Contents
ABSTRACT
ABSTRACT
Stock market prediction and analysis is an important subject of discussion for stock market prediction and analysis. The aim of the study is to explore literature with respect to stock market and its prediction. The paper focuses on the theme of evolution and conceptual cluster of stock market analysis and prediction based on Bibliometric Analysis. The study mainly derives on three keywords, i.e. stock market, market prediction, as well as stock analysis. For this study, 160 Scopus indexed research papers has been used for analysis. In our analysis the journal of prominence, the most cited authors, most cited articles, co-authorship network analysis, keyword occurrences, clustering and visualization. The outcome demonstrates the trends of research within the specified field and will assist the research community in addressing challenges pertaining to each core concept.
INTRODUCTION
The analysis of stocks is crucial to form different investment and trading strategies to make optimal portfolio. The prediction of stock market in terms of movement and price of stocks is very dynamic due to the complex phenomena of stocks.[1] The factors responsible for such dynamic nature of stock market are company-specific variables, political, psychological and economic.[2] The stock price represents the current discounted value of the anticipated future expectationĀ of a company inĀ the real economy. There are studies which have been analysing stock market from different dimensions. The corporate board serves as the governing body of an organization. The restructuring of a company’s board can have a significant impact on its policy. A study quantify the correlation between alterations in company boards and equity market crises.[3] Another study focusedĀ on the investors’ response to the appointment of executives in supply chain and operations management.[4] Some investors depend on corporate disclosure, while others rely on media coverage for information. Controlling media coverage can alter the way investors perceive things. Another study analyzed the potential risk of a stock market crash by examining the function of media in effectively disseminating accurate news to the intended investor.[5] Financial performance of stocks is the foremost indicators of investment. Another study have analyzed stocks using financial metrics/ratios such as profitability, growth, liquidity, solvency and operational efficiency.[6] Several research papers also have analyzed the stock price movement using technical indicator. In some research studies, technical indicators like Bollinger Bands, Relative Strength Index and Chart Patterns have been used for evaluating stocks.[7]
In stock market, the successful prediction of stocks price has greater impact on portfolio return. But the Random Walk theory which states that market do random walk so prediction of stock movement is impossible.[8] But some research studies state that market movement is predictable to some extent.[9] This has started the race of predicting the stock movement among the researchers. Forecasting in the stock market is a complex procedure that incorporates both qualitative and quantitative data. From a financial market context, it covers the forecasting of stock prices, stock market indices. Researchers and practitioners worldwide have been employing various prediction models to make inform decisions on the buying, selling and holding of stocks. Both quantitative and qualitative (sentiment, other qualitative data) data have been taken into account for the purpose of predicting stock movement, both individually and in combination. Prediction techniques can be categorized as Econometric, Machine learning, Deep learningĀ sentiment analysis and hybrid approaches. Each of these broad techniques encompasses various methodologies.[10]
The paper aims to assess the trend of research in the area of analysis and modelling of stock market. The research questions are:
at systematic analysis of the trend of research in the field of analysis and modelling of stock market. The research questions for this paper are as follows:
Who are the top authors dominating the research on stock market analysis and prediction?
Which are the impactful publications, journal, countries and institutions in the given field?
How research on stock market analysis and prediction have emerged in last decade?
What are significant research trends existing in the given topic?
What are future researches suggested by researchers
The structure of the paper is as follows. The section 2 covers the most recent developments in the domain stock market analysis and prediction, section 3 Recent technique used in Stock prediction and analysis. section 4 covers the methodology of the study, section 5 covers the discussion and analysis, section 6 covers conclusion and contribution.
Recent activities and trends in stock market
The stock market is a fundamental component of the organized sector of an economy. The stock market, is a reflection of the actual economy.[11] Stock market serve as a mechanism for channelling individual funds into the gainful investment that are used in productive endeavour.[12] Stakeholder analyse the stocks form different prospectives to make investment decisions. The interconnections among stock markets are examined by employing a cointegration analysisĀ to construct a portfolio.[13] The impact of oil price shock are different at different market and different economic level.[14] The fluctuation of oil price depends on types and timing of shock. The fluctuation of oil price has significant positive relationship with stock market crash.[15] Clean energy stock has become focus point due to global warming and sea level rising. Clean energy stock influence the crude oil price.[16] Oil price and electricity price has significant role in shaping clean energy stock price.[17] Beside these, corporate reputation is the significant aspect of investor perception. Any alteration in corporate reputation due to different reason is having impact on stock price. Environmental, Social and Governance (ESG) is defined as the responsibility on environment, society and governance in terms of companies score on their responsibility metrics and standards for potential investments. The effect of adverse ESG coverage on different sector varies as it has minimum impact on alcohol, gaming and tabaco as comparison to other sectors.[18] The prediction of stock movement is growing rapidly with advancement of information and technical tools. The machine learning and deep learning algorithms used for stock future movement forecasting.[19,20] There are significant features from pool of huge number of features to increase the accuracy of the prediction results and have optimised parameters for stock price forecasting.[21,22] The sentiment of the investors are crucial feature to predict the price of the stocks. The sentiment analysis is being performed using the natural language processing to convert those sentiment data to subjectivity and polarity score. These subjectivity and polarity score being used with historical price and technical indicatorās to predict the future movement of the stocks.[23] Machine learning algorithms like multi objective genetic algorithm, has been used for portfolio optimization.[24]
Recent technique used in Prediction and analysis of stock market
The analysis and prediction of the stock market is changing as per the time and situation. The practitioners and academician employ machine learning, deep learning, sentiment analysisĀ and algorithmic trading for portfolio formation. Both practitioners and academics deploy several other strategies, such as market movement prediction, feature decomposition, feature selection and model optimization to improve prediction accuracy. Features are the primary and crucial inputs for prediction models. The dynamic, noisy and non-stationary nature ofĀ data make models inaccurate. Therefore, it is crucial to preprocess the features before to entering them into the model. The features are decomposed using the Hodrick-Prescott filter, Baxter-King filter and Cristiano FitzGerald filter, empirical mode decomposition, Adaptive Noise and Multivariate Empirical Mode Decomposition.[25–28] The data normalized using the minimax scaler for better prediction accuracy.[27] The inclusion of significant features enhances the reliability of the model. Therefore, the selection of features plays a crucial role in the modelling of stock prices. There are diverse feature selection techniques to identify significant features in different research papers. A study utilized Chi-square based feature selection to determine the important features for the models.[21] It has been evident that the use of Genetic Algorithm to identify the optimal feature for the stock price prediction.[29] The application of Boruta feature selection technique to discover the key features for prediction models.[27] Further the recursive feature elimination to identify suitable features for prediction.[30] The Principal Component Analysis (PCA) and Least Absolute Shrinkage and Selection Operator (LASSO) have been employed to reduceĀ dimensionality of the feature space, as described by.[31] Analysts must carefully select the optimal parameter value of models to ensure that the model achieves improved accuracy and greater generalizability.[28] The application of Grid Search, Genetic Algorithm to determine the optimal parameter.[32,22] The use of AdamĀ AdadeltaĀ optimizers to determine the best parameters for their model.[21,33,34] In some research work the application of Fruit fly optimization technique to optimize the parameters.[35] Bayesian Optimizer is used to determine the optimal parameters in the stock price prediction model.[29] In most of the research papers it has been observed the frequent use of historical index and stock data to forecast the future movement of indexes and stocks. Previous scholars have employed fundamental, historical and technical indicatorsĀ in their research.[33] The research work of different scholars alsoĀ frequently leverage sentiment data obtained from news portals and social media platforms.[36] The financial ratios are used to measure the performance of the companies over the periods, like current ratio, quick ratio, debt ratio, debt-equity ratio, inventory turnover ratio, working capital turnover ratio, fixed assets turnover ratio and other ratios to Data Envelopment analysis (DEA) to select stocks for optimal portfolio making.[37] The application of event study to measure the impact of particular event on stock price. The appointment of an international CEO by a corporation has a substantial influence on the company’s stock.[38] The scholarly journals has used DCC, ADCC and GO-GARCH to model volatilities and conditional corelations between stock market, oil price, VIX and bond price and also analyzed the optimal hedging ratio exist among different assets class.[39] The hedging of the stock market of G-7 countries with WTI oil price and suggested investment diversification with GARCH-BEKK.[40]
This study gives comprehensive overview of stock market prediction and analysis. It covers stock prediction using econometrics, machine learning and deep learning. It also explores the stock analysis from different perspective including appointment of executive, political and geopolitical aspect of stock market, stock market hedging with other alternative assets like oil price, gold price. It also explores sentiment analysis, spillover effect, corporate information reporting and asymmetric, relationship between new energy and fossil fuel stock price. This piece of research helps the investors to understand and manage stock efficiently. It shows lights for future research and helps in identifying stock and take optimal portfolio decisions.
RESEARCH METHODOLOGY
Academic publications provide understanding of scholarly work in the given domain. Bibliometric analysis is commonly employed to examine the rate of growth and understanding of trends in given research disciplines in academia. Bibliometric analysis is a statistical technique used for analysing and measuring many aspects of scholarly publications. This study helps us understand how literature and information are being developed and shared in a specific field. It includes citation analysis, country analysis, country analysis, co-citation analysis, bibliometric linking quotation and co-word analysis for the key words. A bibliometric study aims to analyse and quantify the changes in research and identify patterns in academic literatures based on their citations in a certain field. It also seeks to uncover the trends in publication within a particular subject. The scopus database which provides profile articles for locating the highest number of citations for works related to stock market predictions and analysis. The analysis is performed with Vosviewer software and Biblioshiny package of R.[41]
Corpus Curation
The method of curating the corpus is illustrated in Figure 1.
