Contents
ABSTRACT
This study investigates the variations in lexical richness within English language theses across diverse disciplines, focusing on areas where researchers exhibit higher degrees of lexical richness and the evolution of vocabulary usage over time. By analyzing these variations, the research aims to provide insights into the effective use of lexical richness in academic writing and contribute to the development of more engaging and comprehensible scholarly publications. A total of 320 theses were randomly selected from the Turkey National Thesis Center and classified according to their scientific discipline. Using natural language processing techniques, unique word count, word diversity, and other metrics were analyzed. Results reveal that social sciences tend to exhibit higher lexical richness compared to natural sciences, and no significant difference was observed in word richness between social and natural sciences disciplines. These findings contribute to the understanding of lexical richness in academic writing and highlight the importance of achieving a balance between lexical richness and readability.
INTRODUCTION
In academic writing, a well-crafted language characterized by a high degree of lexical richness greatly aids readers in achieving better comprehension, becoming more persuasive and impactful, and even altering their attitudes and behaviors through the evocation of their emotions. The extent of lexical richness, encompassing the employment of diverse terminologies, intricate sentence structures, and a wide-ranging vocabulary, profoundly influences the caliber of scholarly publications.[1]
Clarity and readability are of paramount importance in academic publications, as these works are frequently aimed at a heterogeneous readership representing a myriad of backgrounds. Consequently, the judicious application of lexical richness is essential in facilitating a reader’s comprehension of the text. Incorporating an array of sentence structures and an advanced vocabulary can further augment the reader’s grasp of the subject matter.
Scholarly publications are typically the medium for the presentation of novel ideas and groundbreaking discoveries.
Employing a high degree of lexical richness enables authors to articulate these innovative concepts in a unique and captivating manner, ultimately leading to more engaging and thought-provoking contributions to the academic community. In the realm of academic writing, the accuracy and expressiveness of an author’s ideas are of utmost significance.[2] An elevated level of lexical richness permits authors to effectively communicate their thoughts, thereby enhancing the reader’s understanding.
It is imperative to strike a balance between lexical richness and readability in academic writing. An overabundance of lexical richness can render a publication challenging to comprehend, ultimately leading to reader fatigue. On the other hand, employing a limited vocabulary may cause the publication to appear monotonous and uninspiring. Attaining the optimal equilibrium between lexical richness and readability is crucial for generating high-quality academic writing.
The primary objective of this study is to examine the variation in lexical richness within English language theses across a diverse array of disciplines. The research emphasizes disciplines in which researchers exhibit a higher degree of lexical richness, as well as the evolution of vocabulary usage over time. The objective of this research is to gain a deeper understanding of how lexical diversity can be effectively utilized in academic writing, with the ultimate goal of enhancing the quality and readability of scholarly publications through valuable insights.
RELATED STUDIES
Natural Language Processing (NLP) has emerged as a prominent area of academic research in recent years.[3–5] The vast availability of natural language data, particularly through the internet and social media platforms, has facilitated the development of NLP models based on large datasets and the exploration of diverse patterns, structures, and relationships within natural language.[6, 7] Artificial intelligence and machine learning techniques are widely employed in NLP research, enabling the creation of algorithms capable of processing extensive datasets and autonomously learning language models.
NLP can be utilized in a multitude of applications, such as machine translation, text classification, sentiment analysis, speech recognition, word suggestions, and dialogue systems. Consequently, NLP research can be applied across various domains, fostering the development of more effective and efficient language processing models. Lexical richness and language quality are vital components of NLP research, with lexical richness serving as a measure of the richness and diversity of natural language.[8]
Several academic methods are available for measuring lexical richness. Heaps’ Law posits that the number of unique words in a document is proportional to the total word count raised to a specific power, providing more detailed information on lexical richness.[9, 10] The Shannon-Weaver Index employs entropy as a natural measure of word distribution, offering insights into not only lexical richness but also the regularity of word distribution. The n-gram method measures lexical richness by counting word combinations within a specific sequence of words.[11, 12]
Dictionary-based methods offer a simple and rapid approach to measuring lexical richness but overlook the varying importance of individual words and the potential for multiple meanings. The unique word count method calculates the number of unique words in a document, providing a straightforward and swift measure, albeit with limitations similar to those of dictionary-based methods. The Type-Token Ratio (TTR) computes the ratio of unique words to the total word count in a document, providing more comprehensive information on lexical richness.[13]
The Guiraud Index calculates the ratio of unique words in a document to the square root of the document’s total word count, providing more in-depth insights into lexical richness and accounting for the relationship between unique word count and document length. The Herdan Index determines the ratio of unique words in a document to half of the document’s total word count, offering detailed information on lexical richness and considering the relationship between unique word count and document length. The lexical richness of senior students was investigated by comparing their written works to academic papers authored by their lecturers.[13] The analysis revealed that lecturers performed better in terms of Type-Token Ratio (TTR) and academic vocabulary usage, while students demonstrated a slightly higher usage of 2000-word level and off-list words. Joe[14] tracked the quality and quantity of encounters with 20 vocabulary items experienced by adult Second-Language (L2) learners over a 3-month period in an English for Academic Purposes course. The differences in vocabulary choices between Chinese master’s degree candidates and advanced writers displayed a higher level of lexical richness and complexity.[15] The lexical richness in research articles published by English as a Second Language (ESL) and English as a Foreign Language (EFL) writers from ASEAN countries has been determined to reveal the presence of significant similarities and differences between the two groups in terms of lexical richness.[16] To answer this question, the researchers employed three different lexical measures: lexical density, lexical diversity, and lexical sophistication. Utilizing analytical tools such as the CLAWS Tagger, Moving-Average Type-Token Ratio (MATTR), and VocabProfiler, they analyzed the data and compared the results between ESL and EFL groups using the Mann-Whitney U test. This study also discussed the factors influencing word usage by both groups and concluded with the study’s limitations and directions for future research.
