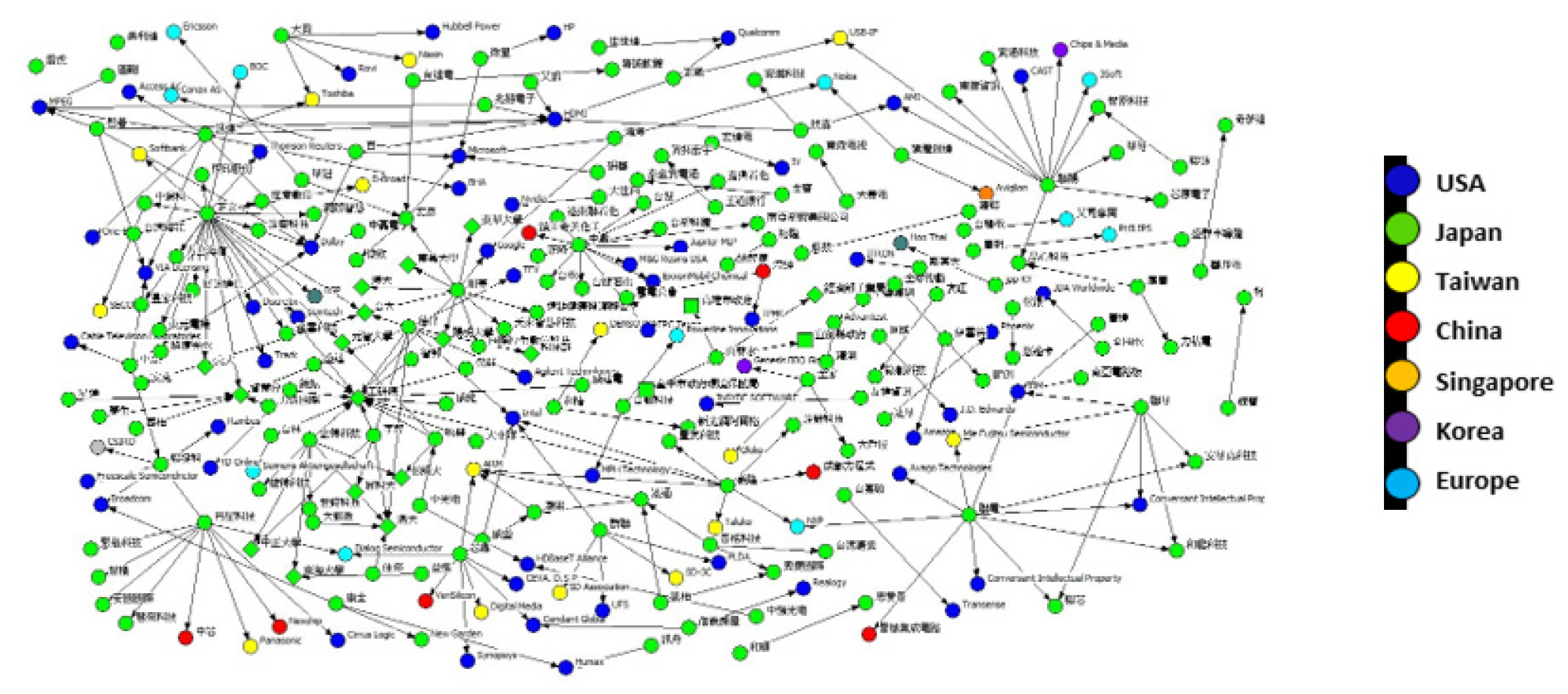
Figure 8:
2014-2018 Business Collaboration Network.
Figure 7 indicates that the intelligent technology industry is highly internationalized, with close collaborations with foreign companies. Utilizing non-profit organizations such as HDMI, MPEG, and USB technology standards is crucial for industry development. These standards facilitate the integration of various technologies, highlighting the importance of collaborative relationships. Regarding business development, research institutions like ITRI and III play pivotal roles, with many firms requiring close collaboration. Academic institutions, notably National Tsing Hua University (NTHU), followed by NTU, have extensive industry-academia collaboration.
Firms predominantly engage with Microsoft, IBM, and Dolby, all American companies, underscoring the United Statesâ leadership position in the intelligent technology industry. Additionally, Figures 7 and 8 show a shift from close collaboration with Chinese mainland firms in the early stages to broader collaboration with companies from various countries later. Elite interviews were conducted to delve into business development:
âWe primarily focus on software development in collaboration with companies, leaving hardware integration to them, preferably with listed companiesâ [(Interview, U1)].
âUniversities are relatively fewer; we usually work directly with manufacturersâ [(Interview, R1)].
âPrimarily, when companies have demands, they approach us for research and development projects, which we then undertake, followed by technology transferâ [(Interview, R3)].
âRegarding Taiwanâs industry, the market is relatively small, whether due to population size, domestic demand, or market orientation toward Europe, America, or Chinaâ [(Interview, F3)].