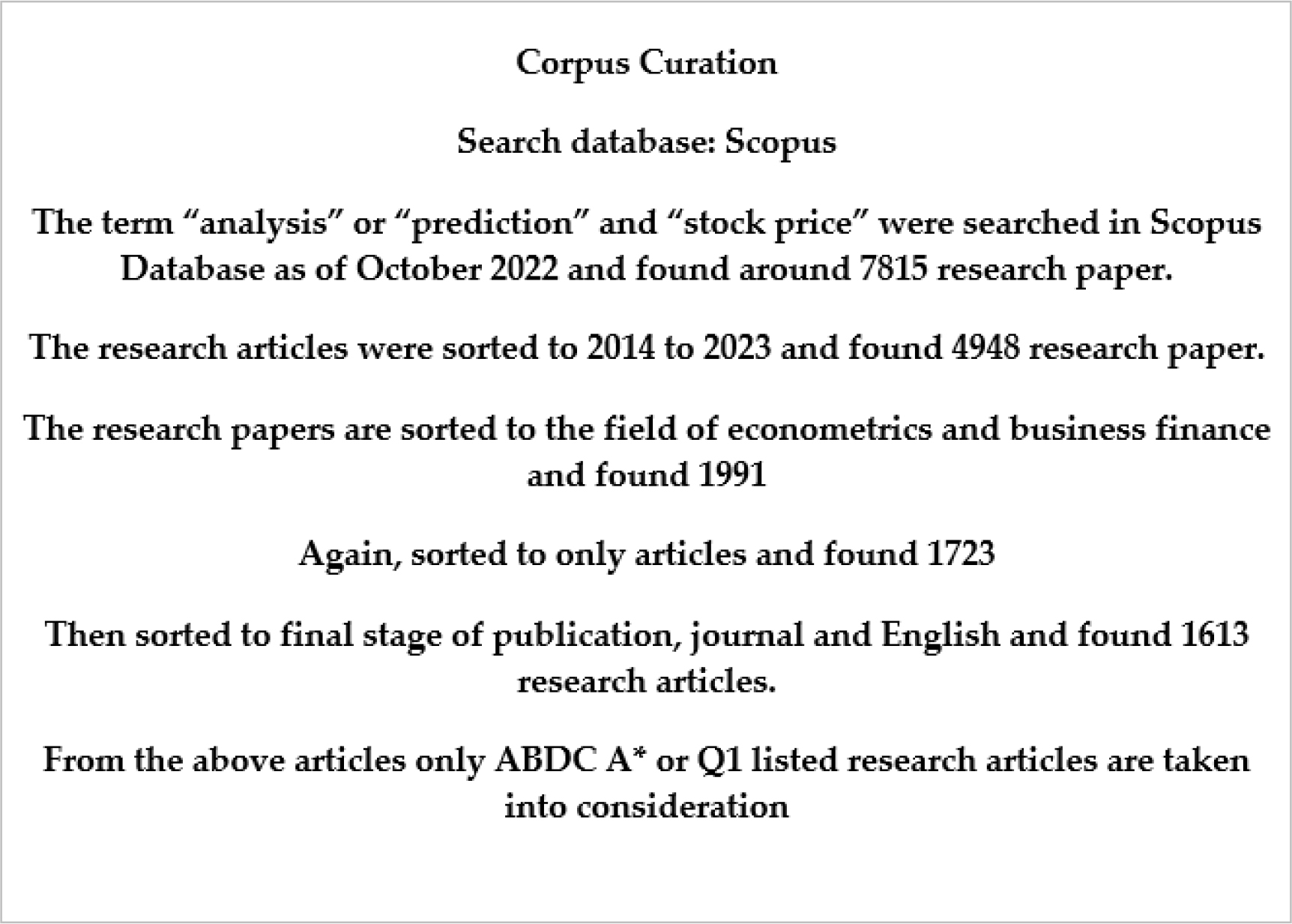
Figure 1:
Corpus Curation (Source: Authors).
In the above Figure 1 corpus the terminologies are actually taken into account based on the Scopus database and sorted to get the highlights of important aspects related to stock market analysis like variability of stocks, stockās price variation, organisation potential.
Corpus Analysis
Bibliometric Analysis consists of three main components: performance analysis, science mapping and enrichment analysis.[42] The Performance analysis and Scientific mapping constitutes main analysis and Visualization constitutes Enrichment analysis.Ā In order to conduct a performance analysis, we investigate the patterns of publication and citation in the given field. This analysis aims to answer two research questions: RQ1 focuses on top contributors in the field, while RQ2 examines the most influential research constituent in this field. This involves determining the articles that have the greatest influence, as well as the journals, authors, countries and institutions that produce the most and most impactful publications. Scientific mapping contains the intellectual and structural connection between research constituents. This analysis aims to [RQ3] examines on the emerging trend in the domain of stock prediction and analysis by forming clusters. The Enrichment analysis is addition to main analysis that gives deeper understanding of relationship between research constituents. This aims at [RQ4] visualize the relationship between key research constituents.
The Table 1 depicts the importance of citation relating to authors, institutions, journals as well as the countries. This study approaches both scientific mapping and enrichment analysis to understand citation, bibliographic coupling, co-word analysis and visualization.
Performance analysis |
Scientific mapping |
Enrichment analysis |
---|---|---|
Top Researchers |
Citation |
Clustering |
Top journal |
Co-Authorship |
Visualisation |
Top institution |
Co-word analysis |
|
Top countries |
Bibliographic Coupling |
PERFORMANCE ANALYSIS
Top Contributing Author Analysis
Here top contributing author analysis shows that Authors contribution in terms of number of research publication. Author with highest number of publications will top the list.
From the above Table 2 of top contributing authors, Li, Y topped the list with 3 publications and others have 2 each. Li, Y has authored three paper specially focused on environmental uncertainty due to fluctuation of oil price and stock market crash relationship, political risk, crude price and stock market relationship and social media coverage, financial reporting opacity and stock market relationship respectively. Li, Y more focused on stock market, macroeconomic variables and media reporting[14,15,43] respectively. These key terms not only shown in the Li yās paper but also are seen in other research paper with high occurrence.
Authors |
Papers |
Authors |
Papers |
---|---|---|---|
Li, Y |
3 |
Lee E |
2 |
Blau Bm |
2 |
Li J |
2 |
Breinlich H |
2 |
Li S |
2 |
Chen Y |
2 |
Proelss J |
2 |
Dutordoir M |
2 |
Reboredo Jc |
2 |
Guo Y |
2 |
Sadorsky P |
2 |
Gupta R |
2 |
Schmitt N |
2 |
Hendricks Kb |
2 |
Schneemeier J |
2 |
Hope O-K |
2 |
Schweizer D |
2 |
Hu J |
2 |
Singhal Vr |
2 |
Jiang W |
2 |
Tauchen G |
2 |
Kim J |
2 |
Todorov V |
2 |
Top Journal Analysis
The top journal analysis reveals the most article publishing journals in the given field by considering Number of Papers published by each journal. The journal publishing highest number of research papers in the given domain will be the top journal.
From the above Table 3, it is found that Journal of Energy Economy, Journal of Corporate Finance, Journal of Financial Economic and Journal of Management Science are encouraging more paper in this area of research as comparison to other journals. The journal of energy economics consistently publishing the papers the throughout the given data range and having maximum of 34 publications followed by Journal of Corporate Finance with 25 publications. Journal of Energy Economy specially focus on oil price, stock market, investment and price dynamic and others while the Journal of Corporate Finance focus on stock market crash risk, bad news hoarding, corporate governance, executive compensation and merger and acquisitions etc.
Sources of documents |
h_index |
g_index |
m_index |
NP |
TC |
Ave. Citations |
Total link strength |
---|---|---|---|---|---|---|---|
Energy Economics |
19 |
34 |
2.111 |
34 |
2010 |
59.11764706 |
5 |
Journal of Corporate Finance |
11 |
20 |
1.375 |
25 |
409 |
16.36 |
9 |
Journal of Financial Economics |
9 |
12 |
1 |
12 |
609 |
50.75 |
2 |
Management Science |
6 |
11 |
0.75 |
11 |
225 |
20.45454545 |
3 |
Review of Accounting Studies |
6 |
7 |
0.667 |
7 |
118 |
16.85714286 |
5 |
Contemporary Accounting Research |
5 |
7 |
1 |
7 |
144 |
20.57142857 |
1 |
Decision Support Systems |
4 |
5 |
0.444 |
5 |
197 |
39.4 |
2 |
Journal of Econometrics |
4 |
4 |
0.5 |
4 |
35 |
8.75 |
0 |
Journal of Economic Dynamics and Control |
4 |
6 |
0.444 |
6 |
64 |
10.66666667 |
0 |
Journal of Accounting Research |
3 |
6 |
0.375 |
6 |
270 |
45 |
0 |
Journal of Financial and Quantitative Analysis |
3 |
3 |
0.375 |
3 |
87 |
29 |
0 |
Journal of International Economics |
3 |
3 |
0.333 |
3 |
51 |
17 |
0 |
British Accounting Review |
2 |
2 |
0.5 |
2 |
17 |
8.5 |
0 |
Journal of Accounting and Economics |
2 |
3 |
0.286 |
3 |
36 |
12 |
0 |
Journal of Monetary Economics |
2 |
2 |
0.286 |
2 |
59 |
29.5 |
0 |
Strategic Management Journal |
2 |
2 |
0.5 |
2 |
56 |
28 |
0 |
American Economic Journal: Macroeconomics |
1 |
1 |
0.125 |
1 |
103 |
103 |
0 |
British Journal of Industrial Relations |
1 |
1 |
0.2 |
1 |
6 |
6 |
0 |
Critical Finance Review |
1 |
1 |
0.25 |
1 |
7 |
7 |
0 |
European Economic Review |
1 |
2 |
0.333 |
2 |
7 |
3.5 |
0 |
European Journal of Marketing |
1 |
1 |
0.2 |
1 |
16 |
16 |
0 |
Industrial Marketing Management |
1 |
1 |
0.333 |
1 |
51 |
51 |
0 |
Information Systems Research |
1 |
1 |
0.5 |
1 |
25 |
25 |
0 |
International Journal of Research in Marketing |
1 |
1 |
0.125 |
1 |
24 |
24 |
0 |
Journal of Construction Engineering and Management |
1 |
1 |
0.333 |
1 |
8 |
8 |
0 |
Top Contributing Institutions
The top institute analysis refers top institutions which are working proactively on stock price predictions and conducting the research in this field. The institutions involvements are ranked on the basis of number of authors from each institution.
From the above Table 4, it is found that Monash university from Australia, Central south university from China and Singapore management university have occupied top three positions respectively. The Monash university collectively worked upon four research articles specially focused upon role of media coverage in deterring firm from withholding of bad news, uses of non-parametric panel data model for crude oil and stock market prices. The Central south university has published 3 research articles in this domain and focused upon relationship between oil price uncertainty and informativeness and stock price crash risk respectively. The Table shows the list of top institution throughout the world, working in the given domain proactively.
Affiliation |
Articles |
Affiliation |
Articles |
---|---|---|---|
Monash university |
9 |
University of Manchester |
4 |
Central South University |
8 |
Utah state university |
4 |
Singapore management university |
6 |
California State University |
3 |
Concordia University |
4 |
Cardiff University |
3 |
Cornell University |
4 |
Central University of Finance and Economics |
3 |
Duke University |
4 |
Chinese University of Hong Kong |
3 |
Indiana University |
4 |
Cyprus University of Technology |
3 |
Renmin University of China |
4 |
Florida State University |
3 |
Scheller College Of Business |
4 |
Fuzhou University |
3 |
Southwestern University of Finance and Economics |
4 |
Hec Paris and Sando Center |
3 |
Ulster University |
4 |
Northwestern University |
3 |
University of Bamberg |
4 |
Southwest Jiao Tong University |
3 |
Top Country Analysis
The top country analysis refers to the highest contributing countries in term of number of research publications. Countries are ranked on basis of number of research article contribution from each country.
