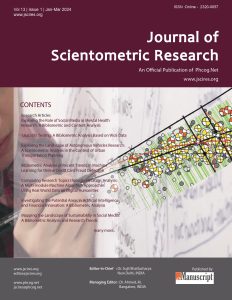
The Journal of Scientometric Research (J. Scientometric Res.) is the official journal of Phcog.Net. The open-access journal publishes peer-reviewed articles after carefully selecting them through a double-blind peer-review process. It encourages the development of scientometric research (in its broadest sense) as well as the use of scientometric data as ‘object of investigation’ or scientometric analysis in innovation and STS studies. It also reaches out to scholars of STS, sociology, economics, and related fields.
JCR IF in 2023 is 0.8
CiteScore in 2023 is 1.7
Frequency: Rapid at a time publication – Triannual (3 issues/year). Special issues are also published on contemporary areas from time-to-time.
Contents:
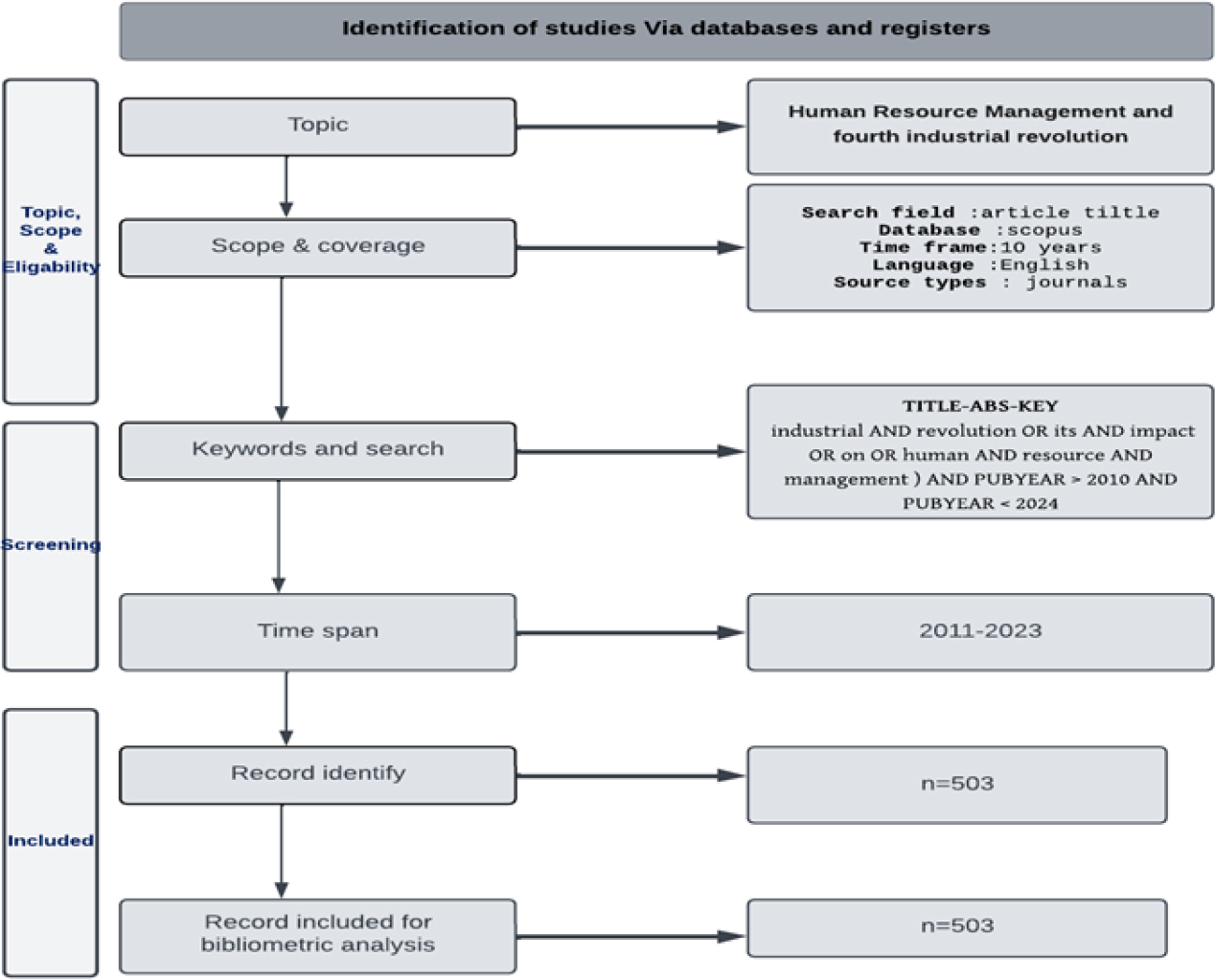
ABSTRACT
The concept of industrial revaluation 4.0 has become a widely accepted idea. Good Human Resource Management (HRM) is necessary for an organization to be successful. Determining the road map for improving the human resource management in industrial sector requires gaining an initial awareness of the body of knowledge regarding HRM in the context of Sector 4.0. Our objective was accomplished through a methodological examination of the literature. It also involves a plan and an approach that is consistent for the process of effectively managing personnel in the industry. Through a scientometric study, we attempted to conduct an effective examination of A review on the 4.0 Industrial Revolution and Its Impact on Human Resource Management Trends. The entire study makes use of Scopus as its database, and the years 2012 to 2023 are considered to be the era under examination. According to the findings of the publication study, the number of publications in Scopus is growing at a rate of 53.44% each year. This research was a collaborative effort between 2800 different researchers who published 916 documents, out of which 32.86% were written by authors from multiple countries. here were 30.39 citations on average per document. As a result of this analysis, we concluded that research in this field may be maintained to better understand the industrial revolution and its impact on human resource management, both of which are effective means by which organizations can be controlled.
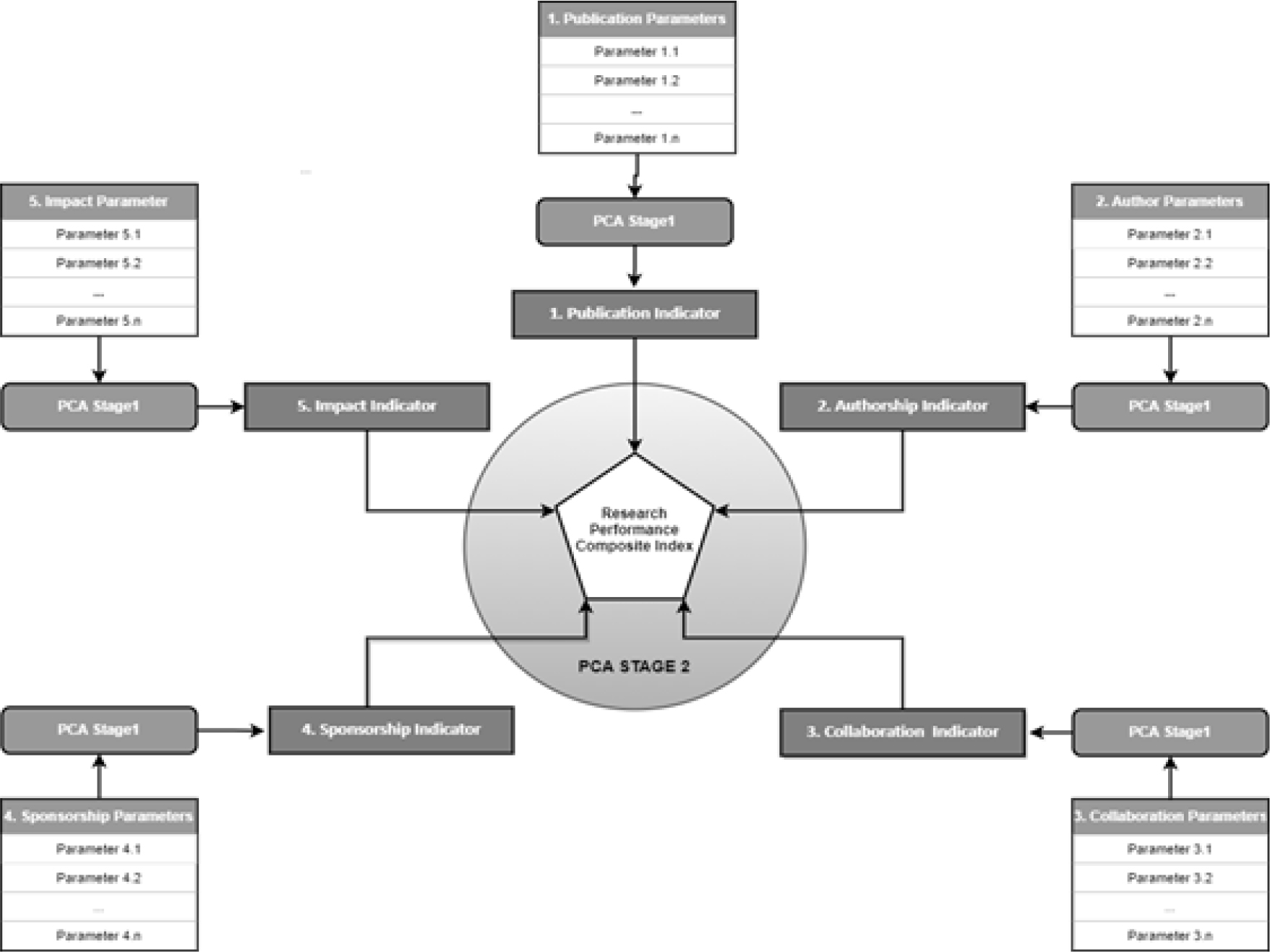
ABSTRACT
Research performance evaluation and ranking are crucial, as policymakers, funding institutions, administrators and researchers rely on these evaluations for resource allocation, strategic decision-making and policy formulation in research and innovation. Bibliometric data have been thoroughly used in measuring research performance and quality at the institutional level using publication and citation-related metrics. This study introduces a novel, multistage PCA-based composite index that integrates five aspects of bibliometric data, namely publication, authorship, collaboration, sponsorship and impact or citation. These aspects represent input, process, output and outcome elements of a system. This data-driven approach provides a comprehensive benchmarking tool to rank countries’ research performance. As a use case, the composite index is used to compare the performance of blockchain research between countries based on a wide selection of bibliometric data prepared to accommodate the demographic variation among countries. The ranks obtained from the composite index perform better than the h-index in differentiating lower-ranked countries by incorporating collaboration, authorship and sponsorship parameters alongside publication and citation metrics, contributing to the index’s robustness. The implications of this study suggest that research performance evaluations should consider a wider set of factors to better reflect the complexities of national research ecosystems. Future policy decisions could be enhanced by adopting such comprehensive benchmarking tools, encouraging strategic decisions such as resource allocation, talent development and collaboration initiatives.