âWith large foreign companies as strategic partners, it enhances our visibility and may provide opportunities for joint customer acquisition.â [(Interview, F18)]
According to the interview, it is observable that collaborations between universities and companies tend to favor listed companies due to their abundant resources, including funding, equipment, and talent. Research institutions reveal less collaboration with universities and closer collaboration with manufacturers. Network analysis also needs more data on collaborations between research institutions and universities, typically between firms and universities or research institutions. Due to Taiwanâs small domestic market, the intelligent technology industry must enter the global market. One of the reasons for promoting collaboration with large foreign companies is to increase company visibility and seek more customer opportunities.
The Development of Knowledge Base
Intelligent technology is a complex and interdisciplinary industry where the foundation of knowledge plays a crucial role. The correlation between science and technology influences the interaction between technological innovation and academic progress. To explore the linkage between science and technology in the innovation system of intelligent technology, this study conducts domain analysis on collected papers and patent data. The analysis visualization is presented using VOSviewer, as shown in Figures 9–12.
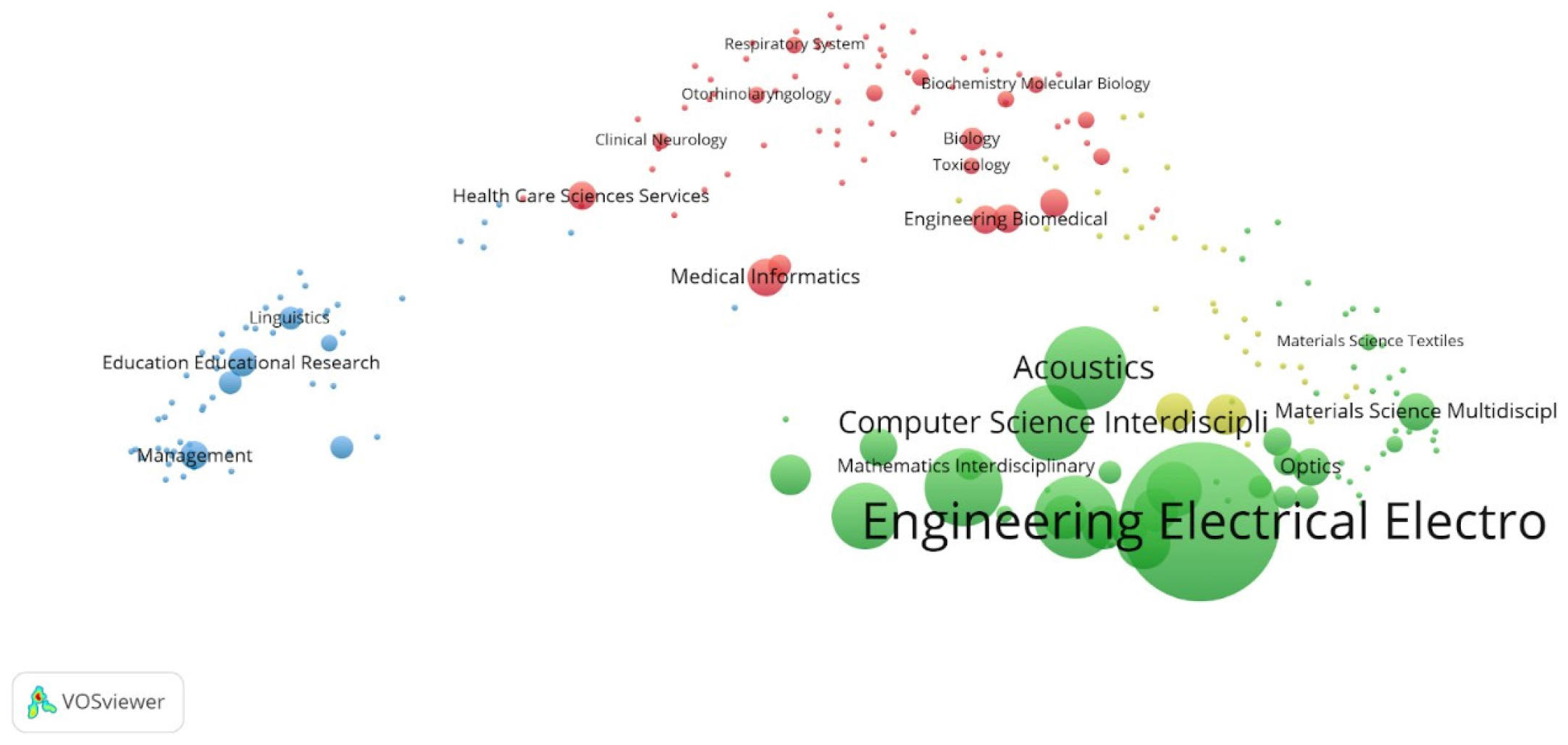
Figure 9:
2009-2013 Intelligent Technology Research Classification.