The relationship between text length and lexical richness from an entropy-based perspective are examined in a study.[17] Their findings indicated a nonlinear growth model for lexical richness as text length increased. Kim’s study compared the lexical richness, specifically lexical diversity, density, and complexity, in research article manuscripts prepared by Chinese doctoral candidates (PhD-Candidate) to those written by native undergraduate and master’s level students (Native Beginner Students, NBS) as well as published and unpublished research articles. In a study discuss methods for measuring linguistic richness from a linguist’s perspective.[18] Assessing the scope and richness of a language is not an area of study exclusive to the English language. Word richness analysis are done in textual documents for French, German, and Portuguese languages in academic studies,[19] while a study[20] focused on French, and other study[21] explored the Turkish language in same matter.
DATA ANALYSIS AND PROPOSED METHOD
A master’s thesis aims to facilitate the student’s in-depth research in their field of study, enabling them to acquire comprehensive knowledge on their thesis topic and contribute creatively within the discipline. The thesis typically spans 50-100 pages and encompasses an extensive literature review, research methodology, results, discussion, and recommendations. A doctoral dissertation, on the other hand, entails a more extensive research endeavor compared to a master’s thesis. Doctoral students engage in interdisciplinary, high-level research to creatively address or uncover new findings within their discipline. Doctoral dissertations are generally 200-300 pages long, incorporating an extensive literature review, research methodology, results, discussion, recommendations, an introduction reflecting the student’s broad research and knowledge within the discipline, and a preface summarizing the topics presented in the thesis.
Both master’s and doctoral theses offer opportunities for students to showcase their in-depth mastery of the subject matter and contribute to the scientific community in their respective disciplines. They also aid in the development of students’ research methodology application, analysis, interpretation, and reporting skills. Theses necessitate academic writing style and language usage, adhering to grammar and writing rules, and employing scientific and technical language.
Lexical richness in theses is a crucial factor, reflecting the researcher’s command of the subject, analytical thinking ability, and writing skills.[22] Emphasis should be placed on lexical diversity rather than merely the number of words used. Lexical richness signifies the researcher’s expertise in the subject, as they must select appropriate words to convey their ideas. Word choice demonstrates the researcher’s familiarity with the topic, and their analytical thinking ability, as they must approach the subject from various angles and select words to express their ideas accurately.
Moreover, lexical richness is essential in terms of thesis quality.[23] Lexical diversity renders the thesis more varied and engaging, while preventing word repetition, ensuring a smooth and comprehensible flow. The characteristics of a scientific subject can influence the lexical richness employed within a thesis. For instance, some scientific topics, particularly those that are technical and abstract, involve fewer common terms and phrases. While such topics necessitate a higher degree of lexical richness, it is also crucial to maintain clarity by using more straightforward and accessible language. Conversely, more general and widely understood topics require less technical terminology and simpler lexical structures.
There are numerous methods for text classification within data mining, including the following:
Naïve Bayes Classification
This algorithm determines whether a text belongs to a particular category by calculating the probability of each word in a given sequence. Naïve Bayes classification is one of the most commonly used methods for text classification.[24]
Decision Trees
Artificial Neural Networks (ANNs)
ANNs process word features within a sequence through a multi-layered network to determine whether a text belongs to a specific category.[27]
For this study, Artificial Neural Networks (ANNs) were chosen for text classification due to their ability to learn from large datasets, which is ideal for solving complex problems such as text classification by providing sufficient data for better results. ANNs offer a flexible and customizable structure for learning relationships and patterns in texts, allowing for greater flexibility in analyzing features and achieving improved results in text classification.[28] ANNs can also perform well when encountering new and different texts, utilizing pre-learned models for successful classification of previously unseen texts. Additionally, ANNs can learn semantic relationships and emotional tones, which are important for text classification.[29]
In this study, a neural network was created using the MLPClassifier from the Python Scikit library, which is an open-source machine learning library written in Python that is frequently preferred for creating ANNs due to its ease of use. Scikit contains many predefined functions and classes necessary for creating ANN models and is a smaller, lighter library compared to larger-scale ANN libraries like TensorFlow and PyTorch, making it more suitable for smaller projects and faster prototyping.
