Contents
ABSTRACT
ABSTRACT
This study examines the intellectual structure and research trends at the intersection of big data, accounting, and finance using a bibliometric analysis of 295 Scopus-indexed papers from 2013 to 2023. The study tackles the need to understand collaboration networks and topic evolutions in this dynamic environment. We discovered dominating research clusters, essential contributors, and topic trajectories before and after COVID-19 using co-authorship and keyword co-occurrence analyses. The methodology uses tools like VOSviewer and Biblioshiny to map collaboration patterns and thematic map concentrations. The findings show that international collaboration, notably amongst scholars from China, the United States, and the United Kingdom, is critical to advance the discipline. Traditional subjects such as “big data”, “finance”, and “accounting” dominate keyword research, while emergent topics such as “artificial intelligence”, “cloud computing”, and “risk assessment” represent technological improvements. The COVID-19 pandemic sparked new research avenues, introducing concepts like “neural networks” and “sustainable development goals”. The findings emphasise big data’s disruptive impact on financial and accounting procedures and the need for future studies to address regulatory, ethical, and transdisciplinary issues. This study adds to the literature by providing a complete analysis of big data research trends and a road map for scholars and practitioners to navigate the changing academic and industrial settings.
INTRODUCTION
Big data has rapidly gained prominence in the fields of accounting and finance.[1] In finance, big data pertains to extensive datasets that are significant in absolute or comparative terms. It’s more than just sheer volume; it also comprises many variables in relation to the sample size, frequently analysed using machine learning techniques. Moreover, big data captures economic activities in conventional and unstructured formats like text, images, and audio. This versatility enables it to explore research areas that traditional data sets might overlook.
However, the term “big data” remains somewhat nebulous. Nissim[2] suggests it’s a catch-all phrase encompassing everything from vast data collections to sophisticated analytical techniques for discerning patterns. The European Commission describes big data as enormous volumes of varied data from diverse origins, whether humans, machines, or sensors1. This definition could include anything from climate data and satellite imagery to transaction records and GPS signals. Big data might also comprise personal data, encompassing individual-specific information, from names and photographs to medical records and IP addresses. Oracle characterises big data by its “Three Vs”: volume, variety, and velocity. Unlike the structured nature of traditional data, big data introduces unstructured formats, demanding advanced processing. Its magnitude can span from terabytes to petabytes, and its swift accumulation rate poses challenges for organisations regarding assimilation and practical application.
Previous research has highlighted several areas of big data in accounting and finance that deserve further exploration, such as risk and security, data visualisation, predictive analytics and data quality.[3] These domains offer promising avenues for research that could refine industry practices and foster interdisciplinary partnerships. While accounting and finance are accustomed to handling vast data volumes, the actual deployment of big data and its analytical methodologies is still budding. This inclination highlights a discernible gap between big data’s potential and its present-day application in these sectors.
The extensive literature on big data in accounting and finance has prompted numerous scholars to review the existing body of work. However, many such reviews have been narrowly focused on specific facets of big data rather than its holistic application in accounting and finance. For instance, the current body of literature has been segmented into particular research areas, such as accounting and auditing,[4,5] finance,[6] sustainable supply chain finance,[7] and accounting.[8] Given that recent studies, notably by Nobanee[6] and Varma et al.,[8] have only conducted bibliometric analyses in 2021, we recognise an urgent need for a comprehensive review in accounting and finance, which has inspired this paper.
In this paper, we set out a tripartite objective. Firstly, we examine the most influential publications, authors, and institutions in big data research within accounting and finance. Next, we scrutinise patterns of co-authorship and the co-occurrence of both author-specific and index keywords. Finally, we turn our attention to the prevailing topics and construct a thematic map based on keywords, aiming to trace the progression of these keywords over time, emphasising the periods before and during the COVID-19 pandemic. To our understanding, our research is pioneering in examining keyword and thematic evolutions across different phases of the COVID-19 pandemic. This unique perspective is our most significant contribution to the burgeoning literature on big data within accounting and finance.
The paper is organised as follows: Section 2 discusses the literature pertaining to big data in accounting and finance. Section 3 delineates the bibliometric data and methodologies employed in this investigation. Section 4 highlights the results and discussion, while Section 5 offers the conclusion of the study.
LITERATURE REVIEW
With numerous potentials for practice and research, big data is generally regarded as a disruptive factor in accounting and finance. Six under-researched areas of big data in accounting and finance were found by Cockcroft and Russell,[3] including risk and security, data visualisation, predictive analytics, and data management. Warren et al.[9] showed how big data might improve the quality and relevance of accounting information, hence fostering more openness and better decision-making among relevant parties. Moreover, Younis[10] emphasised the value of big data methods in auditing procedures and financial forecasting models and their possibilities in stock market prediction and financial failure avoidance.
Big data clearly affects accounting processes, according to research. Abueid and Hakami[11] underlined the great importance of big data analysis in accounting and finance and said that illuminating academics and researchers in this area depends on offering seminars and training. Gepp et al.[5] found an increasing trend towards using big data in accounting processes in their analysis of the existing advancements and projected possibilities of big data approaches in auditing research and practice. Ren,[12] who mentioned its power to increase efficiency, risk control capabilities, and the scope of financial management, also highlighted the tremendous impact of big data on financial management. Furthermore, thanks to big data technology, the evolution of corporate financial information provides tools for the junction of business and finance, as Xu and Zhou[13] note.
Integration of big data has taken the front stage in accounting. Many research have looked at how big data might affect accounting procedures. Concerning the Sustainable Development Goals of the United Nations, Esteban et al.[14] performed a bibliometric study on accounting, big data, and global development. This paper thoroughly evaluates the academic scene in this field. Arnaboldi et al.[15] investigated accountants’ opinions on big data, stressing the benefits and difficulties it presents related to social media and responsibility. Moreover, according to Akbulut and Kaya,[16] big data analytics can change accounting and financial reporting. They showed how well big data analytics might capture real-time cause and correlation processes, therefore transforming conventional financial reporting methods.
Focussing on internal consumption and user-centric visualisation methods, Coyne et al.[17] examined how accountants engage with big data information governance in the information governance framework. This paper highlights how accounting techniques are evolving in reaction to the influx of big data. Dagilienė and Klovienė[18] looked at the reasons for using analytics and big data in external audits, shedding light on companies’ strategies to welcome these technologies for auditing goals.
Big data has revolutionised finance and opened fresh opportunities for investment strategies, technical development, financial management, and technological change.[6] Big data is being used increasingly in finance, as seen by the increasing number of bibliometric evaluations focused on big data research in finance.[19] These research show how important big data is for changing methods of financial management and decision-making procedures.
By using big data analytics, financial institutions can acquire a competitive advantage, lower risks, and investigate fresh development and innovation opportunities in the evolving financial scene of today. By clarifying the trends, challenges, and possibilities connected to big data integration in finance, these bibliometric studies help to prepare the ground for the next studies and sectoral developments. Integrating big data into accounting and finance will likely revolutionise current practices by offering improved insights, efficiency, and decision-making ability. Maintaining competitiveness and satisfying the aspirations of a data-driven financial sector depends on using big data technologies as the industry develops.
Big data in accounting and finance has been the subject of bibliometric study in several works. Tseng et al.[7] bibliometricly investigated Sustainable Supply Chain Financing (SSCF) using big data analytics. Their studies underlined the essential functions of blockchain, cash flow problems, reverse factoring, risk assessment, and the triple bottom line in SSCF. To address field uncertainty, they used qualitative and quantitative approaches. Including big data in accounting systems has also drawn academic interest. Focussing on the interaction between big data and accounting, Varma et al.[8] performed a bibliometric analysis showing how big data technologies change accounting methods.
Murthy and Geerts[20] also examined the relationship between accounting information systems and big data. With an eye towards better integrating structured external data with transactional processing elements, their study presented an ontology-based model that links big data aspects to accounting information systems. Agustí and Orta-Pérez[4] conducted a bibliometric study on utilising big data and artificial intelligence, providing insights into how these technologies are being used inside auditing and accounting firms in the context of auditing and accounting.
Likewise, bibliometric research has investigated how big data might be used in the financial industry. For example, Nobanee[6] primarily examined big data in financial matters, stressing the improvement of cooperation and information sharing through aggregated bibliographic databases that visualised research results. Emphasising the need for data-driven strategies in enhancing operational efficiencies and strategic financial decision-making, Mishra et al.[21] conducted a comprehensive research and bibliometric analysis of the literature on big data and supply chain management. Big data applications in finance enhance decision-making procedures and offer insights into financial technology, supply chain management, and risk assessment.
BIBLIOMETRIC DATA AND METHODS
Bradford[22] was the first to introduce bibliometric analysis, subsequently developed by Price.[23] The primary metric used to evaluate and assess scholarly articles is citation counts, which Price introduced in various bibliometric methods. Kim and McMillan[24] elaborated on this process, emphasising the article as the fundamental unit of analysis. The influential aspects of big data within accounting and finance literature can be highlighted through bibliometric citation analysis. This method is particularly adept at identifying the connections between articles and various authors.
Elsevier Scopus, a renowned repository for its comprehensive collection of peer-reviewed articles, serves as our source for bibliometric data. We implemented the search query TITLE (“big data” AND accounting OR finance) to extract relevant papers within paper titles. 295 records were obtained during this inquiry, conducted on 24 August 2023. These records were subsequently incorporated into our bibliometric analysis. Figure A1 provides a comprehensive overview of our search methodology, outlining our steps to retrieve pertinent papers from the Scopus database.
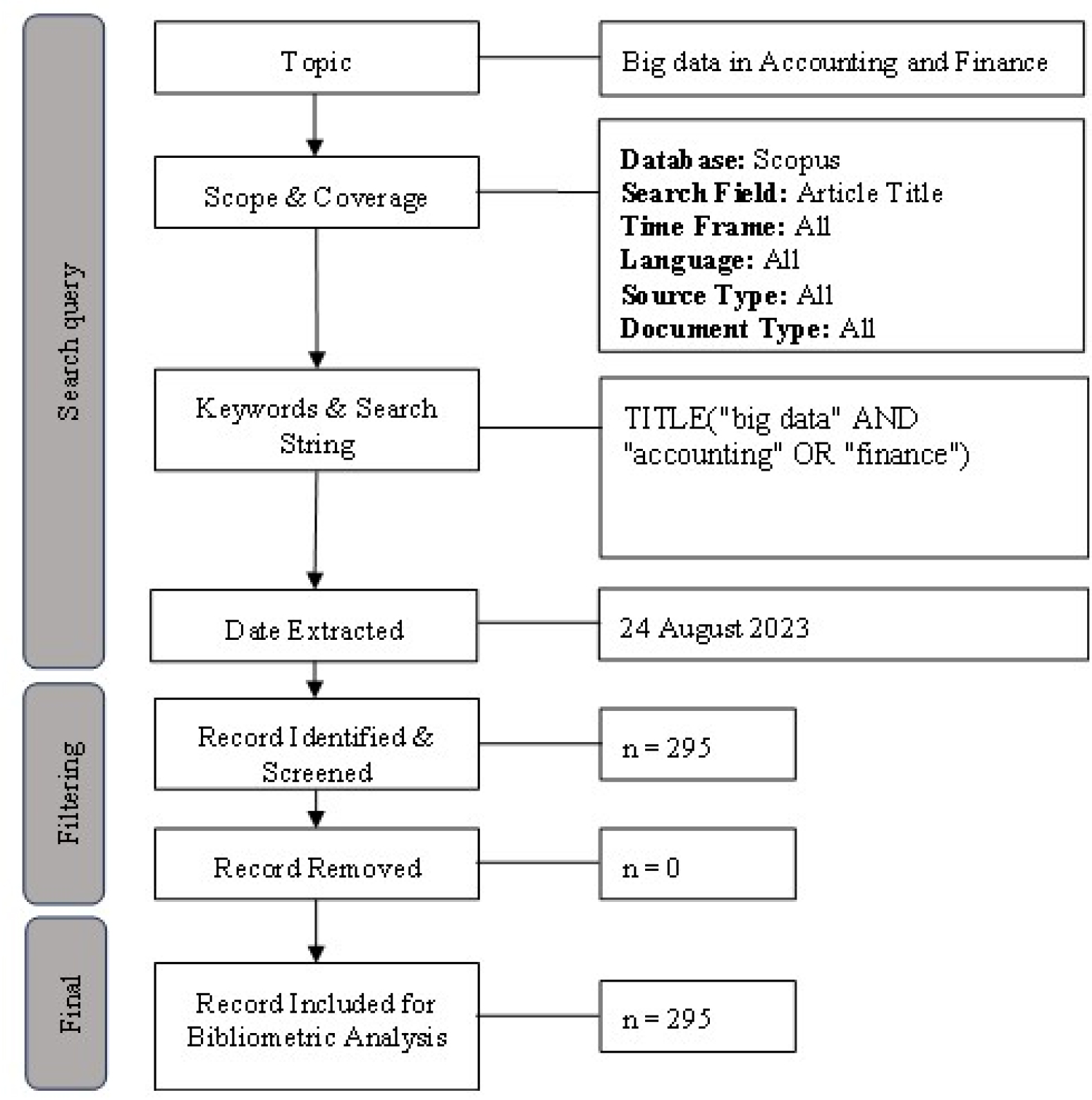
Figure A1:
Bibliometric search query process from Scopus database. Note: This illustration shows the search query process in the Scopus database.
Bibliometric search query process
A systematic approach was employed to identify articles related to “big data” in Accounting and Finance from the Scopus database during this study’s bibliometric search query procedure. The following is a comprehensive account of the procedure:
- Database: We employed the Scopus database, a comprehensive multidisciplinary database that indexes scientific literature.
- Search Field: The search was conducted with a specific concentration on the titles of articles pertinent to the research topic, specifically in the Article Title field.
- Time Frame: The search was comprehensive, encompassing articles from all periods, suggesting no limitations on the publication dates.
- Language: The search encompassed articles in all languages, guaranteeing comprehensive subject matter coverage.
- Source Type: The search was comprehensive, encompassing articles from various publications, including conference proceedings and periodicals.
- Document Type: The search encompassed all document types, indicating that various documents, including research articles and reviews, were considered.