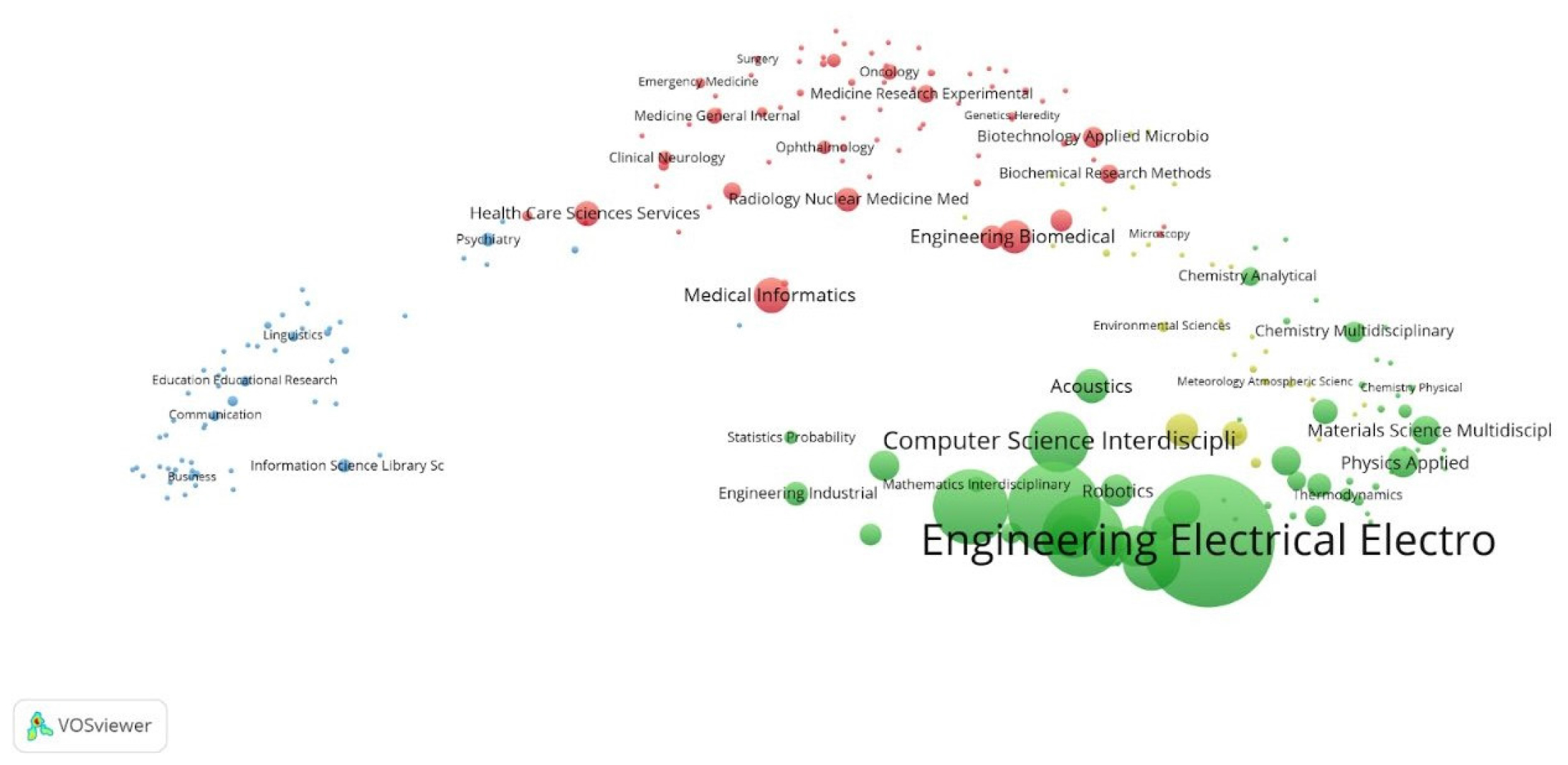
Figure 10:
2014-2018 Intelligent Technology Research Classification.