Data Collection and Analysis Results
The Turkey National Thesis Center,[30] administered by the Higher Education Council (YÖK) of Turkey, serves as a central repository for postgraduate theses completed in Turkish universities, making them accessible to researchers. The main purpose of this center is to facilitate researchers’ access to academic resources and contribute to scholarly endeavors. The Thesis Center houses a comprehensive collection of theses across various disciplines, providing students, academics, and other researchers with a valuable source of information. Furthermore, the center supports the advancement of postgraduate education in Turkey and encourages the dissemination of academic knowledge. Figure 1 displays a sample search result page from the Turkey National Thesis Center website.
For the scope of this study, a total of 320 theses written between 2018 and 2023 were randomly selected from the Turkey National Thesis Center. Only English-language theses were chosen to be included in the sample. Table 1 illustrates the criteria we used for data selection. The theses were classified according to their scientific discipline, with the list of disciplines selected from SpringerLink.[31] For classification, we utilized data from publications available on SpringerLink. Specifically, we focused on the titles and abstracts of the publications for the classification task.
Discipline | Noun | Verb | Adjective | Total W Number | Unique W Number | Word Richness |
---|---|---|---|---|---|---|
Architecture | 24,498 | 4,145 | 3,989 | 579,185 | 26,962 | 0.0466 |
Biomedicine | 30,085 | 2,583 | 3,673 | 456,737 | 31,498 | 0.0690 |
Business and Management | 20,433 | 3,088 | 3,141 | 444,378 | 22,207 | 0.0500 |
Chemistry | 18,784 | 2,581 | 3,254 | 414,607 | 20,655 | 0.0498 |
Computer Science | 13,857 | 2,623 | 2,606 | 253,413 | 15,629 | 0.0617 |
Criminology and Criminal Justice | 30,889 | 5,090 | 4,439 | 636,901 | 33,890 | 0.0532 |
Cultural and Media Studies | 19,358 | 4,582 | 3,667 | 396,268 | 22,974 | 0.0580 |
Earth Sciences | 13,927 | 2,459 | 2,683 | 297,206 | 15,402 | 0.0518 |
Economics | 15,809 | 3,198 | 2,914 | 346,602 | 17,992 | 0.0519 |
Education | 21,130 | 2,895 | 2,961 | 506,856 | 22,461 | 0.0443 |
Energy | 21,569 | 3,938 | 3,637 | 514,555 | 23,601 | 0.0459 |
Engineering | 19,776 | 2,004 | 2,338 | 367,636 | 20,373 | 0.0554 |
Environment | 23,415 | 3,021 | 3,652 | 512,936 | 24,984 | 0.0487 |
Finance | 18,946 | 3,192 | 2,823 | 410,562 | 20,189 | 0.0492 |
Geography | 25,156 | 3,509 | 3,715 | 474,209 | 27,268 | 0.0575 |
History | 70,103 | 5,110 | 4,847 | 2,727,715 | 69,727 | 0.0256 |
Law | 26,332 | 5,322 | 4,324 | 658,072 | 29,417 | 0.0447 |
Life Sciences | 25,619 | 1,808 | 2,640 | 383,625 | 25,911 | 0.0675 |
Linguistics | 22,212 | 3,758 | 3,346 | 806,509 | 24,536 | 0.0304 |
Literature | 28,552 | 5,238 | 4,724 | 543,334 | 32,733 | 0.0602 |
Materials Science | 26,438 | 2,720 | 3,333 | 498,492 | 27,311 | 0.0548 |
Mathematics | 9,324 | 1,359 | 1,294 | 293,081 | 10,266 | 0.0350 |
Medicine and Public Health | 25,289 | 2,153 | 3,237 | 408,395 | 26,115 | 0.0639 |
Philosophy | 31,938 | 4,776 | 4,822 | 728,695 | 35,713 | 0.0490 |
Physics | 12,725 | 2,201 | 2,041 | 281,786 | 14,196 | 0.0504 |
Political Science | 25,945 | 4,987 | 4,544 | 658,645 | 29,669 | 0.0450 |
Popular Science | 23,672 | 3,653 | 3,705 | 465,393 | 25,465 | 0.0547 |
Psychology | 32,445 | 2,898 | 3,907 | 615,173 | 33,422 | 0.0543 |
Religious Studies | 50,216 | 3,574 | 4,234 | 1,093,825 | 50,570 | 0.0462 |
Social Sciences | 24,868 | 4,774 | 4,407 | 618,703 | 28,099 | 0.0454 |
Statistics | 11,077 | 2,161 | 1,978 | 248,033 | 12,375 | 0.0499 |
The Stanford NLP[32] library provides a suite of tools consisting of pre-trained modules for performing Natural Language Processing (NLP) tasks. These modules facilitate various tasks such as tokenization, sentence segmentation, and Part-of-Speech (POS) tagging of a given text. Tokenization is the process of dividing a text into words or symbols. The Stanford NLP library accomplishes this task using a class called TokenizerAnnotator. This class takes a text input and returns a list of type List<CoreLabel>, where each element represents a word or symbol in the input text. Sentence segmentation involves identifying sentences within a text. The Stanford NLP library carries out this task using a class called WordsToSentencesAnnotator. This class takes a tokenized text list as input and returns a list of type List<CoreMap>, where each element represents a sentence in the input text. POS tagging determines grammatical information for each word in a text. The Stanford NLP library performs this task using a class called POSTaggerAnnotator. This class takes a tokenized text list as input and returns a list of type List<CoreMap>, where each element represents a word in the input text along with its grammatical information.