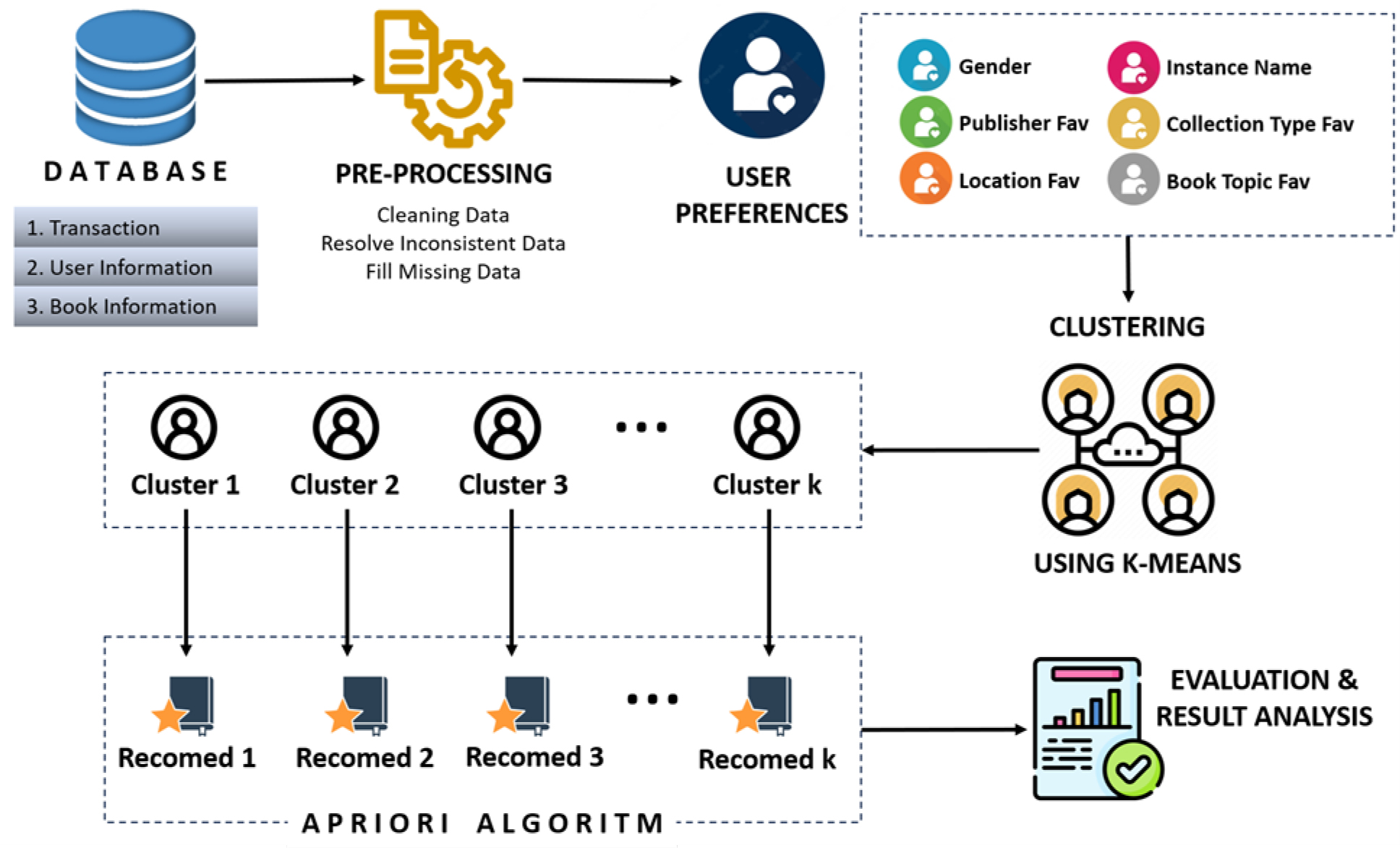
ABSTRACT
The library serves as the primary information source and has a significant impact on raising educational standards. This study aims to improve library service quality by developing a personalized book recommendation system. The personalization of the recommendation system is the design of a prediction model for books that each user will borrow based on the user's interests, behavior and other related information. The novelty of this study lies in the combination of K-Means Clustering and Association Rule techniques to create a more accurate recommendation model tailored to each user's preferences, behavior and other relevant information. One common issue in building recommendation systems is the Cold Start Problem, which refers to the challenge of making accurate recommendations for new users or items with little to no historical data. Therefore, K-Means Clustering is utilized to segment users based on their borrowing patterns, which helps address the Cold Start Problem by recommending popular books within each cluster. User information from book loan transactions is used as input for the clustering model. Next, a recommendation model for each cluster will be made using the Apriori algorithm. Apriori was chosen for its simplicity, low computational cost and ability to efficiently identify frequent patterns, making it ideal for large datasets. This study trials the number of clusters between k=3, 4, 5 and 6. The best results for the recommendation model used a combination of 6 clusters and the Apriori algorithm. The application of the clustering method can improve the recommendation model with a difference of 3.61% average accuracy, 0.67% average precision, 3.61% average recall and 0.49% average F1 score compared to the recommendation model without clustering method.
