ABSTRACT
To combat the effects of climate change and meet the need for clean energy, the global power sector, over the past few decades, has been undergoing a major transformation for which all possible renewable energy sources are currently being utilized. To achieve sustainable growth, India, like many other countries, is also in the process of energy transition, aiming to shift to renewable energy-based power generation. In this transition, research in Ocean Renewable Energy (ORE) technologies is rising to rapid prominence. This study examines the state of ORE research in India and compares it with global research activities in this field using a graph-theoretical framework for collaboration co-authorship networks in ORE using bibliometric data on published scholarly articles indexed in two well-known electronic databases covering two 10-year windows: 1999-2008 and 2009-2018, inclusive. A strategic analysis of a number of metrics characterizing the networks’ large-scale structures reveals that the Indian network is highly fragmented, resulting in a singular dearth of large-scale connections for Indian ORE researchers. We recommend effective research policies to improve knowledge generation and dissemination in ORE research collaboration in India (and many other countries in similar situations), based on our findings for Indian networks and pertinent parallels with global ORE. With growing concerns about sustainable energy utilization, our study has policy implications for pressing issues of energy demands in the country.
INTRODUCTION
Increasing population and rapid industrialization today impose heavy demands on the capacities of future energy generation, subject to the necessary control of carbon emission in the process. Arbitrary and unsustainable consumption of energy leads to a rise in temperature, the melting of glaciers, more frequent climatic disasters, natural calamities, and unprecedented catastrophes,[1] all causing irreversible damage to agriculture, food security, water resources, ecology, and public health.[2] A major reduction of greenhouse emissions was strongly advocated in the 1997 Kyoto Protocol.[3] Further, in the 2015 Paris Agreement, specific goals and objectives were set for lowering carbon emissions.[4] According to the IPCC Report 2022, only extreme reductions in present carbon emissions can hope to prevent large-scale environmental catastrophes[5] (IPCC 2022). The Carbon Dioxide (CO2) emissions from the energy sector reached a record high in 2018, with a 1.7% rise since 2013.[6] Coal, natural gas, and oil contributed, respectively, close to 44%, 20%, and 35% of energy-related CO2 emissions in 2014, in addition to considerable quantities of other greenhouse gases, such as methane and nitrous oxide.[7] Various international bodies like the International Energy Agency (IEA), the International Renewable Energy Agency (IRENA), the World Health Organization (WHO) etc., are trying to achieve SDG 7 to provide affordable, sustainable and modern energy for all.[8] Therefore, the shift of the energy sector to a clean green form of energy remains the only necessity for survival.
In the process of shifting to cleaner green forms of energy various sources of renewable energy are being explored and emerging technologies to harness such forms of energy are in the forefront. One of such sources is Ocean Renewable Energy (ORE), a predictable bountiful source of energy in the form of heat, currents, waves, and tides is gradually gaining prominence since the past few years. Understanding the potential of ORE, the United Kingdom had steadily progressed by leading in technological development, especially in tidal stream and ocean wave technologies, followed by the United States. Canada and Norway (IEA-OES 2007) as ORE energy extraction is possible only through major technological developments for harnessing tidal energy, wave energy, Ocean Thermal Energy Conversion (OTEC), and salinity gradient.[9] Globally more than 30 nations are engaged in rapid development of over 100 distinct ocean energy technologies suffice that harnessing the immense potential of ocean energy is of major consideration worldwide as at present the competitiveness of ocean energy over other forms of renewable energy is its cost-effectiveness which can be attained again only through development of cost-effective technologies. This in turn opens the door to industry which is expected to grow more with conducive regulatory policies to develop ocean energy global markets specially under emerging innovation frameworks like open innovation, responsible innovation and innovation.
Foresight which is moving away from theoretical aspects to more heuristic tools that bridge innovation scales and system boundaries with relevant terms focusing on sustainability, actors and knowledge networks.[10] The global ocean energy market is still in nascent stages with select major companies operating in the global ocean energy market like Abengoa Seapower, Able Technologies LLC, Acubens, AeroVironmentInc, AlbaTERN, Applied Technologies Company, Ltd., (ATC), Aquagen Technologies, Aqua-Magnetics Inc, Aquamarine Power, and Atargis Energy Corporation.[11] Studies indicate that increasing the operational efficiency of ocean energy technologies is a primary requirement that the industry is expecting to take the sector forward. At this critical phase, evidence-based research to policy-makers enables them to formulate effective policies to facilitate the development of ORE technologies in order to achieve sustainable goals in green energy production and use.
India still relies heavily on fossil fuels for their energy requirements. After China, it is the second-largest consumer of coal in the world.[7] India consumed 3.9% of the world’s oil in 2010, making it the fourth-largest user of both oil and gas. In 2020, India’s per-capita greenhouse gas emissions were 2.4 tCO2e (tonne carbon dioxide equivalent), significantly lower than the global average of 6.3 tCO2e.[12] Economic development, limited availability of fossil fuels, and environmental protection are the main constraints on the new energy transition.[13] The energy transition is being regarded as a “multidimensional, complex, nonlinear, nondeterministic, and unpredictable event”.[14]
At present, onshore wind energy and solar energy have been successfully commissioned on land in India. Compared to land, the ocean has more available resources. ORE provides a number of significant benefits over other renewable energy sources: Predictable and trustworthy[15,16] and has received considerable importance for its role as a critical source of renewable energy.[17] India, with its coastline approximately 7,500 kilometers long, has most favourable conditions for exploiting ORE in the future. Moreover, by virtue of its highly favourable geographical location with an average temperature above 20°C between the sea surface and 1,000 meters of depth throughout the year, is able to generate thermal energy from the oceans on its three sides: the Bay of Bengal on the east, the Arabian Sea on the west, and the Indian Ocean on the south. Therefore, both academic and industrial research in ORE for technological innovation and development has featured significantly at the policy level. Out of the 2.3 million square kilometres of an exclusive economic zone (EEZ) that is presently available, an area of 1.5 million square kilometres has already been explored,[18] with a power density of 0.2 Megawatts (MW) per square kilometre of surface area.
ORE research in India is mostly carried out by the public sector, especially by central government ministries and departments and over the past few decades India has been confined to a few scattered and isolated research clusters. These agencies focus primarily on governmental priorities. Our study unravels the patterns of ORE collaboration and maps them to a number of critical inputs to ocean energy policy. It offers a much clearer picture of the current state of ORE research in terms of funding, institutional support and incentives, as well as professional encouragement needed for more extensive collaborative activities, connecting the Indian research community with the rest of the world.[19] Technological developments and innovations critically depend on the state of large-scale collaborative activities in ORE research to foster effective channels of communication, idea-sharing, resource utilization, and knowledge dissemination.[20] Our study examines the large-scale structures of the collaboration networks of ORE researchers in the country as disconnected research networks militates against operations of long-range ties. By contrast, inter-cluster bridges act as vital mediators, leading to a higher rate of generation of new ideas and best practices.[21,22] Intensive research collaboration between academic researchers, industry practitioners, and government scientists can create a strong synergy to heighten public awareness in ORE. The strategic initiatives for policy-making should seek potential partners for extensive knowledge-sharing and technology development.[23] It also helps policymakers to formulate better policies to harness clean, predictable, and renewable energy from the ocean.
In particular, our study addresses on the following two questions:
- What is the current state of ORE research in India?
- What are the structures of collaboration patterns in ORE research in India as compared with those internationally?
Our research study has important implications for policy-makers to review and enhance the current state of ORE research in the country, because ORE is now in active consideration at the government policy level for academic and industrial development in the country. Subsequent technological developments in ORE necessitate large investments in terms of the utilization of diverse categories of both tangible and intangible resources.