From the above Table 5, it is found that USA, UK and China have topped the list with 79, 27 and 22 publications respectively. The United States of America has been consistently working in the field throughout the given time frame. Author from united states have been able to influence the large number of researchers throughout the world. 46 countries are contributing in the given data frame but only 22 countries are shown in the list.
Country |
Documents |
Country |
Documents |
---|---|---|---|
United States |
79 |
Spain |
5 |
United Kingdom |
27 |
South Africa |
4 |
China |
22 |
Finland |
3 |
Canada |
20 |
India |
3 |
Australia |
15 |
New Zealand |
3 |
Hong Kong |
11 |
Brazil |
2 |
Germany |
10 |
Ghana |
2 |
South Korea |
10 |
Italy |
2 |
France |
9 |
Norway |
2 |
Netherlands |
5 |
Sweden |
2 |
Singapore |
5 |
Switzerland |
2 |
SCIENTIFIC MAPPING
Citation Analysis
Citation analysis consider number of citations obtained by a particular research constituent. Citation means number of times other research constituent mentioned your research constituent in their study. The Citation analysis is done to know about the most influential journal.[44] Citation analysis can be done of authors, publications, institutions and country and other research constituent. Citation is used for measuring the impact of research constituent in research community. The citation analysis creates a network research constituent connected with each other.
Author Citation
Authors citation analysis is conducted to know about significant authors in terms of citation of their research papers. The author with a greater number of total citation and average citation will top the list.
From Table 6, the author receiving highest citation is Sadorsky P. and followed by Reboredo J.C. and Ugolini A. The modelling of volatility and conditional correlation ship between emerging stock market price, oil price, copper price and wheat price using VARMA-AGARCH and DCC-AGARCH and GO-GARCH to model the volatility and conditional correlation ship among stock price, oil price, VIX, gold price and bond price.[39,45] Sadorsky P. more stressing on relationship and volatility aspect of stock market and other macroeconomic variables. Some scholars identifies the causality between oil price and renewable energy stock price.[46] The understanding of the impact of Quantile price change in oil, gas and electricity price on renewable energy stock return using multi-variate vine-copula dependency set-up is important part of stock market analysis.[17]
Author |
Documents |
Citations |
Total link strength |
Average citation |
---|---|---|---|---|
Sadorsky p. |
2 |
461 |
4 |
230 |
Reboredo j.c. |
2 |
279 |
33 |
139.5 |
Ugolini a. |
2 |
279 |
33 |
139.5 |
Basher s.a. |
1 |
267 |
4 |
267 |
Piotroski j.d. |
1 |
254 |
3 |
254 |
Wong t.j. |
1 |
254 |
3 |
254 |
Zhang t. |
1 |
254 |
3 |
254 |
Rivera-castro m.a. |
1 |
210 |
28 |
210 |
Ferrer r. |
1 |
198 |
14 |
198 |
JareƱo f. |
1 |
198 |
14 |
198 |
Source Citations
Source Citation reveals the number of times a particular journal got cited in this field of research. Average source citation also calculated to show the citations per documents. Further h-index, g-index and m-index were calculated to add some quality to the source citations. H-index reveals the largest value of h that h numbers of paper got at least h number of citations. G-index is a unique largest number that represents at least g number of paper have got g square citations[g^2]. M-index is used when there is a difference in career length. It assumes unbroken research activity since earliest publications.
From Table 3, The journal of energy economic has occupied the top position in terms of total citation of 2010 followed by journal of financial economic and Journal of Corporate Finance. American economic journal: macroeconomics has topped in term of average citation 103 and followed by energy economy and journal of financial economic. The energy economic covers the divers topic including volatility spillover, oil price uncertainty, stock price informativeness, clean energy, Climate adaption and migration, fossil fuel stock, carbon price, renewable energy, commodity market, merger and acquisition and hedging etc.[15,39,47–55] The journal of corporate finance also covers different significant topic including stock price fluctuation, anti-corruptions campaign, corporate governance, corporate litigation, shareholder litigation, bad news hoarding, share pledging and merger and acquisition etc.[56–61] The journal of financial economic focus on financial journalism, activist settlement, high frequency trading, political sensitive firm, insider trading, stock price manipulation and delta hedging etc.[62–68] the Management Science Journal focus on topic like assets pricing, 10-K filling etc.[69] other journals also focus on other different area.
Citation Analysis of Countries
Total citation and average citation analysis is carried out to know which country leading in this field. This analysis aims at to know the research impact of any country in the given domain.
From the above Table 7, it is found that USA has secured position one followed by Canada, China, Hongkong etc. The France has topped the list with average citation 59 followed by Hong Kong, Canada and Singapore respectively.
Country |
Number of papers |
Total citations |
Ave. Citation |
Total link strength |
---|---|---|---|---|
United states |
79 |
1886 |
23.87341772 |
30 |
Canada |
20 |
948 |
47.4 |
18 |
China |
22 |
775 |
35.22727273 |
28 |
Hong Kong |
11 |
610 |
55.45454545 |
17 |
Spain |
5 |
581 |
116.2 |
17 |
France |
9 |
532 |
59.11111111 |
10 |
United Kingdom |
27 |
472 |
17.48148148 |
22 |
South Korea |
10 |
407 |
40.7 |
8 |
Australia |
15 |
339 |
22.6 |
17 |
Singapore |
5 |
233 |
46.6 |
8 |
Germany |
10 |
154 |
15.4 |
4 |
Netherlands |
5 |
131 |
26.2 |
2 |
India |
3 |
108 |
36 |
8 |
South Africa |
4 |
96 |
24 |
4 |
New Zealand |
3 |
81 |
27 |
6 |
Finland |
3 |
27 |
9 |
5 |
Co-Authorship analysis
This analysis refers to research constituents work together on a particular research constituent. For example, two author worked together on a research paper. The objective of the analysis is to check the relationship among authors and their affiliations.
Co-authorship analysis of authors
Co-authorship analysis refers to two or more author worked together on particular research paper.
From the above Table 8, it is found that Li y has topped the list with co-authorship link 3. It means he has worked as co-author in three research articles. Both Li y and Chen y have worked with eight other researchers. The total link strength indicates with how many authors he or she has worked. Both Chen y and li y has worked with other 8 authors respectively. Li y worked upon financial reporting opacity, stock market crash, crude oil price and political risk in 3 research articles with 8 different authors.[15,70,71] Chen Y worked on sentiment analysis, information hoarding, CEO background Characteristics, disaster experience and stock price risk crash.[72,73]
Author |
No. Documents |
Citations |
Total link strength |
---|---|---|---|
Chen Y. |
2 |
73 |
8 |
Li Y. |
3 |
24 |
8 |
Jiang W. |
2 |
36 |
7 |
Uddin G.S. |
2 |
67 |
7 |
Guo Y. |
2 |
103 |
6 |
Li J. |
2 |
15 |
6 |
Tauchen G. |
2 |
19 |
6 |
Todorov V. |
2 |
19 |
6 |
Wen F. |
2 |
57 |
6 |
Gong Q. |
1 |
53 |
5 |
Hope o.-K. |
2 |
54 |
5 |
Lee E. |
2 |
0 |
5 |
Li Q. |
1 |
53 |
5 |
Lin Z. |
1 |
53 |
5 |
Proelss J. |
2 |
12 |
5 |
Co-Word Analysis
This analysis demonstrates the frequency at which terms occur together. A cluster of interconnected keywords is formed when certain keywords appear together. A study theme is determined based on the provided cluster. The VOSviewer program has been chosen for this analysis. The keywords selected are those that appeared a minimum of 8 times in the study. Twenty keywords have been identified following the screening process. The Table 9 displays a list of keywords and their respective occurrences. Keywords are the fundamental terms or concepts that form the central subject of a research work. The frequency of a keyword indicates how often it has been mentioned along with other significant terms inĀ research articles. This study facilitates the comprehension of the research community regarding the essential concepts that should be addressed and the one that should be freshly introduced. The total link refers to the number of additional keywords that are used in conjunction with a specific term. A total of 202 other keywords have been employed in conjunction with the term “financial markets.” A cluster is formed by the term and the keywords derived from its total link strength.
Keyword |
Occurrences |
total link strength |
keyword |
occurrences |
total link strength |
---|---|---|---|---|---|
Financial Markets |
44 |
202 |
United States |
10 |
64 |
Commerce |
35 |
174 |
Oil Trade |
8 |
62 |
Investments |
32 |
169 |
Energy Market |
9 |
59 |
Stock Market |
29 |
168 |
China |
13 |
58 |
Costs |
29 |
161 |
Oil Prices |
9 |
56 |
Stock Price |
21 |
117 |
Finance |
8 |
53 |
Price Dynamics |
13 |
95 |
Uncertainty Analysis |
9 |
45 |
Investment |
15 |
84 |
Regression Analysis |
10 |
40 |
Oil Supply |
11 |
74 |
Forecasting |
8 |
28 |
Crude Oil |
9 |
70 |
Stock Returns |
10 |
15 |
From the Table 9, it is found that the term financial markets have cooccurred highest number of times followed by commerce, investments, costs and stocks price and others. The term financial markets have highest number of total link strength followed by others.
Three-fold analysis of Author, Source and keywords
Three-fold analalysis measures the association of 20 authors, 20 keywords and 20 journal.
The Figure 2 Threefold Analysis of Authors, Sources and Documents showing associations of authors, keywords and journals in three-fold analysis of author, keywords and journals. Here 20 authors, 20 keywords and 20 journals are considered in the study. The journal of energy economic and journal of corporate analysis covers all 20 keywords. Authors are more focusing on stock price crash, oil price and crude oil price.