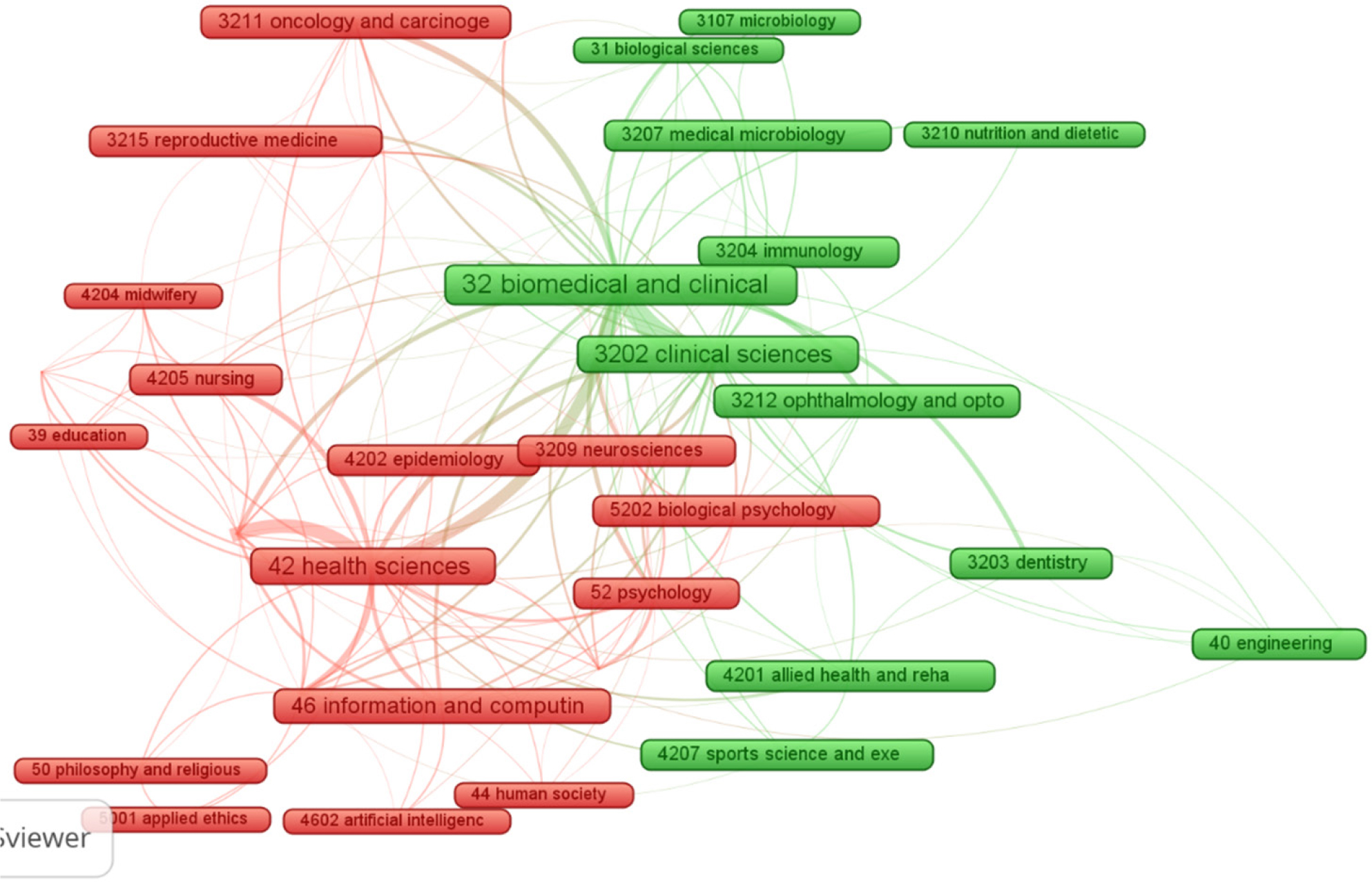
ABSTRACT
This study investigated the application of Large Language Models (LLMs), particularly ChatGPT and Bard, in biomedical and clinical sciences and health sciences via a combination of the altmetric approach and scientometric approach. This study analyzes Altmetric scores across various journals and FoRs, focusing on 942 publications since the launch of the ChatGPT in November 2022 (up to 13 November 2023) in Biomedical and Clinical Sciences and Health Sciences. Key findings highlight the growing impact of LLMs in the biomedical and health sciences, as evidenced by high Altmetric Attention Scores. Discussions revolve around ethical issues such as data privacy, AI biases and LLMs' role at the intersection of computational linguistics, AI and healthcare. The findings underscore the potential and challenges of LLMs in healthcare, emphasizing the need for enhanced accuracy, reliability and social acceptance. This study not only presents current trends and impacts but also provides key recommendations for advancing LLM technology, fostering interdisciplinary collaboration and establishing robust validation and regulatory frameworks to successfully integrate LLMs in biomedicine and health. These insights are crucial for guiding future advancements in healthcare research and practice.
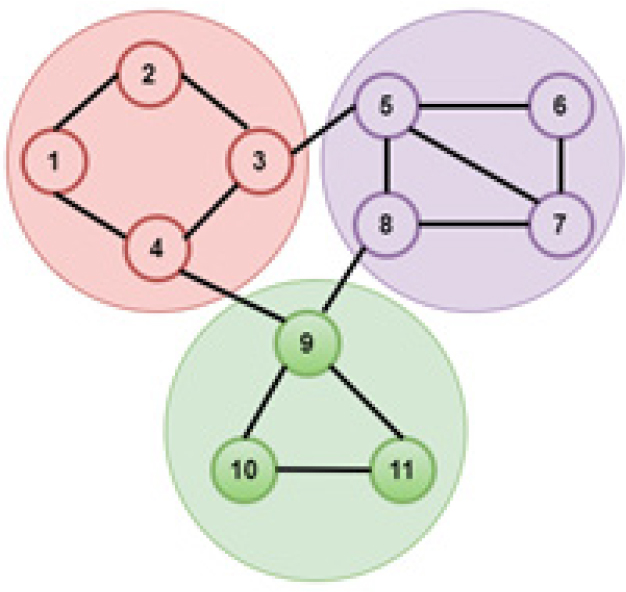
ABSTRACT
Many practical problems and applications are characterized in the form of a network. If the network becomes huge and complex, it becomes very difficult to identify the partitions and the relationships among each of the network's nodes. As a result, the graph is divided into communities and several community detection methods are proposed to associate those communities. The formation of virtual clusters or communities often occurs in networks due to the likelihood of individuals with similar choices and desires associating with one another. Detecting these communities holds significant benefits across various applications, such as identifying shared research areas in collaboration networks, detecting protein interaction in biological networks and finding like-minded individuals for marketing and suggestions. Numerous community detection algorithms are applied in different domains. This paper gives a brief explanation of existing algorithms and approaches for community detection like Louvain, Kernighan-Lin, Girvan Neuman, Label Propagation and Leiden algorithms as well as discusses various applications of community detection. We have evaluated our comparison with six different datasets namely biocelegans, ca-netscience, usair97, webpolblogs, email-univ and powergrid for comparing the efficiency of the methods. The modularity and conductance scores are used to assess the caliber of the partitioned community. A special emphasis on the comparison of these community detection methods is concerned and how the quality resembles and the time taken for its evaluation. We have evaluated all these algorithms and concluded that Louvain and Leiden community detection algorithms are used for effective community division in terms of its structure and time.
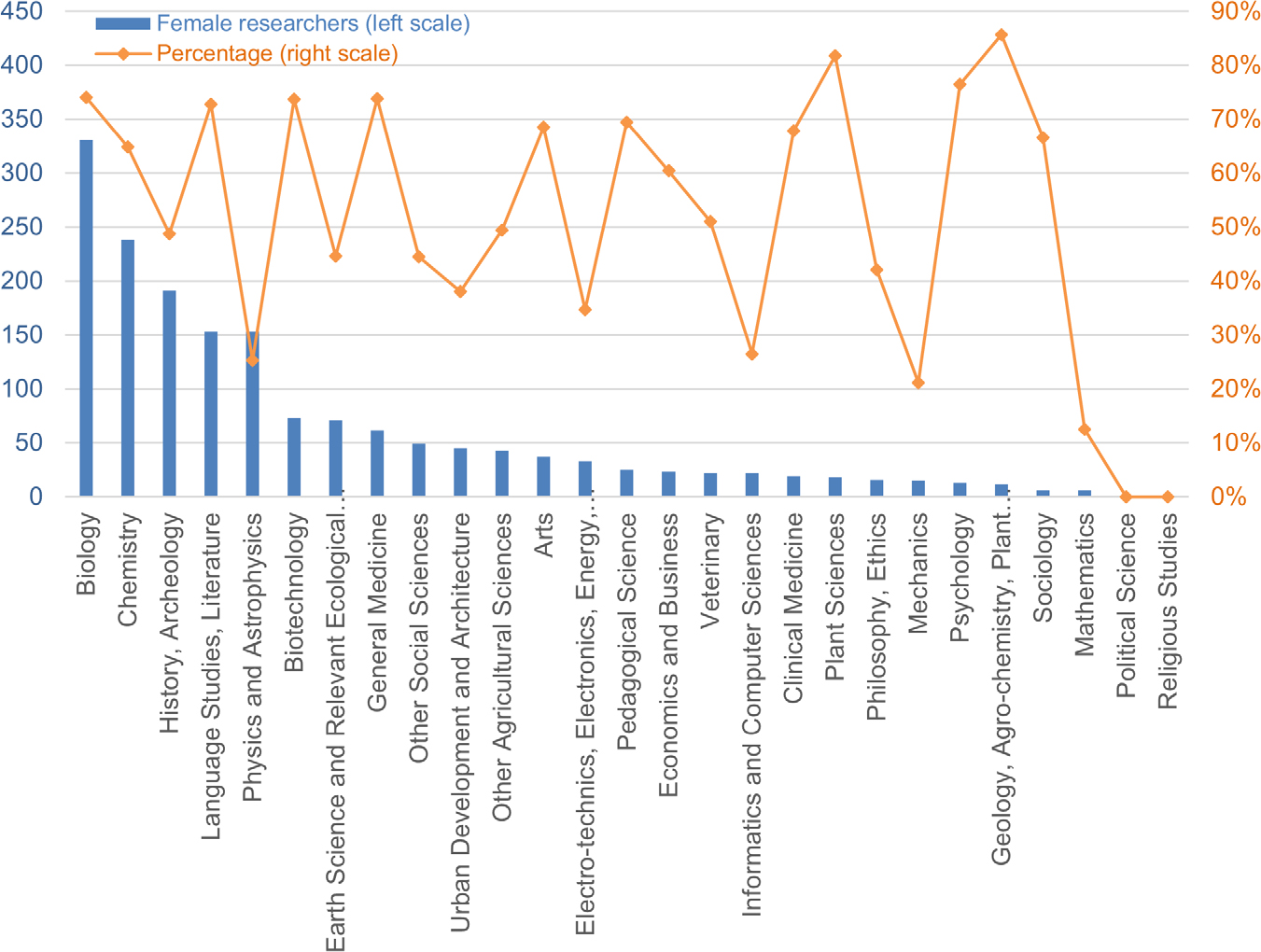
ABSTRACT
This article investigates the role of women scientists in the Republic of Armenia, specifying their involvement and impact on the scientific community. The study is conducted from the dual perspective of biographical data (regarding age, title, academic position) and bibliometric data (regarding publications produced and respective citations). Through the lists provided by the Armenian Higher Education and Science Committee (HESC) and compiled by the Center for Scientific Information Analysis and Monitoring (CSIAM), academic biographical information on female scientists working in research organizations/institutions in the Republic of Armenia was identified. In addition, bibliometric data on the respective publications and citations were collected using the Web of Science database. Armenian women scientists make up nearly 52% of researchers in Armenia, significantly exceeding the global average of 30%. They are primarily engaged in the humanities and social sciences, chemistry, medicine and biology. While the number of women holding Ph.D. degrees has increased, women scientists with the title of so-called doctor of science still remain a minority. Women are underrepresented in leading scientific positions (heads of departments, laboratories, institutions, etc.,). The number of WoS Core Collection publications by women scientists in Armenia has increased almost ninefold since 1991 and women have (co-) authored almost 27% of the totality of publications. Women scientists mostly published their articles as members of research teams. This investigation can help to understand the overall development of the role and share of women scientists in Armenia and, by analogy, also in different corners of the world.