Using the Stanford NLP library, the tokenization, sentence segmentation, and POS tagging processes were applied to the specified text. Subsequently, each word in the text was processed to extract only certain word types, such as nouns, verbs, and adjectives. Words with fewer than two characters were excluded to avoid incorporating non-word values from formulas and tables into the calculation. Similarly, words containing characters outside the English character set were disregarded. This allowed for the computation of the number of unique words. Finally, word diversity was calculated by dividing the number of unique words by the total word count. During the selection of theses, a completely random function was employed, with the condition that no two theses from the same discipline, year, author, or institution were included. Only theses with full-text access available and published in last ten years were chosen for this study.
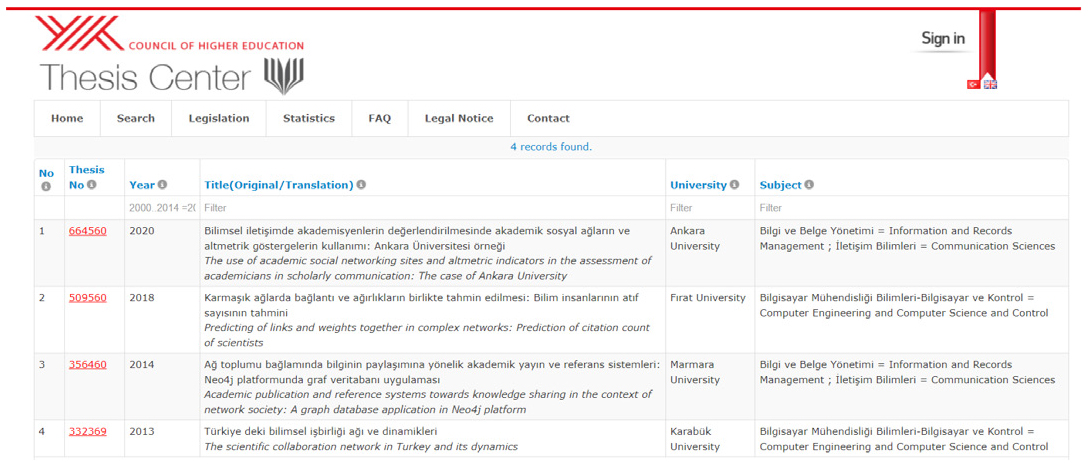
Figure 1:
The Turkey National Thesis Center Search Screen
Table 2 contains data derived from the theses used in this study, with 10 theses selected from each discipline. The columns for Nouns, Verbs, and Adjectives represent the total count of each respective word type in the selected ten theses. The Unique W Number column displays the cumulative sum of distinct words found in the theses for each discipline. Word Richness, on the other hand, is the ratio of unique word count to the total word count.
Discipline | Total Word Number | Unique Word Number | Word Richness | Normalization | UWNAN | WRNAN X 100 |
---|---|---|---|---|---|---|
Biomedicine | 456,737 | 31,498 | 0.069 | 0.909 | 28,621 | 6.27 |
Life Sciences | 383,625 | 25,911 | 0.068 | 0.923 | 23,923 | 6.23 |
Medicine and Public Health | 408,395 | 26,115 | 0.064 | 0.918 | 23,982 | 5.87 |
Computer Science | 253,413 | 15,629 | 0.062 | 0.949 | 14,837 | 5.86 |
Literature | 543,334 | 32,733 | 0.060 | 0.891 | 29,176 | 5.37 |
Cultural and Media Studies | 396,268 | 22,974 | 0.058 | 0.921 | 21,153 | 5.34 |
Geography | 474,209 | 27,268 | 0.058 | 0.905 | 24,682 | 5.20 |
Engineering | 367,636 | 20,373 | 0.055 | 0.926 | 18,875 | 5.13 |
Popular Science | 465,393 | 25,465 | 0.055 | 0.907 | 23,095 | 4.96 |
Materials Science | 498,492 | 27,311 | 0.055 | 0.900 | 24,588 | 4.93 |
Earth Sciences | 297,206 | 15,402 | 0.052 | 0.941 | 14,486 | 4.87 |
Economics | 346,602 | 17,992 | 0.052 | 0.931 | 16,745 | 4.83 |
Psychology | 615,173 | 33,422 | 0.054 | 0.877 | 29,310 | 4.76 |
Physics | 281,786 | 14,196 | 0.050 | 0.944 | 13,396 | 4.76 |
Statistics | 248,033 | 12,375 | 0.050 | 0.950 | 11,761 | 4.74 |
Criminology and Criminal Justice | 636,901 | 33,890 | 0.053 | 0.873 | 29,573 | 4.64 |
Chemistry | 414,607 | 20,655 | 0.050 | 0.917 | 18,942 | 4.57 |
Business and Management | 444,378 | 22,207 | 0.050 | 0.911 | 20,233 | 4.56 |
Finance | 410,562 | 20,189 | 0.049 | 0.918 | 18,531 | 4.52 |
Environment | 512,936 | 24,984 | 0.049 | 0.897 | 22,421 | 4.37 |
Philosophy | 728,695 | 35,713 | 0.049 | 0.854 | 30,508 | 4.19 |
Architecture | 579,185 | 26,962 | 0.047 | 0.884 | 23,839 | 4.12 |
Energy | 514,555 | 23,601 | 0.046 | 0.897 | 21,172 | 4.12 |
Education | 506,856 | 22,461 | 0.044 | 0.899 | 20,184 | 3.98 |
Social Sciences | 618,703 | 28,099 | 0.045 | 0.876 | 24,622 | 3.98 |
Political Science | 658,645 | 29,669 | 0.045 | 0.868 | 25,761 | 3.91 |
Law | 658,072 | 29,417 | 0.045 | 0.868 | 25,545 | 3.88 |
Religious Studies | 1,093,825 | 50,570 | 0.046 | 0.781 | 39,507 | 3.61 |
Mathematics | 293,081 | 10,266 | 0.035 | 0.941 | 9,664 | 3.29 |
Linguistics | 806,509 | 24,536 | 0.030 | 0.839 | 20,578 | 2.55 |
History | 2,727,715 | 69,727 | 0.026 | 0.454 | 31,688 | 1.16 |