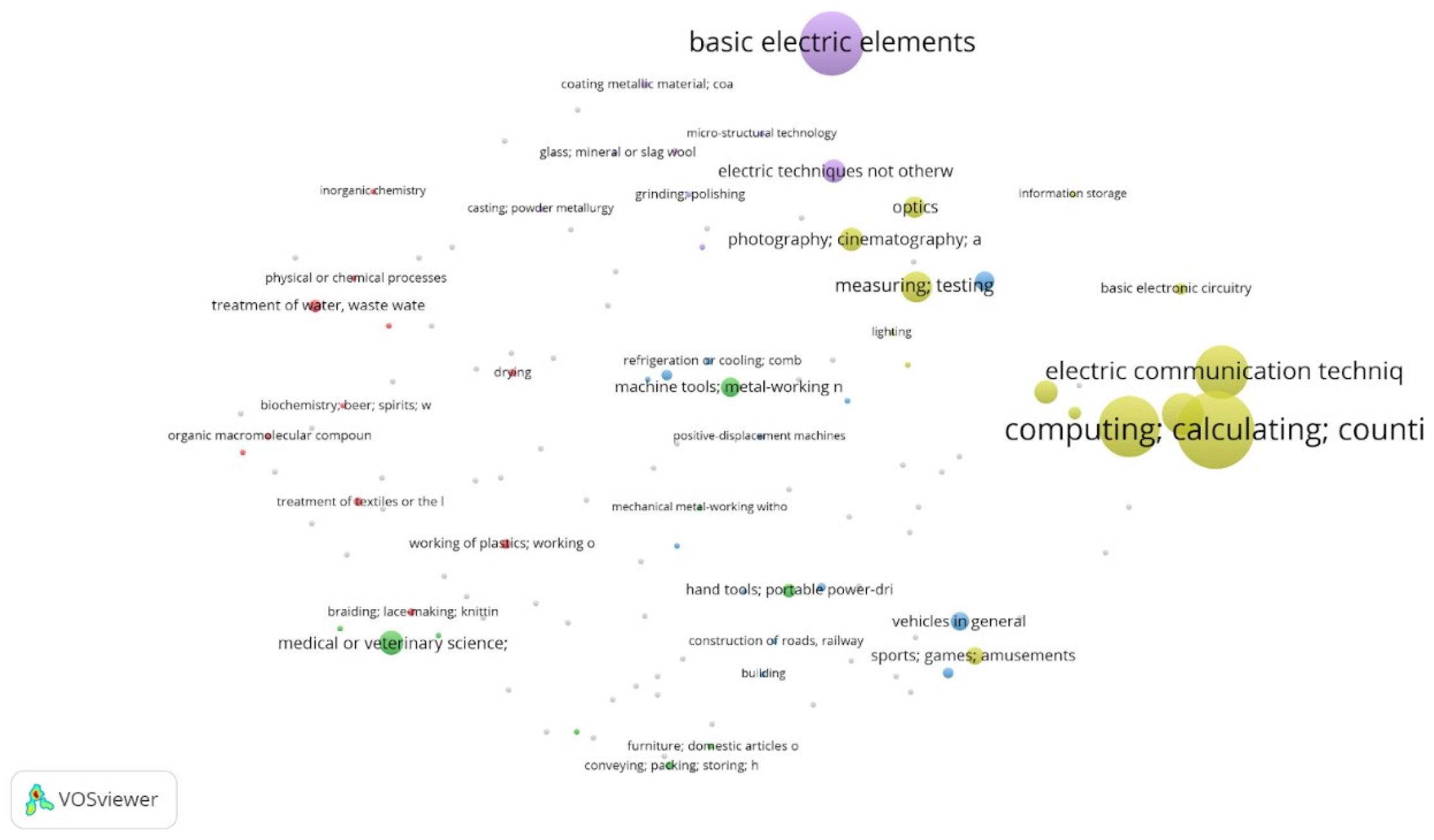
Figure 11:
2009-2013 Intelligent Technology Patents Classification.
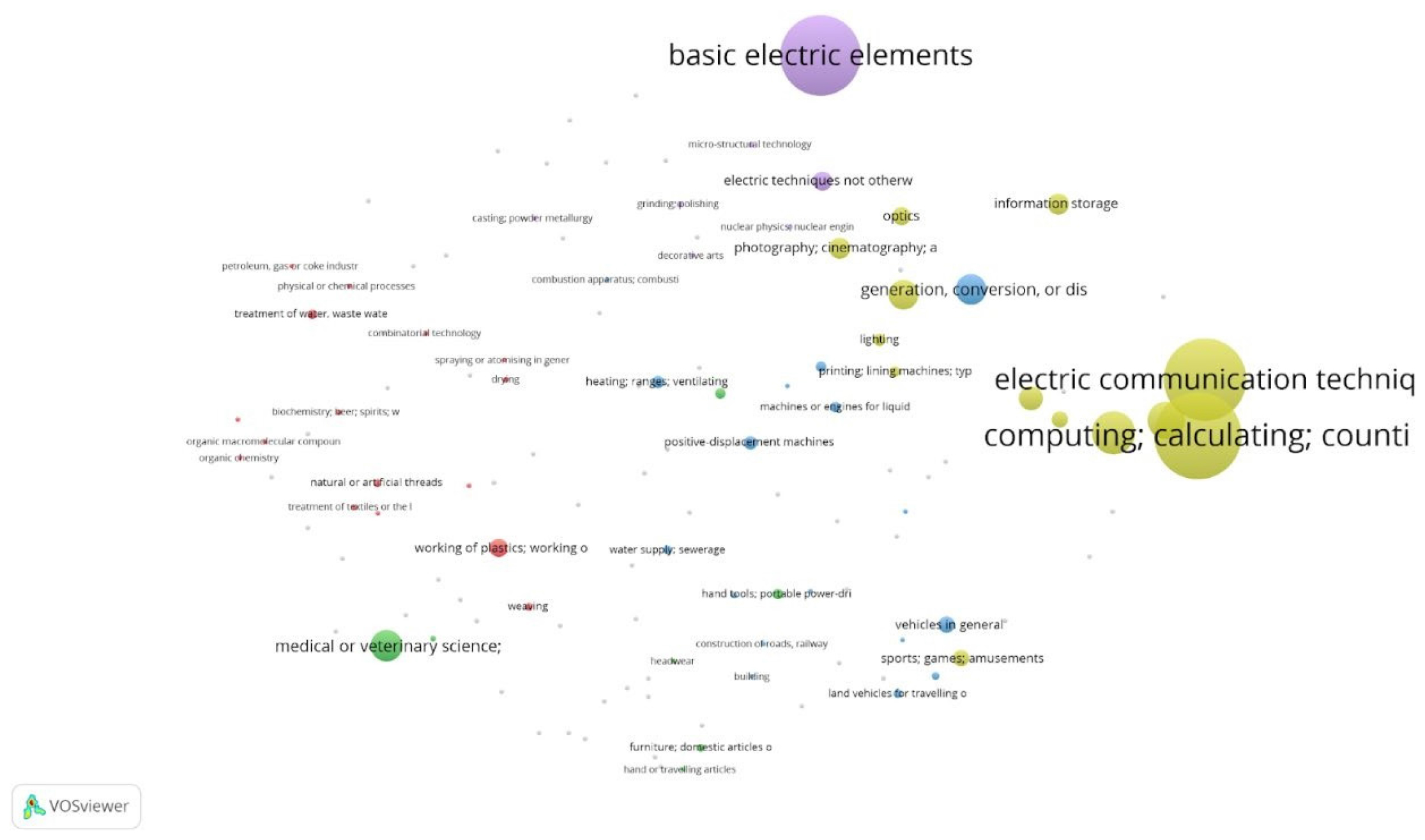
Figure 12:
2014-2018 Intelligent Technology Patents Classification.
In the domain of scientific research, it is observed that the majority of research in the early period was focused on Engineering Electrical Electronic (EEE), followed by computer science interdisciplinary and acoustics. In the later period, EEE and related fields remained the primary research areas, developing emerging fields, particularly in interdisciplinary studies, such as multidisciplinary chemistry, biotechnology applied microbiology, and biochemical research methods. Explain the rise of interdisciplinary research and demonstrate the characteristics of intelligent technology.
In the domain of technological applications, computing, calculating, counting, and essential electric elements were predominant in the early period. In the later period, there were a growing number of applications in electric communication techniques, along with an increase in the application of technologies such as generation, conversion, or distribution of electric power. This indicates the widening application scope of intelligent technology, echoing the rise of cloud computing and big data in 2014.[11,12]
Examining the linkage between science and technology application domains reveals numerous shared areas. Scientific research in fields such as EEE, computer science interdisciplinary, and acoustics provides theoretical foundations and discoveries that can be applied to develop new technologies and products. For instance, research in EEE can lead to the development of new electronic devices, while computer science research can contribute to the development of new computational methods and algorithms. The interdependence between science and technology, where science provides theoretical foundations and discoveries applied by technology to solve practical problems, is a crucial driver of societal progress.