- Search Query: The search query that was employed was as follows: TITLE(“big data” AND “accounting” OR “finance”). This query concentrated on articles that featured the keywords “big data” in conjunction with either “accounting” or “finance.”
- Record Identification and Screening: The search query yielded 295 documents. Subsequently, these records were evaluated for their relevance to the “big data” field in Accounting and Finance.
- Topic Scope and Coverage: Records were filtered to ensure they followed the research concentration on big data in Accounting and Finance.
- Record Inclusion for Bibliometric Analysis: Following the screening and filtering process, no document was excluded, and 295 records were included for bibliometric analysis.
- Date Extracted: The search results were extracted on 24 August, 2023.
Our objective was to identify pertinent articles for bibliometric analysis on the subject of “big data” in Accounting and Finance by adhering to this systematic search query procedure. The search query’s criteria were instrumental in reducing the number of search results and concentrating on articles that were most relevant to the research topic.
Scopus as the source of bibliometric analysis
Scopus is a reliable source for bibliometric analysis because of its extensive coverage, robust search capabilities, and high-quality data. Scopus is employed for bibliometric investigations because it allows for precise analysis of scientific research.[25–27] Scopus provides access to high-quality bibliometric data for academic research, including quantitative science.[28] It is an ideal subject for bibliometric research due to its financing data, citation accuracy, and content coverage.[29]
Due to its extensive scientific literature coverage, Scopus is an exceptional tool for conducting bibliometric assessments and analyses across various domains.[30] The search capabilities and user-friendly interface facilitate the identification of authors, publication years, abstracts, institutions, and countries for bibliometric studies.[31] Scopus is the preferred tool for bibliometric analysis due to its more comprehensive article index.[32] Scholars can analyse bibliometric data with the help of Scopus’s bibliometric software and bibliographic data.[33] The primary international scientific database is regarded as reliable for bibliometric studies.[34] Scopus is the preferred database for bibliometric studies in medical, social, and computer science because of its comprehensive citation coverage and database structure.[35] To reiterate, Scopus is a reliable source for bibliometric analysis across various disciplines due to its extensive coverage, data accuracy, and usability. Scopus’s superior data assists bibliometric researchers in obtaining insights and validating their findings.
Limiting the bibliometric search to the article title
Article titles are often the subject of bibliometric analysis for various reasons supported by research. Titles serve as a basic summary of the study’s main findings and support this methodology.[36] By concentrating on article titles to identify themes and subjects, researchers can swiftly locate pertinent articles for bibliometric analyses.[37] Restricting the search to article titles makes data collection easier and optimises search engines. Titles are carefully crafted to capture the essence of the research, and they might help locate related articles within a specific field of study.[38] Researchers can quickly sort through voluminous literature using this focused approach and get pertinent information for bibliometric analysis.[39]
Researchers can refine their search by concentrating on article titles, which may produce more precise and pertinent results. Titles are a valuable place to look for papers that satisfy research aims since they contain a wealth of phrases that summarise the core ideas of the research.[40] With this targeted search, researchers can improve the quality and relevance of bibliometric analyses by avoiding irrelevant or needless articles.[41] Bibliometric analysis that solely looks up article titles can increase effectiveness, accuracy, and relevance. By concentrating on titles, researchers can expedite data collection, identify important publications quickly, and ensure that retrieved articles align with research objectives-all contribute to a more relevant and focused bibliometric analysis.
Data-cleaning process
We used the proprietary program Bibliomagika to clean the Scopus data after the extraction comprehensively.[42] First, we separated the data from Scopus by using Microsoft Excel’s “Separate Value” macro to separate the full names and affiliations of the authors. Thanks to this process, we could identify every author-even those whose names were missing. We manually looked for the names of any missing or unreachable authors inside the published document. Author affiliations were occasionally missing, necessitating a search of the published papers, publisher websites, or the authors’ Scopus or Google Scholar profiles. The most time-consuming aspect of the procedure turned out to be filling in these blanks. Now and again, Scopus neglected to update this data in its database; thus, to move forward with bibliometric analysis, we had to finish these details.
After completing any missing data, we proceeded to standardise the information. Authors frequently used initials instead of full names, occasionally omitted middle names, and used various first names. Moreover, discrepancies in affiliations or addresses in the published documents that Scopus had recorded required to be aligned. We used OpenRefine to address these inconsistencies and guarantee reliable bibliometric analysis.[43] A useful tool for harmonising and standardising bibliometric data from various sources is OpenRefine. Researchers can improve the quality and usefulness of bibliometric data for analysis by using OpenRefine to clean, convert, and reconcile it.[44]
Researchers frequently encounter data from many sources in bibliometric analysis, each with its own formats and patterns. To facilitate effective data harmonisation, OpenRefine provides features to standardise data fields, fix mistakes, eliminate duplication, and harmonise data formats.[45] The harmonisation process guarantees data consistency and preparedness for analysis, resulting in more accurate and reliable research findings. Researchers can save time and effort while preserving the integrity and coherence of the bibliometric data by using OpenRefine to streamline the data harmonisation process.[46]
Bibliometric thematic map analysis
We then performed cartographic analysis, concentrating on co-occurrence and co-authorship of keywords. VOSviewer is used for this analysis.[47] Furthermore, Biblioshiny is employed for a co-word thematic map analysis.[48]
Cartographic and thematic map analyses study and interpret maps to express particular thematic content and extract spatial information from maps. While thematic map analysis looks at the theme content or specific subject matter portrayed on maps, cartographic analysis concentrates on the visual depiction of geographic data. Through the study of spatial relationships, design features, symbols, and colours, cartographic analysis allows academics to understand the visual communication of geographic information. This study evaluates the map’s scale, projection, legend, and other cartographic elements to identify spatial patterns and relationships.[49] Understanding the creation of maps and the visual representation of geographical information is essential to cartographic analysis.
On the other hand, thematic map analysis looks at maps intended to illustrate particular themes or subjects, such as population distribution, land use, or natural resources. Thematic maps contain thematic data and provide significant information about a topic using visual characteristics such as colour, size, and pattern.[50] Scholars scrutinise thematic maps to derive meaning, detect geographical trends, and arrive at well-informed conclusions about the subject matter.
RESULTS AND DISCUSSION
Table 1 presents the primary data from our dataset, comprising 295 publications from 2013 to 2023, contributed by 675 authors. Notably, publications from 2015 received the highest number of citations. In our attempt to identify the ten most influential publications, we categorised them based on a) number of publications, b) total citations, and c) average citations per cited publication. These findings are detailed in Table 2.
Main information |
Year |
Total publications |
Total citations |
|
---|---|---|---|---|
Publication years |
2013 – 2023 |
2013 |
1 |
1 |
Total publications |
295 |
2014 |
5 |
228 |
Citable year |
11 |
2015 |
10 |
729 |
Number of contributing authors |
675 |
2016 |
10 |
155 |
Number of single-authored papers |
114 |
2017 |
12 |
544 |
Number of multiple-authored papers |
181 |
2018 |
14 |
245 |
Number of cited papers |
161 |
2019 |
23 |
292 |
Total citations |
2956 |
2020 |
43 |
244 |
Citation per paper |
9.92 |
2021 |
68 |
325 |
Citation per cited paper |
18.36 |
2022 |
80 |
170 |
Citation per year |
295.60 |
2023 |
29 |
23 |
Citation per author |
4.38 |
Grand total |
295 |
2956 |
Author per paper |
2.27 |
|||
Citation sum within h-core |
2698 |
|||
h-index |
27 |
|||
g-index |
51 |
|||
m-index |
2.45 |
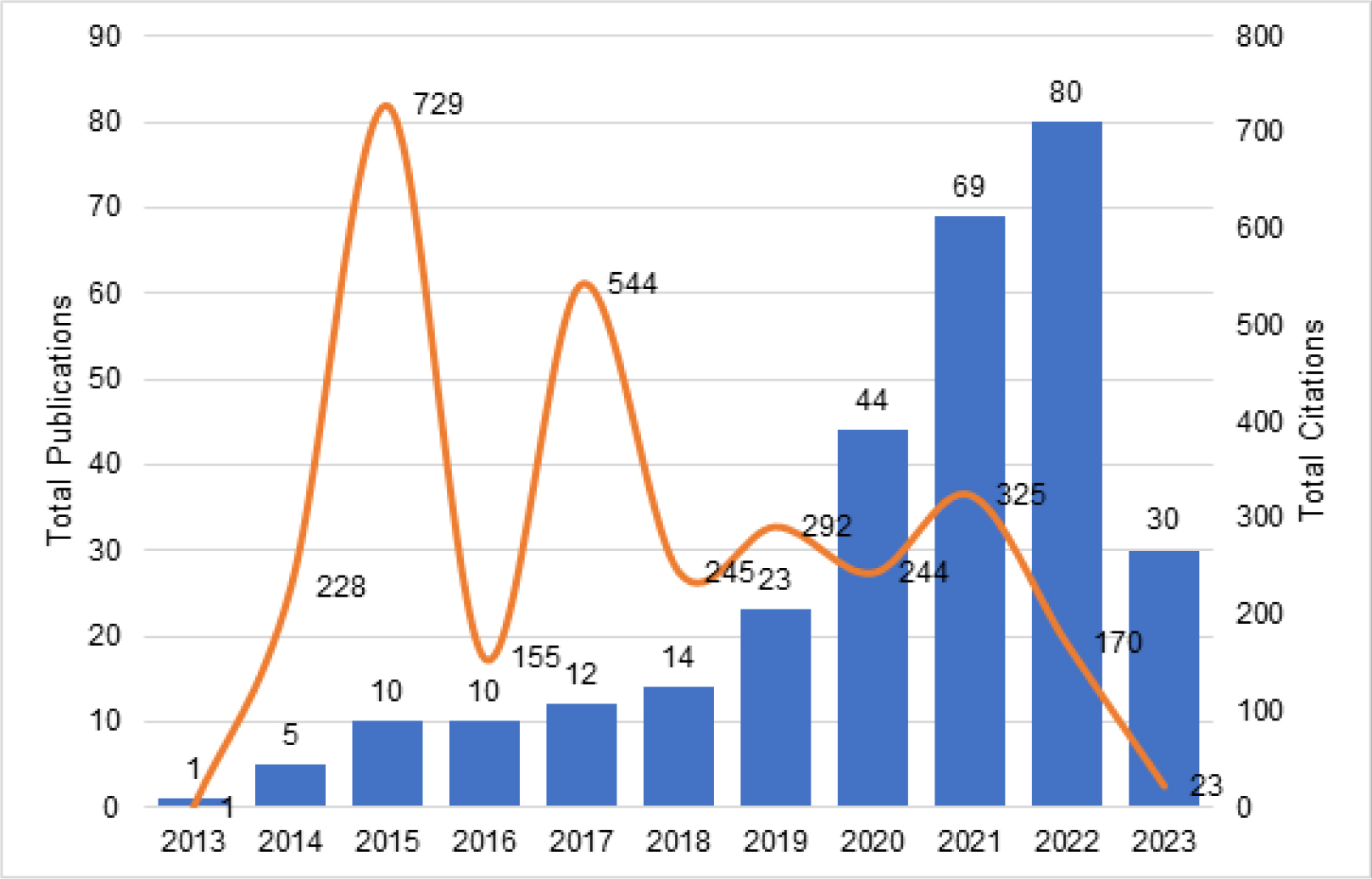
A. By number of Publications (TP) |
||||||||||
Source title |
TP |
NCA |
NCP |
TC |
C/P |
C/CP |
h-index |
g-index |
m-index |
|
1 |
ACM International Conference Proceeding Series |
22 |
57 |
6 |
25 |
1.14 |
4.17 |
3 |
4 |
0.60 |
2 |
Journal of Physics: Conference Series |
20 |
33 |
14 |
34 |
1.70 |
2.43 |
3 |
4 |
0.60 |
3 |
Advances in Intelligent Systems and Computing |
11 |
16 |
3 |
6 |
0.55 |
2 |
2 |
2 |
0.33 |
4 |
Lecture Notes on Data Engineering and Communications Technologies |
11 |
15 |
1 |
1 |
0.09 |
1 |
1 |
1 |
0.50 |
5 |
Lecture Notes in Electrical Engineering |
8 |
14 |
0 |
0 |
0 |
0 |
0 |
0 |
0.00 |
6 |
Wireless Communications and Mobile Computing |
8 |
13 |
5 |
15 |
1.88 |
3 |
2 |
3 |
0.67 |
7 |
IOP Conference Series: Materials Science and Engineering |
6 |
18 |
4 |
5 |
0.83 |
1.25 |
1 |
1 |
0.17 |
8 |
E3S Web of Conferences |
6 |
8 |
2 |
3 |
0.50 |
1.5 |
1 |
1 |
0.25 |
9 |
Accounting Horizons |
5 |
12 |
5 |
665 |
133 |
133 |
5 |
5 |
0.56 |
10 |
Lecture Notes of the Institute for Computer Sciences, Social-Informatics and Telecommunications Engineering |
4 |
4 |
2 |
3 |
0.75 |
1.5 |
1 |
1 |
0.33 |
B. By Total Citations (TC) |
||||||||||
Source title |
TP |
NCA |
NCP |
TC |
C/P |
C/CP |
h-index |
g-index |
m-index |
|
1 |
Accounting Horizons |
5 |
12 |
5 |
665 |
133 |
133 |
5 |
5 |
0.56 |
2 |
Accounting and Business Research |
2 |
3 |
2 |
223 |
112 |
112 |
2 |
2 |
0.20 |
3 |
Journal of Information Systems |
3 |
8 |
3 |
219 |
73 |
73 |
3 |
3 |
0.43 |
4 |
Journal of Accounting Education |
3 |
8 |
3 |
160 |
53.3 |
53.3 |
2 |
3 |
0.29 |
5 |
Accounting, Auditing and Accountability Journal |
1 |
3 |
1 |
131 |
131 |
131 |
1 |
1 |
0.14 |
6 |
Emerging Markets Finance and Trade |
3 |
11 |
2 |
117 |
39 |
58.5 |
2 |
3 |
0.33 |
7 |
Journal of Monetary Economics |
2 |
4 |
2 |
88 |
44 |
44 |
2 |
2 |
0.33 |
8 |
Journal of Big Data |
1 |
3 |
1 |
79 |
79 |
79 |
1 |
1 |
0.25 |
9 |
Annals of Operations Research |
2 |
10 |
1 |
73 |
36.5 |
73 |
1 |
2 |
0.50 |
10 |
Australian Accounting Review |
1 |
2 |
1 |
71 |
71 |
71 |
1 |
1 |
0.17 |
C. By number of Citations per Cited Publications (C/CP) |
||||||||||
Source title |
TP |
NCA |
NCP |
TC |
C/P |
C/CP |
h-index |
g-index |
m-index |
|
1 |
Accounting Horizons |
5 |
12 |
5 |
665 |
133 |
133 |
5 |
5 |
0.56 |
2 |
Accounting, Auditing and Accountability Journal |
1 |
3 |
1 |
131 |
131 |
131 |
1 |
1 |
0.14 |
3 |
Accounting and Business Research |
2 |
3 |
2 |
223 |
112 |
112 |
2 |
2 |
0.20 |
4 |
Journal of Big Data |
1 |
3 |
1 |
79 |
79 |
79 |
1 |
1 |
0.25 |
5 |
Journal of Information Systems |
3 |
8 |
3 |
219 |
73 |
73 |
3 |
3 |
0.43 |
6 |
Annals of Operations Research |
2 |
10 |
1 |
73 |
36.5 |
73 |
1 |
2 |
0.50 |
7 |
Australian Accounting Review |
1 |
2 |
1 |
71 |
71 |
71 |
1 |
1 |
0.17 |
8 |
Emerging Markets Finance and Trade |
3 |
11 |
2 |
117 |
39 |
58.5 |
2 |
3 |
0.33 |
9 |
Journal of Accounting Education |
3 |
8 |
3 |
160 |
53.3 |
53.3 |
2 |
3 |
0.29 |
10 |
International Journal of Production Economics |
1 |
4 |
1 |
53 |
53 |
53 |
1 |
1 |
0.33 |
Conference proceedings, including the ACM International Conference Proceeding Series, Journal of Physic Conference Series, and Advances in Intelligent Systems and Computing, overwhelmingly dominate the top publications by volume. However, Accounting Horizons stands out as the sole journal publication in this category, ranking ninth. Intriguingly, when assessing total and average citations per cited publication, Accounting Horizons emerges as the frontrunner. For both these metrics, journals occupy most of the top ten spots. Specifically, the journals leading in total citations are Accounting Horizons, Accounting and Business Research, and Journal of Information Systems. On the other hand, in terms of average citations per cited publication, Accounting Horizons, Accounting, Auditing and Accountability Journal, and Accounting and Business Research take the lead. This result suggests that journals garner more academic citations while conference proceedings frequently publish on big data in accounting and finance.