Upon examining Table 2, it is evident that publications in the field of History utilize a significantly greater number of distinct nouns compared to other disciplines, followed by Religious Studies. Other disciplines display a similar usage of distinct nouns. The three disciplines with the lowest count of noun-type words are Mathematics, Statistics, and Physics. This data suggests that social sciences employ a more diverse set of nouns than natural sciences, possibly due to the broader range of area and descriptive information in social sciences. Regarding verb usage, Law exhibits the highest diversity, which may be attributed to the unique characteristics of legal studies. Mathematics, on the other hand, has the lowest verb diversity. In general, 8 of the top 10 disciplines with the highest verb diversity belong to social sciences, while the bottom 10 belong to natural sciences. When analyzing adjectives, there is no striking relationship between their usage and the disciplines. Although History, Philology, and Literature have higher adjective usage, and the bottom five are natural sciences, the difference is relatively small compared to other word types. The total word number does not present a distinctive characteristic for the disciplines, despite the differences between the lowest and highest values. One crucial metric for determining word richness is the unique word count. Social sciences exhibit a clear dominance in this regard, with History, Religious Studies, and Philosophy taking the top three spots. Excluding Biomedicine at the seventh position, 10 out of the top 11 disciplines belong to social sciences. When comparing the average unique word count of the lowest three disciplines (Physics, Statistics, and Mathematics) with the top three, there is a more than three-fold difference. Word richness is defined as the ratio of unique word count to the total word count for each category. The top three highest word richness values belong to health sciences, which may be attributed to the discipline’s unique and Latin-based vocabulary. There is no significant difference in word richness between social and natural sciences disciplines.
Normalization is a process used to eliminate scale differences between various features of a dataset. Min-Max normalization is a technique that transforms the values of a feature in a dataset to a specific range by rescaling the feature values between the minimum and maximum values.[33] As a result, the feature values range between 0 and 1. In this study, Min-Max normalization was applied to the total word count and then subtracted from 1. This was done to ensure that a higher total word count negatively impacts the result.
The Min-Max normalization function was executed with the lowest total word count set to 0 and the highest total word count set to 5 million. After this step, the normalization coefficient was recorded in a new column by applying normalization to all disciplines as mentioned above, subtracting the result from 1, and then multiplying the unique word count. The product of this calculation was then multiplied by the word richness value and by 100 to obtain the normalized word richness value. Multiplying by 100 was done to enhance the readability of the Table 2.
The normalization of the unique count resulted in minor position changes in the table, but no significant alterations were observed. There were more changes in the normalized word richness values, but again, not significant enough to alter the previous findings. Although there were changes within the top 10 and bottom 10, no external changes were observed. Table 3 contains two abbreviations: UWNAN (Unique Word Number After Normalization) and WRNAN (Word Richness Number After Normalization). In Equation 1, the normalization function used in the application is presented.
Figure 2 shares the same content as Table 2. In Figure 2, the exceptional case in history is more clearly visible. Additionally, it can be observed that the word richness in social sciences is somewhat higher compared to natural sciences, although the general averages are relatively close to each other.