BACKGROUND
Global Developments
The increasing impact on climate change even after the Paris Agreement of COP21 in 2015 made it indispensable to reflect that there is a need for setting a more realistic target and the outcome of COP26 therefore was the Glasgow Climate Pact in 2021, negotiated through consensus representatives of 197 nations. The pact was the first climate deal to clearly commit to decrease the use of coal. The major challenge for achieving the targets lies in the measures to modify the business environment from the perspective of resource consumption that generates pollution. Clean energy can unlock and bring sustained economic growth along with meeting the needs of social sectors including health, education, clean cooking, digital transactions and reducing poverty which can also light up these poor nations.[8] Achieving the United Nations’ Sustainable Development Goals (SDG) which calls for “affordable and clean energy,” is a direction in which ocean energy is rapidly advancing.1 The ocean’s waves, tides, and winds contain vast amounts of energy, all of which are naturally occurring and devoid of carbon dioxide. To fully utilize its potential, it is not only essential to overcome a series of technological and financial obstacles but also solve multiple socio-economic challenges, including, for instance, maritime tourism, aquaculture, and blue biotechnology.[24] Globally, the cumulative installed capacity of all ocean energy systems is now 535 MW. It is projected to reach 10 gigawatts of installed capacity by 2030.[25] Even during the recent Covid-19 pandemic, significant advancement has taken place in R&D as well as demonstration and validation of ORE technologies.[26] The integration of renewable energy and sustainable development (RE&SD) can help overcome existing obstacles and create opportunities for renewable energy deployment to achieve sustainable development goals. In 2021, two projects – EU-SCORES and FORWARD-2030 – started with €35m and €21m of funding from the European Union’s Horizon 2020 programme to develop a multi-vector energy system that integrates ocean energy systems with wind or solar generation, grid export, battery storage and green hydrogen production. This is a significant step toward fast-tracking the commercial deployment of ORE technologies.
Ocean Renewable Energy in India: Then and Now
Because the current renewable energy development plans of the Indian Government are essentially land-intensive, intermittent, and low-capacity, it is necessary to diversify India’s renewable energy portfolio to include ocean renewable energy.[27] As shown in Figure 1, the estimated potential of ORE from different sources in India is quite high, constituting nearly 38% of the sum of total electricity produced from different sources and the estimated potential of ORE.
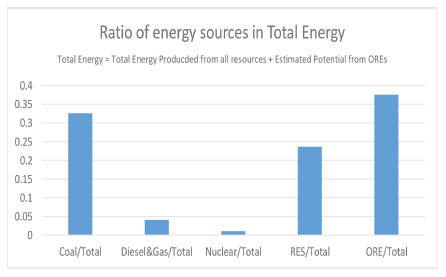
Figure 1:
Proportion of energy source with respect to total energy in India
Energy derived from the oceans by the use of offshore renewables helps decarbonize the power sector and is critical to the growth of a blue economy. However, expanding operations further offshore necessitates the availability of regular, reliable power independent of land-based power grids. On the Indian coast and in the Indian Ocean’s island groupings, attractive locations for land-based and floating Ocean Thermal Energy Conversion (OTEC) plants are in operation.
With ORE investments driven by the Ministry of Earth Sciences (MoES) of the Government of India, the country now aspires for becoming a global manufacturing hub for wave energy devices. Along the coast of India and in the island groupings of the Indian Ocean, there are enticing areas suited for both land-based and floating OTEC facilities.[28] A legislative committee has now requested the federal government to re-evaluate the potential of tidal, wave, and ocean-thermal energy to examine the nation’s exploitable potential.
In recent years, India has made a number of important technological developments in ORE, such as the Deep Ocean Mission and an OTEC powered desalination plant in Kavaratti, Lakshadweep.[27,29] India, a member of the International Energy Agency’s Ocean Energy Systems Group (IEA-OES), has also taken initiatives aimed at boosting the expansion of ocean energy systems in Asia.
The Indian Ocean Rim nations have taken steps to harness renewable energy resources to fulfil the region’s rising energy demand. In this regard, some recent government policies and enhancements may encourage production in ORE. Apart from expanding diverse local renewable energy sources, which include ocean energy, India, at the policy level, places a high premium on research collaboration in ORE.
Theoretical Background and Research Methodology
Earlier scholars have analysed technology networks to determine the relative importance of technology areas,[30] to evaluate and compare the significance of collaboration research communities within and across networks, and to visualize the global structures of networks.[31–33] Evolution of technology’s social network structure has been examined in terms of global network characteristics, actor centralities, and cooperating connections.[30] The discovery of relationships between resources and scholars and the interpretation of the relationships among them can help distinguish the most influential people, particularly in interdisciplinary sciences. Those people are important as the result of linking several scientific fields.[34]
In this study, we used bibliometric data from Elsevier’s SciVerse Scopus and Clarivate’s Web of Science (WoS) electronic databases to construct research collaboration networks in ORE. The following keywords were employed to retrieve the data: wave energy; tidal energy; ocean thermal energy conversion; OTEC; salinity gradient. These keywords comprised the title, the article’s keywords, and the abstract. Additionally, all document categories were saved for processing and analysis, including articles, conference papers, reviews, letters, research notes, editorials, book chapters, and abstract reports. We used the Pajek software package[35] to compute a few metrics. Fast numerical algorithms were used for others.
The networks
Through the investigation of co-authorship relationships among researchers in a certain field, one can determine the finest scholars in the field based on their distinguished social activities, meaning their social influence defined as the way an individual interacts with his colleagues in co-authorship social networks.[34] Co-authorship networks are formed by treating authors as nodes in the network; authors are connected if they co-authored a paper. The core units are ORE researchers. The network is described as a collection of nodes and edges as follows: G = {V, E}, where V is a set of n nodes (V1,…,Vn), and E is a set of m edges (E1,…,Em). Tie directionality is irrelevant; what matters is whether collaborative contacts between researchers result in the publishing of scientific papers. Our network is therefore undirected and is represented by a symmetric n × n adjacency matrix.
Author name resolution algorithms
The database sizes of Scopus and WoS are different, and the data we extracted varied in total size as well. In none of the datasets used to construct the network, it was possible to determine the exact number of authors. This is primarily due to the fact that an author’s name may appear differently in different papers.
Furthermore, two authors may have the same name. To distinguish names, additional resolution through home institutions and fields of specialization or disciplines is not fool proof either, since researchers may publish papers from more than one institution as well as in multiple disciplines or specialized areas.[36]
In order to account for multiple name variations, we employed an algorithm to construct two distinct versions of networks.[36] In the First Version (FI), we identified an author by his/her surname and only the first initial. This method introduces an error in the estimate by identifying two people as one; however, it hardly fails to identify two names that truly belong to the same person. In the Second Version (AI), we identified an author by surname and all the initials. This method enables us to distinguish names from one another but introduces an error in the estimate by identifying one person as two if their initials are specified differently in different papers, overestimating the number of authors in a database. We used the numbers of authors obtained in these two different versions to fix the upper and lower bounds of an interval [FI, AI] that contains the actual, albeit practically unobservable, number of authors in the networks.[37]
METRICS AND DISTRIBUTIONS
Co-authors distribution
We explored two important distributions in this work: (1) publications per author and (2) authors per paper. The degree centrality metric is the number of direct collaborators of a researcher. Someone with a high degree is related to many others in the network and is of relevance in a socio-professional setting. In this context, we investigated whether the networks were scalable. While some collaboration co-authorship networks are indeed scale-free,[38] there are notable exceptions as well.[36]
Density and Centralization
A network’s density (p) characterizes its degree of connections between nodes. It is quantified by the ratio of the network’s total number of edges to the total number of potential edges.[39] A related measure is the centralization (G) that indicates the degree to which collaborative relationships are tied to the network’s core players.[39] In particular, it indicates whether the network topology is bi-polar with a few core researchers and a large number of peripheral ones. A combined evaluation of degree, density, centralization, and cohesiveness reveals the presence of holes in the network.2
Small world
A network becomes a small world when most actors are not directly connected to one another but to others through a small number of intermediaries.[40] Globally, the average distance between pairs of actor’s scales as log (p). Because of this, pairs tend to be connected by short paths through the network.[38,41] Thus, research-related information does not have to travel far and wide through a small-world collaboration network. A useful comparison benchmark is a random network consisting of n nodes l ln(n) with a mean degree of θ. The spread scales as [41] Furthermore, networks tend to exhibit small-world characteristics over time.[41] In this situation, a combination of many local ties and a few more distant ones make knowledge transfer between nodes very efficient.[42]
Percolation
A giant cluster in a collaboration network is a large subset of researchers that are all connected to one another through intermediate ties. In its absence, the possibility of large-scale collaborative connectivity is minimal. When a new researcher joins the network, there is an overwhelmingly large probability that they will be connected to the giant cluster and not to one of the small clusters in it, which are typically independent of the number of researchers in the network. Empirically, a value greater than or equal to 65% commonly serves as a percolation threshold for collaboration networks in many disciplines.[36]
Cohesion and clustering
Theoretically, a research collaboration network should possess clustering,[43,44] where tightly coupled groups possess high internal ties and low external ones. We are not concerned here with local, ego-centred clustering but only with global cohesion, which is captured by the network’s transitivity characterizing the symmetry of interactions among transitive triads.[45] A random network, by contrast, should exhibit weak transitivity.[41] We computed the fraction of transitive triads in the complete network by the Clustering Coefficient (CC). This is the probability that any two randomly selected neighbouring researchers of a focal researcher are themselves collaborating neighbours. While density is a marginally global measure, global cohesion encodes the clustering of the complete network measured in terms of the maximally cohesive local triads. Thus, a globally sparse network may yet have highly clustered local sections.