Table 3 investigates the trend of single author versus co-authored publications. Of the 295 publications, 114 are solo authored, while 181 have multiple authors. As a general trend, publications with multiple authors have been more prevalent. Table 4 highlights the most influential papers based on total citations and average citations per citable year. The top three papers, all centred on big data and accounting, are: 1) “Big Data in Accounting: An Overview”;[51] Accounting Horizons); 2) “Digitisation, Big Data and the Transformation of Accounting Information”;[52] Accounting and Business Research); and 3) “How Big Data Will Change Accounting”;[9] Accounting Horizons). The leading paper on big data in finance is “Big Data in Finance and the Growth of Large Firms” by Begenau et al.;[53] Journal of Monetary Economics. Evidently, the most influential work on big data is predominantly focused on accounting rather than finance.
No of author(s) per document |
Single-vs multiple-authors |
||||
---|---|---|---|---|---|
No of author(s) |
Frequency |
Year |
Single |
Multiple |
Total |
1 |
114 |
2013 |
. |
1 |
1 |
2 |
72 |
2014 |
1 |
4 |
5 |
3 |
54 |
2015 |
. |
10 |
10 |
4 |
32 |
2016 |
5 |
5 |
10 |
5 |
14 |
2017 |
4 |
8 |
12 |
6 |
7 |
2018 |
4 |
10 |
14 |
7 |
1 |
2019 |
8 |
15 |
23 |
8 |
1 |
2020 |
21 |
22 |
43 |
Grand total |
295 |
2021 |
25 |
43 |
68 |
2022 |
35 |
45 |
80 |
||
2023 |
11 |
18 |
29 |
||
Grand Total |
114 |
181 |
295 |
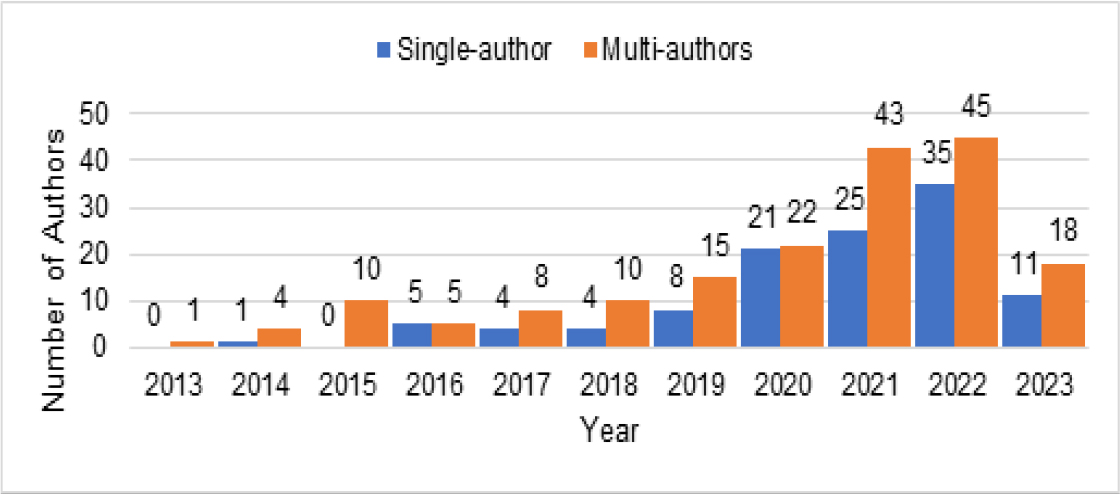
Sl.No. |
Author(s) |
Paper title |
Journal |
TC |
C/Y |
---|---|---|---|---|---|
1 |
Vasarhelyi M.A.; Kogan A.; Tuttle B.M. (2015) |
Big data in accounting: An overview |
Accounting Horizons |
260 |
28.89 |
2 |
Bhimani A.; Willcocks L. (2014) |
Digitisation, Big Data and the transformation of accounting information |
Accounting and Business Research |
206 |
20.60 |
3 |
Warren J.D., Jr.; Moffitt K.C.; Byrnes P. (2015) |
How big data will change accounting |
Accounting Horizons |
201 |
22.33 |
4 |
Richins G.; Stapleton A.; Stratopoulos T.C.; Wong C. (2017) |
Big data analytics: Opportunity or threat for the accounting profession? |
Journal of Information Systems |
135 |
19.29 |
5 |
Arnaboldi M.; Busco C.; Cuganesan S. (2017) |
Accounting, accountability, social media and big data: revolution or hype? |
Accounting, Auditing and Accountability Journal |
131 |
18.71 |
6 |
Krahel J.P.; Titera W.R. (2015) |
Consequences of big data and formalisation on accounting and auditing standards |
Accounting Horizons |
120 |
13.33 |
7 |
Sledgianowski D.; Gomaa M.; Tan C. (2017) |
Toward integration of Big Data, technology and information systems competencies into the accounting curriculum |
Journal of Accounting Education |
95 |
13.57 |
8 |
He P.; Niu H.; Sun Z.; Li T. (2020) |
Accounting Index of COVID-19 Impact on Chinese Industries: A Case Study Using Big Data Portrait Analysis |
Emerging Markets Finance and Trade |
86 |
21.50 |
9 |
Begenau J.; Farboodi M.; Veldkamp L. (2018) |
Big data in finance and the growth of large firms |
Journal of Monetary Economics |
85 |
14.17 |
10 |
Hasan M.M.; Popp J.; Oláh J. (2020) |
Current landscape and influence of big data on finance |
Journal of Big Data |
79 |
19.75 |
The most well-known papers in Table 4 show how much study and interest incorporating big data into accounting and finance processes generate. Emphasising big data’s transforming power in redefining accounting procedures, Vasarhelyi et al.[51] offer an overview of its impact on accounting, stressing its interaction with traditional data sources and its influence on audit judgement and behavioural research. Bhimani and Willcocks[52] challenge conventional accounting classification and highlight the evolving terrain of accounting information in the digital era by exploring how digitisation and big data modify accounting data. Warren et al.[9] also emphasise how, by raising the quality and relevance of accounting data, big data would change accounting processes-especially those in financial accounting. Using big data in accounting can help to improve openness and stakeholder decision-making procedures.
Emphasising structured and unstructured data analysis, Richins et al.[54] also offer a conceptual framework for big data analytics in accounting. Emphasising the advantages and drawbacks of big data in accounting procedures, this paradigm provides insights into exploratory study and problem-solving. Emphasising the transforming power of these technologies in decision-making processes and governance structures, Arnaboldi et al.[15] also look at the convergence of accounting, responsibility, social media, and big data. They also classify study projects and point up possible future directions for inquiry in the evolving terrain of accounting practices. Seeking to raise the value and relevance of the accounting profession, Krahel and Titera[55] advocate a change in accounting and auditing rules to give data analysis first priority above presentation. This shift in focus on data operations and analysis could empower customers and increase capital market efficiency at the same time.
Furthermore, underlined by Sledgianowski et al.[56] is the need to include information systems, technology, and big data knowledge in accounting education. They contend that curricula should reflect the evolving technology environment and that it is imperative to enable accounting professionals to apply big data in their daily work. He et al.[57] use big data portrait analysis to investigate how COVID-19 affects Chinese businesses. Their studies underline the need to leverage big data to better grasp the pandemic’s economic effects and optimum resource allocation for effective crisis control.
In the field of big data in finance, Begenau et al.[53] look at the paths of major financial companies under the effect of big data adoption. Emphasising the value of data-driven insights in driving success, their research clarifies how big data technologies influence major financial institutions’ operational efficiency, competitive positioning, and growth plans. Focussing on numerous aspects of financial markets, including return estimates, volatility projections, risk evaluations, and algorithmic trading, Hasan et al.[58] investigate the impact of big data on finance, thereby highlighting the relevance of big data in forming financial decision-making procedures.
Underlining the requirement of adaptation, creativity, and integration of technology developments in the area, these well-known publications essentially highlight the transforming potential of big data in reforming accounting and financial procedures.
Table A5 ranks the most eminent writers based on total citations and publishing count. Three most prolific writers are a) Ionescu, L.; b) Faccia, A.; and c) McAleer, M. Table 4 shows that the writers with the most citations have helped to produce the most often cited works. For example, Vasarhelyi et al.[51] co-authored “Big Data in Accounting: An Overview,” which ran in Accounting Horizons in 2015.
A. By number of Publications (TP) |
||||||||||
Author |
TP |
NCP |
TC |
C/P |
C/CP |
h-index |
g-index |
m-index |
Pub year start |
|
1 |
Ionescu, Luminița |
4 |
2 |
35 |
8.75 |
17.5 |
2 |
1 |
0.4 |
2019 |
2 |
Faccia, Alessio |
4 |
3 |
19 |
4.75 |
6.3 |
2 |
1 |
0.4 |
2019 |
3 |
McAleer, Michael |
3 |
2 |
6 |
2 |
3 |
2 |
1 |
0.25 |
2016 |
4 |
Rao, Sandeep |
2 |
1 |
73 |
36.5 |
73 |
1 |
1 |
0.5 |
2022 |
5 |
Sharma, Dipasha |
2 |
1 |
73 |
36.5 |
73 |
1 |
1 |
0.5 |
2022 |
6 |
Kumar, Satish |
2 |
1 |
73 |
36.5 |
73 |
1 |
1 |
0.5 |
2022 |
7 |
Lim, Weng Marc |
2 |
1 |
73 |
36.5 |
73 |
1 |
1 |
0.5 |
2022 |
8 |
Mangla, Sachin Kumar |
2 |
1 |
73 |
36.5 |
73 |
1 |
1 |
0.5 |
2022 |
9 |
Zhang, Peng |
2 |
2 |
61 |
30.5 |
30.5 |
2 |
0 |
0.25 |
2016 |
10 |
Mosteanu, Narcisa Roxana |
2 |
2 |
15 |
7.5 |
7.5 |
1 |
1 |
0.2 |
2019 |
B. By Total Citations (TC) |
||||||||||
Author |
TP |
NCP |
TC |
C/P |
C/CP |
h-index |
g-index |
m-index |
Pub year start |
|
1 |
Tuttle, Brad M. |
1 |
1 |
260 |
260 |
260 |
1 |
0 |
0.11 |
2015 |
2 |
Vasarhelyi, Miklos A. |
1 |
1 |
260 |
260 |
260 |
1 |
0 |
0.11 |
2015 |
3 |
Kogan, Alexander |
1 |
1 |
260 |
260 |
260 |
1 |
0 |
0.11 |
2015 |
4 |
Willcocks, Leslie |
1 |
1 |
206 |
206 |
206 |
1 |
0 |
0.10 |
2014 |
5 |
Bhimani, Alnoor |
1 |
1 |
206 |
206 |
206 |
1 |
0 |
0.10 |
2014 |
6 |
Warren, J. Donald |
1 |
1 |
201 |
201 |
201 |
1 |
0 |
0.11 |
2015 |
7 |
Moffitt, Kevin C. |
1 |
1 |
201 |
201 |
201 |
1 |
0 |
0.11 |
2015 |
8 |
Byrnes, Paul |
1 |
1 |
201 |
201 |
201 |
1 |
0 |
0.11 |
2015 |
9 |
Richins, Greg |
1 |
1 |
135 |
135 |
135 |
1 |
0 |
0.14 |
2017 |
10 |
Wong, Christopher |
1 |
1 |
135 |
135 |
135 |
1 |
0 |
0.14 |
2017 |
11 |
Stapleton, Andrea |
1 |
1 |
135 |
135 |
135 |
1 |
0 |
0.14 |
2017 |
12 |
Stratopoulos, Theophanis C. |
1 |
1 |
135 |
135 |
135 |
1 |
0 |
0.14 |
2017 |
Table A6 showcases the most influential institutions based on their publication volume and average citations per cited publication. The three institutions with the highest number of publications are Spiru Haret University (Romania), Yunnan University DianChi College (China), and Swinburne University of Technology (Malaysia). In terms of citations per publication, the top three institutions are the University of South Carolina (US), The State University of New Jersey (US), and the London School of Economics (UK). A clear pattern emerges: Chinese universities are the most prolific (with 7 out of the top 12 institutions by publication count), and US institutions dominate in citations, with 9 out of the top 12.