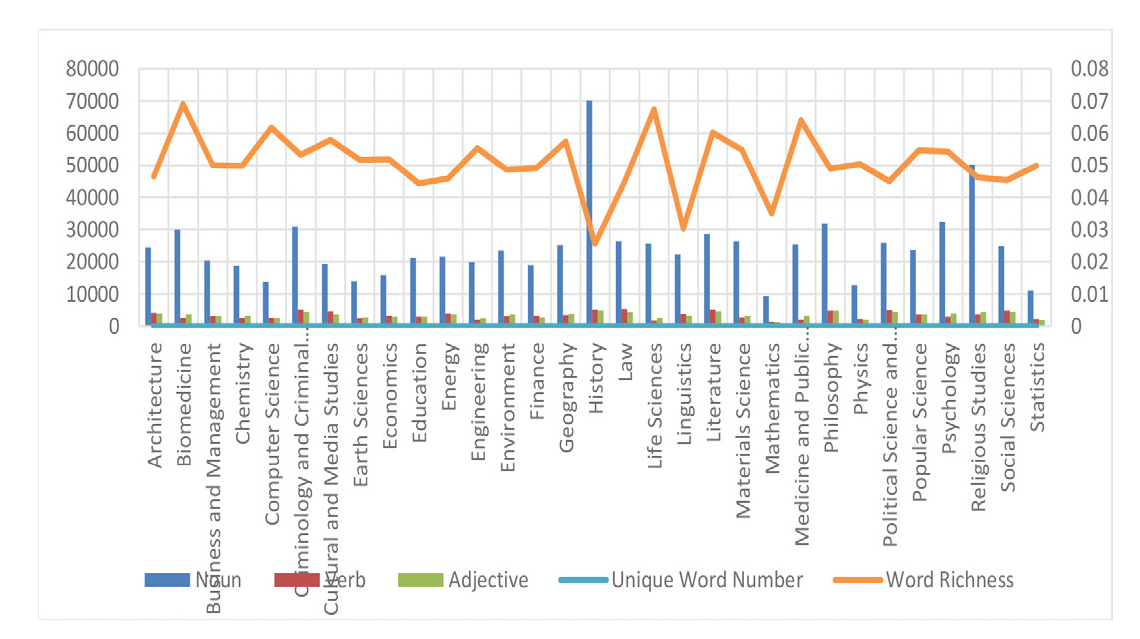
Figure 2:
Word Types Statistics by Disciplines

Figure 3:
Word cloud by common usage of the most frequently used verbs in data.

Figure 4:
Word cloud by common usage of the most frequently used nouns in data
Another test conducted within the scope of this study is a list of the most frequently occurring words by discipline. The list of the top ten most common verbs for each discipline is provided in Appendix 1. When Appendix 2 is examined, it is evident that the adjectives are almost entirely different for each discipline. Upon examining this list, it is evident that the verbs vary minimally across disciplines. Figure 3, on the other hand, displays a word cloud generated from the most frequently used verbs across the entire dataset.
Figure 4 presents a word cloud generated from the most frequently used nouns. The inclusion of the term “Turkey” is believed to be a result of examining theses from Turkey. The other words in the word cloud are consistent with thesis writing and academic language.
DISCUSSION
Our research findings delineate a heightened lexical richness in the social sciences compared to the natural sciences. Notably, the variability in lexical richness across these disciplines was not significantly distinct. These insights contribute to our understanding of lexical diversity in academic writing and underscore the necessity of striking a balance between word richness and readability. The results suggest that various academic disciplines possess distinct language and style attributes, with both social and natural sciences exhibiting discernible lexical richness. This demonstrates that each discipline possesses its unique lexicon shaped by the discipline’s specific topics and research methods. In the natural sciences, which often employ a more technical and specific language, a lower lexical richness might be anticipated, given the typically narrower focus of the studies. Conversely, the social sciences typically engage with broader and varied topics, necessitating a more extensive language repertoire. These results underscore the significance of language in scientific communication and highlight the need to recognize the critical role of language in the transmission of scientific knowledge. We propose that academic writing should consider this role of language to enhance interdisciplinary comprehension.
Table 2 reveals significant variances in word richness, unique word count, and total word count across different disciplines. For instance, despite having the highest total word count (2,727,715), the discipline of History exhibits the lowest lexical richness (0.0256). This could be attributed to historical studies often covering a broad range of topics, thereby necessitating a larger vocabulary. Nevertheless, it also reveals the tendency of historical studies to utilize a particular language and style that tends to be more general and broader. Conversely, the discipline of Biomedicine displays the highest lexical richness (0.0690), indicative of its usage of technical and specific language. This suggests that research in this field often focuses on very specific and specialized topics, thereby necessitating a larger vocabulary. Furthermore, the high lexical richness in Literature (0.0602) indicates that literary studies often cover broad and varied topics, requiring a wider vocabulary. The varying emphasis on different word types (noun, verb, adjective) across disciplines highlights distinct stylistic and linguistic differences.
The effects of the normalization process on lexical richness are evident in Table 2. For instance, the lexical richness of the Biomedicine discipline rose from 0.069 to 6.27 after normalization. Similarly, the lexical richness of History increased from 0.0256 to 1.16 after normalization. This demonstrates that through normalization, each discipline’s lexical richness becomes proportional to the total word count. Disciplines with increased lexical richness ratio post-normalization (Biomedicine, Life Sciences, Medicine and Public Health, Computer Sciences, Literature) are generally those requiring the use of specific terms and jargon. Conversely, disciplines with a reduced lexical richness ratio post-normalization (Mathematics, Linguistics, History) tend to write about more general and broad concepts.