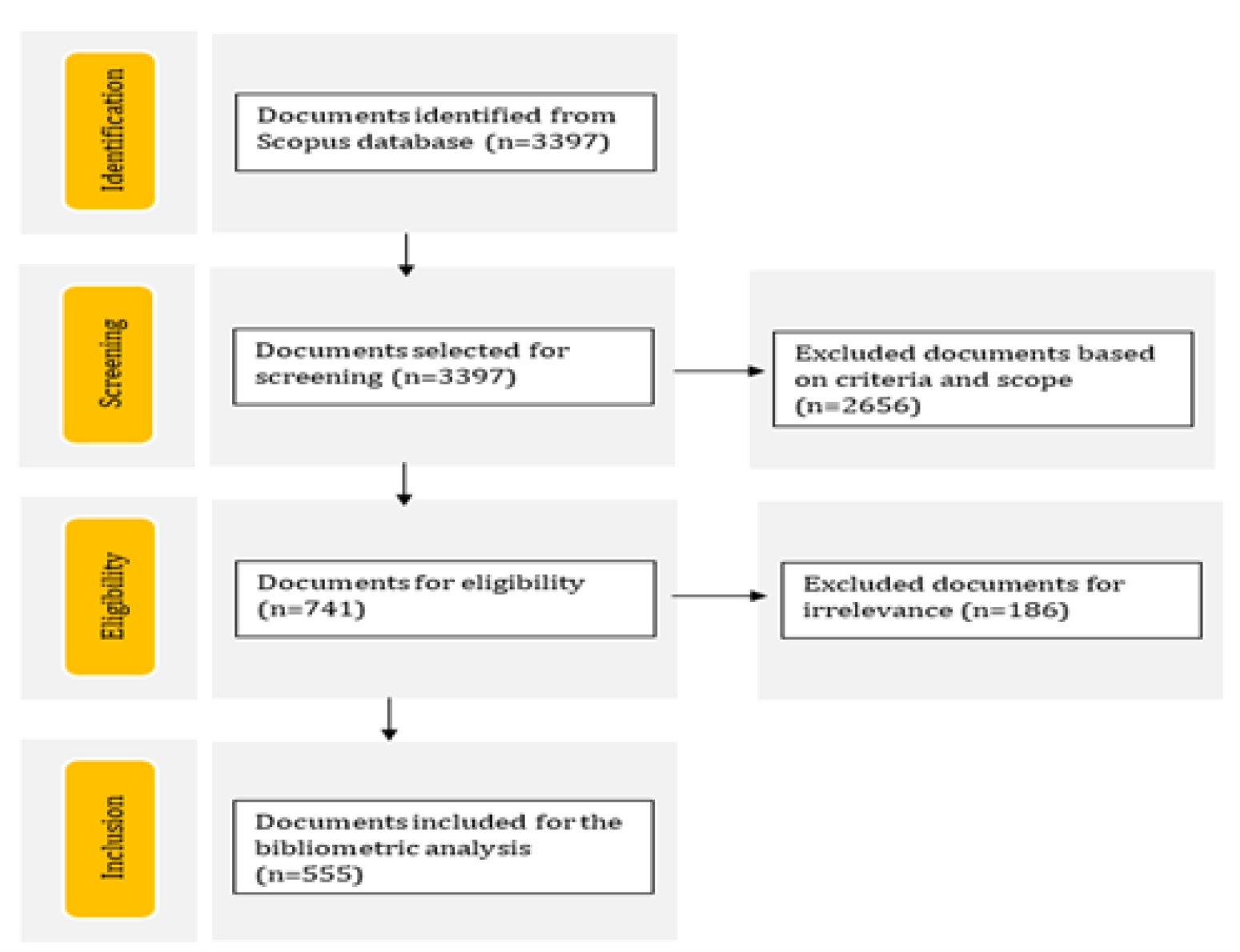
ABSTRACT
This bibliometric analysis elucidates the evolution and impacts of Project-Based Learning (PBL) within vocational education, emphasizing its relevance in translating career goals and addressing educational gaps, particularly from 1993 to 2023. Analyzing 555 Scopus-indexed documents, this study utilized advanced bibliometric tools to uncover PBL's growing appeal and its innovative pedagogical integration into design thinking, challenge-based education, and multidisciplinary approaches. Our findings highlight PBL's significant outcomes, including its role in bolstering STEM curricula and fostering critical skill development, thus bridging the gap between theoretical knowledge and practical application essential for the workforce. Despite the documented benefits, our analysis also identifies substantial gaps in research, particularly the scarcity of longitudinal studies on PBL's long-term effectiveness and its comparative efficacy against traditional pedagogies within diverse educational contexts. The study underscores PBL's utility in vocational education as a transformative approach, aligning with dynamic career expectations and the requirements of the knowledge economy. By offering a detailed thematic evolution and pinpointing influential contributions, this research serves as a strategic guide for educators and policymakers, advocating for informed, future-ready educational strategies that embrace experiential learning to meet the evolving needs of students and the labor market.
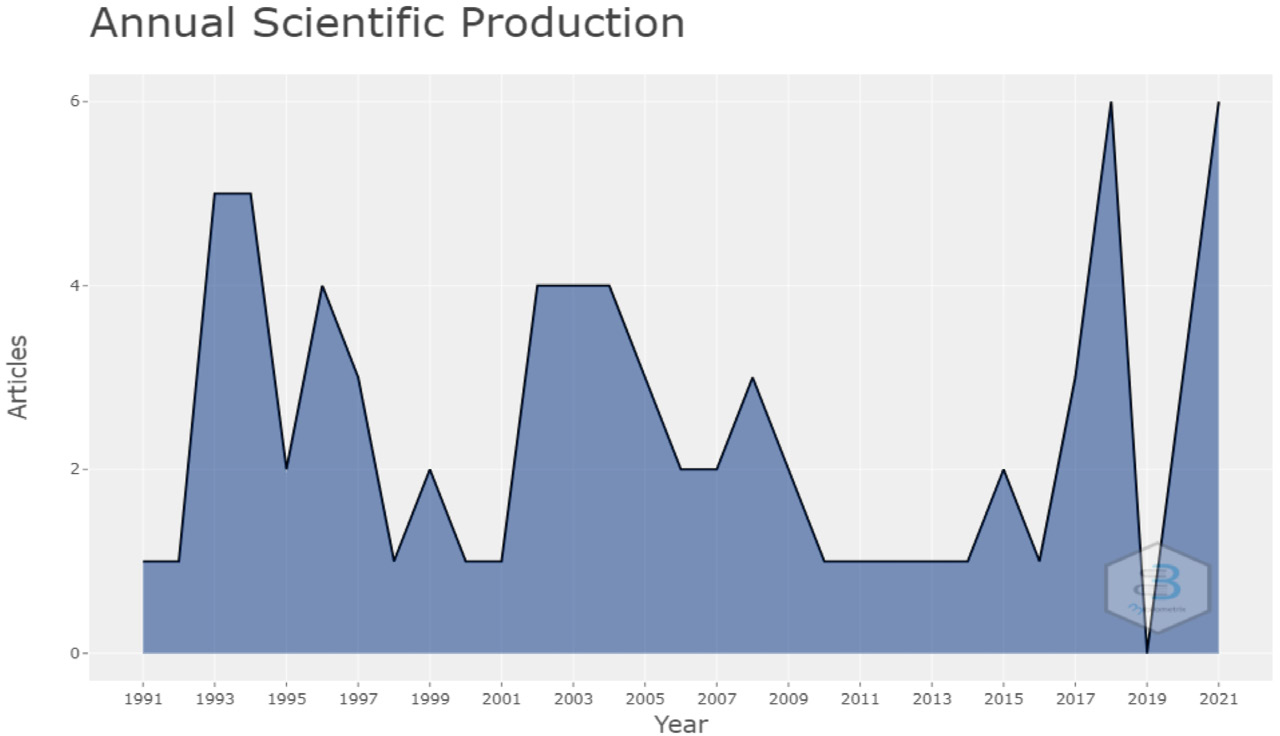
ABSTRACT
The primary focus of this study is to identify barriers and facilitators to women’s participation in the field of Urban Planning through a comprehensive literature review. In addition, this work focuses on a quantitative and qualitative thorough assessment of 76 research publications. The study identified the research gap by conducting bibliometric analysis using R-Studio tools version 4.1.2 and finding reveals that hardly any articles are found on women role in urban planning and barriers to women’s progression in urban planning worldwide and in India up to now. Significant barriers include discrimination in the built environment, a perceived glass ceiling in the workplace, a lack of mentoring policies, a lack of training in organizations, unequal pay wages, a lack of women's participation in the planning field and a lack of data on women’ professional skills in India are observed. Nobody can disagree that women have a difficult time managing their personal lives and jobs under the masculine professional planning paradigm. So, to resolve all the barriers, facilitators are found. The present study will pave a path to conduct research in the field of women’s professional advancement in urban planning.