Impacts of Institutions on the Dynamics of Systems of Innovation
Through expert questionnaires conducted by 22 experts who have been working in research institutes, universities, and firms for more than ten years. Table 1 presents a structured analysis of the influences of various dimensions and criteria on the development path of emerging technologies at both national and sectoral levels.
Dimension | Criteria | Weights | Rank |
---|---|---|---|
Knowledge Base | Technological Accumulation in Related Sectors | 0.040 | 7 |
National Funding of Basic Research | 0.047 | 5 | |
National Scientific Education | 0.017 | 8 | |
National/Sectoral Institutional | The Impact of the Pandemic | 0.222 | 2 |
Context | Government Industrial Policy | 0.005 | 10 |
Venture Capital Market | 0.395 | 1 | |
Actor Network | Business Transformation Strategy | 0.043 | 6 |
Collaboration With Firms, Universities, and Research Institutions | 0.140 | 3 | |
Introduction of Emerging Technologies and Technology Cross-domain Integration | 0.016 | 9 | |
Introduction of Foreign Technologies | 0.075 | 4 |
In the dimension of knowledge base, technological accumulation in related sectors is considered as the moderate impact (Weight: 0.040, Rank: 7). National funding of basic research, demonstrates its moderately influential role in shaping the knowledge base. National scientific education is perceived as a less immediately impactful factor with a lower weight and rank (Weight: 0.017, Rank: 8). Representative technology accumulation plays an important role. However, we found that national science education is a part that needs to be improved to improve the quality of science education in the field of intelligent technology.