We employed a same-sized random network for benchmarking cohesion. Here, clustering probability is equal to the probability that any two randomly selected researchers are themselves co-authors of papers. This is given by , where θ is the average collaboration degree, and n is the network size. The CC for most collaboration networks does not seem to decrease with n for constant 0 but remains largely independent of n.[36,43,44] The CC of a random network is given by CCrand = n–1, where n is the number of nodes. Naturally, the CC in such a network becomes exceedingly small in the limit of large network size.[41] In a co-authorship network, by contrast, interconnected social ties tend to increase the CC by a considerable margin.[36]
RESULTS
We compared the large-scale characteristics of collaboration co-authorship networks of Indian ORE researchers with those of the global network. Tables 2, 3, 4 and 5 exhibit the relevant network metrics and their associated distributions over the period of 20 years, from 1999 to 2018 (inclusive). The first entry in a cell in each Table 2–5 in the appendix is the AI value, and the second entry (in the bracket) is the FI. The exponent and cut-off parameters of the collaborator distributions are computed using the full 20-year period. For WoS, very few papers in ORE were published from India prior to 2009. Since this year, there has been a continuous flow of papers, although the total number of papers was not large. For example, in 2009 there were only seven papers published by 20 authors (AI); it reached 20 papers by 77 different authors in 2018. By contrast, the number of global publications in ORE in 1999 was 43 with 112 authors (AI), which increased to 583 papers with 2107 authors in 2018 (AI). SCOPUS shows a continuous flow of publications in ORE from India: in 1999, there were six papers with 14 authors (AI), which then increased to 70 publications with 251 authors in 2018. Globally, 314 papers in ORE were published by 831 authors in 1999 (AI), which then increased to 1548 papers in 2018 with 5743 authors (AI).
We illustrate the individual results in terms of AI only. As and when appropriate, we point out the significance of the FI results as well.
AUTHOR COUNTS
As seen from Tables 2, 3, 4 and 5 as well as from Figures 2A and 2B, the number of authors in the ORE networks gradually increases over the period 1999–2018 in India and globally in both the SCOPUS and the WoS databases. However, the average number of authors in the global network is higher than that in the Indian network; in particular, contributors from India occupy 4-5.5% share in global ORE in both WoS and SCOPUS. For India, the number of authors in SCOPUS increased approximately 18
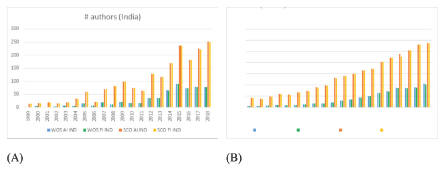
Figure 2:
Number of authors for AI and FI (India and global)
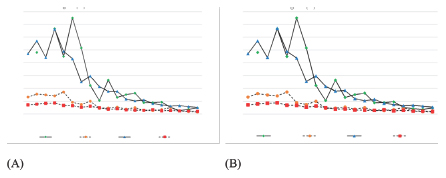
Figure 3:
Mean degree for AI and FI network for SCOPUS and WOS for 1999-2018 (India and global)
times over 20 years from 1999 to 2018; in WoS, the number of authors increased approximately four times over 10 years from 2009 to 2018. Globally, the number of authors increased 18 times in WoS and by 6 times in SCOPUS.
Collaborators per author
The Scopus average collaborators per author (that is, the mean degree) approximately doubled in 20 years (1999-2018) for AI in the global ORE network (and almost similarly for FI). In both India and globally, the average maximum mean degree is about 6.0 in 2013. In India, the AI mean degree is 1.875 in 1999 and 3.960 in 2018, the maximum mean degree being 11.94 in 2015. The AI average collaborators per author in WoS varies from 2.304 in 1999 to 4.775 in 2018. Globally, in the FI network, collaborator per author is the same in 1999, and in 2018 it is 4.891. In India, for both AI and FI WoS networks, the mean degree is 2.4 in 2000 and 3.740 in 2018.
The mean degree for AI and FI network varies between 2.0-7.3 and 1.8-11.9 for WoS and SCOPUS data respectively for India for the period 1999-2018 during which the number of authors increased from 5 to 90 in WoS coverage data and the number of authors increased from 14 to 249 authors SCOPUS data in last 20 years. However, the variation in mean degree is low for global research data from both WoS (2.3-5.9) and SCOPUS (2.5-6.0), whereas the number of authors increased more than 20 times for WoS (112-2107) and SCOPUS (831-5743).
As shown in Table 1, the Scopus exponents for the India AI and FI networks are about 2.0 and the corresponding WoS exponents are 2.5 and 3.1 respectively (Figure 4 (A-D). The Scopus exponents for AI and FI global networks are 3.2 and 3.1 respectively, and the corresponding WoS exponents are 3.1 and 2.9 respectively. The mean degrees for AI and FI Scopus networks in India are 5.9 and 6.1 respectively, and the WoS results are 4.2 and 4.3. Globally, these are 6.3 and 7.1 respectively for Scopus AI and FI and 5.3 and 5.7 for WoS. These results seem to indicate that compared with the global situation, ORE collaboration research in India is slowly gathering momentum in recent years.
The giant cluster size has decreased over the last 20 years both in India and globally in both WoS and SCOPUS, which indicates that new researchers or research groups join the research network without collaborating with any of the existing groups. The diverse nature of ORE research arises from activities in many subareas, such as electricity generating turbines, transmissions, capturing technologies, and different engineering streams. However, energy harnessing from the ocean in the form of waves, tides, salinity gradients and ocean thermals, the normalized mean degree reduces over the years (Figure 3A , 3B).
The collaborator distributions are shown in Figures 5 A-D. These are somewhat long-tailed; moreover, they exhibit some curvature and seem to follow truncated power laws of the form where α and kC are parameters of the distribution. Similar results have been reported by earlier researchers.[36,38] As is usually the case, the fits are performed in the tails of the distributions, shown in the figures by lines on a log–log scales. The power laws are cut off at finite values of collaboration strength, proportional to the maximum degrees of the distributions.[36,44] Found similar results with a very large cut-off amounting to about 5,800 for the MEDLINE biomedicine database.[46,47] Also reported large cut-offs in management and cancer research.
Density Centralization scores
The network density investigated here is small, in the range 10–3 – 10–4. With the addition of new researchers to the network over time, it is likely that the density will eventually become quite small. However, it appears implausible that a researcher’s collaborators rise in proportion to the number of newly added researchers to the network. Although our networks are sparse, the density scores do not imply that the Scopus and WoS databases have identical topological structures. Additionally, one must analyse the mean degrees and centralization scores.
For both Scopus and WoS, the centralization scores G are very low. In the WoS-India network, the normalized G varies from 0 to 0.067 for AI and in a similar range for FI for the period 1999-2018. However, G is 0.019 in 2007 for the WoS India AI and FI networks. For SCOPUS India, it varies from 0.003 to 0.026 for AI and 0.002 to 0.029 for FI respectively (Figure 6 right figures in the panel). Globally, varies from 0.0004 to 0.006 for WoS-AI and 0.0004 to 0.006 for WoS-FI networks (Figure 6 left figures in the panel). The centralization score for the global SCOPUS-AI network lies between 0.0002 and 0.0009 and in a similar range for SOUPUS-FI. Because the Indian and the global ORE networks are not centralized, one must examine the density and the associated centralization scores together with global structural cohesion in the network as well as with the sizes of giant clusters.