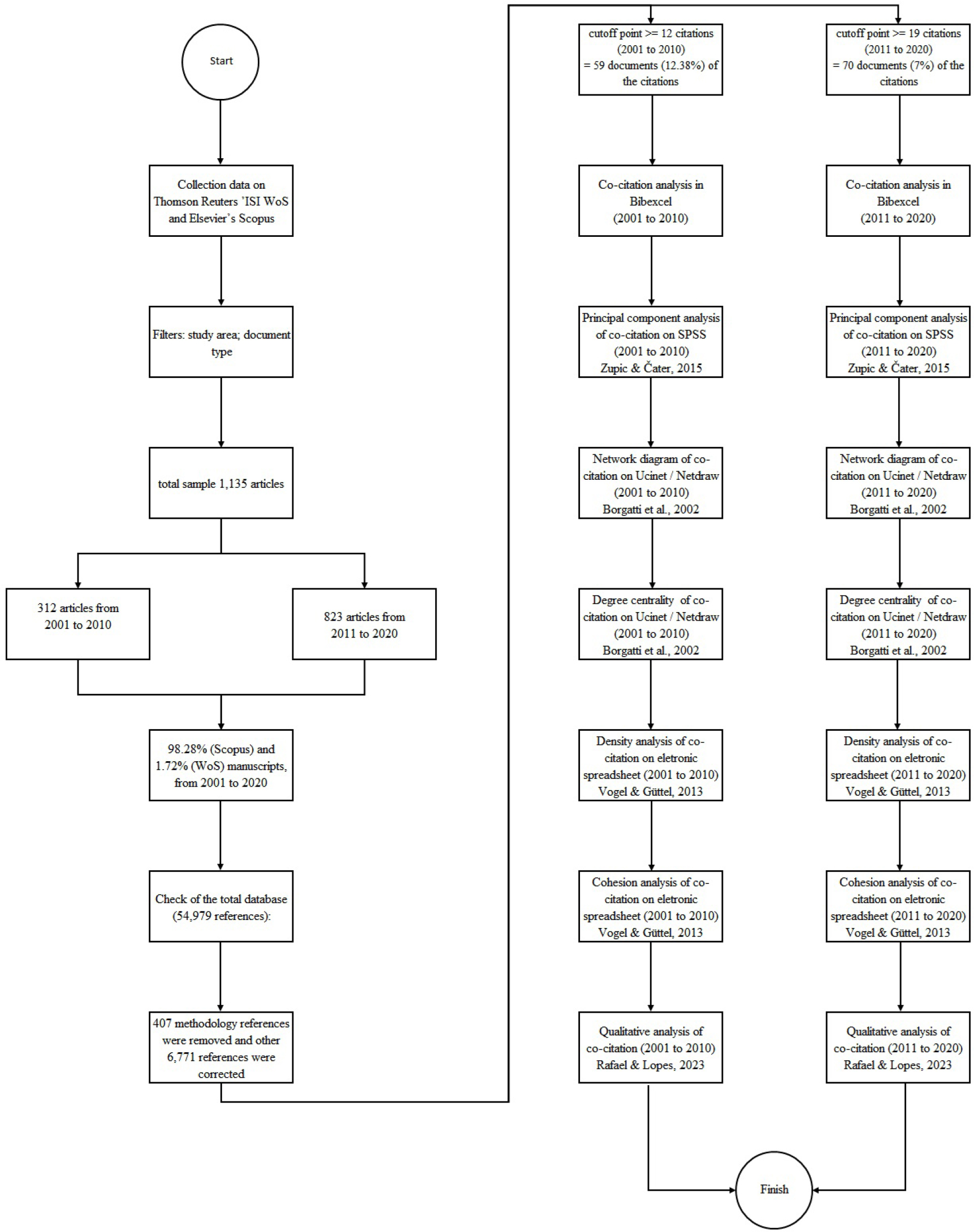
ABSTRACT
Framing effect is a cognitive bias that leads people to display a preference for one option between alternatives that are factually equivalent but differ in the way they are presented. The effect was demonstrated by Tversky and Kahneman following the development of Prospect Theory, gained momentum in the early 1980s, and continues to be a widely researched topic. Employing a bibliometric co-citation analysis, the current paper maps the intellectual structure of research in framing effects of the last 20 years of publications. The results reveal four clusters that represent the main areas of concentration of scholarly research on framing, providing an interesting case of the scientific development process. The first cluster reveals that framing research, although initially based on Prospect Theory, which confronted traditional expected utility theory in the field of economics, was mainly advanced in the field of psychology. Initially applied to choices between risky-choices, scholars expanded framing research to contexts where no risk is at stake, and other theories and cognitive, emotional, and motivational mechanisms were proposed. The second and third clusters revealed that framing research has been mainly applied in the fields of communication / political sciences and health, respectively. The final cluster, composed of seminal papers on framing, reveals that the framing effect remains relevant and still requires additional studies to better assess its effects on different profiles of individuals, scenarios, contexts, and methodological approaches.
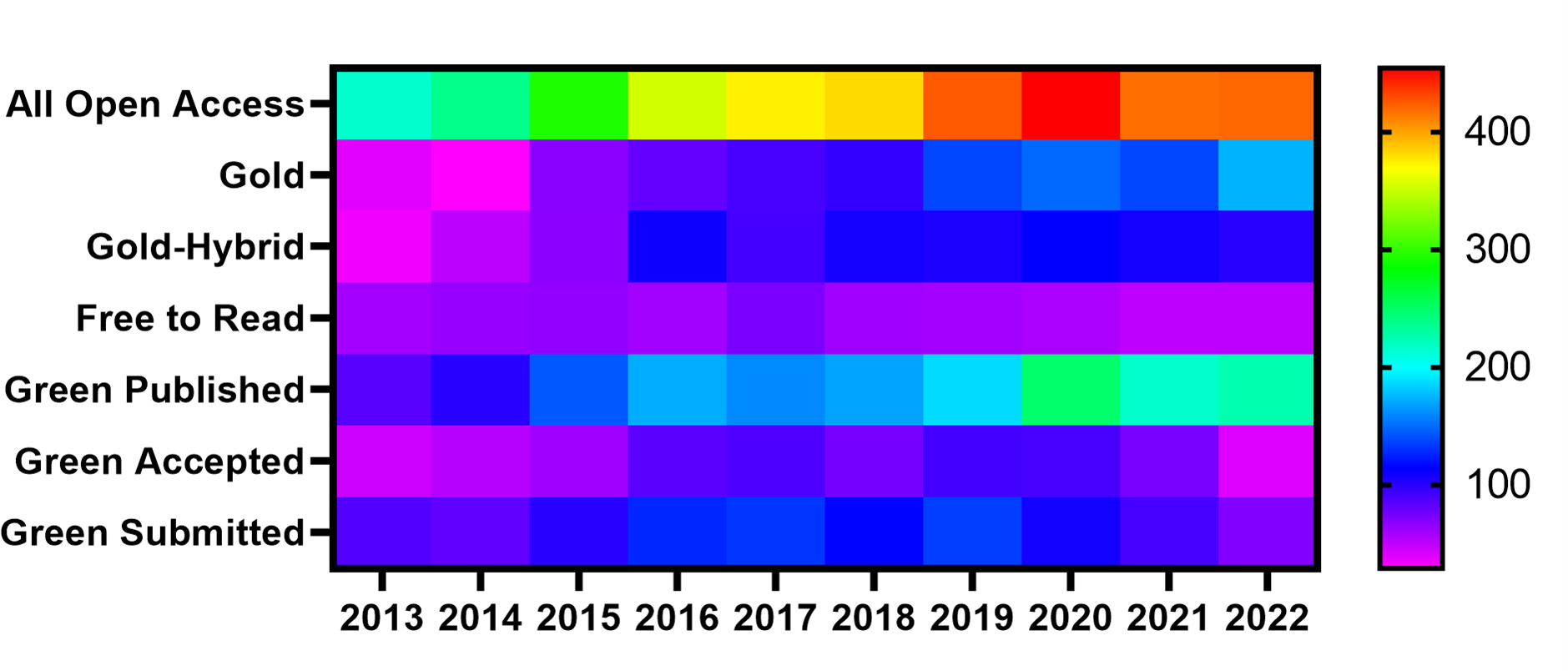
ABSTRACT
This study addresses the critical need for understanding journal publication trends in carbon research, which plays a vital role in advancing the field, guiding research priorities, informing policy decisions, and assessing the sustainability of efforts to combat carbon-related challenges. The primary objective is to analyze 19,155 highly cited articles and review papers published globally between 2013 and 2022, indexed in the Science Citation Index-Expanded (SCIE) dataset of the Web of Science Core Collection. The study examines key aspects of publication platforms, including publishers, journal titles, affiliated categories, and open access status, to assess changes in publication dissemination. Text mining techniques were employed to analyze author keywords and abstracts, identifying emerging trends in carbon research across multiple academic disciplines. Additionally, the geographic distribution of researchers was studied to track shifts in the global landscape of expertise in this field. The study introduces an innovative 3-dimensional analysis system, categorizing research subjects into platform (x-axis), text (y-axis), and scholars (z-axis). Methodologies such as text mining, CiteSpace, and ArcGIS were applied to analyze the trends over the past decade, offering new insights into the evolution of carbon research. This approach provides a comprehensive framework for understanding the development of carbon research, offering valuable tools for scholars and institutions engaged in the field.