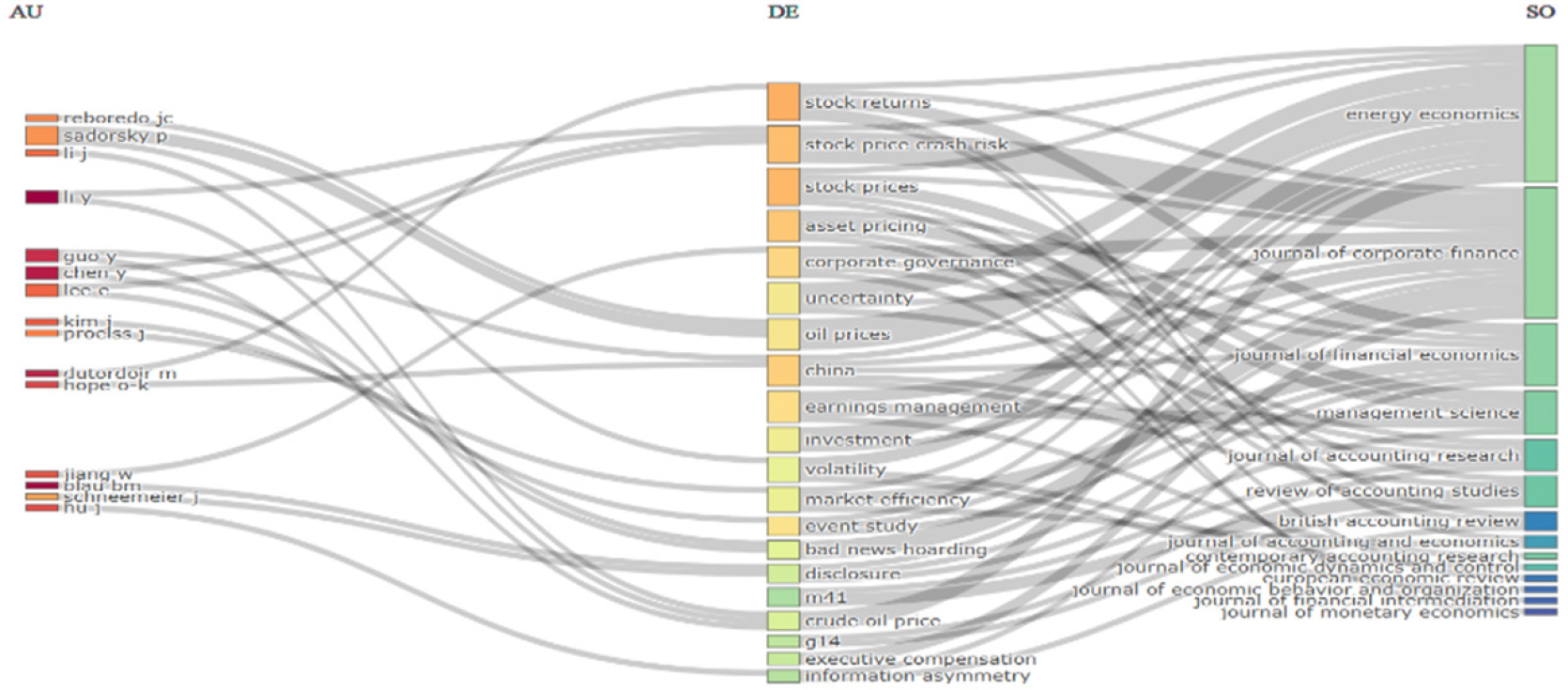
Figure 2:
Threefold Analysis of Authors, Sources and Documents.
Co-occurrence network of key words
Each keyword is represented by a circle indicating the number of connections associated with the keyword. The size of the circle is directly proportional to the number of associations the keyword possesses. The proximity of one circle to another denotes the degree of correlation between the two keywords and the colour of the circles represents the cluster to which they belong. The lines represent the connections that occur together between two keywords. The thickness of the line between the keywords increases as the frequency of their co-occurrence increases.
The above Figure 3 showing the co-occurrence analysis of a keywords. The VOS viewer program has been chosen for this analysis. The keywords selected are those that appeared a minimum of 8 times in the study. 20 keywords are selected following the screening process. There are 3 clusters on the basis of Keyword occurrence. The cluster 1 consists of 8 terms [red colour] which illustrate role of finance, financial market, commerce and costs, cluster 2 consists of 4 words (blue colour) which illustrate stock market and energy market and its allied terms and cluster 3 consists of 8 words (green colour) focusing on investments.
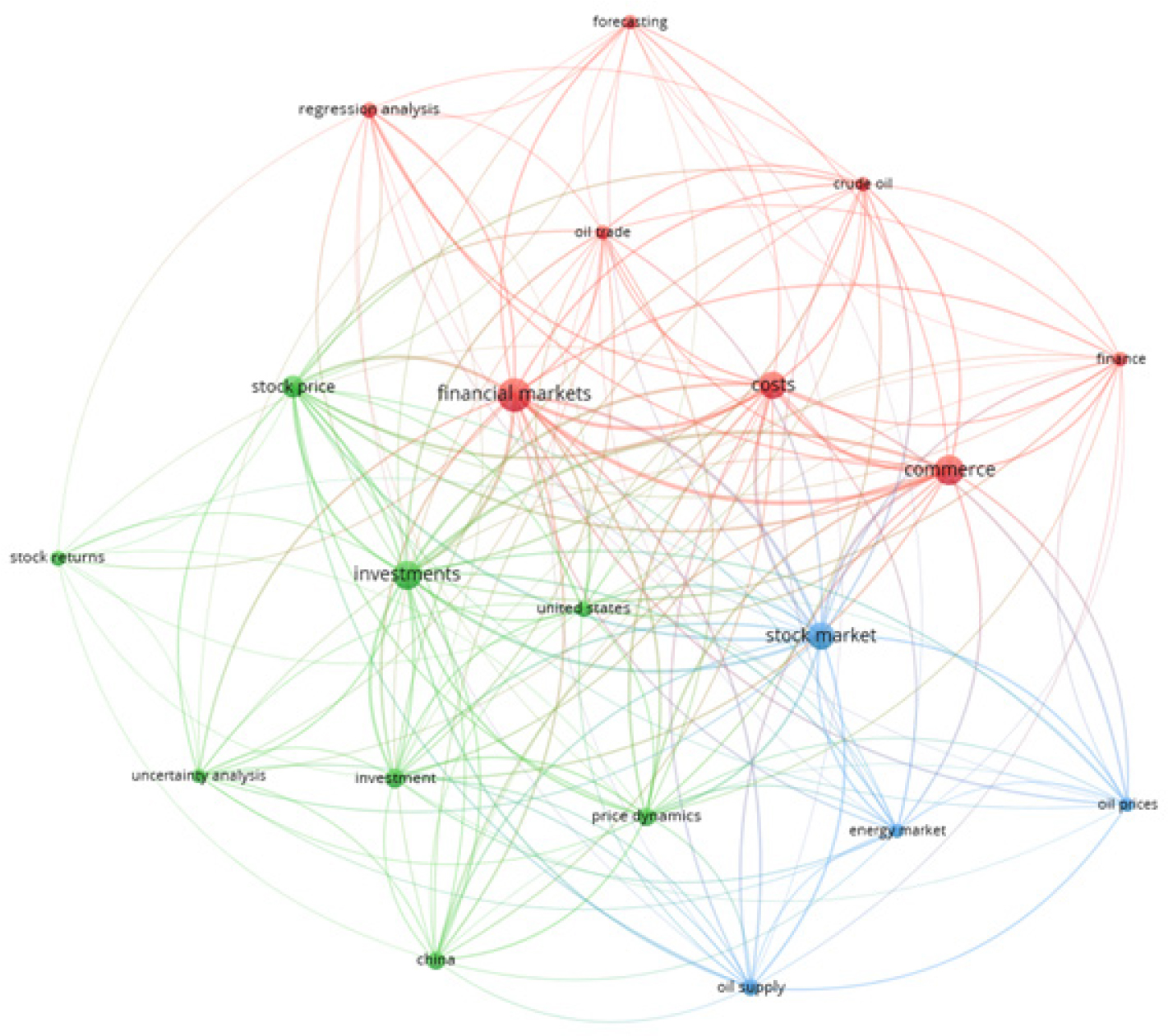
Figure 3:
Co-occurrence analysis of keywords (Source: VOSviewer).
Thematic Evolution of Keywords
The Figure 4 shows the entire data range was divided into four time slices of 2014-2016, 2017-2019, 2020-2021 and 2022-2023. The key word commerce was introduced in 2014 along with Stock market, investments, export.[74] Statistical tests and regression analysis were introduced in between 2017-2019. Crude oil, carbon and uncertainty analysis were introduced in between 2020-2021. Event though, no keyword has been introduced in 2022-2022 slice but work on financial market and uncertainty analysis is extended further.
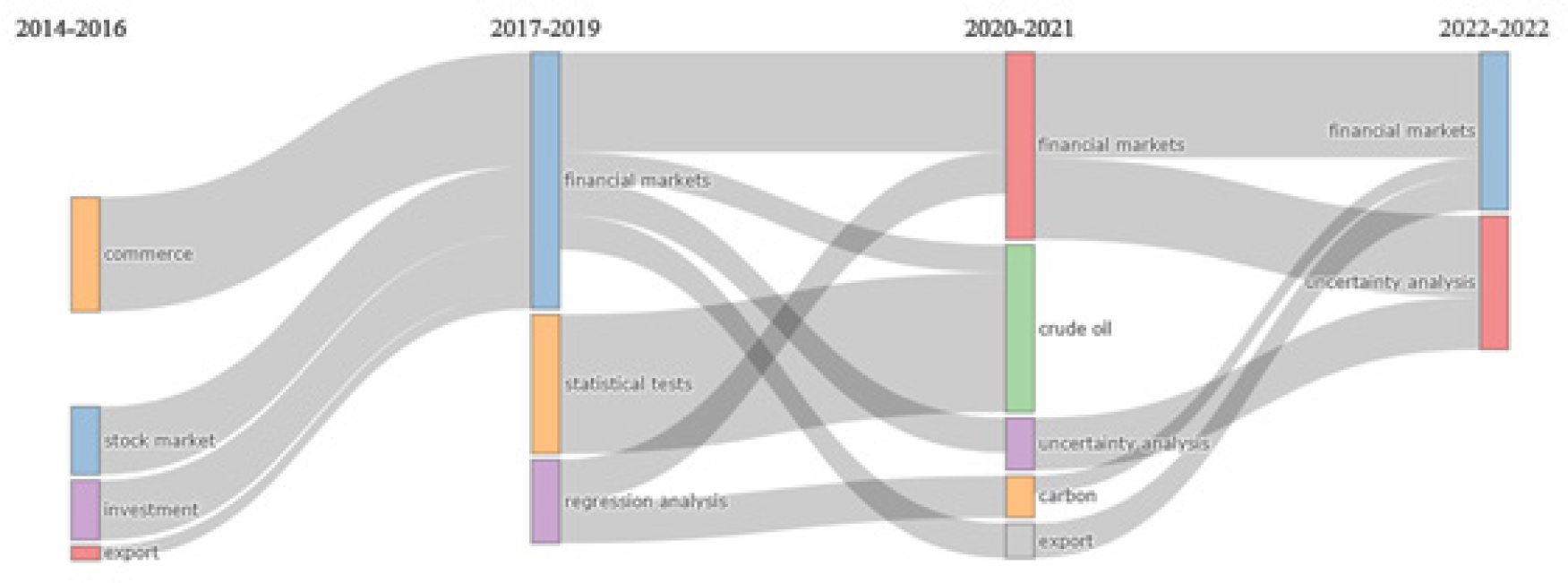
Figure 4:
Thematic evolution of keywords (Source: VOSviewer).