Average distance
Figures 7A and 7B display the average geodesic distance between a pair of researchers of Indian and global networks for the period 1999-2018 for both AI and FI. The average geodesic distance between researches lies in the range of 1-1.5 for the Indian network for Scopus and WoS database for AI and FI both. On the other hand, average geodesic distance between the researches of the global network lies between 1-5.9 for AI and FI network from WoS database and 1-6.8 for the Scopus database network for both the AI and FI. The difference arises from the database coverages. The cumulative value for average geodesic is calculated using a normalized (by mean degree of the respective year) measure.
India | Global | |||||||
---|---|---|---|---|---|---|---|---|
WoS | SCOPUS | WoS | SCOPUS | |||||
AI | FI | AI | FI | AI | FI | AI | FI | |
Mean Degree | 4.238 | 4.346 | 5.910 | 6.141 | 5.364 | 5.756 | 6.392 | 7.173 |
Exponent (a) | 2.523 | 3.129 | 2.002 | 2.045 | 3.185 | 2.929 | 3.242 | 3.142 |
Cut-off | 19.238 | 5.489 | 16.340 | 20.080 | 11.136 | 54.951 | 50.505 | 63.291 |
Density | 8.56*10-3 | 9.07*10-3 | 3.81*10-3 | 4.13*10-3 | 4.44*10-4 | 5.18*10-4 | 1.58*10-4 | 2.03*10-4 |
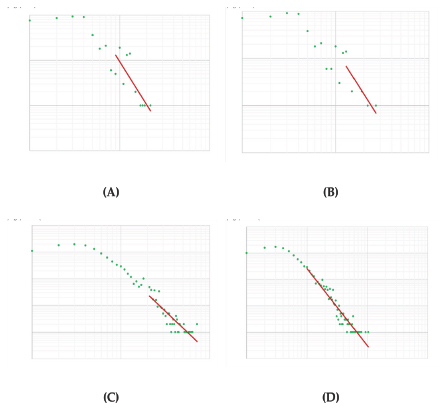
Figure 4:
Collaborators per author and exponents for WoS India and globally (1999-2018)
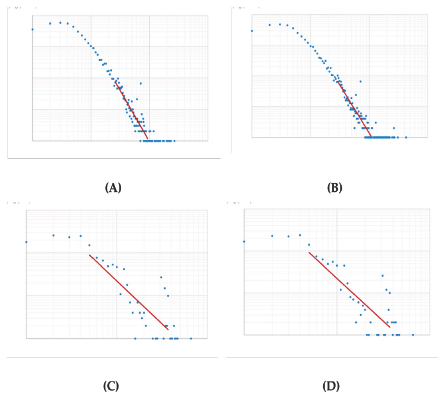
Figure 5:
Collaborators per author and exponents for SCOPUS India and globally (1999-2018)
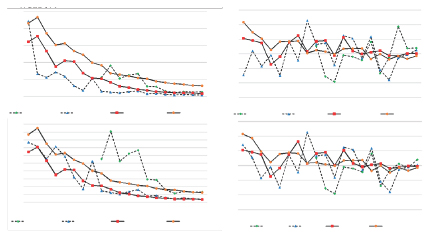
Figure 6:
Density and centralization scores of Indian and global ORE WoS and SCOPUS
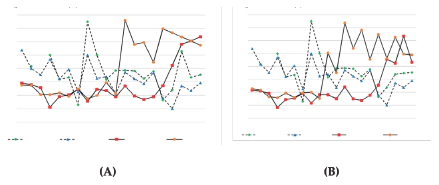
Figure 7:
Mean-degree-normalized average geodesic distance
Giant Cluster
Over the previous two decades, the size of large clusters in SCOPUS India has decreased from 28.5% to 4.7%, because a small number of co-authored papers were published. Its size grows from 5.35% to 13.7% for WoS-AI and from 5.35% to 14.44% for WoS-FI. It increases from 1.56% and 2.3% to 18.21% and 29.29% for SCOPUS-AI and SCOPUS-FI, respectively (Figure 8). Interestingly, the FI giant cluster is often significantly larger than the AI one for both SCOPUS and WoS. The reason is that the FI algorithm is prone to misidentify two distinct researchers as a single individual in some circumstances. As a result, some dispersed researchers may have joined the giant cluster in this manner. On a related issue, combining two researchers with the same first initial in FI might occasionally boost the size of the FI giant cluster if these researchers were previously members of distinct clusters. By contrast, if they are members of the same cluster, merging them will result in the expansion of the AI giant cluster. Indeed, there is a strong indication that both the Indian and the global networks lie much below the percolation threshold. While it is true that a tiny fraction of published researchers is related by collaboration, the networks contain many small clusters.
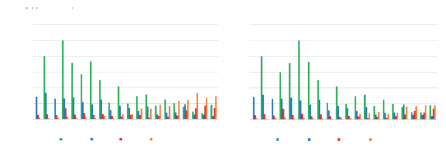
Figure 8:
Giant cluster evolution in Indian and global ORE WoS and SCOPUS
Clustering coefficient (CC)
Unlike in a random network, in most practical situations, linked social ties are likely to significantly increase CC.[41] The reason is that there is a greater likelihood that two researchers who are connected through a common collaborator may become collaborators with each other. This effect is readily apparent across all of our networks.
As shown in Tables 2-5 and Figure 9, our AI and FI networks exhibit CC values that are significantly larger than the equivalent random benchmark values. The CCs for both SCOPUS and WoS are in the range of 0.8–0.9, with the Indian and global values being the closest. Because of the low publication volume during the period 1999-2012, the CCs from the initial period for WoS-India have not been taken into consideration for the period 1999 to 2012.
CONCLUSION AND POLICY IMPLICATIONS
Our study has explored the network of collaborating researchers in ORE in India and globally to better understand and enhance current practices of research collaboration in India. Although India has made progress in ORE technology development, this is viewed as insufficient in today’s rapidly evolving technological landscape. Public, private, and international participation is urgently needed to hasten the development of the desired technologies that would support greater utilization of ORE in India, as environmental protection is the driving force behind a sustainable Blue Economy and the use of such technologies. A comparison of the Indian network with the global one also helps us to understand the current position of India in relation to international research in ORE. To deal with the concerned issues and their associated challenges, it is necessary to examine the microstructures of the collaboration processes. We performed a global analysis as a first approximation to the general scheme, for without such an analysis, the ego-centred microanalysis has nothing to guide it in determining the underlying causal structures of researchers’ collaborative associations.
The existence of large-scale network connections is determined by the degree of fragmentation as well as by bridges between small clusters in the network. Our analysis finds that the ORE network in India is still in its infancy and is largely fragmented into tiny research groups over the period from 1999 to 2018. Research in OTEC technology began in 1980 by researchers from NIOT, Chennai, under the Ministry of Earth Science, and was subsequently expanded by other research institutes such as IITs, Council of Scientific and Industrial Research (CSIR), Indian Institute of Science, and a number of other university departments interested in ORE. In this regard, difficulties were encountered as central researchers active in the field either retired from active employment or moved on to work in other areas due to various reasons, such as the unavailability of funds or the absence of more ambitious projects in ORE. To strengthen collaborative research structures in this area, policymakers should institute vigorous measures to encourage the creation of inter-cluster linkages. At present there seems to be hardly any presence of industry in ORE research in India. Our analysis also indicates this. Policy interventions for promoting strong academia-industry linkages are now of the essence, which will alleviate the disconnectedness in the network structure. The harnessing of tidal energy might become cost-effective by 2030 if its efficiency could be improved by 40% over current expectation or if costs could be reduced by 50%.[48] In this context, providing institutional and governmental incentives is likely to prove advantageous in fostering collaborations. It might also be worthwhile to organize national and international conferences to build a vibrant environment for bringing together Indian researchers to engage in more socio-professional interactions. This is likely to enhance the likelihood of a larger number of links in the ego-centred networks of researchers from several specialized fields of ORE in the nation.