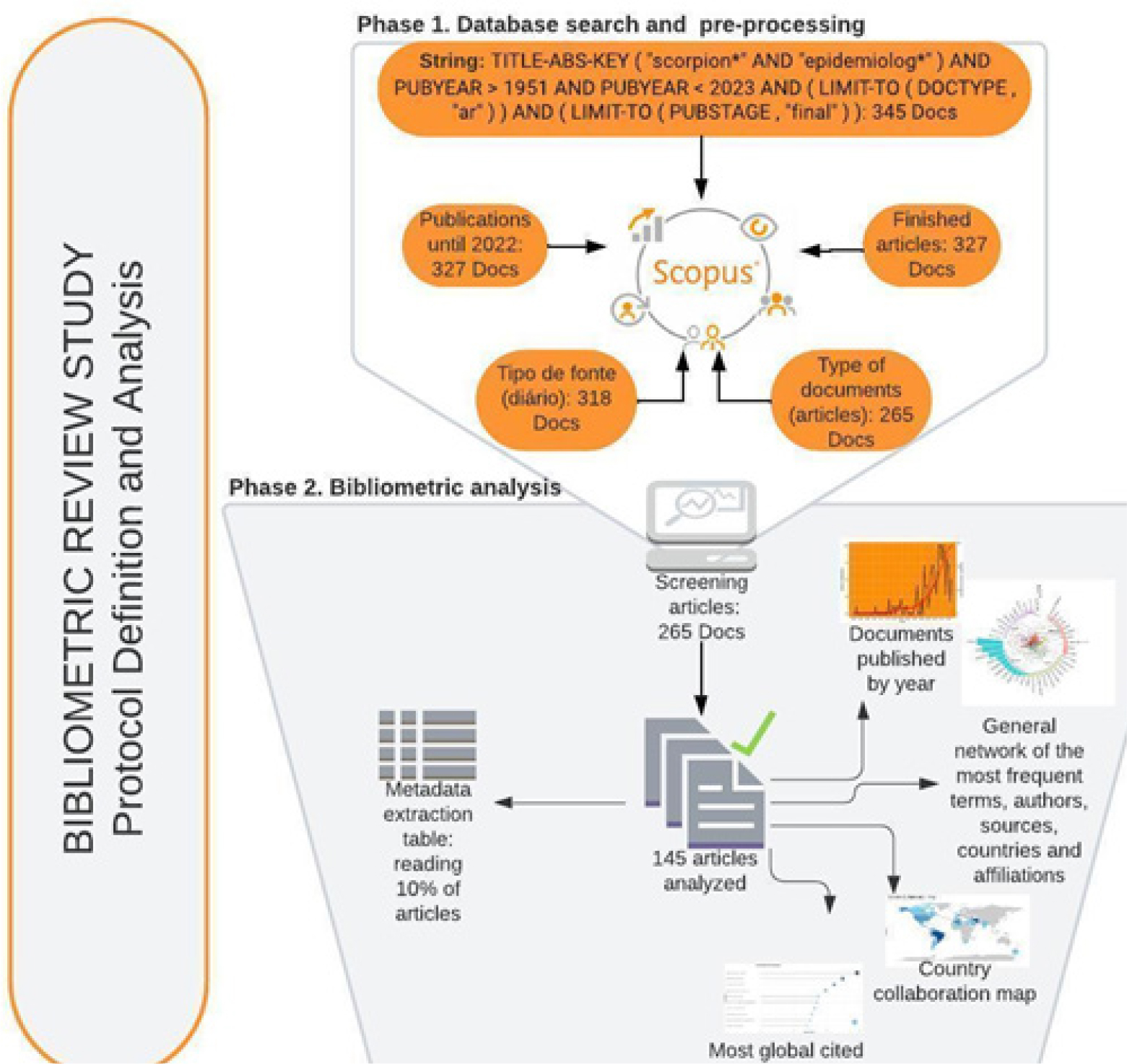
ABSTRACT
Scorpionism has driven studies around the world on data relating to epidemiological and clinical aspects in humans. From this perspective, a bibliometric review was carried out to analyze the historical and evolutionary construction of the epidemiology of scorpionism to identify the main authors, journals, countries and years of emphasis, as well as the most cited studies and most frequent terms, through quantitative and qualitative data extracted from the manuscripts. The review also investigated studies that explored the association of the topic with environmental, climatic and anthropogenic factors. The analysis was based on publications indexed in the Scopus database, covering the period from 1952 to 2022. The result of the global analysis, based on 145 articles, demonstrates a growing evolution of the topic. Brazil (17.93%) and Iran (17.24%) emerged as the most productive countries in terms of research production. However, the US, Mexico, Algeria and Canada (11.11%) have the most substantial collaborative efforts. The magazine “Toxicon” stands out as the predominant source of scientific dissemination (25%). Only eight authors have unique authorship and the author Semlali Ilham emerged as the most prominent. Universities were the affiliations with the highest productivity. The results of the metadata extraction analysis, based on a sample of 14 documents, revealed consistent and sustained epidemiological data since 1982. Of these, only one study (7.1%) from French Guiana used environmental variables to elucidate data on the occurrence of scorpion stings. The genera Tityus, Centruroides and Androctonus emerged as the most representative among the works compiled and evaluated.