In the dimension of national/sectoral institutional context, the impact of the pandemic emerges as a standout factor with a substantial weight and rank (Weight: 0.222, Rank: 2), underscoring its significant sway over the institutional landscape. In the contrast, government industrial policy, notably low weight and rank (Weight: 0.005, Rank: 10), hints at a more peripheral role in shaping the institutional context. The venture capital market is identified and celebrated with the highest weight and rank (Weight: 0.395, Rank: 1), emphasizing its pivotal and indispensable role in propelling emerging technology development at national and sectoral. In recent years, the pandemic has accelerated digitization and affected investment markets, making its impact significant. In contrast, government industrial policies have minimal influence on emerging technology development. This shows room for improvement in government formulation and implementation in the rapidly changing intelligent technology sector.
In the dimension of the actor network, the business transformation strategy, with its relatively lower weight and rank (Weight: 0.043, Rank: 6). This means whilst the business transformation strategy is a factor to be considered, its impact might be less. In contrast, collaboration with firms, universities, and research institutions emerges as a more potent force, boasting a higher weight and rank (Weight: 0.140, Rank: 3), indicative of its pivotal role in steering strategies and shaping the dynamic landscape of emerging technology development. In intelligent technology, introducing foreign technologies (Weight: 0.075, Rank: 4) seems more critical than introducing emerging technologies and technology interdisciplinary integration (Weight: 0.016, Rank: 9). This also can be found in Figures 5 and 6.
FINDINGS AND DISCUSSION
The study finds that in scientific innovation, there is a rise in interdisciplinary research on intelligent technology. From 2014 to 2018, more and more research began to involve the intersection between different disciplines. These innovations primarily occur within academic research institutions. Through interviews, it was found that universities tend to commercialize research outcomes by obtaining patents before engaging with firms. This approach is primarily driven by the need to protect intellectual property and ensure the recovery of research and development costs.[45,46] However, patenting research outcomes requires significant time and resources, leading many academic institutions to halt research at the development stage and hindering further commercialization efforts.[47]
Regarding technological innovation, the early stage focuses on researching and developing more basic calculations, counting, and necessary electronic components. In the later period, due to the rise of cloud computing and big data,[6,7] technology shifted to power communication technology. Meanwhile, due to the improvement in intelligent technology, individual patented inventions have gradually decreased and turned to corporate invention patents. However, the overall number of patent applications is not significant. The result of MCDM shows that the proportion of imported foreign technologies is relatively high, which shows that in terms of commercial applications, firms prefer to introduce foreign technology directly, which illustrates the need for more technological research and development.
Regarding how institutional factors affect the innovation system, this study found that experts asked the governmentâs industrial policy to pay less attention than other indicators. Based on the interview result, we found that the governmentâs industrial policy is relatively indifferent to small and medium- sized firms, and regulations cannot keep up with industrial development speed, which hasnât provided. However, the venture capital market is the most critical factor influencing sector development. In contrast, government industrial policies have minimal influence on emerging technology development. This shows the gap for improving future policy implementation in the rapidly changing intelligent technology sector. From the network analysis of this study, it can be observed that most of the participating companies in the field of intelligent technology are Small and Medium-sized firms (SMEs), and the government should create more regulations and policies for promoting the emerging sectors.
CONCLUSION AND FUTURE WORK
Summary of Findings
The study found the vertical innovation value chain of the intelligent technology sector in Taiwan havenât been well-developed.[5] There was much scientific development, but the number of commercial applications based on intellectual property rights considerations was insignificant. The number of patent applications in technology was insignificant. Companies tend to introduce foreign technologies directly through international business network. Such a result will lead to the sector over-reliance on foreign technology, and a lack of local innovation emerging technology, which highlights the weakness capability of the intelligent technology innovation ecosystem in Taiwan. Therefore, this study suggests that future policy should pay more attention to improve R&D commercialization abilities. The future policy should promote academia-sector collaborations enhance collaboration between firms, research institute, and academia.
Contributions and Future Work
This study contributes by in integrating various methods and tools, including scientometric mapping, social network analysis, and MCDM, to understanding the develop of intelligent technology innovation system. Noteworthy, this research integrates the NBIS framework and MCDM, offering a novel approach to evaluate the innovation system comprehensively. Moreover, based on the findings, policymakers should focus on enhancing support for interdisciplinary research, fostering domestic research and development capabilities, and streamlining regulatory frameworks to promote innovation and competitiveness in the intelligent technology sector. There is a need for strategic investments in emerging technologies to maintain the competitiveness in the rapidly evolving market landscape. This research contributes to enhance the knowledge flow in the innovation system, thus, to upgrade the national capabilities of small emerging economies in East Asia.