The average geodesic distance is related to the total collaboration size and is also a good indicator of the degree of network connectivity. Our findings suggest that a high proportion of the ORE researchers in India publish papers with 2-5 co-workers on average. This low value works against the rapid creation of a giant cluster. Presumably, it also indicates that there is a significant concentration on specific research issues that tend to be confined to small groups. Although such a concentration might be
Year | No of Authors | Mean Degree | Average Distance | Density | Degree Centralisation | Betweenness Centrality | Eigenvector Centrality | cc | Giant Cluster |
---|---|---|---|---|---|---|---|---|---|
2009 | 20(20) | 2.600 (0.821)2.600 (0.821) | 1.000 (1.000) | 0.137(0.137) | 0.140(0.140) | 0(0) | 0.803(0.803) | 1.000 (1.000) | 20.000 (20.000) |
2010 | 17(17) | 2.588 (1.278)2.588 (1.278) | 1.000 (1.000) | 0.162(0.162) | 0.375(0.375) | 0(0) | 0.375(0.375) | 1.000 (1.000) | 29.412(29.412) |
2011 | 16(16) | 2.625 (1.204)2.625 (1.204) | 1.000 (1.000) | 0.175(0.175) | 0.367(0.367) | 0(0) | 0.39(0.39) | 1.000 (1.000) | 31.250(31.250) |
2012 | 35(35) | 3.086 (1.541)3.086 (1.541) | 1.000 (1.000) | 0.091(0.091) | 0.394(0.394) | 0(0) | 0.388(0.388) | 1.000 (1.000) | 17.143(17.143) |
2013 | 36(36) | 3.222 (1.514)3.222 (1.514) | 1.216(1.216) | 0.092(0.092) | 0.614(0.614) | 0.027(0.027) | 0.217(0.217) | 0.905(0.905) | 25.000(25.000) |
2014 | 65(64) | 6.062 (4.283)6.156 (4.258) | 1.000 (1.015) | 0.095(0.098) | 0.503(0.495) | 0(0.002) | 0.298(0.298) | 1.000(0.998) | 20.000(20.313) |
2015 | 90(89) | 4.689 (4.110)4.742 (4.108) | 1.121(1.128) | 0.053(0.054) | 0.646(0.642) | 0.007(0.007) | 0.089(0.089) | 0.98(0.979) | 15.556(15.73) |
2016 | 73(73) | 2.000 (1.707)3.123 (1.707) | 1.058(1.058) | 0.028(0.043) | 0.486(0.486) | 0.002(0.002) | 0.282(0.282) | 0.968(0.968) | 9.589(9.589) |
2017 | 80(78) | 3.000 (1.432)2.923 (1.236) | 1.000 (1.017) | 0.038(0.038) | 0.506(0.421) | 0(0.001) | 0.087(0.087) | 1(0.993) | 8.75(7.692) |
2018 | 77(77) | 3.740 (1.795)3.740 (1.794) | 1.32(1.320) | 0.049(0.049) | 0.634(0.634) | 0.010(0.010) | 0.104(0.104) | 0.876(0.876) | 18.182(18.182) |
Year | No ofAuthors | Mean Degree | AverageDistance | Density | DegreeCentralisation | BetweennessCentrality | EigenvectorCentrality | cc | Giant Cluster |
---|---|---|---|---|---|---|---|---|---|
2008 | 81 (81) | 3.827 (2.290)3.827 (2.290) | 1.299(1.299) | 0.048(0.048) | 0.714(0.714) | 0.013(0.013) | 0.183(0.2) | 0.898(0.898) | 17.284(17.284) |
2009 | 98(97) | 5.337 (3.815)5.392 (3.804) | 1.117(1.280) | 0.055(0.056) | 0.596(0.591) | 0.006(0.012) | 0.172(0.174) | 0.978(0.976) | 14.286(14.433) |
2010 | 74(74) | 2.838(1.664)2.838 (1.664) | 1.054(1.054) | 0.039(0.039) | 0.603(0.603) | 0.002(0.002) | 0.139(0.139) | 0.98(0.98) | 10.811(10.811) |
2011 | 64(63) | 3.219(2.746)3.206 (2.783) | 1.037(1.038) | 0.051(0.052) | 0.653(0.654) | 0.002(0.002) | 0.151(0.153) | 0.991(0.991) | 15.625(15.873) |
2012 | 128(128) | 3.617 (1.957)3.617 (1.957) | 1.037(1.037) | 0.028(0.028) | 0.487(0.487) | 0.001(0.001) | 0.076(0.088) | 0.989(0.989) | 6.250(6.250) |
2013 | 117(117) | 3.145(1.728)3.145 (1.728) | 1.156(1.156) | 0.027(0.027) | 0.656(0.656) | 0.002(0.002) | 0.166(0.185) | 0.94(0.94) | 8.547(8.547) |
2014 | 169(169) | 6.024(4.173)6.024 (4.173) | 1.093(1.093) | 0.036(0.036) | 0.573(0.573) | 0.001(0.001) | 0.084(0.065) | 0.979(0.979) | 8.876(8.876) |
2015 | 236(235) | 11.941(13.293)11.991 (13.300) | 1.212(1.214) | 0.051(0.051) | 0.725(0.724) | 0.006(0.006) | 0.23(0.231) | 0.982(0.982) | 19.068(19.149) |
2016 | 180(180) | 4.244(2.584)4.244 (2.584) | 1.147(1.147) | 0.024(0.024) | 0.579(0.579) | 0.002(0.002) | 0.082(0.049) | 0.955(0.955) | 6.111(6.111) |
2017 | 225(221) | 4.356(2.999)4.380 (2.983) | 1.020(1.040) | 0.019(0.02) | 0.640(0.638) | 0(0) | 0.089(0.087) | 0.996(0.992) | 5.778(5.882) |
2018 | 251(249) | 3.960 (2.059)5 Q7A nçvt | 1.157(1.147) | 0.016(0.016) | 0.643(0.605) | 0.001(0.001) | 0.076(0.082) | 0.939(0.938) | 4.781(4.418) |
Year | No of Authors | Mean Degree | AverageDistance | Density | DegreeCentralisation | BetweennessCentrality | EigenvectorCentrality | cc | Giant Cluster |
---|---|---|---|---|---|---|---|---|---|
1999 | 831(824) | 2.604(1.732) | 1.071(1.191) | 0.003(0.003) | 0.784(0.783) | 0.0001(0.0002) | 0.040(0.0401) | 0.966(0.9416) | 1.564(2.306) |
2.612(1.772) | |||||||||
2000 | 768(753) | 2.594(1.747) | 1.063(1.133) | 0.003(0.003) | 0.677(0.74) | 0(0.0001) | 0.037(0.0411) | 0.977(0.9507) | 1.172(1.461) |
2.611 (1.784) | |||||||||
2001 | 962(953) | 3.505(3.121) | 1.082(1.171) | 0.004(0.004) | 0.826(0.815) | 0.0001(0.0002) | 0.020(0.0212) | 0.982(0.9632) | 2.183(2.099) |
3.536 (3.178) | |||||||||
2002 | 1183(1166) | 4.453(4.873) | 1.373(1.422) | 0.004(0.004) | 0.847(0.846) | 0.0004(0.0003) | 0.022(0.022) | 0.949(0.9437) | 2.959(3.087) |
4.501 (4.904) | |||||||||
2003 | 1146(1131) | 3.391(2.482) | 1.109(1.331) | 0.003(0.003) | 0.758(0.756) | 0.0001(0.0002) | 0.034(0.0126) | 0.9695(0.9474) | 1.483(2.299) |
3.429 (2.536) | |||||||||
2004 | 1336(1314) | 3.865(4.510) | 1.117(1.271) | 0.003(0.003) | 0.867(0.885) | 0.0001(0.0002) | 0.021(0.0222) | 0.9780(0.9649) | 2.246(2.664) |
3.919 (4.578) | |||||||||
2005 | 1462(1440) | 3.418(2.639) | 1.257(1.349) | 0.002(0.002) | 0.773(0.770) | 0.0002(0.0002) | 0.014(0.0114) | 0.951(0.9341) | 1.778(1.875) |
3.455 (2.686) | |||||||||
2006 | 1803(1771) | 4.797(5.141) | 1.263(1.95) | 0.003(0.003) | 0.851(0.897) | 0.0001(0.001) | 0.025(0.0095) | 0.976(0.9469) | 1.83(4.517) |
4.872 (5.287) | |||||||||
2007 | 1955(1927) | 4.183(3.610) | 1.249(1.291) | 0.002(0.002) | 0.791(0.789) | 0.0001(0.0001) | 0.010(0.0129) | 0.962(0.9509) | 1.176(1.245) |
4.227 (3.654) | |||||||||
2008 | 2635(2576) | 4.362(3.480) | 1.927(4.487) | 0.002(0.002) | 0.802(0.798) | 0.0003(0.002) | 0.020(0.0197) | 0.920(0.8952) | 2.609(6.677) |
4.451 (3.574) | |||||||||