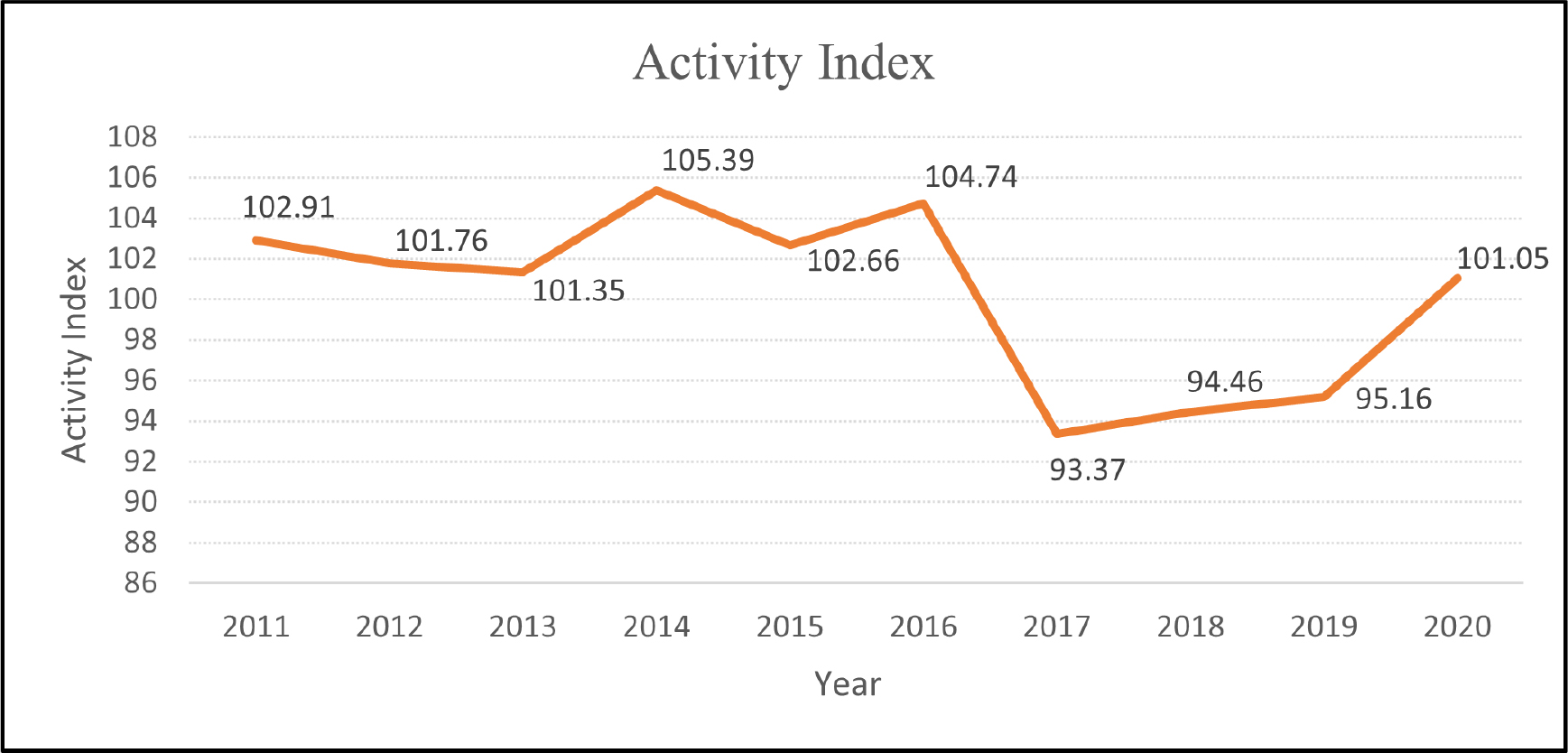
ABSTRACT
This study delineates India’s pharmaceutical sciences research landscape from 2011 to 2020 through a scientometric analysis of data from the Scopus database. During this period, India contributed 241,142 publications, constituting 6.90% of the global total of 3,493,821 in the field. The analysis revealed significant variations across different dimensions, including year, country, subject area, funding agency, and leading organizations. Key metrics such as annual growth rate, relative growth rate, doubling time, publication efficiency index, citations per publication, research quality index, research priority index, and h-index were employed to assess overall development, research focus, and quality. The production of research output exhibited a steady year-on-year increase, reaching its zenith in 2016, with pharmacology leading at 115,558 publications. The Activity Index reveals increased research activity in India from 2011 to 2016 and in 2020, alongside a significant rise in citations for publications from 2019 and 2020. The quality of Indian contributions from 2011-2014 and 2019-2020 exceeds the global average, with research quality index values range from 1.00 to 1.09. Together, the top ten sponsors have funded 69686 publications, and out of them, 18.80% of publications were funded by the Department of Science and Technology, underscoring government support for scientific research. India's contribution of 6.90% to global pharmaceutical publications is unexpectedly low, given its pivotal role in drug production. This disparity underscores the necessity for improved research capabilities and collaboration. The academic institutions dominate the top contributors, with CSIR as a notable exception. While India's research efficiency has been robust from 2011 to 2016, subsequent years' troubling descent signals an urgent need to address these challenges to revitalise India's position in the global research framework by creating a conducive environment for groundbreaking research. This can be achieved through the strengthening of research infrastructure and providing access to cutting-edge technologies for researchers, thereby ensuring sustained innovation and global competitiveness.
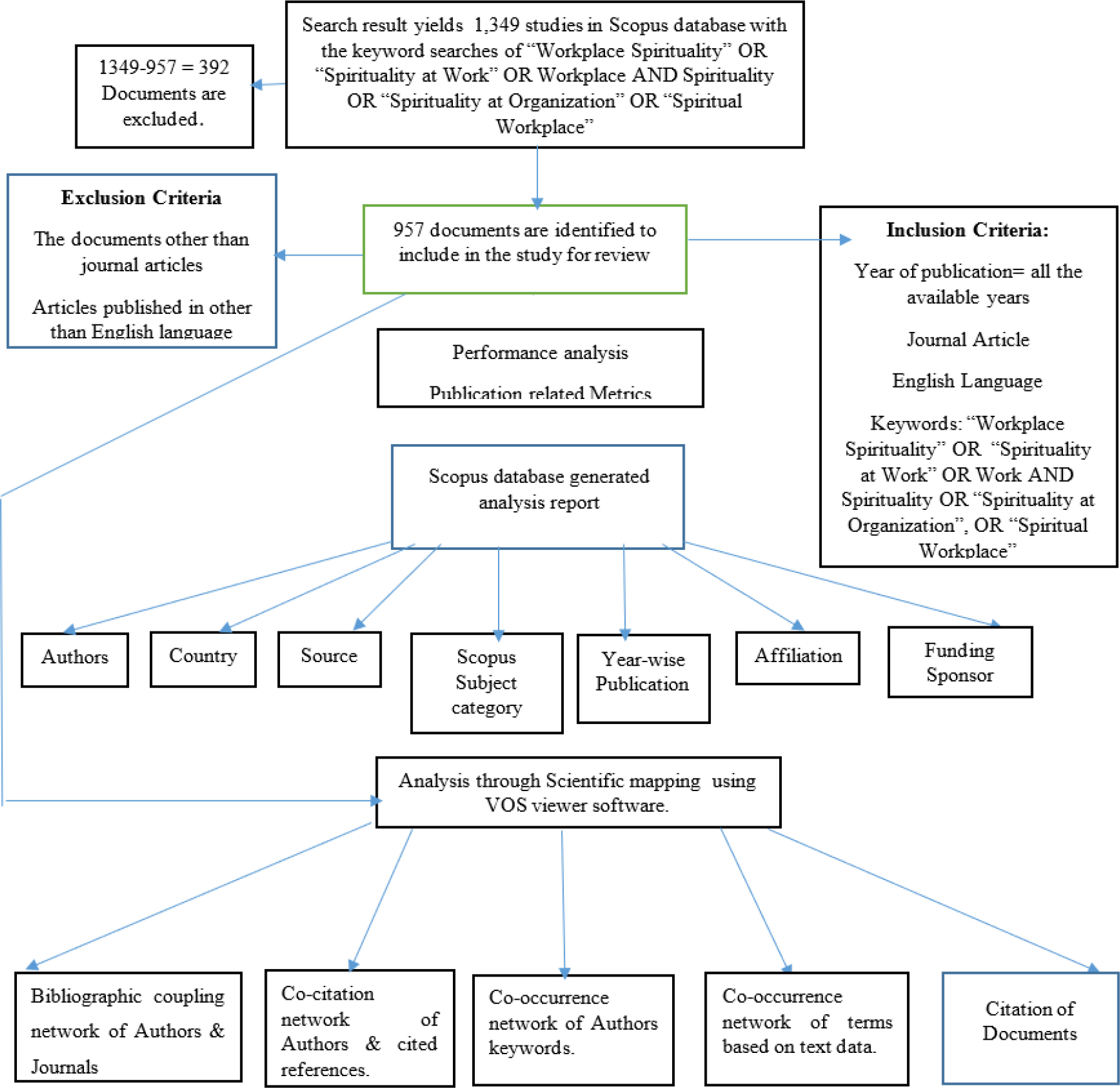
ABSTRACT
The present study aims to understand the publishing trends related to workplace spirituality over the last thirty years and potential areas for future research. For bibliometric analysis, using VOS viewer software, 957 entries are extracted from the Scopus database by the keyword search of “workplace Spirituality”, ‘Spirituality at work”, and workplace AND spirituality, “Spirituality at organization”, OR “Spiritual Workplace”. The publication period ranged from 1995 to 2024. A bibliometric analysis showed that WPS research is interdisciplinary, and covers various subject categories. Three decades of WPS research has shown a significant surge over the years. The United States and India remain the most productive countries for WPS research. The first decade of research is marked by theoretical development, followed by quantitative analysis. Then, the research trend moves towards mediation and moderation analysis to explore the underlying mechanisms behind the relationship between WPS and various other variables. A research gap is identified regarding the lack of a qualitative study or mixed method approach, antecedent conditions of WPS, the Indian model of WPS, and the intervention model of WPS. This study further advances the WPS literature by adding to the scope and applicability of Indian psychology in this domain. It is important to note that this study is limited to the Scopus database, which may affect the generalisability of the findings.