A. By number of Publications (TP) |
||||||||||
Institution |
TP |
NCP |
TC |
C/P |
C/CP |
h-index |
g-index |
m-index |
Pub year start |
|
1 |
Spiru Haret University. |
4 |
35 |
2 |
8.75 |
17.5 |
2 |
1 |
0.4 |
2019 |
2 |
Yunnan University DianChi College. |
4 |
5 |
1 |
1.25 |
5 |
1 |
1 |
0.33 |
2021 |
3 |
Swinburne University of Technology Malaysia. |
4 |
146 |
2 |
36.5 |
73 |
2 |
1 |
1 |
2022 |
4 |
Harbin Finance University. |
3 |
2 |
1 |
0.667 |
2 |
1 |
1 |
0.25 |
2020 |
5 |
Yunnan University of Finance and Economics. |
3 |
3 |
2 |
1 |
1.5 |
1 |
1 |
0.25 |
2020 |
6 |
Shandong Women’s University. |
3 |
8 |
2 |
2.667 |
4 |
2 |
1 |
0.22 |
2015 |
7 |
Bina Nusantara University. |
3 |
0 |
0 |
0 |
0 |
0 |
1 |
0 |
2022 |
8 |
Beijing Normal University. |
3 |
23 |
1 |
7.667 |
23 |
1 |
1 |
0.2 |
2019 |
9 |
Asia University. |
3 |
25 |
2 |
8.333 |
12.5 |
2 |
1 |
0.25 |
2016 |
10 |
Yunnan Technology and Business University. |
3 |
2 |
1 |
0.667 |
2 |
1 |
1 |
0.25 |
2020 |
11 |
The University of Sydney Business School. |
3 |
134 |
2 |
44.67 |
67 |
2 |
1 |
0.29 |
2017 |
12 |
Renmin University of China. |
3 |
67 |
2 |
22.33 |
33.5 |
2 |
1 |
0.33 |
2018 |
B. By average Citations per Cited Publication (C/CP) |
||||||||||
Institution |
TP |
NCP |
TC |
C/P |
C/CP |
h-index |
g-index |
m-index |
Pub year start |
|
1 |
University of South Carolina US. |
1 |
260 |
1 |
260 |
260 |
1 |
0 |
0.11 |
2015 |
2 |
The State University of New Jersey US. |
2 |
461 |
2 |
230.5 |
230.5 |
2 |
0 |
0.22 |
2015 |
3 |
London School of Economics UK. |
1 |
206 |
1 |
206 |
206 |
1 |
0 |
0.1 |
2014 |
4 |
University of Hartford US. |
1 |
201 |
1 |
201 |
201 |
1 |
0 |
0.11 |
2015 |
5 |
University of Waterloo Canada. |
1 |
135 |
1 |
135 |
135 |
1 |
0 |
0.14 |
2017 |
6 |
Politecnico di Milano Italy. |
1 |
131 |
1 |
131 |
131 |
1 |
0 |
0.14 |
2017 |
7 |
Roehampton University US. |
1 |
131 |
1 |
131 |
131 |
1 |
0 |
0.14 |
2017 |
8 |
Loyola University Maryland US. |
1 |
120 |
1 |
120 |
120 |
1 |
0 |
0.11 |
2015 |
9 |
Ernst and Young Professional Practice group US. |
1 |
120 |
1 |
120 |
120 |
1 |
0 |
0.11 |
2015 |
10 |
Hofstra University US. |
1 |
95 |
1 |
95 |
95 |
1 |
0 |
0.14 |
2017 |
11 |
Centre for Economic Policy Research US. |
1 |
85 |
1 |
85 |
85 |
1 |
0 |
0.17 |
2018 |
12 |
Stanford University US. |
2 |
85 |
1 |
42.5 |
85 |
1 |
1 |
0.17 |
2018 |
One specific aspect of bibliometrics is the analysis of networks formed through collaborations, known as co-authorship networks. Analysing these networks can provide insights into the collaborative patterns of researchers, institutions, or countries and help identify influential entities, emergent trends, and clusters of research activity. Using VOSviewer, we perform co-authorship analysis and present the results for author level in Figure A2 and country level in Figure A3. These analyses explore the nationality of researchers who collaborate with their international counterparts. These figures show that researchers from China, the US and the UK are generally the most active in global collaboration.
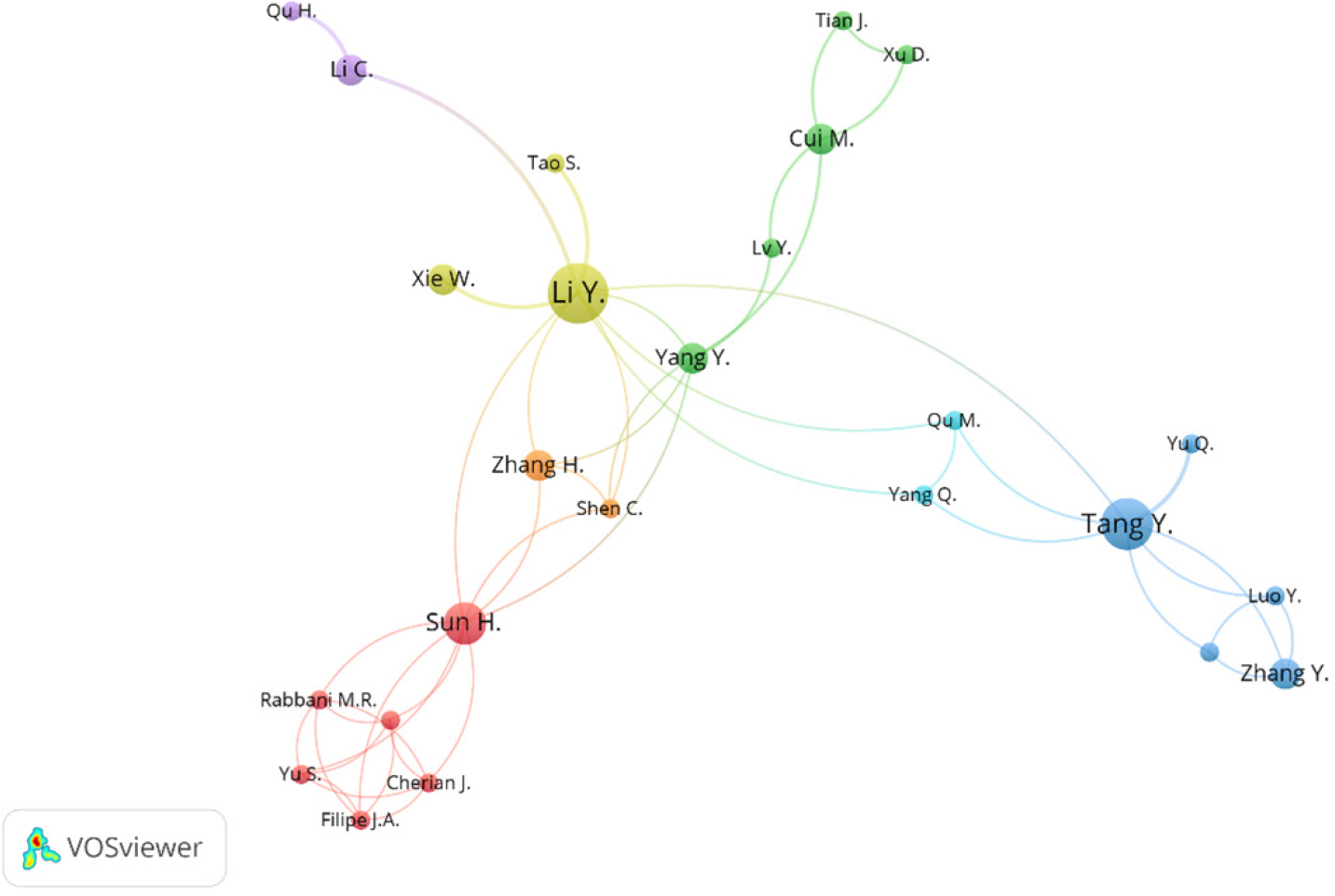
Figure A2:
Co-authorship analysis (author level). Note: This figure, created using fractional counting in VOSviewer, shows the network between co-authors at author level.
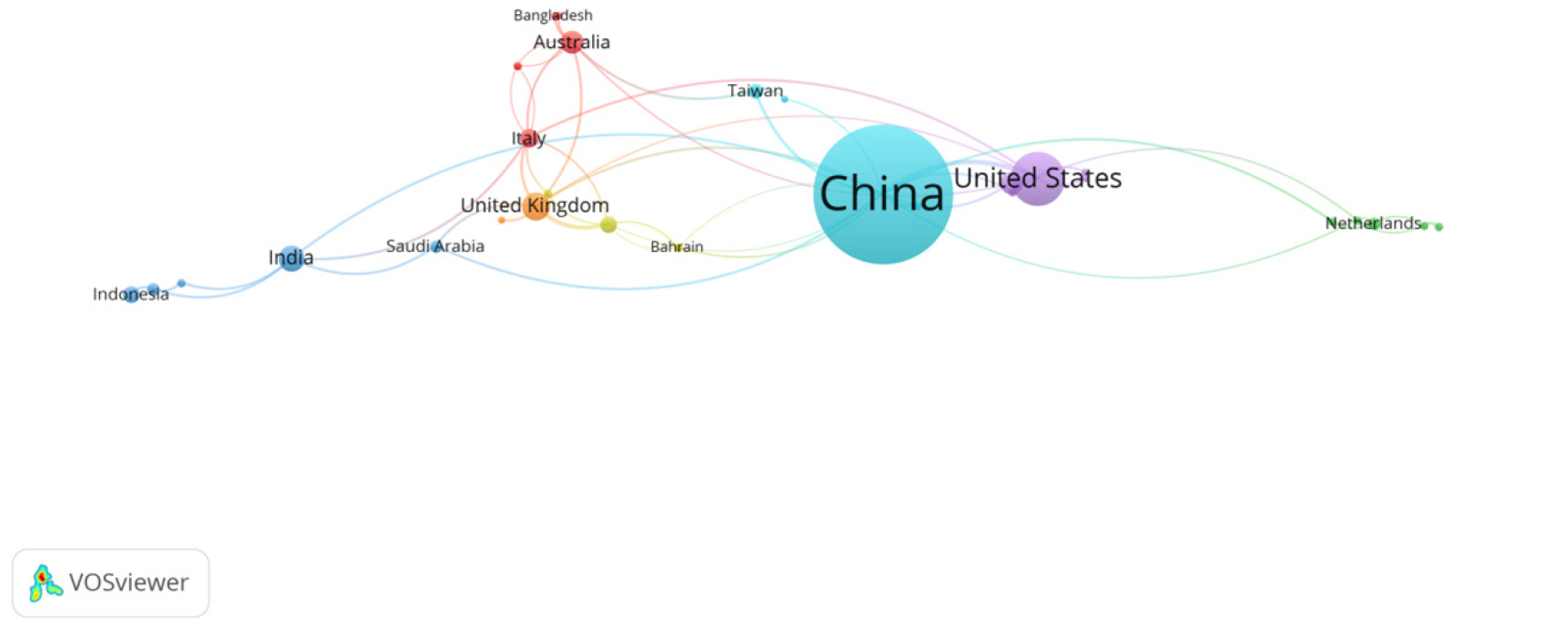
Figure A3:
Co-authorship analysis (country level). Note: This figure, created using fractional counting in VOSviewer, shows the network between co-authors at country level.
Scopus offers a myriad of metadata for each publication. A significant element among these is the keywords. These can be divided broadly into two categories: “Author keywords” and “Index keywords”. The publication’s authors provide the former, encapsulating the most pertinent terms to their research. By incorporating these keywords, authors aim to bolster the discoverability of their work, giving readers a concise snapshot of the paper’s focal points. Conversely, index keywords are added by Scopus, derived from its controlled vocabulary titled “Scopus Subject Areas”. This vocabulary, curated by experts, seeks to standardise terminology, enhancing the search and discovery process across the database. Table A7 enumerates the most prevalent keywords, illustrating distinctions between author and index keywords.