Bibliographic Coupling
Bibliographic coupling is technique used under scientific mapping that operates under the assumption that two research constituents share a common research constituent are similar in content. This analysis is used to identify the thematic cluster in the particular area of study in the given timeframe. It is very much useful for uncovering the latest development in this field
The Table 10 shows that the article by Chen y (2021) has created a huge network of 241 research article working on similar context followed by Bao d (2018). Li y has a network of 1776 author working of similar topic.
Documents |
Citations |
Total link strength |
Authors |
Number of Documents |
Total link strength |
---|---|---|---|---|---|
Chen Y. (2021) |
20 |
241 |
Li Y. |
3 |
1776 |
Bao D. (2018) |
22 |
210 |
Lee E. |
2 |
1588 |
Kim C. (2019) |
70 |
207 |
Chen Y. |
2 |
1472 |
Fang X. (2021) |
10 |
203 |
Li S. |
2 |
1307 |
Andreou Ck. (2021) |
10 |
202 |
Guo Y |
2 |
1117 |
An Z. (2020) |
32 |
198 |
Wang Q. |
2 |
1095 |
Silvapulla P. (2017) |
71 |
186 |
Weng F. |
2 |
1091 |
He G. (2015) |
54 |
184 |
Fan Q. |
1 |
1071 |
Kim J-B. (2022) |
0 |
180 |
Yang X. |
1 |
1071 |
Hu J. (2020) |
39 |
177 |
Zolotoy l. |
1 |
1071 |
ENRICHMENT ANALYSIS
Clustering analysis
Clustering basically involves with forming clusters/group on the basis of some criteria. This study considers bibliographic coupling network to from clusters. Research paper having more than 30 citations are considered for forming cluster. Each cluster is named on the basis their keywords. all papers from each cluster is given in Table 11.[75]
Cluster-1 market sentiment and appointments of executives |
||
---|---|---|
Blau BM, Delisle JR, Price SM |
Do sophisticated investors interpret earnings conference call tone differently than investors at large? Evidence from short sales. |
Disclosure; Earnings conference calls; Information processing; Linguistic analysis; Short selling. |
Conrad J, Wahal S, Xiang J |
High-frequency quoting, trading and the efficiency of prices. |
High frequency trading; Market efficiency; Market microstructure. |
Durand Rodolphe, Luc Paugam HS |
Do investors actually value sustainability indices? Replication, development and new evidence on CSR visibility. |
Analyst coverage; CSR; long-term investors; replication; stock market returns; sustainability. |
Feuerriegel S, Prendinger H |
News-based trading strategies. |
Decision support; Financial news; Sentiment analysis; Text mining; Trading simulation; Trading strategies. |
Griffin PA, Jaffe AM, Lont DH, Dominguez-Faus R |
Science and the stock market: Investors’ recognition of unburnable carbon. |
Event study; Media attention; Nature journal; Stranded assets; U.S. energy companies; Unburnable carbon. |
Hendricks KB, Hora M, Singhal VR |
An empirical investigation on the appointments of supply chain and operations management executives. |
Appointments of supply chain executives; Operating performance; Stock market value. |
Hu J |
Does option trading convey stock price information. |
Delta hedging; Information asymmetry; Options; Order flow; Price discovery. |
Hui KW, Nelson KK, Yeung PE |
On the persistence and pricing of industry-wide and firm-specific earnings, cash flows and accruals. |
Accruals; Cash flows; Earnings; Persistence; Stock returns. |
Kim S-H, Kim D |
Investor sentiment from internet message postings and the predictability of stock returns. |
Internet posting messages; Investor sentiment; Return predictability; Text classification; Trading volume; Volatility. |
Li Q, Wang T, Gong Q, Chen Y, Lin Z, Song S |
Media-aware quantitative trading based on public Web information. |
Sentiment analysis; Stock market; Trading strategy; Web information. |
Liu X |
Analysing the impact of user-generated content on B2B Firms’ stock performance: Big data analysis with machine learning methods. |
Big data; LDA; Machine learning; Sentiment analysis; User-generated content. |
Nam KH, Seong NY |
Financial news-based stock movement prediction using causality analysis of influence in the Korean stock market. |
Causal relationship; Multiple kernel learning; Stock movement prediction; Text mining; Transfer entropy. |
Sajko M, Boone C, Buyl T |
CEO Greed, Corporate Social Responsibility and Organizational Resilience to Systemic Shocks. |
Corporate social responsibility; event history analysis; executive compensation; panel and repeated-measure designs; research methods; top management teams/upper echelon. |
Schmid S, Dauth T |
Does internationalization make a difference? Stock market reaction to announcements of international top executive appointments. |
Corporate performance; Event study; Firm internationalization; Top management team; Top managers’ internationalization; Upper echelons. |
Shynkevich Y, McGinnity TM, Coleman SA, Belatreche A |
Forecasting movements of health-care stock prices based on different categories of news articles using multiple kernel learning. |
Decision support systems; Financial news; Multiple kernel learning; Stock price prediction; Text mining. |
Sueyoshi T, Wang D |
Radial and non-radial approaches for environmental assessment by Data Envelopment Analysis: Corporate sustainability and effective investment for technology innovation. |
Corporate sustainability; DEA; Energy; Environment; Investment; Technology innovation. |
Xiong G, Bharadwaj S |
Prerelease buzz evolution patterns and new product performance. |
Evolution pattern; Forecasting; Functional data analysis; New product sales; Prerelease buzz dynamics; Stock market value. |
Cluster-2 Corporate reporting and information availability |
||
An Z, Chen C, Naiker V, Wang J |
Does media coverage deter firms from withholding bad news? Evidence from stock price crash risk. |
Disciplinary role; Informational role; Media coverage; Stock Price crash risk. |
Cao X, Wang Y, Zhou S |
Anti-corruption campaigns and corporate information release in China. |
Anti-corruption campaign; CCDI; China; Corporate governance; Event study; Information release. |
Hope O-K, Wu H, Zhao W |
Block holder Exit Threats and Financial Reporting Quality. |
Block holders; exit theory; financial reporting quality; liquidity; wealth-performance sensitivity. |
Edmans A, Jayaraman S, Schneemeier J |
The source of information in prices and investment-price sensitivity. |
Financial efficiency; Insider trading; Real effects of financial markets; Real efficiency. |
Eun CS, Wang L, Xiao SC |
Culture and R2. |
Culture; G02; G12; G15; Individualism; Openness; R2; Tightness. |
He Guanming |
The effect of CEO inside debt holdings on financial reporting quality. |
Abnormal accruals; Accruals quality; CEO inside debt holdings; Earnings misstatement; Earnings target beating. |
Kim CF, Deangelis M, Griffin P, Gu Z, Oler D, Park CW |
Readability of 10-K Reports and Stock Price Crash Risk. |
Readability; textual analysis; crash risk; SOX; 10-K. |
Li S, Zhan X |
Product market threats and stock crash risk. |
Market competition; Product market threats; Stock crash risk. |
Muslu V, Radhakrishnan S, Subramanyam KR, Lim D |
Forward-looking MD&A disclosures and the information environment. |
10-K filings; Disclosure; Forward looking. |
Piotroski JD, Wong TJ, Zhang T |
Political incentives to suppress negative information: Evidence from Chinese listed firms. |
China; Corporate governance; Information environment; Political costs; State ownership. |
Wagner AF, Zeckhauser RJ, Ziegler A |
Company stock price reactions to the 2016 election shock: Trump, taxes and trade. |
Corporate interest payments; Corporate taxes; Election surprise; Event study; Market efficiency; post-news drift; Price contribution analysis; Stock returns; Trade policy. |
Yuan R, Sun J, Cao F |
Directors’ and officers’ liability insurance and stock price crash risk. |
Corporate governance; Corporate social responsibility; Directors’ and officers’ liability insurance; Financial restatements; Stock price crash risk. |
Cluster-3 Stock market and clean energy |
||
Bhattarai S, Chatterjee A, Park WY |
Global spillover effects of US uncertainty. |
Emerging market economies; Emerging market monetary policy minutes; Monetary policy response; Panel VAR; US Uncertainty. |
Demiralay S, Kilincarslan E |
The impact of geopolitical risks on travel and leisure stocks. |
Geopolitical risks; Quantile regressions; Stock returns; Travel and leisure industry. |
Ferrer R, Shahzad SJH, López R, Jareño F |
Time and frequency dynamics of connectedness between renewable energy stocks and crude oil prices. |
Connectedness; Crude oil price; Information transmission; Renewable energy stocks; Time-frequency space. |
Liu LX, Shu H, Wei KCJ |
The impacts of political uncertainty on asset prices: Evidence from the Bo scandal in China. |
Cash flow news; Discount rate news; Political uncertainty; Politically sensitive firms; Stock returns. |
Lundgren AI, Milicevic A, Salah G, Hoon S |
Connectedness network and dependence structure mechanism in green investments. |
Causality; Connectedness network; Non-renewable energy; Renewable energy; Uncertainty |
Reboredo JC, Rivera-Castro MA, Ugolini A |
Wavelet-based test of co-movement and causality between oil and renewable energy stock prices. |
Causality; Oil prices; Renewable energy; Wavelet coherence; Wavelets. |
Reboredo JC, Ugolini A |
The impact of energy prices on clean energy stock prices. A multivariate quantile dependence approach. |
Clean energy stock price returns; copulas; Energy prices. |
Wen F, Zhao L, He S, Yang G |
Asymmetric relationship between carbon emission trading market and stock market: Evidences from China. |
Asymmetric relationship; Carbon emission trading market; China stock market; NARDL model. |
Wen X, Guo Y, Wei Y, Huang D |
How do the stock prices of new energy and fossil fuel companies correlate? Evidence from China. |
Asymmetric BEKK; China; Fossil fuel stocks; Market spillovers; New energy stocks. |
Cluster-4 Dynamic interaction between Oil and stock market |
||
Lean HH, Badeeb RA |
Asymmetric impact of oil price on Islamic sectoral stocks. |
Islamic stocks; Non-linear ARDL; Oil price; Sectoral. |
Balcilar M, Gupta R, Wohar ME |
Common cycles and common trends in the stock and oil markets: Evidence from more than 150Ā years of data. |
Common cycles; Common features; Oil market; Permanent and transitory shocks; Stock market; Trend-cycle decomposition. |
Gambetti L |
The effects of monetary policy on stock market bubbles: Some evidence. |
Not Available. |
Gupta R, Wohar M |
Forecasting oil and stock returns with a Qual VAR using over 150Ā years off data. |
Business cycle turning points; Forecasting; Oil and stock prices; Vector autoregressions. |
Jiang Z, Yoon S-M |
Dynamic co-movement between oil and stock markets in oil-importing and oil-exporting countries: Two types of wavelet analysis. |
Co-movement; Lead-lag relationship; Wavelet coherence; Wavelet multi-scale decomposition; Wavelet-based Granger causality test. |
Le T-H, Chang Y |
Effects of oil price shocks on the stock market performance: Do nature of shocks and economies matter? |