The findings of this study hold significant implications for academic writing instruction. By identifying frequently used word types and general lexical richness in specific disciplines, educators can guide students in adopting language and stylistic features to enhance their writing efficacy.
Such an analysis can also assist in enhancing interdisciplinary communication. In interdisciplinary studies, the specific vocabulary and language use of a certain discipline may be complex and unintelligible for individuals outside the discipline. By providing more information about interdisciplinary language use, this study can help to overcome such barriers.
Given the constraints of this study, it’s pertinent to mention the need for further research in this area. Comparisons between theses from different centers and countries, as well as between native and non-native English-speaking contexts, along with larger sample sizes, could allow for a better understanding of the differences in language use across various academic disciplines and contexts.
This study represents a significant step in analyzing lexical richness in academic texts across specific disciplines. However, certain limitations are present, which must be considered when interpreting and generalizing the findings.
Primarily, the inclusion of only ten theses from each discipline restricts the generalizability of the results. Theses often represent the most profound part of research, therefore, there can be substantial stylistic and lexical variations among them. Consequently, a larger sample size could potentially yield more reliable and generalizable results. The fact that these were sourced from a single country’s thesis center also confines the generalizability of the outcomes. This is particularly true given that the center is located in a non-native English-speaking country. What different countries and linguistic backgrounds influence students’ academic writing styles and vocabularies is largely unknown, and this study presumes the universality and standardization of language.
These limitations underscore the need for a cautious interpretation of this study’s findings. However, even with these constraints, the study provides valuable insights into how vocabulary and lexical richness vary across different academic disciplines. This research could form the foundation for future studies in this area, and larger sample sizes, theses from diverse geographical locations, and a more detailed evaluation of the classification algorithm could enhance the strength and generalizability of such studies.
CONCLUSION
In conclusion, this study investigated the lexical richness in English-language theses across a wide range of academic disciplines. The analysis revealed that social sciences tend to exhibit greater lexical richness compared to natural sciences, with disciplines like History, Religious Studies, and Philosophy demonstrating higher unique word counts. The findings also highlighted that verb diversity is generally higher in social sciences, while noun diversity presents more variation across disciplines. The application of normalization to the unique word count and word richness values led to minor changes in the rankings but did not significantly alter the overall conclusions. The word clouds generated from the most frequently used verbs and nouns in the dataset provided further insights into the similarities and differences in word usage across disciplines.
Several limitations of this study should be noted, including the relatively small sample size of ten theses per discipline and the selection of theses from a single thesis center in a non-English- speaking country. Future research could address these limitations by increasing the sample size, incorporating theses from multiple centers and countries, and comparing the results between native and non-native English-speaking contexts. Moreover, advanced NLP methods and deep learning techniques could be employed in future research to analyze the contextual meaning of words, further enhancing the evaluation of lexical richness in academic texts.
Cite this article
Müngen AA. Exploring Lexical Richness in English-Language theses Across Disciplines: A Comparative Analysis. J Scientometric Res. 2023;12(2):459-68.
References
- Tweedie FJ, Harald Baayen R. How variable may a constant be? measures of lexical richness in perspective. Comput Hum [Internet]. 1998;32(5):323-52. [CrossRef] | [Google Scholar]
- Fischman GE, Alperin JP, Willinsky J. Visibility and Quality in Spanish-Language Latin American Scholarly Publishing. Information Technologies and International Development [Internet]. 2010;6(4):1-21. Available fromhttps://itidjournal.org/index.php/itid/article/view/639
[CrossRef] | [Google Scholar] - Torfi A, Shirvani RA, Keneshloo Y, Tavaf N, Fox EA. Natural Language Processing Advancements by Deep Learning: A Survey. 2020 Available fromhttps://arxiv.org/abs/2003.01200v4
[CrossRef] | [Google Scholar] - Otter DW, Medina JR, Kalita JK. A Survey of the Usages of Deep Learning for Natural Language Processing. IEEE Trans Neural Netw Learn Syst. 2021;32(2):604-24. [CrossRef] | [Google Scholar]