Top 30 Author keywords |
||||||||
Sl.No. |
Word |
Freq |
No |
Word |
Freq |
No |
Word |
Freq |
1 |
Big data |
161 |
11 |
Machine learning |
9 |
21 |
Data mining |
5 |
2 |
Data analytics |
31 |
12 |
Auditing |
8 |
22 |
Financial accounting |
5 |
3 |
Finance |
20 |
13 |
Accounting informatisation |
7 |
23 |
Financial services |
5 |
4 |
Accounting |
18 |
14 |
Big data era |
7 |
24 |
Forensic accounting |
5 |
5 |
Management accounting |
13 |
15 |
Cloud computing |
7 |
25 |
Internet |
5 |
6 |
Accounting information system |
12 |
16 |
Green finance |
7 |
26 |
Micro small and medium-sized enterprises |
5 |
7 |
Artificial intelligence |
12 |
17 |
Accounting education |
6 |
27 |
Sustainable development goals |
5 |
8 |
Cloud accounting |
12 |
18 |
Bibliometric |
6 |
28 |
Financial management |
4 |
9 |
Technology |
12 |
19 |
Blockchain |
6 |
29 |
Fintech |
4 |
10 |
Internet finance |
9 |
20 |
Risk management |
6 |
30 |
Risk assessment |
4 |
Top 30 Index keywords |
||||||||
Sl. No. |
Word |
|
No |
Word |
|
No |
Word |
|
1 |
Big data |
190 |
11 |
Data handling |
22 |
21 |
Continuous development |
12 |
2 |
Finance |
69 |
12 |
Management accounting |
20 |
22 |
Economics |
12 |
3 |
Accounting information |
60 |
13 |
Data analytics |
17 |
23 |
Financial accounting |
12 |
4 |
Information management |
44 |
14 |
Fintech |
16 |
24 |
Competition |
11 |
5 |
Risk assessment |
30 |
15 |
Financial management |
15 |
25 |
Information systems |
11 |
6 |
Data mining |
28 |
16 |
Risk management |
15 |
26 |
Informatisation |
11 |
7 |
Data technologies |
28 |
17 |
Blockchain |
14 |
27 |
Investments |
11 |
8 |
Decision making |
27 |
18 |
Artificial intelligence |
13 |
28 |
Advanced analytics |
10 |
9 |
Cloud computing |
26 |
19 |
Colleges and universities |
13 |
29 |
Cost accounting |
10 |
10 |
Economic and social effects |
24 |
20 |
Information use |
13 |
30 |
Credit risks |
10 |
Subsequently, by analysing co-occurrence patterns using VOSviewer, we discern the clusters of frequently paired keywords. Figures A4 and A5 depict the author keywords co-occurrence network and overlay visualisations, with a minimum occurrence of three. In Figure A4, the green cluster-centred on big data and finance-emerges as the most prominent. This is followed by the blue cluster, anchored in data analytics and accounting, and then by the red cluster, focusing on cloud and advanced technologies. Each cluster, while intersecting with finance and accounting, has its unique thematic emphasis. Figure A4 also indicates that the latest research in big data in accounting and finance frequently employs author keywords such as artificial intelligence and cloud computing.
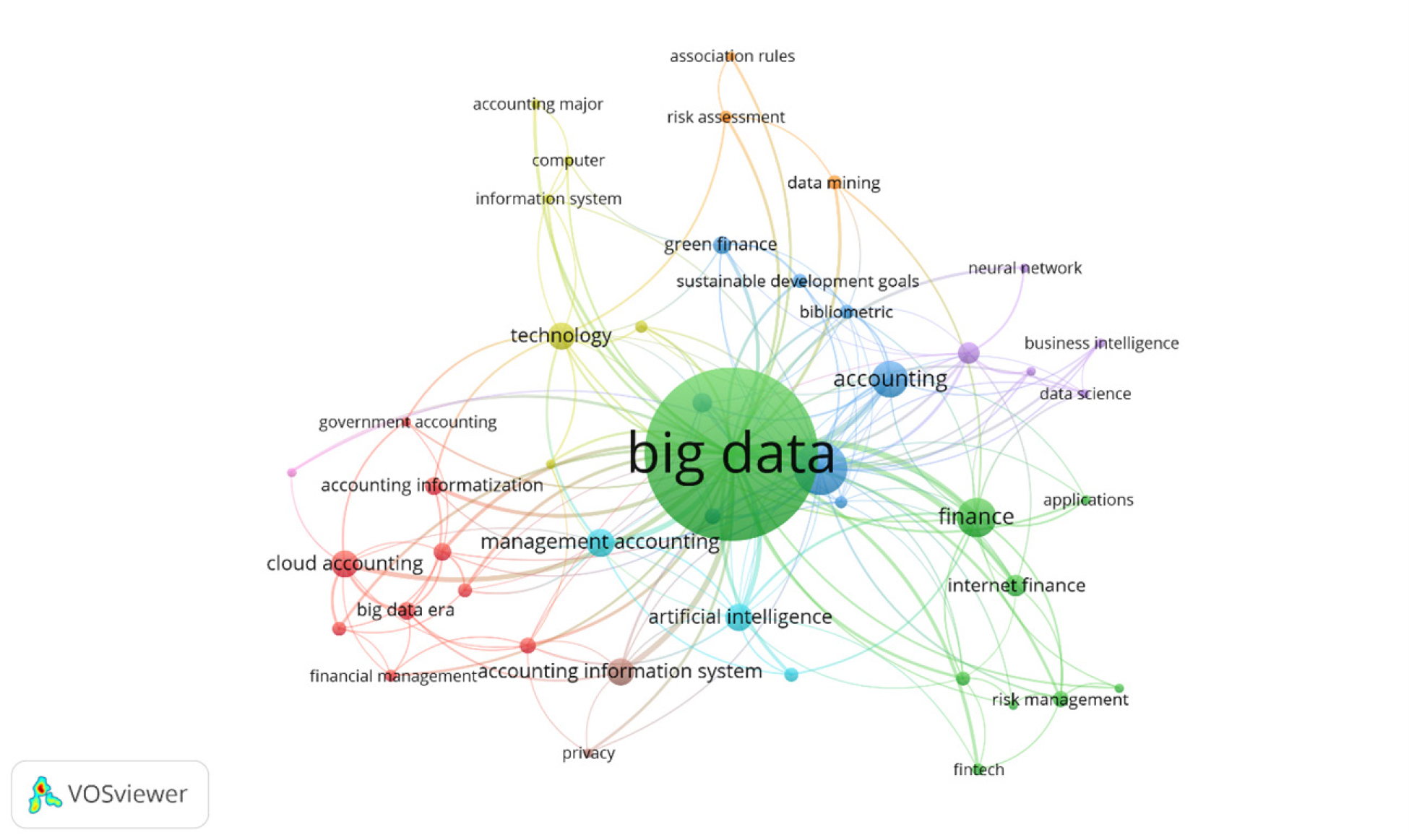
Figure A4:
Author keywords co-occurrence network visualisation. Note: This figure, created using fractional counting in VOSviewer, visualises the network of co-occurrence within authorial cues, with a minimum occurrence of three.
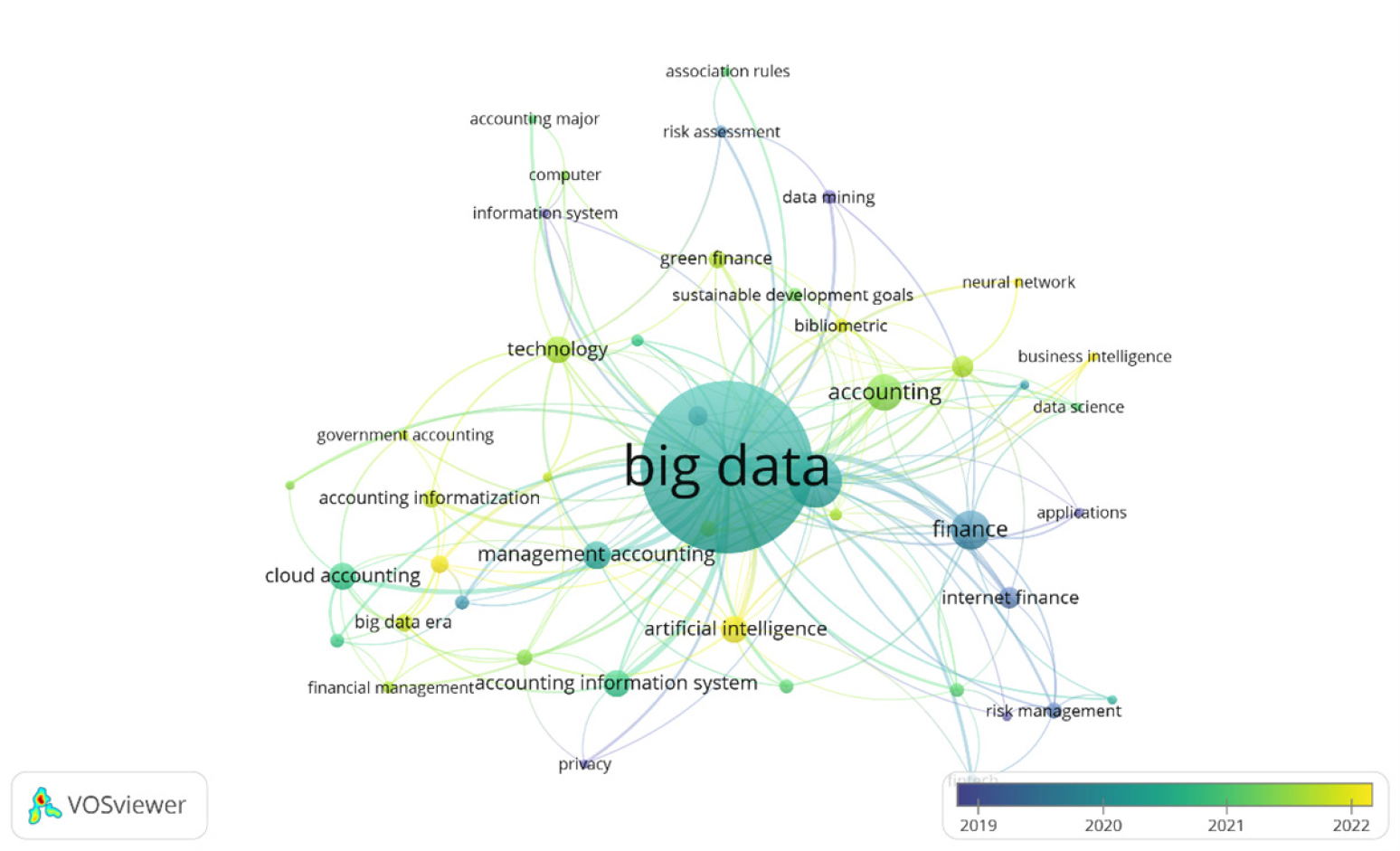
Figure A5:
Author keywords co-occurrence overlay visualisation. Note: This figure, created using fractional counting in VOSviewer, illustrates the overlay of co-occurrence within authorial cues, where at least three must occur.
Figures A6 and A7 display the index keywords’ co-occurrence network and overlay visualisations, with a minimum occurrence of five. In Figure A6, the red cluster, encompassing modern data technologies and fintech, stands out. It’s followed by the yellow cluster, which revolves around data and financial management, and then the green cluster, which focuses on information systems and accounting. These figures shed light on the centrality and interconnectedness of an index keyword within the broader nexus. In Figure A7, specific index keywords like development strategies, cloud accounting, and digital storage appear to be recent additions to research in big data within the accounting and finance sectors.
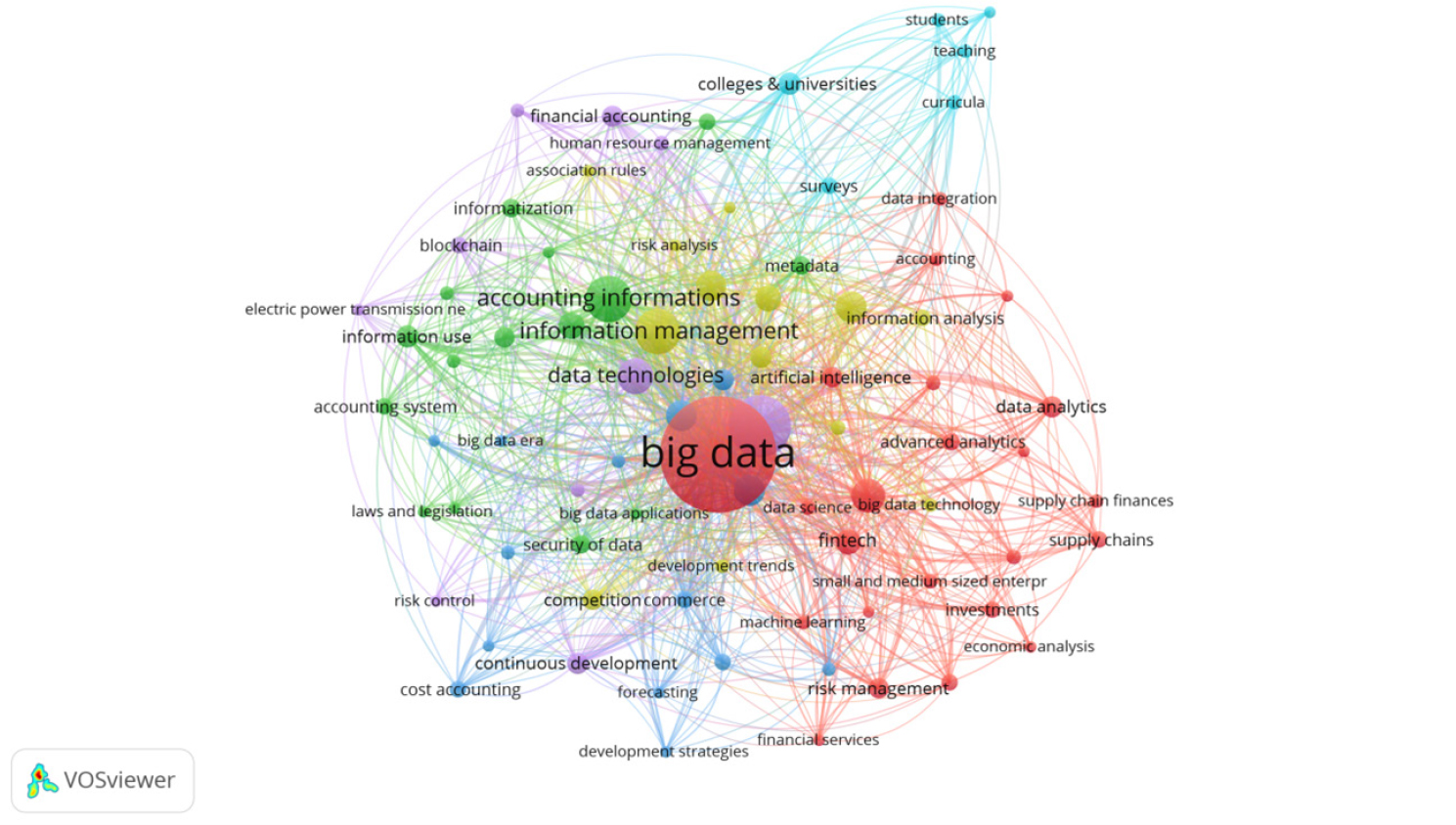
Figure A6:
Index keywords co-occurrence network visualisation. Note: This figure, created using fractional counting in VOSviewer, visualises the network of co-occurrence within index keywords, with a minimum occurrence of five.