2009 | 2795(2709) | 4.999(5.045) | 1.591(3.505) | 0.002(0.002) | 0.865(0.865) | 0.0002(0.0022) | 0.172(0.0199) | 0.957(0.907) | 1.932(6.534) |
5.107 (5.245) | |||||||||
2010 | 2996(2884) | 4.230 (3-253) | 4.824(6.228) | 0.001(0.001) | 0.824(0.824) | 0.003(0.0085) | 0.139(0.015) | 0.922(0.8762) | 7.276(13.627) |
4.354 (3.431) | |||||||||
2011 | 3285(3165) | 4.353(3.133)4.480 (3.332) | 3.783(4.696) | 0.001(0.001) | 0.85(0.850) | 0.0025(0.0049) | 0.151(0.0206) | 0.901(0.8508) | 7.945(13.365) |
2012 | 3441(3304) | 4.524(3.410) | 4.035(6.172) | 0.001(0.001) | 0.887(0.887) | 0.0031(0.0112) | 0.076(0.0156) | 0.863(0.7858) | 9.91(18.432) |
4.681 (3.770) | |||||||||
2013 | 4049(3895) | 6(9.640) | 4.018(5.454) | 0.001(0.001) | 0.923(0.923) | 0.0024(0.007) | 0.166(0.0095) | 0.971(0.9549) | 7.409(17.022) |
6.207 (9.876) | |||||||||
2014 | 4424(4211) | 4.897(3.986) | 5.122(6.347) | 0.001(0.001) | 0.852(0.852) | 0.0024(0.0164) | 0.084(0.0193) | 0.8964(0.8169) | 8.929(23.201) |
5.128 (3.374) | |||||||||
2015 | 4776(4533) | 5.691(5.753) | 5.69(5.481) | 0.001(0.001) | 0.868(0.871) | 0.0046(0.0103) | 0.023(0.0251) | 0.9026(0.8447) | 16.227(24.423) |
5.941 (6.142) | |||||||||
2016 | 5091(4812) | 5.419(4.634) | 5.168(6.784) | 0.001(0.001) | 0.9(0.9) | 0.0044(0.0303) | 0.0817(0.0131) | 0.7984(0.7214) | 16.834(33.188) |
5.698 (5.141) | |||||||||
2017 | 5615(5368) | 5.956(7.102) | 5.413(5.905) | 0.001(0.001) | 0.92(0.92) | 0.0082(0.0161) | 0.0888(0.0132) | 0.864(0.8194) | 18.219(27.459) |
6.171 (7.359) | |||||||||
2018 | 5743(5462) | 5.521(4.510) | 4.749(5.392) | 0.001(0.001) | 0.919(0.919) | 0.0059(0.0157) | 0.0764(0.0141) | 0.767(0.6882) | 18.219(29.293) |
5.778 (5.052) |
Year | No of Authors | Mean Degree | AverageDistance | Density | DegreeCentralisation | BetweennessCentrality | EigenvectorCentrality | cc | Giant Cluster |
---|---|---|---|---|---|---|---|---|---|
1999 | 112(112) | 2.304 (1.354) | 1.000(1.000) | 0.021 (0.021) | 0.544 (0.544) | 0(0) | 0.144(0.144) | 1(1) | 5.357(5.357) |
2.304 (1.355) | |||||||||
2000 | 102(102) | 2.549 (1-520) | 1.065(1.065) | 0.025 (0.025) | 0.581 (0.581) | 0.001 (0.001) | 0.118 (0.123) | 0.953 (0.953) | 6.863(6.250) |
2.549 (1.520) | |||||||||
2001 | 135(135) | 2.607(1.644) | 1.000(1.000) | 0.02 (0.02) | 0.57 (0.57) | 0(0) | 0.150 (0.150) | 1.000 (1.000) | 5.185(5.185) |
2.607(1.644) | |||||||||
2002 | 205(204) | 5.946 (8.582) | 1.000(1.003) | 0.029 (0.029) | 0.784 (0.783) | 0(0) | 0.139 (0.139) | 1.000 (1.000) | 13.659(13.725) |
5.976 (8.594) | |||||||||
2003 | 171(170) | 3.661 (2-648) | 1.040(1.049) | 0.022 (0.022) | 0.597 (0.600) | 0(0) | 0.132 (0.076) | 0.985 (0.982) | 5.848(5.882) |
3.635 (2.657) | |||||||||
2004 | 175(174) | 3.303 (3-078) | 1.007(1.033) | 0.019 (0.019) | 0.729 (0.727) | 0 (0.001) | 0.111 (0.112) | 0.999 (0.993) | 7.429(7.471) |
3.322 (3.092) | |||||||||
2005 | 263(260) | 2.882(2-141) | 1.055(1.109) | 0.011 (0.011) | 0.715 (0.781) | 0 (0.001) | 0.071 (0.04) | 0.982 (0.963) | 4.183(5.385) |
2.885 (2.194) | |||||||||
2006 | 335(334) | 4.331 (4-763) | 1.015(1.022) | 0.013 (0.013) | 0.796 (0.796) | 0(0) | 0.072 (0.073) | 0.998 (0.997) | 6.567(6.587) |
4.344 (4.768) | |||||||||
2007 | 342(340) | 3.175 (2-396) | 1.158(1.162) | 0.009 (0.009) | 0.713 (0.712) | 0.001 (0.001) | 0.027 (0.027) | 0.979 (0.977) | 4.386(4.412) |
3.194 (2.394) | |||||||||
2008 | 431(426) | 3.350 (2-179) | 1.173(1.222) | 0.008 (0.008) | 0.763 (0.762) | 0(0) | 0.020 (0.021) | 0.965 (0.962) | 3.48(3.521) |
3.357 (2.189) | |||||||||
2009 | 601(589) | 4.672 (4-463) | 1.318(1.4334) | 0.008 (0.008) | 0.789 (0.832) | 0.001 (0.001) | 0.062 (0.059) | 0.962 (0.929) | 4.659(5.603) |
4.744 (4.627) | |||||||||
2010 | 688(677) | 3.526 (2.596) | 1.417(1.721) | 0.005 (0.005) | 0.848 (0.839) | 0.001 (0.002) | 0.024 (0.025) | 0.848 (0.88) | 3.779(5.465) |
3.572 (2.634) | |||||||||
2011 | 886(875) | 3.963(3.090) | 1.161(1.1967) | 0.005 (0.005) | 0.737 (0.734) | 0(0) | 0.027 (0.027) | 0.970 (0.964) | 2.144(2.171) |
3.997 (3.115) | |||||||||
2012 | 1003(975) | 4.838 (4-008) | 1.225(1.359) | 0.005 (0.005) | 0.845 (0.837) | 0.001 (0.001) | 0.024 (0.026) | 0.965 (0.937) | 3.39(4.410) |
4.923 (4.151) | |||||||||
2013 | 1293(1271) | 4.666 (3-758)4.731 (3.815) | 1.319(1.683) | 0.004 (0.004) | 0.845 (0.843) | 0(0) | 0.013 (0.015) | 0.947 (0.932) | 2.398(3.068) |
2014 | 1436(1399) | 4.460 (3-443) | 1.810(2.317) | 0.003 (0.003) | 0.835 (0.832) | 0.001 (0.001) | 0.015 (0.018) | 0.923 (0.889) | 4.526(4.718) |
4.558 (3.576) | |||||||||
2015 | 1745(1704) | 4.824 (3-776) | 3.057(4.485) | 0.003 (0.003) | 0.808 (0.804) | 0.002 (0.005) | 0.021 (0.028) | 0.94(0.917) | 6.246(11.209) |
4.920 (3.870) | |||||||||
2016 | 1742(1693) | 5.009 (4-151) | 4.369(4.363) | 0.003 (0.003) | 0.834 (0.862) | 0.007 (0.008) | 0.014(0.021) | 0.908 (0.861) | 10.964(13.94) |
5.135 (4.384) | |||||||||
2017 | 1790(1746) | 4.633 (3-609) | 4.212(5.971) | 0.003 (0.003) | 0.815 (0.812) | 0.003 (0.017) | 0.020 (0.017) | 0.880 (0.864) | 8.939(17.640) |
4.708 (3.681) | |||||||||
2018 | 2107(2042) | 4.775 (3-280) | 4.564(4.230) | 0.002 (0.002) | 0.841 (0.837) | 0.006 (0.005) | 0.019 (0.020) | 0.846 (0.813) | 13.716(14.447) |
4.891 (3.420) |
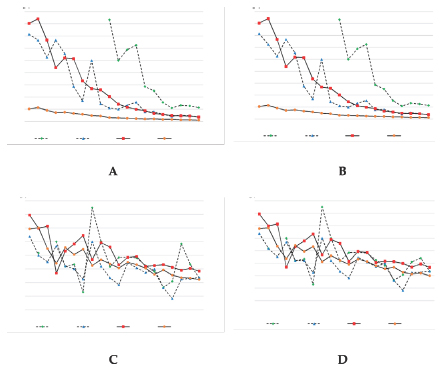
Figure 9:
Clustering coefficients of the Indian and the global ORE networks in WoS and SCOPUS (A-B: author-number-normalized; C-D: mean-degree-normalized)
effective when dealing with highly specific research problems in the short term, the activity could as well run the risk of becoming progressively isolated from mainstream ORE research over time. Many potential opportunities for developing innovative solutions might be missed, and the consequences might become severely restricted. To ameliorate the situation, research policy should prioritize the integration of small-sized collaboration clusters into larger, network-spanning clusters. This might be accomplished even with only a small number of weak couplings between the clusters.