Gregory-Hansen co-integration test; Oil price fluctuations; Stock market returns; Toda-Yamamoto Granger non-causality test. |
Lin B, Wesseh PK, Appiah MO |
Oil price fluctuation, volatility spillover and the Ghanaian equity market: Implication for portfolio management and hedging effectiveness. |
Hedge ratios; Oil prices; Stock market return; Volatility. |
Silvapulle P, Smyth R, Zhang X, Fenech J-P |
Nonparametric panel data model for crude oil and stock market prices in net oil importing countries. |
Local linear estimator; Panel data analysis; Time-varying coefficient functions; Time-varying trend functions. |
Cluster-5 Hedging strategies and Volatilities Spillover |
||
Basher SA, Sadorsky P |
Hedging emerging market stock prices with oil, gold, VIX and bonds: A comparison between DCC, ADCC and GO-GARCH. |
DCC-GARCH; Emerging market stock prices; GO-GARCH; Hedging; Oil prices. |
Khalfaoui R, Boutahar M, Boubaker H |
Analyzing volatility spillovers and hedging between oil and stock markets: Evidence from wavelet analysis. |
BEKK-GARCH; Crude oil prices; Discrete wavelet analysis; Hedge ratio; Stock prices; Volatility spillovers; Wavelet coherence. |
Sadorsky P |
Modeling volatility and correlations between emerging market stock prices and the prices of copper, oil and wheat. |
Emerging markets; Multivariate GARCH; Oil prices; Volatility. |
Cluster-1 Market sentiment and event study
The sentiments of the stakeholders have significant impact on stock price of the companies. Measuring the sentiments of stakeholders is called as sentiment analysis. The sentiment is measured with help of natural language processing.[76] The sentiment may be of normal course business, change policy companies and economy and others. The sentiment analysis takes data from social media site, financial news, microblogging site.[77–79] These textual data were cleaned and used as feature in the sentiment analysis. The sentiment analysis involves with tokenization, normalization, stop word removal, lemmatization, bag of words, TF-IDF and assigning polarity and subjectivity score. The polarity and subjectivity score are used to predict the movement of stock. The reaction of stakeholders can be also measured through the event study. The event study measures the impact of policy change or change in management on stock prices. it considers pre-event performance and post event performance of the companies to see the significant statistical difference. The measurement of the reaction of investors to appointment of supply chain executive is crucial for stock analysis.[4] The impact of investors sentiment/reaction reflected in stock price change due to appointment of international Chief Executive Officers.[80] The stock market has significant influenced by un-burnable carbon.[81]
Cluster-2 Corporate Reporting
The information and its reporting by organisation and its stakeholders are key for investment and trading decision making. Mis-management of information by any party goes against the shareholders. Most of the studies has stressed on the surpassing of negative informationās by politicians and their affiliated firms for the incentives of political and non-political events.[82] In id evident from the literature that Corporates also uses complex statement in K-10 report to hide the adverse news, which in turn increases the crash risk of stock price.[83] The informational efficiency of stocks are sometime less. To overcome the same, the corporations uses greater level of forward-looking Management discussion and Analysis section of K-10 report.[84] Media is the vital medium of transmitting the information. Research studies have found that there is a negative relationship between higher media coverage and stock price crash risk.[5] The chief executive officers insider debt holding also promotes the high quality financial reporting.[85] Management sometimes uses high quality reporting to avoid the exit threat of block holders.[86]
Cluster-3 Stock market and clean energy
The raising transaction between countries making global economic more interconnected. The shock in a particular economic can be felt across the borders. The negative spillover impact of US uncertainty shock on stock, exchange rate, country spread of emerging economics of the world.[87] The effect of the shock of one market can be felt on other market of that economic and markets of other countries also. The enablers like Financial stress, VIX, EU_EPU, STOXX600 and Wilder Hill clean energy index are net transmitter of volatilities whereas US_EPU, USD/EUR, ERIX, S&P500, S&PGEC and treasury bill are net receiver of volatilities.[88] The outcome of the studies are relating to the greater Return and volatilities connectedness among clean energy stock and stock market in short run after Sub-prime Crises 2007.[89] It has been found for the studies that linear bidirectional causality among oil price and renewable stock price at lower frequency and also found non-linear unidirectional causality from renewable energy stocks to oil price.[53] The new energy and fossil fuel stock returns spills over into variances of their counter assets.[90] The relationship between carbon emission trading market and overall stock market is significantly negative in short run as well as long run in China.[91]
Cluster-4 Dynamic interaction between Oil and stock market
Oil is the energy of every economic. Interaction oil price with stock market varies on the basis of economic and time periods. The oil price and stock share a common stochastic trend since 1859 but a common cycle post-world war II.[92] There is significant difference in price on stock price in different periods and different places.[93] The oil price shocks positive affects the stock performance in different sub-sample period in oil exporting countries. The oil price shocks affect stock price negatively of oil importing countries and oil refinery countries. There are ssectors specific impacts of the oil price shocks vary across the sectors of Islamic composite Indexes even though a very weak correlation exist between the oil price and Islamic composite index. Healthcare, consumer service, finance service and telecommunications have been suggested by researcher as alternative to oil price shock.[94] The oil price shock affects stock performance of oil exporting countries more than oil importing countries.[95]
Cluster-5 Hedging strategies and volatilities spillover
The stock market is impacted by different markets including oil market, commodity market, renewable stock market, carbon market and gold market etc. These markets share some kind of relationship with stock market. To protect the investment, the opposite trading is carried out in other assets markets. The optimal hedge ratio varies in different time horizon. The stocks in G-7 countries are more volatile than WTI oil price.[40] The hedging of the stock price of emerging market has been done with oil, VIX, bond and gold using DCC, ADCC and GO-GARCH.[96] The oil is the most effective assets to hedge stocks of emerging market using optimal hedging ratio from ADCC. The Gold is the most expensive hedging using GO-GARCH. DCC-AGARCH is most effective for hedging emerging stock price. The cheapest hedging assets is oil followed by Copper and Wheat against Emerging market stock price.[45]
Research directions- Some of the research gaps suggested by authors in their papers
- The channels and mechanism used by politician and affiliated firm to temporary surpass the flow of negative information into stock market.[97]
- How does the market actually price politically driven information risk.[97]
- How does the market interpret the silence during the politicalized period and what is the impact on realized return over these window.[97]
- Differentiation of the impact on the change of oil price on Islamic stock exporting and importing countries.[94]
- Investigating the non-dependence and connectedness network of all world region among green investment, conventional investment and uncertainty.[88]
- B2B stock analysis using textual data from Facebook, amazon, yelp and other.[98]
- Using of photo, video, virtual reality to analyse the stocks.[98]
- mproved measure of managerial bad news hoarding.[5]
- Comprehensive analysis of emerging market economies central bank minutes to capture the fear of capital flow.[87]
- The sensitivity of geopolitical events using company level data across different countries.[99]
- Effect of interest rate change on assets pricing bubbles.[100]
CONCLUSION AND CONTRIBUTIONS
The subject of business resilience has greater impact to overcome shocks in business to achieve the desirable business.[101] Further Research in the domain of stock market is dynamic in nature. As of the given duration, Li Y, has published highest number of articles even though Sadorsky p. has highest number of average citations per articles. Li y and Chen Y have worked with other eight authors in the given time frame followed be Jiang W. The journal of Energy Economy is publishing highest number of articles followed by Journal of Corporate Finance and Journal of Financial Economic. The journal of Energy Economic is also most influential journal with average citation of 59 followed by journal of financial Economic and journal of Econometrics with average citation of 50 and 39 respectively. USA, UK and China are the largest producer of research articles. France, Hong Kong and Canada are most influential journals in terms of citations. The most occurred keywords are financial markets, commerce and investments. These keywords have highest number of association (total link strength) with other keywords. From three-fold analysis, it is clear that journal of Energy Economy is associated with 20 most occurred keywords in the study followed by journal of corporate finance. There are 5 key cluster/theme found on the basis of bibliometrics coupling. The Cluster 1- focus on measuring sentiments stakeholders and reaction of investors to significant events. The data for sentiment analysis were collected from news articles, social media post and microblogging site etc. A pre and post event comparative analysis is conducted to measure the impact of particular event. The Cluster-2 focus on financial reporting. In this cluster, researchers have measured the impact of news hoarding, regular media coverage, readability of K-10 reports etc on stock price. The Cluster-3 focus on stock market and clean energy stock relationship. In this section, the volatilities spillover and causality of stock market, oil market and renewable energy market. The Cluster-4 focus on Oil market and stock market relationship. This cluster covers the relationship between oil market and stock market of different countries, including oil importing Countries, oil exporting countries and oil refining countries. The Cluster-5 focus on hedging and diversifying investment. This cluster also focus on which model is best for reaching optimal hedging ratio, which are the cheap hedging assts and which most expensive hedging assets etc.