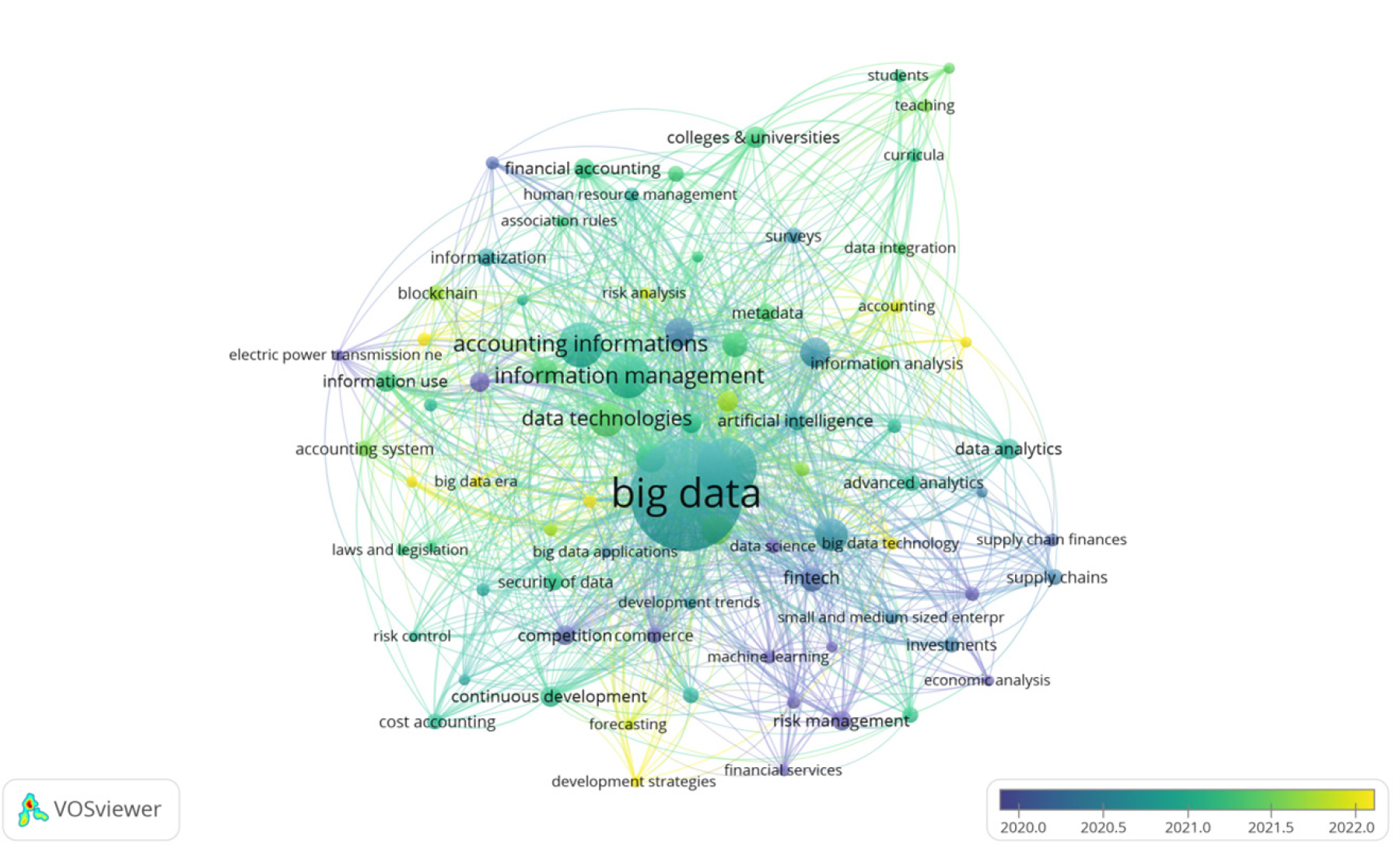
Figure A7:
Index keywords co-occurrence overlay visualisation. Note: This figure, created using fractional counting in VOSviewer, visualises the overlay of co-occurrences within the index keywords, with a minimum number of occurrences of five.
Figures A8 and A9, crafted using Biblioshiny, present the trending author and index keywords. “Big data” emerges as a favoured choice in both categories. Within the author’s keywords, “artificial intelligence” is a recent addition. In contrast, terms like integration, cloud accounting, cloud computing, and economic and social effects have recently gained popularity for index keywords. An intriguing query arises: How have these keywords evolved, especially during the pre-and post-onset of the COVID-19 pandemic? A visual assessment indicates a propensity for terms like “internet finance”, “finance”, and “risk management” before the pandemic. However, during the pandemic, there’s an evident tilt towards contemporary data and technology keywords such as “big data”, “technology”, “artificial intelligence”, and more traditional terms like “accounting” and “management accounting”. Similarly, “data visualisation” and “commerce” were the preferred index keywords before the pandemic. During the pandemic, there’s a clear lean towards terms like “big data”, “integration”, and “cloud accounting”, among others.
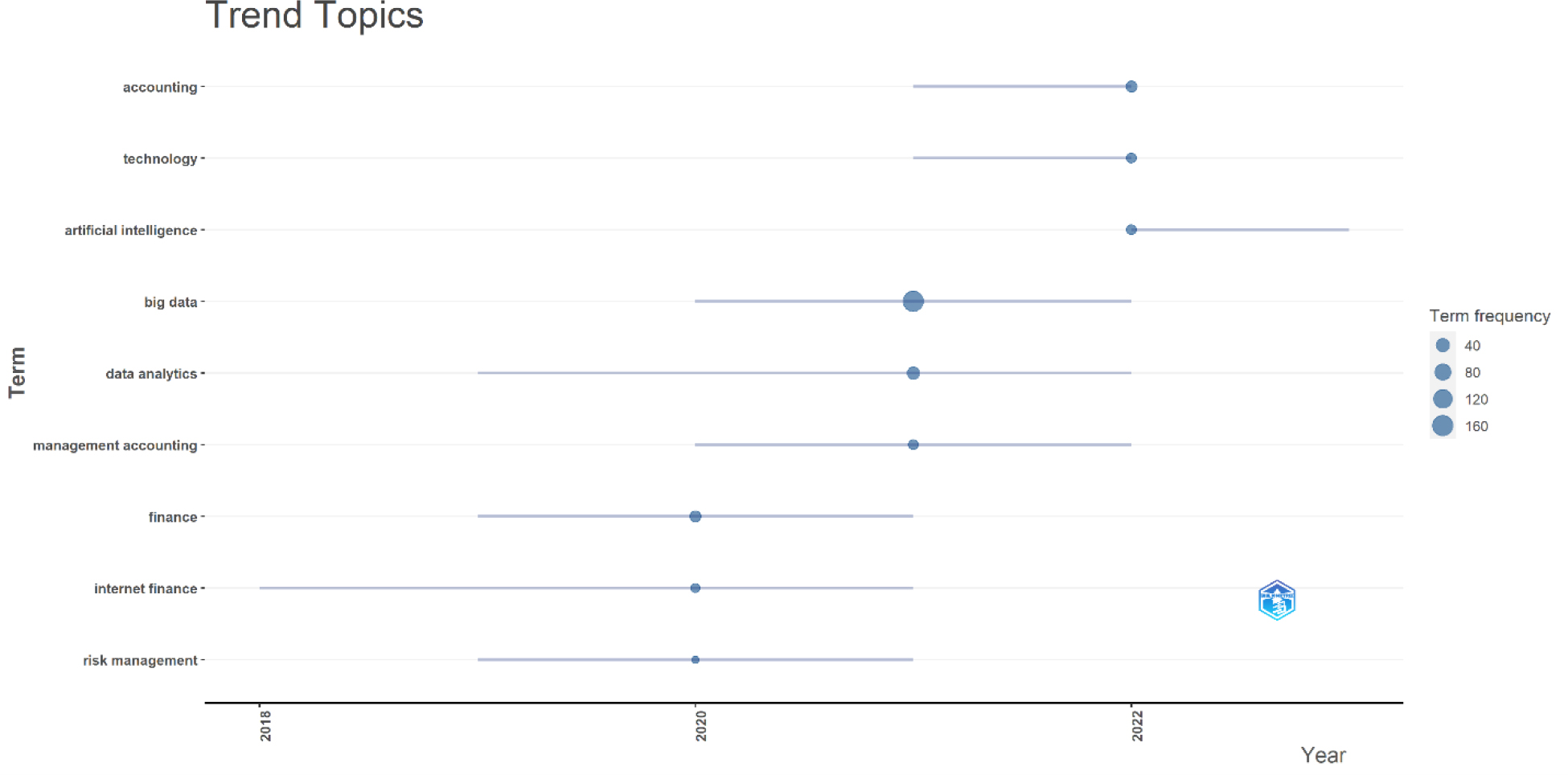
Figure A8:
Trending topics based on author keywords. Note: This figure, derived from Biblioshiny, presents the trending topics based on the author keywords.
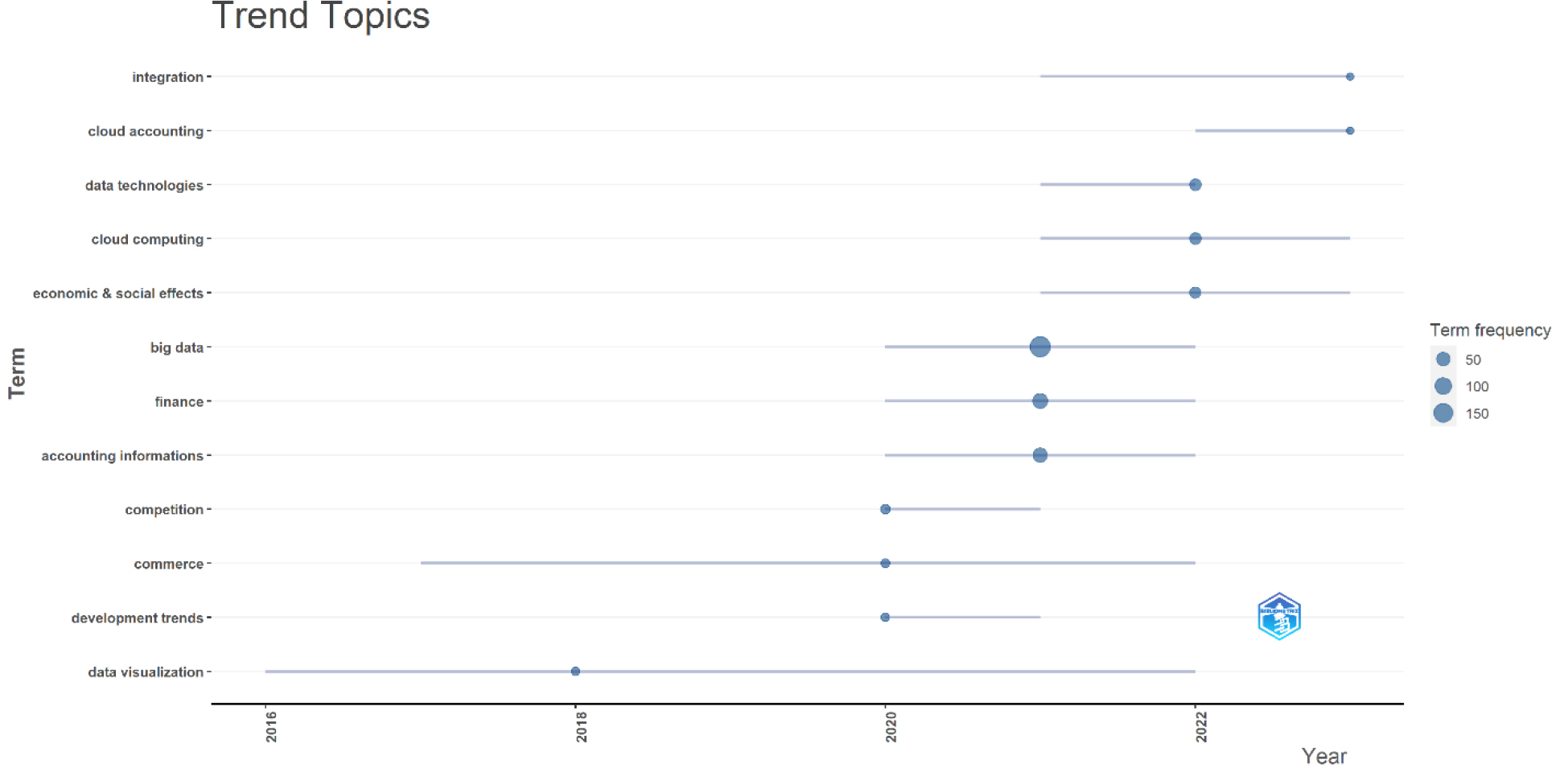
Figure A9:
Trending topics based on index keywords. Note: This figure, derived from Biblioshiny, presents the trending topics based on the index keywords.
We used Biblioshiny to do a co-word thematic map study to grasp the theme evolution in the field. We split the data into the 2013–2020 pre-COVID-19 era and the 2020–2022 COVID-19 era. The theme maps in Figures A10 and A11 use different colours to indicate distinctive term groups. Keywords including “finance, accounting information, and information management” helped the cluster centred on “big data” to become the central issue in the pre-pandemic period. The high degrees of “betweenness centrality” for these phrases highlight their essential function as links between pieces of the network. Moreover, the “big data” cluster showed the most critical average betweenness centrality and commanded the highest frequency of occurrences. This trend suggests that the body of research under examination primarily depends on “big data” issues, which act as fundamental connectors in the terminological network.