We found substantial evidence that the Indian ORE networks are highly fragmented, containing a large number of disconnected, non-percolating clusters. However, the good news is that some potential bridging links do exist in the networks that hold promise for future development. At this moment, it is unknown what socio-professional factors prevent clusters from being bridged. Possibilities include, for instance, decreased extramural funding, lack of consolidated government policies, intellectual migration, regular retirements, and the dominating control exercised by a few important ORE scholars. We hope that further study based on the ego-networks of researchers will provide more insight into this critical problem.
In general, the ORE network in India is relatively well-clustered, in which the collaboration of three or more researchers is typical. As a result, one researcher who has cooperated with two others has a higher probability of convincing the other two to collaborate as well. When the researchers have significant socio-professional relationships, the likelihood increases. This commonly happens when researchers belong to the same research group in a research institute, or meet and network with other researchers during national or international conferences, or serve on the same editorial boards of journals, or function on the same research evaluation committees. A local dominance of this nature can produce holes in networks. Nevertheless, a certain degree of structural cohesiveness will always remain in any tiny cluster based on a small research group till the time the principal researchers remain active in maintaining the group together. When primary researchers retire from active research or leave the present field to work in other fields of study, connectivity challenges become serious.
To summarize, our findings reveal that current ORE researchers in India are working predominantly in small groups, confining their research work to familiar circles of close collaborators, advisers, or mentors, and are typically hesitant to reach out to others beyond their local groups. As a result, these small clusters miss critical inter-cluster linkages, resulting in highly fragmented networks. Such clusters also cause connectivity problems at the national level (and even at the global level in some cases). The outcome is an inevitable breakdown of network connectedness, which, in practice, leads to a lack of diversity in the exchange of scientific knowledge. Research policy administrators should focus on providing a variety of external incentives to support active multidisciplinary research and promote collaborations in ORE. As additional incentives, the researchers’ governing institutions or governmental research funding agencies should think about inter-institutional, multidisciplinary projects in ORE.[47] Research administrators and policy makers can also strategize to seek ways to increase the establishment of inter-cluster links in order to achieve stronger nationwide connectedness. In this respect, institutional or governmental incentives can be highly advantageous.[49–51]
LIMITATIONS
Our examination of network topologies for ORE research collaboration in India and the world showed a number of intriguing features. We discussed the general policy implications of these features, which may be considered in making policies to boost research collaboration in the country. Despite these benefits, our study has a number of limitations. Addressing them will open up new avenues of research in this area in the future.
The current study is concerned with an assessment of research collaboration patterns based on a comprehensive network perspective. The problem of author name resolution always remained a hurdle. Moreover, the databases we accessed had inconsistencies in the organization and presentation of researcher names with their institutional affiliations, which involved manual processing for ascertaining their correct forms in a number of uncertain cases. The subject area coverage also varied across the databases; therefore, even with the current methods, bias could not be totally removed. Researchers’ institutional ties were eliminated from the current networks which would certainly have reduced the data accuracy somewhat. We address this in a future work.
An indirect assumption used in this study is that, once a researcher joins a collaboration network, they remain linked for the entire duration of the study horizon. Although we did not find any major variations in our analyses, researchers are known to quit networks from time to time. Therefore, it would have been good if we had removed researchers if they had remained unpublished as co-authors for a certain period of time. As such, this is not hard to implement in calculations, but we did not go for it due to our limited window sizes. We were also unable to determine the damaging consequences of local dominance in collaborations by examining the global level. This additional investigation might well have interesting cultural ramifications.[52] In some cases, it has resulted in a distinct lack of heterogeneity in study paths. In the present work, while specializing in the ORE sector, we were unable to separate these select coteries of researchers from a more comprehensive global analysis. This will be undertaken in our next phase of the study.
References
- Reuveny R. Climate change-induced migration and violent conflict. PolitGeogr. 2007;26:656-73. [CrossRef] | [Google Scholar]
- Wang J, Zhou Y, Cooke FL. Low-carbon economy and policy implications:a systematic review and bibliometric analysis. Environ Sci Pollut Res. 2022;29:65432-51. [CrossRef] | [Google Scholar]
- Johnstone N, Haščič I, Popp D. Renewable energy policies and technological innovation:evidence based on patent counts. Environmental and resource economics. 2010;45(1):133-55. [CrossRef] | [Google Scholar]
- Rogelj J, Elzen M, Höhne N, Fransen T, Fekete H, Winkler H, Meinshausen M, et al. Paris Agreement climate proposals need a boost to keep warming well below 2C. Nature. 2016;534(7609):631-9. [CrossRef] | [Google Scholar]
- Pörtner H-O, Roberts DC, Tignor M, Poloczanska ES, Mintenbeck K, Alegría A, et al. IPCC. Climate Change 2022:Impacts, Adaptation and Vulnerability. Contribution of Working Group II to the Sixth Assessment Report of the Intergovernmental Panel on Climate Change. :3056 [CrossRef] | [Google Scholar]
- IEA. Global Energy and CO Status Report 2019, IEA, Paris. 2019 https://www.iea.org/reports/global-energy-co2-status-report-2019
License:CC BY 4.0. [CrossRef] | [Google Scholar] - World Energy and Climate Statistics –Yearbook 2022. Enerdata [CrossRef] | [Google Scholar]
- Chaudhuri SK. Innovation Ecosystem for Sustainable Energy in Low-income Countries of Africa towards the Goal of the Agenda 2030 of the UNEP and the Agenda 2063 of the AU:A Critical Review. Journal of Scientometric Research. 2021;10(3):444-55. [CrossRef] | [Google Scholar]
- Pelc R, Fujita RM. Renewable energy from the ocean. Marine Policy. 2002;26(6):471-9. [CrossRef] | [Google Scholar]
- Arjune V, Singh R, Desai PN, Tripathi K, Pandey N, Desai PN, et al. Scientometric Analysis of the Evolution and Changing Structure in Systems of Innovation Approaches. Journal of Scientometric Research. 2021;10(2):212-24. [CrossRef] | [Google Scholar]
- Ocean Energy Market Size, Share and Trends Analysis Report by Application, Regional Outlook, Competitive Strategies, And Segment Forecasts, 2019 To 2025. [(accessed on 01.12.2022)]. Web reference available on https://www.grandviewresearch.com/industry-analysis/ocean-energy-market/methodology