ACKNOWLEDGEMENT
This work was funded by the National Science and Technology Council under grant number NSTC 109-2511-H-009-007, 110-2628-H-A49-002-MY3, and 113-2410-H-A49-019-MY2. This research was also partially funded by the Higher Education Sprout Project of the National Yang Ming Chiao Tung University, funded by the Ministry of Education (MOE), Taiwan. Duen-Kai Chen acknowledged the Taiwan Visiting Fellowship funded by the Taipei Economic and Cultural Representative Office (TECRO) in the United States, Taiwan Ministry of Foreign Affairs. Duenkai is also grateful for the support of the Indo- Pacific Program (formerly Asia Program) at the Woodrow Wilson International Center. Shih-Hsin Chen would like to express her sincere gratitude for the visiting fellowships at the Center for Asia Policy Studies (CEAP) at the Brookings Institution, the support and help from the Global Taiwan Institute (GTI), and the funding provided by the Taipei Economic and Cultural Representative Office (TECRO) in the United States. Hung-Chi Chang appreciates the financial support of National Yang Ming Chaio Tung University (NYCU) abroad research scholarship. HungChi additionally acknowledged the guidance and support from the Urban Innovation Policy Lab of Edinburgh Napier University. The view expressed, and the remaining errors are the authorsâ own.
This work was funded by the National Science and Technology Council under grant number NSTC 109-2511-H-009-007, 110-2628-H-A49-002-MY3, and 113-2410-H-A49-019-MY2. This research was also partially funded by the Higher Education Sprout Project of the National Yang Ming Chiao Tung University, funded by the Ministry of Education (MOE), Taiwan. Duen-Kai Chen acknowledged the Taiwan Visiting Fellowship funded by the Taipei Economic and Cultural Representative Office (TECRO) in the United States, Taiwan Ministry of Foreign Affairs. Duenkai is also grateful for the support of the Indo- Pacific Program (formerly Asia Program) at the Woodrow Wilson International Center. Shih-Hsin Chen would like to express her sincere gratitude for the visiting fellowships at the Center for Asia Policy Studies (CEAP) at the Brookings Institution, the support and help from the Global Taiwan Institute (GTI), and the funding provided by the Taipei Economic and Cultural Representative Office (TECRO) in the United States. Hung-Chi Chang appreciates the financial support of National Yang Ming Chaio Tung University (NYCU) abroad research scholarship. HungChi additionally acknowledged the guidance and support from the Urban Innovation Policy Lab of Edinburgh Napier University. The view expressed, and the remaining errors are the authorsâ own.
References
- . The Emerald Handbook of Computer-Mediated Communication and Social Media. 2022:481-92.
- Matin A, Islam MR, Wang X, Huo H, Xu G. AIoT for sustainable manufacturing: Overview, challenges, and opportunities. Internet of Things. 2023;24 [Google Scholar]
- Amsden AH. Taiwan from an international perspective. Taiwan Firms in Global Perspective. 2020:25-52. [Google Scholar]
- Hauge J. Industrial policy in the era of global value chains: Towards a developmental framework drawing on the industrialization experiences of South Korea and Taiwan. The World Economy. 2020;43(8):2070-92. [Google Scholar]
- Rotolo D, Hicks D, Martin BR. What is an emerging technology?. Research policy. 2015;44(10):1827-43. [Google Scholar]
- McCarthy J. Artificial Intelligence, Logic, and Formalising Common Sense. Machine Learning and the City: Applications in Architecture and Urban Design. 2022:69-90. [Google Scholar]
- Durao F, Carvalho JFS, Fonseka A, Garcia VC. A systematic review on cloud computing. The Journal of Supercomputing. 2014;68:1321-46. [Google Scholar]
- Yang C, Huang Q, Li Z, Liu K, Hu F. Big Data and cloud computing: innovation opportunities and challenges. International Journal of Digital Earth. 2017;10(1):13-53. [Google Scholar]
- Dosi G, Grazzi M, Moschella D. Technology and costs in international competitiveness: From countries and sectors to firms. Research policy. 2015;44(10):1795-814. [Google Scholar]
- Dosi G, Grazzi M, Mathew N. The cost-quantity relations and the diverse patterns of âlearning by doingâ: Evidence from India. [Google Scholar]
- Nordmann A. Converging technologies: shaping the future of European societies: Office for Official Publications of the European Communities Luxembourg. 2004 [Google Scholar]
- Wolbring G. Why NBIC? Why human performance enhancement?. Innovation: The European journal of social science research. 2008;21(1):25-40. [Google Scholar]
- Antonopoulou K, Begkos C. Strategizing for digital innovations: Value propositions for transcending market boundaries. Technological Forecasting and Social Change. 2020;156 [Google Scholar]
- Drucker PF. The essential drucker. 2020 [Google Scholar]
- Freeman C. The Long-Wave Debate. 1987 [Google Scholar]
- Lundvall B-Ă . The Elgar Companion to Innovation and Knowledge Creation. 2017:472-89. [Google Scholar]
- Nelson RR. Regional innovation, knowledge and global change. 2013:11-26. [Google Scholar]