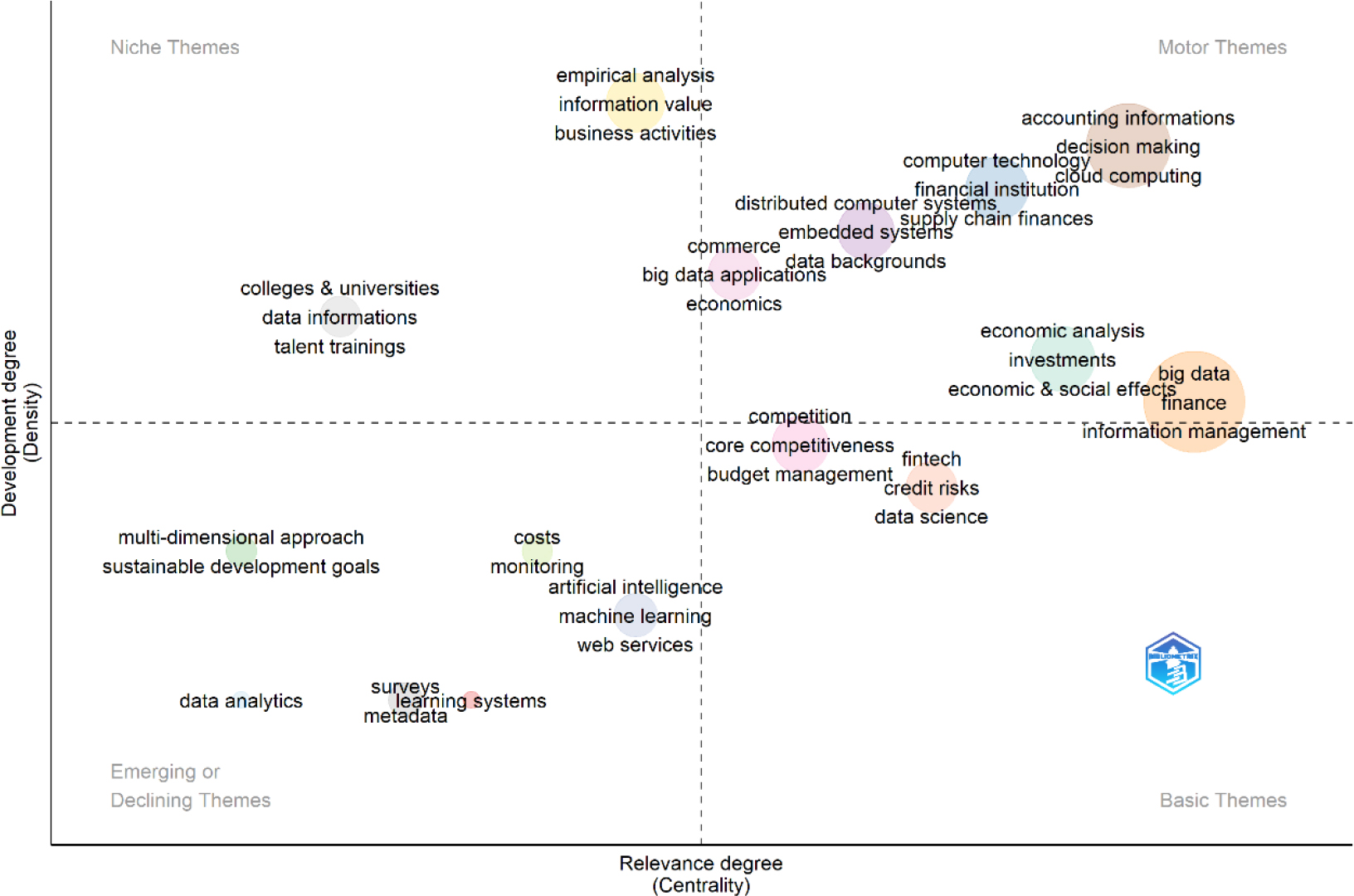
Figure A10:
Thematic map 2013-2020 (pre-COVID-19 pandemic era).
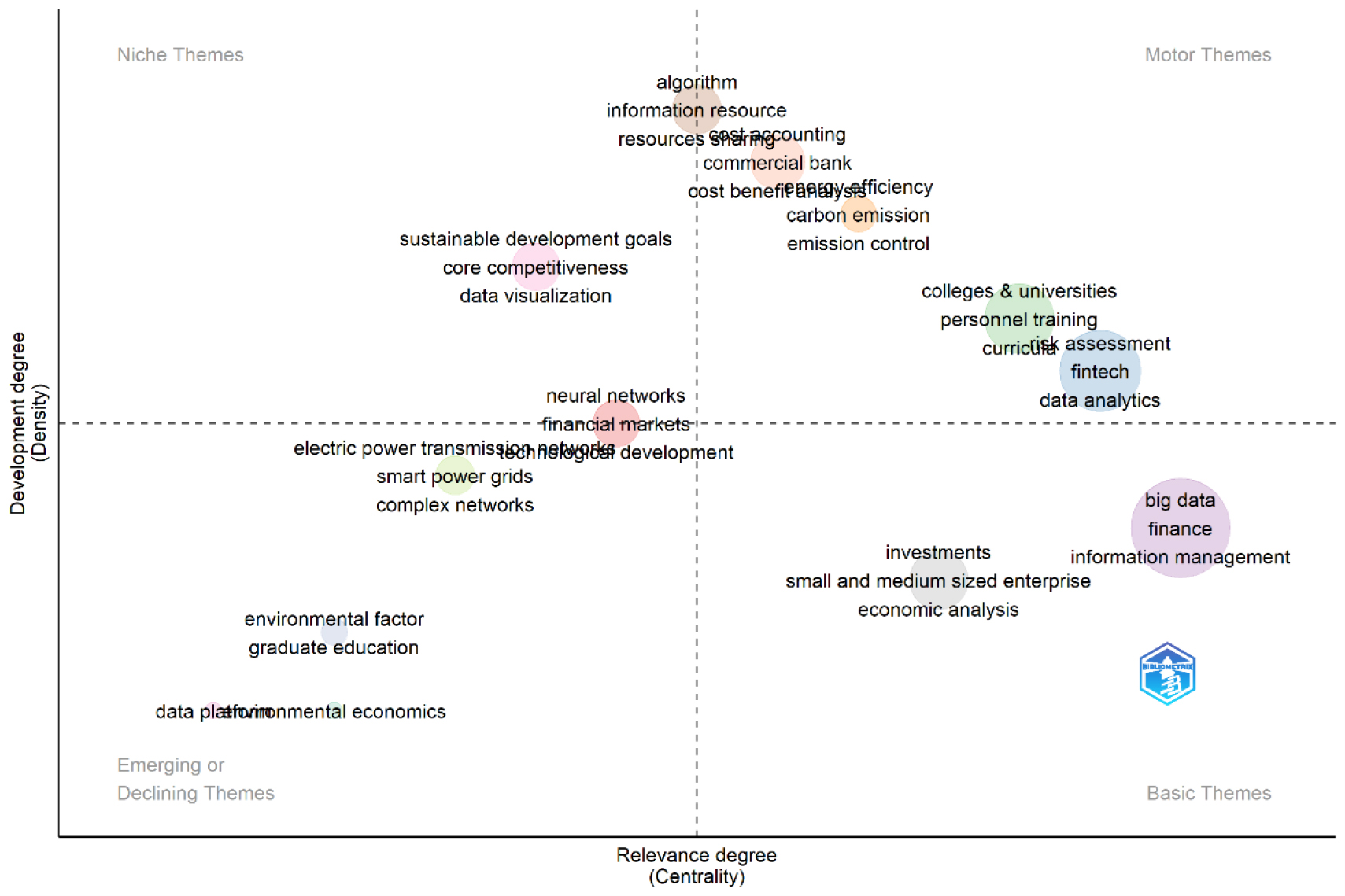
Figure A11:
Thematic map 2020-2022 (COVID-19 era).
The “big data” cluster kept its central importance in frequency and betweenness centrality during the COVID-19 pandemic. Still, this era also saw the emergence of fresh theme clusters like “neural networks” and “sustainable development goals,” suggesting a change in research direction. Although “big data” stayed a significant influence, other terms like “colleges and universities” and “investments” started to rise in importance, implying a subtle change in academic debate during the pandemic. The thematic study reveals minor but significant shifts in research concentration between the two eras. Although “big data analytics” is still a constant focus, the COVID-19 period has expanded the thematic terrain to embrace many subjects, from technology advancements like neural networks to societal needs like sustainability and education. This diversified attention could be seen as a reaction to the difficult issues and conversations sparked by the pandemic, underlined by the growing significance of concepts connected to decision-making in many spheres.
Further discussion-Major factors influencing emerging trends of big data in accounting and finance
This subsection discusses the major factors driving the evolution of big data research in accounting and finance, such as technological advancements, interdisciplinary integration, international collaboration, the impact of the COVID-19 pandemic, shifting academic and industry focus, and regulatory concerns. It offers a concentrated analysis of the critical elements influencing research trends and a structured yet brief picture of how these drivers collectively affect the field.
First of all, the trends seen are much influenced by the fast development of big data tools, including artificial intelligence, machine learning, and cloud computing. These technologies transform conventional accounting and financial practices by allowing enormous, unstructured data to be studied. As companies try to manage big datasets more effectively, the theme study suggests an increasing focus on artificial intelligence and cloud computing.[59] The COVID-19 pandemic accelerated the acceptance of these technologies; during worldwide lockdowns, cloud-based solutions became absolutely essential for operational continuity.[60]
Second, integrating big data with other fields, such as artificial intelligence, risk management, and sustainability, has extended study frontiers. Including sustainability in big data, research represents a more significant societal movement towards Environmental, Social, and Governance (ESG) concerns. Given that companies are under more and more pressure to include sustainability in their operations, researchers are looking at how big data might track ESG performance. Artificial intelligence is also fast becoming a primary instrument for risk assessment and financial decision-making, particularly in predictive analytics.[61]
Thirdly, the discipline is much influenced by worldwide collaboration, particularly between academics from China, the US, and the UK. These countries’ big data infrastructure investments drive this cooperation extensively. As scholars and organisations strive to innovate in financial risk assessment, data-driven decision-making, and financial management, globalising financial markets has further fostered cross-border partnerships. The globalisation of financial markets and the prevalence of investment have contributed to the rise of cooperation between these nations. Financial institutions in these nations seek cutting-edge methods to evaluate risk and make data-driven choices in response to the increased cross-border cash flow. Researchers in these countries are thus more likely to work together, pool their resources and knowledge, to anticipate and adapt to emerging global trends in financial innovation.[62] This pattern was supported by the co-authorship analysis of the document, which reveals that the areas with the highest levels of funding have the strongest cooperation.
Fourth, the pandemic changed research objectives and sped the acceptance of big data technology. As companies tried to negotiate the uncertainty of the pandemic, risk management and real-time data analysis took the front stage. While themes like Sustainable Development Goals (SDGs) developed as academics focused on societal implications and global concerns, neural networks-a type of artificial intelligence-gained attention for their capacity to analyse real-time data.[63] The pandemic highlighted the need for sophisticated data analytics to control risks and guarantee corporate resilience.
Fifth, big data research initially concentrated primarily on accounting, especially in improving financial reporting and auditing procedures.[64] With rising interest in fintech, algorithmic trading, and financial risk assessment, recent developments nonetheless indicate a turn towards finance. This change captures the more prominent digital revolution in finance and the rising application of data analytics in investment plans. The emergence of fintech and blockchain technology has also helped to bring about this shift since companies search for creative ideas to improve security and efficiency.
Finally, the paper suggests an understudied but essential influence: the shape of big data research by ethics and regulation. Privacy, data security, and ethical data use are increasingly crucial as accounting and finance gather and evaluate more data. Investigating how extensive data can be utilised ethically while following new rules, such as the General Data Protection Regulation (GDPR) in Europe, researchers are probably reacting to these problems.[65] This issue is especially pertinent in finance, where data abuse can result in significant financial and reputation losses. Research on risk assessment and compliance has thus acquired momentum as academics investigate how big data may enable companies to negotiate ever more complex regulatory surroundings.
In summary, technical, societal, and economic considerations all propel the trends highlighted in this paper. Developments have significantly influenced the research landscape in big data technologies, the increasing significance of multidisciplinary research, and the globalisation of finance. Researchers were driven to investigate new topics, including sustainability and risk management, as the COVID-19 pandemic accelerated the use of digital tools.[66] Finally, regulatory demands and changes in academic priorities are pushing academics in different directions when studying the convergence of big data, accounting, and finance. Understanding these underlying causes enhances the discussion by giving a more comprehensive context for the trends seen in the bibliometric analysis.
CONCLUSION
This study has fulfilled its three main objectives quite successfully. First, it identified the most influential papers, authors, and institutions in big data research within accounting and finance. Focusing on institutions and academics from China, the US, and the UK, this study emphasises the leading players forming the research scene. These discoveries highlight significant personalities and places of excellence in the field, offering important direction for future research.
Second, studying co-authorship networks and keyword co-occurrence patterns helped one grasp the area’s conceptual framework and cooperative dynamics. Big data research in accounting and finance is worldwide, as shown by the frequency of international cooperation, especially among academics from China, the US, and the UK. This trend emphasises how crucial cross-border cooperation is for fostering creativity and increasing the influence of research in a fast-changing field under development.
Thirdly, the thematic mapping of keywords provided a whole picture of the change of study subjects across time. While developing fields like “artificial intelligence,” “cloud computing,” and “risk assessment,” the study revealed that “big data,” “finance,” and “accounting” remain prominent themes reflecting the increasing relevance of modern technologies in the industry. The COVID-19 pandemic especially had a transforming influence, hastening the establishment of new study areas, including “neural networks” and “sustainable development goals”, implying a change towards combining technologically driven solutions with more general societal issues.
This paper contributes significantly to the field by thoroughly mapping newly developed research topics and pointing out the main forces behind the big data debate on accounting and finance. The research offers a road map for future studies by stressing established and new trends, especially in fields like AI-driven financial decision-making, cloud-based accounting systems, and integrating sustainability criteria in financial reporting. This work not only meets its stated goals but also advances the more general subject by providing a critical analysis of the direction of big data research and its consequences for accounting, finance, and multidisciplinary study. It emphasises the transforming power of big data technology and demands ongoing research of new ideas that fit world issues, including sustainability and technical development.
The study does, yet, also recognise its limitations. The exclusive reliance on the Scopus database could lead to a limited perspective of the research scene since other significant works could be indexed in other databases. Furthermore, considering the dynamic character of the topic, depending just on bibliometric data could not adequately represent the most recent advancements or fast-changing patterns. Future studies could overcome these constraints by including a broader spectrum of databases, including Web of Science (WoS) and Dimensions, offering a more complete and sophisticated grasp of big data research in accounting and finance.