- United Nations Environment Programme (UNEP). Emissions Gap Report 2022:The Closing Window — Climate crisis calls for rapid transformation of societies. Nairobi. 2022 https://www.unep.org/emissions-gap-report-2022
- Apostu SA, Panait M, Vasile V. The energy transition in Europe—a solution for net zero carbon?. Environ SciPollut Res. 2022;29:71358-79. [CrossRef] | [Google Scholar]
- Blazquez J, Fuentes R, Manzano B. On some economic principles of the energy transition. Energy Policy. 2020;147:111807 [CrossRef] | [Google Scholar]
- Chen L, Li W, Li J, Fu Q, Wang T. Evolution Trend Research of Global Ocean Power Generation Based on a 45-Year Scientometric Analysis. Journal of Marine Science and Engineering. 2021;9(2):218 [CrossRef] | [Google Scholar]
- Adesanya A, Misra S, Maskoliunas R, Damasevicius R. Prospects of ocean-based renewable energy for West Africa’s sustainable energy future. Smart and Sustainable Built Environment. 2021;10(1):37-50. [CrossRef] | [Google Scholar]
- Nguyen PQP, Dong VH. Ocean Energy-A Clean Energy Source. European Journal of Engineering Research and Science. 2019;4(1) [CrossRef] | [Google Scholar]
- Haripriya R, Misra A, Jeyaraj S, Ramakrishnan B. Wave energy assessment for 39 years along the Exclusive Economic Zone (EEZ) of India. Regional Studies in Marine Science. 2021;45 [CrossRef] | [Google Scholar]
- Bozeman B, Corley E. Scientists’ collaboration strategies:Implications for scientific and technical human capital. Research Policy. 2004;33(4):599-616. [CrossRef] | [Google Scholar]
- . Bandwidth and echo:Trust, information, and gossip in social networks. Networks and Markets:Contributions from Economics and Sociology. 2001:30-74. [CrossRef] | [Google Scholar]
- Burt RS. Structural holes and good ideas. American Journal of Sociology. 2004;110(2):349-99. [CrossRef] | [Google Scholar]
- Granovetter MS. The strength of weak ties. American Journal of Sociology. 1973;78(6):1360-80. [CrossRef] | [Google Scholar]
- de Paulo AF, Porto GS. Solar energy technologies and open innovation:A study based on bibliometric and social network analysis. Energy Policy. 2017;108:228-38. [CrossRef] | [Google Scholar]
- García PQ, Ruiz JACR, Sanabria JG. Blue energy and marine spatial planning in Southern Europe. Energy Policy. 2020;140 [CrossRef] | [Google Scholar]
- IRENA. Innovation outlook:Ocean energy technologies, International Renewable, Energy Agency, Abu Dhabi. 2020 https://www.irena.org/-/media/Files/IRENA/Agency/Publication/2020/Dec/IRENA_Innovation_ Outlook_Ocean_Energy_2020. pdf
[CrossRef] | [Google Scholar] - IEA-OES. Annual Report:An Overview of Ocean Energy Activities in. 2021 [CrossRef] | [Google Scholar]
- Chakraborty S, Dwivedi P, Chatterjee SK, Gupta R. Factors to Promote Ocean Energy in India. Energy Policy. 2021:159 [CrossRef] | [Google Scholar]
- Ravindran M, Abraham R. The Indian 1 MW demonstration OTEC plant and the development activities. InOCEANS’02 MTS/IEEE. 2002;3:1622-1628. [CrossRef] | [Google Scholar]
- Ocean Thermal Energy Association. OTEC Powered Desalination Plant at Kavaratti. 2020 [Accessed on January 15, 2022]. http://www.ocean-thermal.org/otec-powered-desalination-plant-at-kavaratti
- Ma D, Yongsheng Y, Zhang Y. Dynamics of waste-to-energy incineration R&D collaboration networks:a social network analysis based on patent data. Geosystem Engineering. 2006;20(2):1-12. [CrossRef] | [Google Scholar]
- Chen Y, Fang S. Mapping the evolving patterns of patent assignees’ collaboration networks and identifying the collaboration potential. Scientometrics. 2014;101(2):1215-31. [CrossRef] | [Google Scholar]
- Yanqing H, Yuchao N, Yang H, Cheng J, Ying F, Zengru D, et al. Measuring the significance of community structure in complex networks. Physical. 2010;82(2):66-106. [CrossRef] | [Google Scholar]
- Chen SH, Huang MH, Chen DZ. Identifying and visualizing technology evolution:a case study of smart grid technology. Technological Forecasting and Social Change. 2012;79(6):1099-110. [CrossRef] | [Google Scholar]
- Rahimi S, Soheili F, Nia YA. Social Influence, Research Productivity and Performance in the Social Network Co-authorship:A Structural Equation Modelling. Journal of Scientometric Research. 2020;9(3):326-34. [CrossRef] | [Google Scholar]
- Batagelj V, Mrvar A, Zaveršnik M. 2012 http://pajek.imfm.si/doku.php
[CrossRef] | [Google Scholar] - Newman ME. The structure of scientific collaboration networks. Proceedings of the National Academy of Sciences. 2001a;98(2):404-9. [CrossRef] | [Google Scholar]
- Milojević S. Accuracy of simple, initials-based methods for author name disambiguation. Journal of Informetrics. 2013;7(4):767-73. [CrossRef] | [Google Scholar]
- Barabási AL, Jeong H, Ravasz R, Ne´da Z, Vicsek T, Schubert A, et al. On the topology of the scientific collaboration networks. Physica. 2002;311:590-614. [CrossRef] | [Google Scholar]
- Wasserman S. Faust K Social network analysis. 1994 [CrossRef] | [Google Scholar]
- Milgram S. The small-world problem. Psychology Today. 1967;1(1):61-7. [CrossRef] | [Google Scholar]
- Watts DJ, Strogatz SH. Collective dynamics of ‘small-world’ networks. Nature. 1998;393:440-2. [CrossRef] | [Google Scholar]
- Cowan R, Jonard N. Network structure and the diffusion of knowledge. Journal of Economic Dynamics and Control. 2004;28:1557-75. [CrossRef] | [Google Scholar]
- Albert R, Barabási AL. Statistical mechanics of complex networks. Reviews of Modern Physics. 2002;74(1):47-97. [CrossRef] | [Google Scholar]
- Newman ME. Scientific collaboration networks. I. Network construction and fundamental results. Physical Review E. 2001b;64(016131) [CrossRef] | [Google Scholar]
- Newman ME. The structure and function of complex networks. SIAM Review. 2003;45(2):167-256. [CrossRef] | [Google Scholar]
- Ghosh J, Kshitij A. An integrated examination of collaboration co-authorship networks through structural cohesion, holes, hierarchy, and percolating clusters. Journal of the American Society for Information Science and Technology. 2014;65(8):1639-61. [CrossRef] | [Google Scholar]
- Kshitij A, Ghosh J, Gupta BM. Embedded information structures and functions of co-authorship networks:evidence from cancer research collaboration in India. Scientometric. 2015;102:285-306. [CrossRef] | [Google Scholar]
- Sgobbi A, Simões SG, Magagna D, Nijs W. Assessing the impacts of technology improvements on the deployment of marine energy in Europe with an energy system perspective. Renewable Energy. 2016;89:515-25. [CrossRef] | [Google Scholar]
- Hara N, Solomon P, Kim SL, Sonnenwald DH. An emerging view of scientific collaboration:Scientists’ perspectives on collaboration and factors that impact collaboration. Journal of the American Society for Information Science and Technology. 2003;54(10):952-65. [CrossRef] | [Google Scholar]
- Birnholtz J, Guha S, Yuan YC, Gay G, Heller C. Cross-campus collaboration:A scientometric and network case study of publication activity across two campuses of a single institution. Journal of the American Society for Information Science and Technology. 2013;64(1):162-72. [CrossRef] | [Google Scholar]
- Shrum W, Genuth J, Chompalov I. Structures of Scientific Collaboration. 2007 [CrossRef] | [Google Scholar]
- BBernard HR, Killworth PD, Evans MJ, McCarty C, Shelley GA. Studying social relations cross-culturally. Ethnology. 1988;27(2):155-79. [CrossRef] | [Google Scholar]