- Fernandes C, Farinha L, Ferreira JJ, Asheim B, Rutten R. Regional innovation systems: what can we learn from 25 years of scientific achievements?. Regional studies. 2021;55(3):377-89. [Google Scholar]
- Malerba F. Sectoral systems of innovation and production. Research policy. 2002;31(2):247-64. [Google Scholar]
- Markard J. The life cycle of technological innovation systems. Technological forecasting and social change. 2020;153 [Google Scholar]
- Bartholomew S. National Systems of Biotechnology Innovation: Complex Interdependence in the Global System. Journal of International Business Studies. 1997;28(2):241-66. [Google Scholar]
- Chaturvedi S. Evolving a National System of Biotechnology Innovation. Science, Technology and Society. 2005;10(1):105-27. [Google Scholar]
- Chen S, Forbes I, Martin P. Innovation Systems and Capabilities in Developing Regions. 2012:97-118. [Google Scholar]
- Skov F. Science maps for exploration, navigation, and reflectionâA graphic approach to strategic thinking. Plos one. 2021;16(12) [Google Scholar]
- Rafols I, Porter AL, Leydesdorff L. Science overlay maps: A new tool for research policy and library management. Journal of the American Society for Information Science and Technology. 2010;61(9):1871-87. [Google Scholar]
- Rotolo D, Rafols I, Hopkins MM, Leydesdorff L. Strategic intelligence on emerging technologies: Scientometric overlay mapping. Journal of the Association for Information Science and Technology. 2017;68(1):214-33. [Google Scholar]
- Dahesh MB, Tabarsa G, Zandieh M, Hamidizadeh M. Reviewing the intellectual structure and evolution of the innovation systems approach: A social network analysis. Technology in Society. 2020;63 [Google Scholar]
- Singh A, Malik SK. Major MCDM Techniques and their application-A Review. IOSR Journal of Engineering. 2014;4(5):15-25. [Google Scholar]
- Saaty TL. Decision making with the analytic hierarchy process. International journal of services sciences. 2008;1(1):83-98. [Google Scholar]
- Asadabadi MR, Chang E, Saberi M. Are MCDM methods useful? A critical review of analytic hierarchy process (AHP) and analytic network process (ANP). Cogent Engineering. 2019;6(1) [Google Scholar]
- Archibugi D, Pianta M. Specialization and size of technological activities in industrial countries: The analysis of patent data. Research Policy. 1992;21(1):79-93. [Google Scholar]
- Robinson DK, Ruivenkamp M, Rip A. Tracking the evolution of new and emerging S&T via statement-linkages: Vision assessment in molecular machines. Scientometrics. 2007;70:831-58. [Google Scholar]
- Huang Y, Schuehle J, Porter AL, Youtie J. A systematic method to create search strategies for emerging technologies based on the Web of Science: illustrated for âBig Dataâ. Scientometrics. 2015;105:2005-22. [Google Scholar]
- Zitt M, Bassecoulard E. Delineating complex scientific fields by a hybrid lexical-citation method: An application to nanosciences. Information processing & management. 2006;42(6):1513-31. [Google Scholar]
- Xia J. Chinaâs telecommunications evolution, institutions, and policy issues on the eve of 5G: A two-decade retrospect and prospect. Telecommunications Policy. 2017;41(10):931-47. [Google Scholar]
- Frith J. Smartphones as locative media. 2018 [Google Scholar]
- Feigenbaum EA. Assuring Taiwanâs innovation future. 2020 [Google Scholar]
- Van Eck N, Waltman L. Software survey: VOSviewer, a computer program for bibliometric mapping. scientometrics. 2010;84(2):523-38. [Google Scholar]
- Leydesdorff L, de Nooy W. Can âhot spotsâ in the sciences be mapped using the dynamics of aggregated journalâjournal citation Relations?. Journal of the Association for Information Science and Technology. 2017;68(1):197-213. [Google Scholar]
- Xia W, Jiang Y, Zhu W, Zhang S, Li T. Research Fronts of Computer Science: A Scientometric Analysis. Journal of Scientometric Research. 2021;10(1):18-26. [Google Scholar]
- Callon M, Courtial JP, Laville F. Co-word analysis as a tool for describing the network of interactions between basic and technological research: The case of polymer chemistry. Scientometrics. 1991;22:155-205. [Google Scholar]
- Tate WL, Ellram LM, Kirchoff JF. Corporate social responsibility reports: a thematic analysis related to supply chain management. Journal of supply chain management. 2010;46(1):19-44. [Google Scholar]
- Sharma G, Dahlstrand Ă L. Innovations, informality, and the global south: A thematic analysis of past research and future directions. Technology in Society. 2023 [Google Scholar]
- Yao N, Guo Q, Tsinopoulos C. The bright and dark sides of institutional intermediaries: Industry associations and small-firm innovation. Research Policy. 2022;51(1) [Google Scholar]
- Baldini N. University patenting and licensing activity: a review of the literature. Research Evaluation. 2006;15(3):197-207. [Google Scholar]
- Fabrizio KR. University patenting and the pace of industrial innovation. Industrial and Corporate Change. 2007;16(4):505-34. [Google Scholar]
- Daniel AD, Alves L. University-industry technology transfer: the commercialization of universityâs patents. Knowledge Management Research & Practice. 2020;18(3):276-96. [Google Scholar]