Cite this article:
Mohamad A. Mapping the Intellectual Landscape of Big Data in Accounting and Finance: A Decade of Bibliometric Analysis (2013-2023). J Scientometric Res. 2025;14(1):201-20.
References
- Goldstein I, Spatt CS, Ye M.. Big data in finance. Rev Financ Stud.. 2021;34(7):3213-25. [CrossRef] | [Google Scholar]
- Nissim D.. Big data, accounting information, and valuation. J Finan Data Sci [Internet].. 2022;8:69-85. [CrossRef] | [Google Scholar]
- Cockcroft S, Russell M.. Big data opportunities for accounting and finance practice and research. Aust Acc Rev [Internet].. 2018;28(3):323-33. [CrossRef] | [Google Scholar]
- Agustí MA, Orta-Pérez M.. Big data and artificial intelligence in the fields of accounting and auditing: a bibliometric analysis. Rev Esp Financ Contab [Internet].. 2022;52(3):412-38. [CrossRef] | [Google Scholar]
- Gepp A, Linnenluecke MK, O’Neill TJ, Smith T.. Big data techniques in auditing research and practice: current trends and future opportunities. J Acc Lit [Internet].. 2018;40(1):102-15. [CrossRef] | [Google Scholar]
- Nobanee H.. A bibliometric review of big data in finance. Big Data [Internet].. 2021;9(2):73-8. [PubMed] | [CrossRef] | [Google Scholar]
- Tseng ML, Bui TD, Lim MK, Tsai FM, Tan RR.. Comparing world regional sustainable supply chain finance using big data analytics: a bibliometric analysis. Ind Manag Data Syst.. 2021;121(3):657-700. [CrossRef] | [Google Scholar]
- Varma A, Piedepalumbo P, Mancini D.. Big data and accounting: A bibliometric study. Int J Digit Acc Res.. 2021;21(October):203-38. [CrossRef] | [Google Scholar]
- Warren JD, Moffitt KC, Byrnes P.. How big data will change accounting. Acc Horiz [Internet].. 2015;29(2):397-407. [CrossRef] | [Google Scholar]
- Younis NM.. Big data and the future of the accounting profession. Indian J Sci Technol.. 2020;13(8):883-92. [CrossRef] | [Google Scholar]
- Abueid R, Hakami TA.. Impact of big data and data analysis on accounting function: evidence from Palestine. Cent Eur Manag;J. 2023 [CrossRef] | [Google Scholar]
- Ren S.. Optimization of enterprise financial management and decision-making systems based on big data. J Math.. 2022;2022(1) [CrossRef] | [Google Scholar]
- Xu Z, Zhou W.. A data technology oriented to information fusion to build an intelligent accounting computerized model [sci program]. Scientific Programming.. 2021;2021:1-12. [CrossRef] | [Google Scholar]
- Esteban SA, Urquía-Grande E, de Silva AM, Pérez-Estébanez R.. Big Data, Accounting and International Development: trends and challenges. Cuad Gest.. 2022;22(1) [CrossRef] | [Google Scholar]
- Arnaboldi M, Busco C, Cuganesan S.. Accounting, accountability, social media and big data: revolution or hype?. Acc Audit Acc.. 2017;30(4):762-76. [CrossRef] | [Google Scholar]
- Akbulut DH, Kaya I.. Big data analytics in financial reporting and accounting. Pressacademia.. 2018;7(1) [CrossRef] | [Google Scholar]
- Coyne EM, Coyne JG, Walker KB.. Big Data information governance by accountants. Int J Acc Inf Manag.. 2018;26(1):153-70. [CrossRef] | [Google Scholar]
- Dagilienė L, Klovienė L.. Motivation to use big data and big data analytics in external auditing. Manag Audit J.. 2019;34(7):750-82. [CrossRef] | [Google Scholar]
- Ar-Raisi FA, Sakti E, Anggono A, Tarjo T.. Bibliometric analysis of big data research in finance. J Magister Akunt Trisakti.. 2023;10(1):1-18. [CrossRef] | [Google Scholar]
- Murthy US, Geerts GL.. An REA ontology-based model for mapping big data to accounting information systems elements. J Inf Syst [Internet].. 2017;31(3):45-61. [CrossRef] | [Google Scholar]
- Mishra D, Gunasekaran A, Papadopoulos T, Childe SJ.. Big Data and supply chain management: a review and bibliometric analysis. Ann Oper Res.. 2018;270(1–2) [CrossRef] | [Google Scholar]
- Bradford SC.. Sources of information on specific subjects 1934. J Inf Sci [Internet].. 1985;10(4):176-80. [CrossRef] | [Google Scholar]
- Price DJ.. Networks of scientific papers. Science.. 1965;149(3683):510-5. [PubMed] | [CrossRef] | [Google Scholar]
- Kim J, McMillan SJ.. Evaluation of internet advertising research: A bibliometric analysis of citations from key sources. J Advert [Internet].. 2008;37(1):99-112. [CrossRef] | [Google Scholar]
- Mahmudin A., Abdul-Mohsin AM.. Mapping research publications related to business intelligence: an overview of bibliometric data. Eur Proc Soc Behav Sci EpSBS. 2019 [CrossRef] | [Google Scholar]
- Mohamad A.. Navigating through pandemics: a bibliometric analysis of research trends in Islamic finance and Islamic banking. JIABR.. 2024 [CrossRef] | [Google Scholar]
- Jamaludin H.. Evolution of waste and circular economy: pre- and post-Covid-19. Circ econ sustain [Internet]. 2024 [CrossRef] | [Google Scholar]
- Sobral SR.. Mobile learning in higher education: A bibliometric review. Int J Interact Mob Technol.. 2020;14(11) [CrossRef] | [Google Scholar]
- Bhairawa Putera P, Pasciana R.. Big Data for Public Domain: A bibliometric and visualized study of the scientific discourse during 2000-2020. Policy Gov Rev.. 2021;5(3) [CrossRef] | [Google Scholar]
- Ianchuk S.. Bibliometric analysis and visualization of funding social housing: connection of sociological and economic research. Socioecon Chall.. 2021;5(1):144-53. [CrossRef] | [Google Scholar]
- Miah MS, Hossain Lipu MS, Meraj ST, Hasan K, Ansari S, Jamal T, et al. Optimized energy management schemes for electric vehicle applications: A bibliometric analysis towards future trends. Sustainability.. 2021;13(22) [CrossRef] | [Google Scholar]
- Sweileh WM, Huijer HA, Al-Jabi SW, Zyoud SH, Sawalha AF.. Nursing and midwifery research activity in Arab countries from 1950 to 2017. BMC Health Serv Res.. 2019;19(1):340 [PubMed] | [CrossRef] | [Google Scholar]
- Ngetich BK, Nuryakin INQ, Qamari Ika Nurul. Research trends of supply chain management practice before and during pandemic: A bibliometric analysis. Int J Res Bus Soc Sci.. 2022;11(2):1-15. [CrossRef] | [Google Scholar]
- Elaish MM, Shuib L, Ghani NA, Mujtaba G, Ebrahim NA.. A bibliometric analysis of m-learning from topic inception to 2015. Int J Mob Learn Organ.. 2019;13(1) [CrossRef] | [Google Scholar]
- Balzano M.. Serendipity in management studies: a literature review and future research directions. Manag Decis.. 2022;60(13):130-52. [CrossRef] | [Google Scholar]
- Shi J, Duan K, Wu G, Si H, Zhang R.. Sustainability at the community level: A bibliometric journey around a set of sustainability-related terms. Sustain Dev.. 2022;30(1):256-74. [CrossRef] | [Google Scholar]
- Yani NF, Soebagyo J.. Bibliometric analysis of mathematical communication skills using Scopus database. JPMIPA.. 2023;14(1) [CrossRef] | [Google Scholar]
- Zyoud SH, Al-Jabi SW, Sweileh WM, Al-Khalil S, Alqub M, Awang R., et al. Global methaemoglobinaemia research output (1940-2013): a bibliometric analysis. Springerplus.. 2015;4(1):626 [PubMed] | [CrossRef] | [Google Scholar]
- Ekin CC, Gul A.. Bibliometric analysis of game-based researches in educational research. Int J Technol Educ.. 2022;5(3):499-517. [CrossRef] | [Google Scholar]
- Umar E, Ikram M, Haider J, Nabgan W, Imran M, Nazir G., et al. A state-of-art review of the metal oxide-based nanomaterials effect on photocatalytic degradation of malachite green dyes and a bibliometric analysis. Glob Chall.. 2023;7(6):2300001 [PubMed] | [CrossRef] | [Google Scholar]
- Turmuzi M, Suharta IG, Astawa IW, Suparta IN.. Mapping of mobile learning research directions and trends in Scopus-indexed journals: A bibliometric analysis. Int J Interact Mob Technol.. 2023;17(3):39-69. [CrossRef] | [Google Scholar]
- Ahmi A.. biblioMagika [Internet]. 2023 [Aug 31 2023].
- Ahmi AA.. OpenRefine: An approachable tool for cleaning and harmonizing bibliographical data [Internet]. AIP Conf Proc.. 2023;2827:1-11. [CrossRef] | [Google Scholar]
- Mongeon P, Paul-Hus A.. The journal coverage of Web of Science and Scopus: a comparative analysis. Scientometrics.. 2016;106(1):213-28. [CrossRef] | [Google Scholar]
- Caputo A, Kargina M.. A user-friendly method to merge Scopus and Web of Science data during bibliometric analysis. J Mark Anal.. 2022;10(1):82-8. [CrossRef] | [Google Scholar]
- Villa E, Ruiz L, Valencia A, Picón E.. Electronic commerce: factors involved in its adoption from a bibliometric analysis. J Theor Appl Electron Commer Res.. 2018;13(1):39-70. [CrossRef] | [Google Scholar]
- van Eck NJ, Waltman L.. Software survey: VOSviewer, a computer program for bibliometric mapping. Scientometrics [Internet].. 2010;84(2):523-38. [PubMed] | [CrossRef] | [Google Scholar]
- Aria M, Cuccurullo C.. bibliometrix: an R-tool for comprehensive science mapping analysis. J Informetr [Internet].. 2017;11(4):959-75. [CrossRef] | [Google Scholar]
- Roth RE, Kelly M, Underwood N, Lally N, Vincent K, Sack C., et al. Interactive and multiscale thematic maps: A preliminary study. Abstr ICA.. 2019;1:1-2. [CrossRef] | [Google Scholar]
- Tsorlini A, Sieber R, Hurni L, Klauser H, Gloor T.. Designing a rule-based wizard for visualizing statistical data on thematic maps. Cartogr Perspect.. 2017;2017(86):5-23. [CrossRef] | [Google Scholar]
- Vasarhelyi MA, Kogan A, Tuttle BM.. Big data in accounting: an overview. Acc Horiz [Internet].. 2015;29(2):381-96. [CrossRef] | [Google Scholar]
- Bhimani A, Willcocks L.. Digitisation, Big Data and the transformation of accounting information. Acc Bus Res [Internet].. 2014;44(4):469-90. [CrossRef] | [Google Scholar]
- Begenau J, Farboodi M, Veldkamp L.. Big data in finance and the growth of large firms. J Monet Econ [Internet].. 2018;97:71-87. [CrossRef] | [Google Scholar]
- Richins G, Stapleton A, Stratopoulos TC, Wong C.. Big data analytics: opportunity or threat for the accounting profession?. J Inf Syst.. 2017;31(3):63-79. [CrossRef] | [Google Scholar]
- Krahel JP, Titera WR.. Consequences of big data and formalization on accounting and auditing standards. Acc Horiz.. 2015;29(2):409-22. [CrossRef] | [Google Scholar]
- Sledgianowski D, Gomaa M, Tan C.. Toward integration of Big Data, technology and information systems competencies into the accounting curriculum. J Acc Educ.. 2017;38:81-93. [CrossRef] | [Google Scholar]
- He P, Niu H, Sun Z, Li T.. Accounting index of COVID-19 impact on Chinese industries: A case study using big data portrait analysis. Emerg Mark Financ Trade.. 2020;56(10):2332-49. [CrossRef] | [Google Scholar]
- Hasan MM, Popp J, Oláh J.. Current landscape and influence of big data on finance. J Big Data.. 2020;7(1) [CrossRef] | [Google Scholar]
- Arora M, Sharma RL.. Artificial intelligence and big data: ontological and communicative perspectives in multi-sectoral scenarios of modern businesses. Foresight.. 2023;25(1):126-43. [CrossRef] | [Google Scholar]
- Alhomdy S, Thabit F, Abdulrazzak FH, Haldorai A, Jagtap S.. The role of cloud computing technology: A savior to fight the lockdown in COVID 19 crisis, the benefits, characteristics and applications. Int J Intell Netw.. 2021;2:166-74. [CrossRef] | [Google Scholar]
- Nguyen DK, Sermpinis G, Stasinakis C.. Big data, artificial intelligence and machine learning: A transformative symbiosis in favour of financial technology. Eur Financ Manag.. 2023;29(2):517-48. [CrossRef] | [Google Scholar]
- Mariani MM, Machado I, Magrelli V, Dwivedi YK.. Artificial intelligence in innovation research: A systematic review, conceptual framework, and future research directions. Technovation.. 2023;122 [CrossRef] | [Google Scholar]
- Goel A, Goel AK, Kumar A.. The role of artificial neural network and machine learning in utilizing spatial information. Spat Inf Res.. 2023;31(3):275-85. [CrossRef] | [Google Scholar]
- . Big data, data analytics and artificial intelligence in accounting: an overview. In:. Handbook of big data research methods.. 2023:32-51. [CrossRef] | [Google Scholar]
- Haddara M, Salazar A, Langseth M.. Exploring the impact of GDPR on big data analytics operations in the e-commerce industry. Procedia Comput Sci.. 2023;219:767-77. [CrossRef] | [Google Scholar]
- Wu T.. Digital project management: rapid changes define new working environments. J Bus Strategy.. 2022;43(5):323-31. [CrossRef] | [Google Scholar]