- Chowdhary KR. Natural Language Processing. Fundamentals of Artificial Intelligence [Internet]. 2020:603-49. Available from [CrossRef] | [Google Scholar]
- Yang S, Ning Z, Wu Y. NLP Based on Twitter Information: A Survey Report. 2020:620-5. [CrossRef] | [Google Scholar]
- Hasan MR, Maliha M, Arifuzzaman M. Sentiment Analysis with NLP on Twitter Data. 2019 [CrossRef] | [Google Scholar]
- Garg S, Saini A, Khanna N. Is sentiment analysis an art or a science? Impact of lexical richness in training corpus on machine learning. 2016 [CrossRef] | [Google Scholar]
- Babhulgaonkar A, Shirsath M, Kurdukar A, Khandare H, Tekale A, Musale M, et al. Empirical Laws of Natural Language Processing for Hindi Language. Advances in Intelligent Systems and Computing [Internet]. 2021;1245:217-23. [CrossRef] | [Google Scholar]
- Gelbukh A, Sidorov G. Zipf and heaps laws? Coefficients depend on language. Lecture Notes in Computer Science (including subseries Lecture Notes in Artificial Intelligence and Lecture Notes in Bioinformatics) [Internet]. 2001;2004:332-5. [CrossRef] | [Google Scholar]
- Christopher Wang, Evelyne Thrasher, Xiaolong Viegas, Bo-june Li, Hsu K. An Overview of Microsoft Web N-gram Corpus and Applications. 2010:45-8. Available fromhttp://research.microsoft.com/web-ngram
[CrossRef] | [Google Scholar] - Gledec G, Soic R, Dembitz S. Dynamic N-Gram system based on an online croatian spellchecking service. IEEE Access. 2019;7:149988-95. [CrossRef] | [Google Scholar]
- Djiwandono PI. Lexical richness in academic papers: A comparison between students’ and lecturers’ essays. Indonesian Journal of Applied Linguistics. 2016;5(2) [CrossRef] | [Google Scholar]
- Joe A. The quality and frequency of encounters with vocabulary in an English for Academic Purposes programme. Reading in a Foreign Language. 2010;22(1) [CrossRef] | [Google Scholar]
- Liu Z, Liu H. Quantitative analysis of academic writing as to informality and vocabulary features. Glottometrics. 2020:49 [CrossRef] | [Google Scholar]
- Choemue S, Bram B. Lexical Richness in Scientific Journal Articles: A Comparison between ESL and EFL Writers. Indonesian Journal of EFL and Linguistics. 2021;6(1) [CrossRef] | [Google Scholar]
- Shi Y, Lei L. Lexical Richness and Text Length: An Entropy-based Perspective. J Quant Linguist. 2022;29(1) [CrossRef] | [Google Scholar]
- Kyle K. Measuring Lexical Richness. In: The Routledge Handbook of Vocabulary Studies. 2019:454-76. [CrossRef] | [Google Scholar]
- Vanhove J, Bonvin A, Lambelet A, Berthele R. Predicting perceptions of the lexical richness of short French, German, and Portuguese texts using text-based indices. J Writ Res [Internet]. 2019;10(3):499-525. Available fromhttps://jowr.org/index.php/jowr/article/view/603
[CrossRef] | [Google Scholar] - Vold ET. Development of lexical richness among beginning learners of French as a foreign language. Nordic Journal of Language Teaching and Learning [Internet]. 2022;10(2):182-211. Available fromhttps://journal.uia.no/index.php/NJLTL/article/view/1007
[CrossRef] | [Google Scholar] - Mete F. Yabanci Dilde Kelime Öğretiminde Resimlerin Seviye Ve Taksonomiye Uygun Kullanimi. Hacettepe Üniversitesi Yabancı Dil Olarak Türkçe Araştırmaları Dergisi [Internet]. 2015(2):81-94. Available fromhttps://dergipark.org.tr/en/pub/huydotad/issue/37784/436232
[CrossRef] | [Google Scholar] - González-López S, López-López A, García-Gorrostieta JM, Rodríguez Espinoza I. TURET2.0: Thesis Writing Tutor Aimed on Lexical Richness in Students’Texts. Research in Computing Science. 2016;129(1) [CrossRef] | [Google Scholar]
- Halim SW. Lexical Richness in English Language and Culture Department Students’ Undergraduate Theses. Journal of English Language and Culture. 2018;8(2) [CrossRef] | [Google Scholar]
- Johnson AA, Ott MQ, Dogucu M. Naive Bayes Classification. Bayes Rules!. 2022 [CrossRef] | [Google Scholar]
- Chao W, Junzheng W. Cloud-service decision tree classification for education platform. Cogn Syst Res. 2018:52 [CrossRef] | [Google Scholar]
- Shaheen M, Zafar T, Ali Khan S. Decision tree classification: Ranking journals using IGIDI. Journal of Information Science. 2020;46(3) [CrossRef] | [Google Scholar]
- Doğan E, Buket KA, Müngen A. Generation of Original Text with Text Mining and Deep Learning Methods for Turkish and Other Languages. 2019 [CrossRef] | [Google Scholar]
- Yasar A, Saritas MM. Performance Analysis of ANN and Naive Bayes Classification Algorithm for Data Classification. International Journal of Intelligent Systems and Applications in Engineering. 2019;7(2) [CrossRef] | [Google Scholar]
- Bala R, Kumar D. Classification Using ANN: A Review. International Journal of Computational Intelligence Research. 2017;13(7) [CrossRef] | [Google Scholar]
- Turkey Higher Education Council. Turkey National Thesis Center [Internet]. 2023 Available fromhttps://tez.yok.gov.tr/
- SpringerLink. 2023 Available fromhttps://link.springer.com/
- Stanford University. The Stanford NLP Group. stanford.edu. 2023 [CrossRef] | [Google Scholar]
- Henderi H. Comparison of Min-Max normalization and Z-Score Normalization in the k-Nearest Neighbor (kNN) Algorithm to Test the Accuracy of Types of Breast Cancer. International Journal of Informatics and Information Systems. 2021;4(1) [CrossRef] | [Google Scholar]