References
- Abu-mostafa YS, Atiya AF.. Introduction to financial forecasting. Appl Intell.. 1996;6(3):205-13. [CrossRef] | [Google Scholar]
- Zhong X, Enke D.. Forecasting daily stock market return using dimensionality reduction. Expert Syst Appl [Internet].. 2017;67:126-39. [CrossRef] | [Google Scholar]
- Hu J, Li S, Taboada AG, Zhang F.. Corporate board reforms around the world and stock price crash risk. J Corp Finan [Internet].. 2020;62:101557 [CrossRef] | [Google Scholar]
- Hendricks KB, Hora M, Singhal VR.. An empirical investigation on the appointments of supply chain and operations management executives. Manag Sci.. 2015;61(7):1562-83. [CrossRef] | [Google Scholar]
- An Z, Chen C, Naiker V, Wang J.. Does media coverage deter firms from withholding bad news? Evidence from stock price crash risk. J Corp Finan [Internet].. 2020:64 [CrossRef] | [Google Scholar]
- Shen K, Tzeng G.. Combined soft computing model for value stock selection based on fundamental analysis. Appl Soft Comput J [Internet].. 2015:1-14. [CrossRef] | [Google Scholar]
- Prasad TD, Kumar AT.. A study on stockā S volatility in banking sector using technical analysis. Int Res J Eng Technol.. 2018:1016-22. [CrossRef] | [Google Scholar]
- Fama EF.. The behavior of stock-market prices. J Bus.. 1965;38(1):34-105. [CrossRef] | [Google Scholar]
- AW Lo A, MacKinlay AC.. A nonrandom walk down Wall Street;. 1999 [CrossRef] | [Google Scholar]
- Shah D, Isah H.. Stock market analysis: a review and taxonomy of prediction techniques. 2019;ii [CrossRef] | [Google Scholar]
- Zhou YY, Liu CZ, Tang L.. Empirical analysis of influences of fictitious economy on real economy in China Int Conf Logist Syst Intell Manag ICLSIM 2010. 2010;Vol. 1(1):305-9. [CrossRef] | [Google Scholar]
- Appiah-otoo I, Appiah-otoo I.. A bibliometric assessment of the finance-growth literature: current status, development and future direction. J Scientometr Res.. 2023;12(2):321-31. [CrossRef] | [Google Scholar]
- Caporale GM, Gil-alana LA, You K, Caporale GM.. Stock Market Linkages between the Asean Countries, China and the US: A Fractional Integration / cointegration Approach. Emerg Mark Financ Trade [Internet].. 2021;00(00):1-14. [CrossRef] | [Google Scholar]
- Guo Y, Li J, Li Y, You W.. The roles of political risk and crude oil in stock market based on quantile cointegration approach: A comparative study in China and US. Energy Econ [Internet].. 2021;97:105198 [CrossRef] | [Google Scholar]
- Xiao J, Chen X, Li Y, Wen F.. Oil price uncertainty and stock price crash risk: evidence from China. Energy Econ [Internet].. 2022;112:106118 [CrossRef] | [Google Scholar]
- Yahya M, Kanjilal K, Dutta A, Uddin GS, Ghosh S.. Can clean energy stock price rule oil price? New evidences from a regime-switching model at fi rst and second moments. Energy Econ [Internet].. 2021;95:105116 [CrossRef] | [Google Scholar]
- Reboredo JC, Ugolini A.. The impact of energy prices on clean energy stock prices. A multivariate quantile dependence approach. Energy Econ [Internet].. 2018;76:136-52. [CrossRef] | [Google Scholar]
- Wong JB, Zhang Q.. Br Account Rev [Internet].. 2022;Vol. 54(1):101045 [Jul 3 2024]. Stock market reactions to adverse {ESG} disclosure via media channels.
- Houssein EH, Dirar M, Abualigah L, Mohamed WM.. An efficient equilibrium optimizer with support vector regression for stock market prediction [Internet]. Neural Comput Appl.. 2022;34(4):3165-200. [CrossRef] | [Google Scholar]
- Nourbakhsh Z, Habibi N.. Combining LSTM and CNN methods and fundamental analysis for stock price trend prediction. Multimed Tools Appl.. 2023;82(12):17769-99. [CrossRef] | [Google Scholar]
- Gunduz H, Yaslan Y, Cataltepe Z.. Intraday prediction of Borsa Istanbul using convolutional neural networks and feature correlations. Knowl Based Syst [Internet].. 2017;137:138-48. [CrossRef] | [Google Scholar]
- Jujie Wang DQ.. An experimental investigation of two hybrid frameworks for stock index prediction using neural network and support vector regression. Econ Comput Econ Cybern Stud Res.. 2018;52(4):193-210. [CrossRef] | [Google Scholar]
- Jing N, Wu Z, Wang H.. A hybrid model integrating deep learning with investor sentiment analysis for stock price prediction. Expert Syst Appl [Internet].. 2021;178(May 2020):115019 [CrossRef] | [Google Scholar]
- CorberÔn-vallet A, Vercher E, Segura JV, Bermúdez JD.. A new approach to portfolio selection based on forecasting. Expert Syst Appl [Internet].. 2023;215(August 2022):119370 [CrossRef] | [Google Scholar]
- Ouahilal M, El MM, Chahhou M, El MBE.. A novel hybrid model based on Hodrick-Prescott filter and support vector regression algorithm for optimizing stock market price prediction. J Big Data.. 2017;4(1):1-22. [CrossRef] | [Google Scholar]
- Jin Z, Yang Y, Liu Y.. Stock closing price prediction based on sentiment analysis and LSTM. Neural Comput Appl [Internet].. 2020;32(13):9713-29. [CrossRef] | [Google Scholar]
- Li J, Liu Y, Gong H, Huang X.. Stock price series forecasting using multi-scale modeling with boruta feature selection and adaptive denoising. Appl Soft Comput [Internet].. 2024;154(Feb):111365 [CrossRef] | [Google Scholar]
- Yao Y, Zhang Z, Zhao Y.. Stock index forecasting based on multivariate empirical mode decomposition and temporal convolutional networks. Appl Soft Comput [Internet].. 2023;142:110356 [CrossRef] | [Google Scholar]
- Yun KK, Yoon SW, Won D.. Prediction of stock price direction using a hybrid GA-XGBoost algorithm with a three-stage feature engineering process. Expert Syst Appl [Internet].. 2021;186(Mar):115716 [CrossRef] | [Google Scholar]
- Wei X, Tian Y, Li N, Peng H.. Evaluating ensemble learning techniques for stock index trend prediction: a case of China. Port Econ J.. 2024;23(3):505-30. [CrossRef] | [Google Scholar]
- Gao Y, Wang R, Zhou E.. Stock prediction based on optimized LSTM and GRU models [sci. program]. Scientific Programming.. 2021;2021:1-8. [CrossRef] | [Google Scholar]
- Beniwal M, Singh A, Kumar N.. Forecasting long-term stock prices of global indices: A forward-validating genetic algorithm optimization approach for Support Vector Regression. Appl Soft Comput [Internet].. 2023;145:110566 [CrossRef] | [Google Scholar]
- Bhandari HN, Rimal B, Pokhrel NR, Rimal R, Dahal KR, Khatri RK., et al. Predicting stock market index using LSTM. Mach Learn Appl [Internet].. 2022;9(May):100320 [CrossRef] | [Google Scholar]
- Beniwal M, Singh A, Kumar N.. Forecasting multistep daily stock prices for long-term investment decisions: A study of deep learning models on global indices. Eng Appl Artif Intell [Internet].. 2024;129(June 2023):107617 [CrossRef] | [Google Scholar]
- Gowthul Alam MM, Baulkani S.. Local and global characteristics-based kernel hybridization to increase optimal support vector machine performance for stock market prediction. Knowl Inf Syst [Internet].. 2019;60(2):971-1000. [CrossRef] | [Google Scholar]
- Picasso A, Merello S, Ma Y, Oneto L, Cambria E.. Technical analysis and sentiment embeddings for market trend prediction. Expert Syst Appl [Internet].. 2019;135:60-70. [CrossRef] | [Google Scholar]
- Mohseny-Tonekabony NM, Sadjadi SJ, Mohammadi E, Tamiz M, Jones DF.. Robust, extended goal programming with uncertainty sets: an application to a multi-objective portfolio selection problem leveraging DEA. Ann Oper Res [Internet].. 2024 [CrossRef] | [Google Scholar]
- Schmid S, Dauth T.. Does internationalization make a difference? Stock market reaction to announcements of international top executive appointments. J World Bus [Internet].. 2014;49(1):63-77. [CrossRef] | [Google Scholar]
- Basher SA, Sadorsky P.. Hedging emerging market stock prices with oil, gold, VIX and bonds: A comparison between DCC, ADCC and GO-GARCH. Energy Econ [Internet].. 2016;54:235-47. [CrossRef] | [Google Scholar]
- Khalfaoui R, Boutahar M, Boubaker H.. Analyzing volatility spillovers and hedging between oil and stock markets: evidence from wavelet analysis. Energy Econ [Internet].. 2015;49:540-9. [CrossRef] | [Google Scholar]
- Tahiru F, Parbanath S, Agbesi S.. Machine learning-based predictive systems in higher education: A bibliometric analysis. J Scientometr Res.. 2023;12(2):436-47. [CrossRef] | [Google Scholar]
- Donthu N, Kumar S, Mukherjee D, Pandey N, Lim WM.. How to conduct a bibliometric analysis: an overview and guidelines. J Bus Res [Internet].. 2021;133(May):285-96. [CrossRef] | [Google Scholar]
- Ding R, Zhou H, Li Y.. Social media, financial reporting opacity and return comovement: evidence from Seeking Alpha. J Financ Mark [Internet].. 2019:100511 [CrossRef] | [Google Scholar]
- Podsakoff PM, Mackenzie SB, Bachrach DG, Podsakoff NP.. The influence of management journals in the 1980s and 1990s. Strateg Manag J.. Array;488(5):473-88. [CrossRef] | [Google Scholar]
- Sadorsky P.. Modeling volatility and correlations between emerging market stock prices and the prices of copper, oil and wheat. Energy Econ [Internet].. 2014;43:72-81. [CrossRef] | [Google Scholar]
- Reboredo JC, Rivera-castro MA, Ugolini A.. Wavelet-based test of co-movement and causality between oil and renewable energy stock prices. Energy Econ [Internet].. 2017;61:241-52. [CrossRef] | [Google Scholar]
- Sarwar S, Shahbaz M, Anwar A, Tiwari AK.. The importance of oil assets for portfolio optimization: the analysis of firm level stocks. Energy Econ [Internet].. 2019;78:217-34. [CrossRef] | [Google Scholar]
- Proelss J, Schweizer D, Seiler V.. Do announcements of WTO dispute resolution cases matter? Evidence from the rare earth elements market. Energy Econ [Internet].. 2018;73:1-23. [CrossRef] | [Google Scholar]
- Pham L, Hao W, Truong H, Trinh HH.. The impact of climate policy on U.S. environmentally friendly firms: A firm-level examination of stock return, volatility, volume and connectedness. Energy Econ [Internet].. 2023;119(Feb):106564 [CrossRef] | [Google Scholar]
- Fahmy H.. The rise in investorsā awareness of climate risks after the {Paris} {Agreement} and the clean energy-oil-technology prices nexus. Energy Econ [Internet].. 2022;106:105738 [CrossRef] | [Google Scholar]
- Wen X, Guo Y, Wei Y, Huang D.. How do the stock prices of new energy and fossil fuel companies correlate? Evidence from China {Evidence} from {China}. Energy Econ [Internet].. 2014;41:63-75. [CrossRef] | [Google Scholar]
- Chun D, Cho H, Kim J.. The relationship between carbon-intensive fuel and renewable energy stock prices under the emissions trading system. Energy Econ [Internet].. 2022;114:106257 [CrossRef] | [Google Scholar]
- Reboredo JC, Rivera-Castro MA, Ugolini A.. Wavelet-based test of co-movement and causality between oil and renewable energy stock prices. Energy Econ [Internet].. 2017;61:241-52. [CrossRef] | [Google Scholar]
- Badshah I, Demirer R, Suleman MT.. The effect of economic policy uncertainty on stock-commodity correlations and its implications on optimal hedging. Energy Econ [Internet].. 2019;84:104553 [CrossRef] | [Google Scholar]
- Zhu L, Shen J, Yeerken A.. Impact analysis of mergers and acquisitions on the performance of Chinaās new energy industries. Energy Econ [Internet].. 2024;129(July 2023):107189 [CrossRef] | [Google Scholar]
- Liu R, Si H, Miao M.. One false step can make a great difference: does corporate litigation cause the exit of the controlling shareholder?. J Corp Finan [Internet].. 2022;73:102192 [CrossRef] | [Google Scholar]
- Cao X, Wang Y, Zhou S.. Anti-corruption campaigns and corporate information release in China. J Corp Finan [Internet].. 2018;49:186-203. [CrossRef] | [Google Scholar]
- Xie S, Lin B, Li J.. Political control, corporate governance and firm value: the case of China. J Corp Finan [Internet].. 2022;72(December 2021):102161 [CrossRef] | [Google Scholar]
- Obaydin I, Zurbruegg R, Hossain MN, Adhikari BK, Elnahas A.. Shareholder litigation rights and stock price crash risk. J Corp Finan [Internet].. 2021;66:101826 [CrossRef] | [Google Scholar]
- Dang VA, Lee E, Liu Y, Zeng C.. Bank deregulation and stock price crash risk. J Corp Finan [Internet].. 2022;72:102148 [CrossRef] | [Google Scholar]
- Zhu B, Xia X, Zheng X.. One way out of the share pledging quagmire: {Evidence} from mergers and acquisitions. J Corp Finan [Internet].. 2021;71:102120 [CrossRef] | [Google Scholar]
- Goldman E, Martel J, Schneemeier J.. A theory of financial media. J Financ Econ [Internet].. 2022;145(1):239-58. [CrossRef] | [Google Scholar]
- Bebchuk LA, Brav A, Jiang W, Keusch T.. Dancing with activists. J Financ Econ [Internet].. 2020;137(1):1-41. [CrossRef] | [Google Scholar]
- Conrad J, Wahal S, Xiang J.. High-frequency quoting, trading and the efficiency of prices. J Financ Econ [Internet].. 2015;116(2):271-91. [CrossRef] | [Google Scholar]
- Liu LX, Shu H, Wei KC.. The impacts of political uncertainty on asset prices: evidence from the Bo scandal in China. J Financ Econ.. 2017;125(2):286-310. [CrossRef] | [Google Scholar]
- Edmans A, Jayaraman S, Schneemeier J.. The source of information in prices and investment-price sensitivity. J Financ Econ [Internet].. 2017;126(1):74-96. [CrossRef] | [Google Scholar]
- Titman S, Wei C, Zhao B.. Corporate actions and the manipulation of retail investors in {China}: {An} analysis of stock splits. J Financ Econ [Internet].. 2022;145(3):762-87. [CrossRef] | [Google Scholar]
- Hu J.. Does option trading convey stock price information? J Financ Econ [Internet]. 2014;111(3):625-45. [CrossRef] | [Google Scholar]
- Muslu V, Radhakrishnan S, Subramanyam KR, Lim D, Muslu V, Radhakrishnan S, et al. Environment forward-looking MD & A disclosures and the. Manag Sci.. 2014(February 2015) [CrossRef] | [Google Scholar]
- Guo Y, Li J, Li Y, You W.. The roles of political risk and crude oil in stock market based on quantile cointegration approach: A comparative study in China and US. Energy Econ [Internet].. 2021;97:105198 [CrossRef] | [Google Scholar]
- Ding R, Zhou H, Li Y.. Social media, financial reporting opacity and return comovement: evidence from Seeking Alpha. J Financ Mark [Internet].. 2020;50:100511 [CrossRef] | [Google Scholar]
- Chen Y, Fan Q, Yang X, Zolotoy L.. CEO early-life disaster experience and stock price crash risk. J Corp Finan [Internet].. 2021;68(December 2020):101928 [CrossRef] | [Google Scholar]
- Li Q, Wang T, Gong Q, Chen Y, Lin Z, Song S., et al. Media-aware quantitative trading based on public Web information {Web} information. Decis Support Syst [Internet].. 2014;61:93-105. [CrossRef] | [Google Scholar]
- Khalfaoui R, Boutahar M, Boubaker H.. Analyzing volatility spillovers and hedging between oil and stock markets: evidence from wavelet analysis. Energy Econ [Internet].. 2015;49:540-9. [CrossRef] | [Google Scholar]
- Baker HK.. Twenty-five years of Review of Financial Economics: A bibliometric overview. 2019:1-21. [CrossRef] | [Google Scholar]
- Jena JR, Biswal SK, Panigrahi RR, Shrivastava AK.. Investigating the potential areas in artificial intelligence and financial innovation: A bibliometric analysis. J Scientometr Res.. 2024;13(1):71-80. [CrossRef] | [Google Scholar]
- Wang H, Lu S, Zhao J.. Aggregating multiple types of complex data in stock market prediction: A model-independent framework. Knowl Based Syst [Internet].. 2019;164:193-204. [CrossRef] | [Google Scholar]
- Anbaee Farimani S, Vafaei Jahan M, Milani Fard A, Tabbakh SR.. Investigating the informativeness of technical indicators and news sentiment in financial market price prediction. Knowl Based Syst [Internet].. 2022;247:108742 [CrossRef] | [Google Scholar]
- Oliveira N, Cortez P, Areal N.. Stock market sentiment lexicon acquisition using microblogging data and statistical measures. Decis Support Syst [Internet].. 2016;85:62-73. [CrossRef] | [Google Scholar]
- Schmid S, Dauth T.. Does internationalization make a difference? Stock market reaction to announcements of international top executive appointments. J World Bus [Internet].. 2014;49(1):63-77. [CrossRef] | [Google Scholar]
- Griffin PA, Jaffe AM, Lont DH, Dominguez-Faus R.. Science and the stock market: investorsā recognition of unburnable carbon. Energy Econ.. 2015;52:1-12. [CrossRef] | [Google Scholar]
- Piotroski JD, Wong TJ, Zhang T.. Political incentives to suppress negative information: evidence from Chinese listed firms. J Acc Res [Internet].. 2015;53(2):405-59. [CrossRef] | [Google Scholar]
- Kim C (Francis), Wang K, Zhang L.. Readability of 10ā{K} {reports} and {stock} {price} {crash} {risk}. Contemp Acc Res [Internet].. 2019;36(2):1184-216. [CrossRef] | [Google Scholar]
- Muslu V, Radhakrishnan S, Subramanyam KR, Lim D.. Forward-{looking} {MD}&{A} {disclosures} and the {information} {environment}. Manag Sci [Internet].. 2015;61(5):931-48. [CrossRef] | [Google Scholar]
- He G.. The effect of CEO inside debt holdings on financial reporting quality. Rev Account Stud [Internet].. 2015;20(1):501-36. [CrossRef] | [Google Scholar]
- Dou Y, Hope O, Thomas WB, Zou Y.. Blockholder {exit} {threats} and {financial} {reporting} {quality}. Contemp Acc Res [Internet].. 2018;35(2):1004-28. [CrossRef] | [Google Scholar]
- Bhattarai S, Chatterjee A, Park WY.. Global spillover effects of US uncertainty. J Monet Econ [Internet].. 2020;114:71-89. [CrossRef] | [Google Scholar]
- Lundgren AI, Milicevic A, Uddin GS, Kang SH.. Connectedness network and dependence structure mechanism in green investments. Energy Econ [Internet].. 2018;72:145-53. [CrossRef] | [Google Scholar]
- Ferrer R, Shahzad SJ, López R, Jareño F.. Time and frequency dynamics of connectedness between renewable energy stocks and crude oil prices. Energy Econ [Internet].. 2018;76:1-20. [CrossRef] | [Google Scholar]
- Wen X, Guo Y, Wei Y, Huang D.. How do the stock prices of new energy and fossil fuel companies correlate? Evidence from China. Energy Econ [Internet].. 2014;41:63-75. [CrossRef] | [Google Scholar]
- Wen F, Zhao L, He S, Yang G.. Asymmetric relationship between carbon emission trading market and stock market: {Evidences} from {China}. Energy Econ [Internet].. 2020;91:104850 [CrossRef] | [Google Scholar]
- Balcilar M, Gupta R, Wohar ME.. Common cycles and common trends in the stock and oil markets: evidence from more than 150 years of data. Energy Econ [Internet].. 2017;61:72-86. [CrossRef] | [Google Scholar]
- Le TH, Chang Y.. Effects of oil price shocks on the stock market performance: do nature of shocks and economies matters?. Energy Econ [Internet].. 2015;51:261-74. [CrossRef] | [Google Scholar]
- Lean HH, Badeeb RA.. Asymmetric impact of oil price on Islamic sectoral stocks. Energy Econ [Internet].. 2017 [CrossRef] | [Google Scholar]
- Jiang Z, Yoon SM.. Dynamic co-movement between oil and stock markets in oil-importing and oil-exporting countries: {Two} types of wavelet analysis. Energy Econ [Internet].. 2020;90:104835 [CrossRef] | [Google Scholar]
- Basher SA, Sadorsky P.. Hedging emerging market stock prices with oil, gold, VIX and bonds: A comparison between DCC, ADCC and GO-GARCH. Energy Econ [Internet].. 2016;54:235-47. [CrossRef] | [Google Scholar]
- Piotroski JD, Wong TJ. TZH.. Political Incentives to Suppress Negative Information: evidence from Chinese listed Firms. J Acc Res.. 2015;53(2) [CrossRef] | [Google Scholar]
- Liu X.. Analyzing the impact of user-generated content on B2B Firmsā stock performance: big data analysis with machine learning methods. Ind Mark Manag [Internet].. 2020;86(Feb):30-9. [CrossRef] | [Google Scholar]
- Demiralay S, Kilincarslan E.. The impact of geopolitical risks on travel and leisure stocks. Tour Manag [Internet].. 2019;75(Jun):460-76. [CrossRef] | [Google Scholar]
- GalĆ J, Gambetti L.. The effects of monetary policy ā on stock market bubbles: some evidence. Am Econ J Macroecon.. 2015;7(1):233-57. [CrossRef] | [Google Scholar]
- Yunita PI, Salim U, Rofiaty R, Indrawati NK.. Resilience in business: A bibliometric analysis. J Scientometr Res.. 2024;13(2):333-48. [CrossRef] | [Google Scholar]