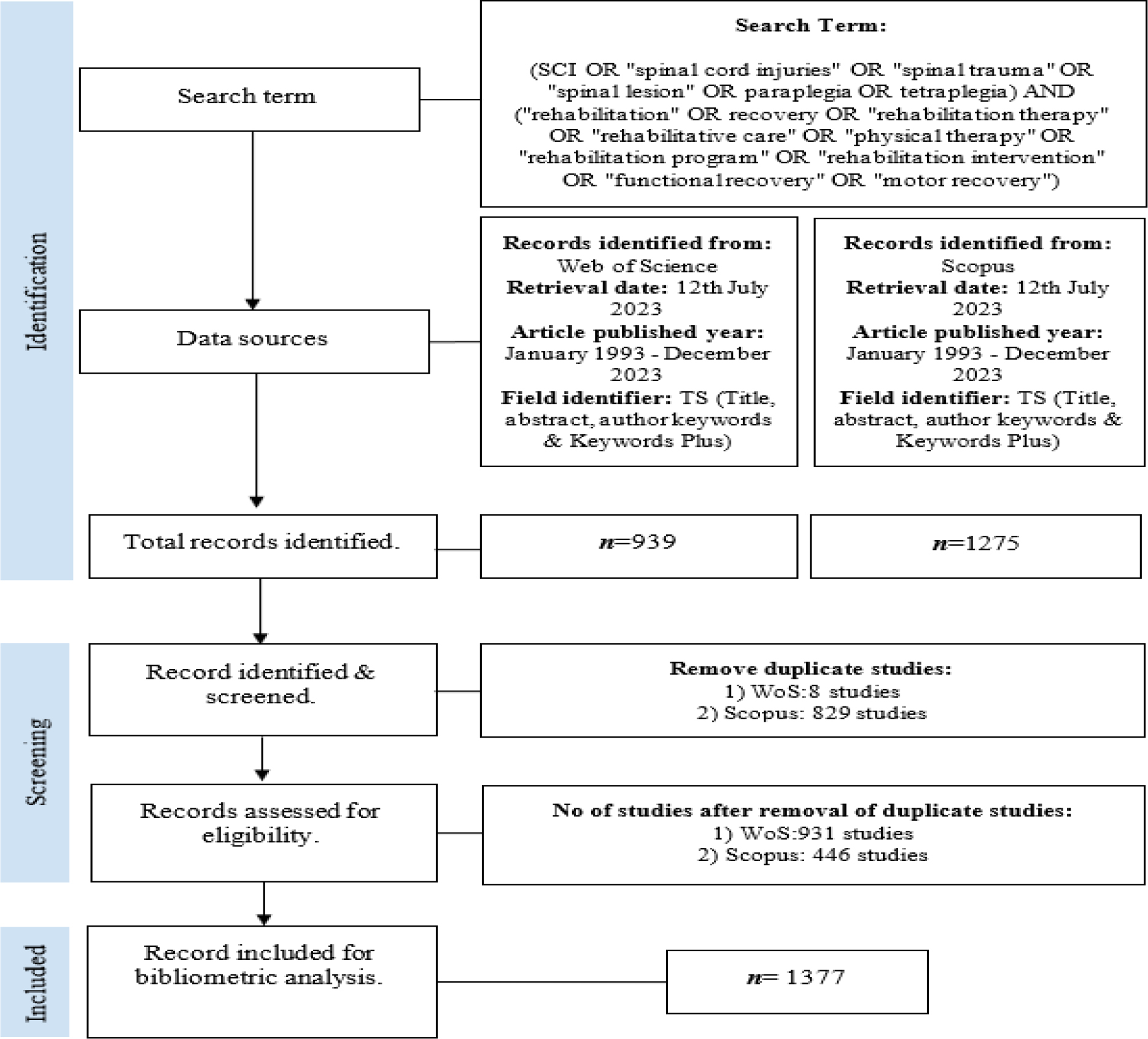
ABSTRACT
Background
Research trends in Spinal Cord Injury (SCI) rehabilitation remain relatively unexplored within the scientific literature. Despite increasing interest, there is a notable lack of comprehensive bibliometric analysis to map and synthesize global research trends in SCI rehabilitation, especially over the last three decades.
Materials and Methods
We conducted a detailed bibliometric analysis using data extracted from the Web of Science (WoS) Core Collection and Scopus databases, covering the period from 1993 to 2023. The analysis employed both qualitative and quantitative approaches, utilizing bibliometric software tools such as VOSviewer to examine publication outputs, journals, authors, institutions, countries, cited references, keywords, and emerging terms in the field. Specific attention was given to identifying trends in technological interventions and research frontiers in SCI rehabilitation.
Results
This study identified 1,377 unique articles for further analysis. The United States emerged as the leading contributor to SCI research, followed by Canada and Australia. Among institutions, the University of Toronto was the most active, with significant contributions also from the University of Groningen and the University of British Columbia. The top research fields in SCI rehabilitation were Neuroscience and Neurology, followed by Sports Science and General and Internal Medicine. Additionally, this study highlighted key thematic areas shaping the field, including recovery and function, social and psychological aspects, neurological and medical complications, assessment and measurement, and psychological well-being.
Conclusion
This bibliometric study underscores SCI rehabilitation as a mature and expanding research field with significant global collaboration. However, there is a pressing need for higher-quality research to further advance the field. Our findings offer valuable insights for researchers to shape future research directions and enhance the impact of SCI rehabilitation studies.
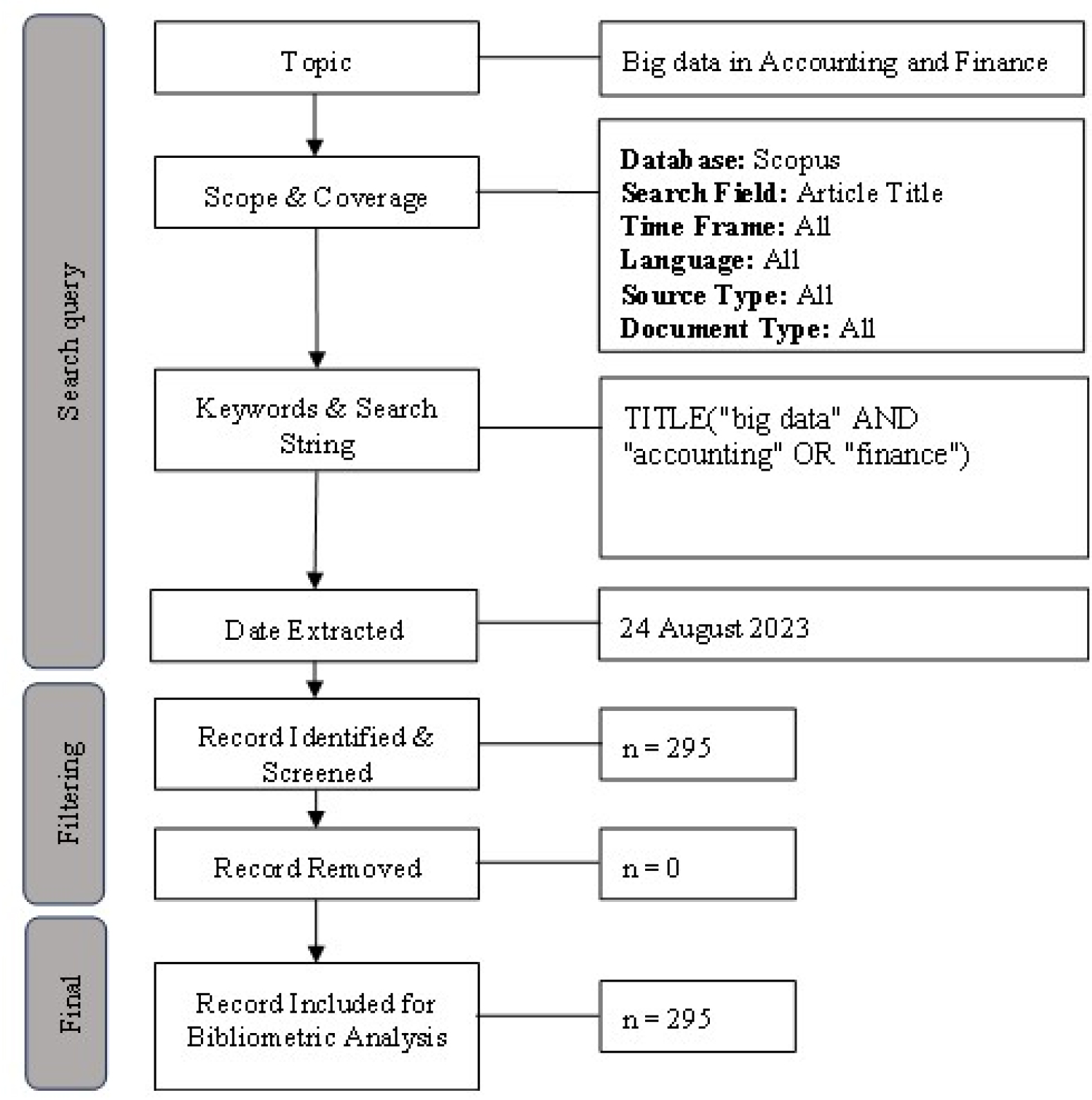
ABSTRACT
This study examines the intellectual structure and research trends at the intersection of big data, accounting, and finance using a bibliometric analysis of 295 Scopus-indexed papers from 2013 to 2023. The study tackles the need to understand collaboration networks and topic evolutions in this dynamic environment. We discovered dominating research clusters, essential contributors, and topic trajectories before and after COVID-19 using co-authorship and keyword co-occurrence analyses. The methodology uses tools like VOSviewer and Biblioshiny to map collaboration patterns and thematic map concentrations. The findings show that international collaboration, notably amongst scholars from China, the United States, and the United Kingdom, is critical to advance the discipline. Traditional subjects such as “big data”, “finance”, and “accounting” dominate keyword research, while emergent topics such as “artificial intelligence”, “cloud computing”, and “risk assessment” represent technological improvements. The COVID-19 pandemic sparked new research avenues, introducing concepts like “neural networks” and “sustainable development goals”. The findings emphasise big data’s disruptive impact on financial and accounting procedures and the need for future studies to address regulatory, ethical, and transdisciplinary issues. This study adds to the literature by providing a complete analysis of big data research trends and a road map for scholars and practitioners to navigate the changing academic and industrial settings.
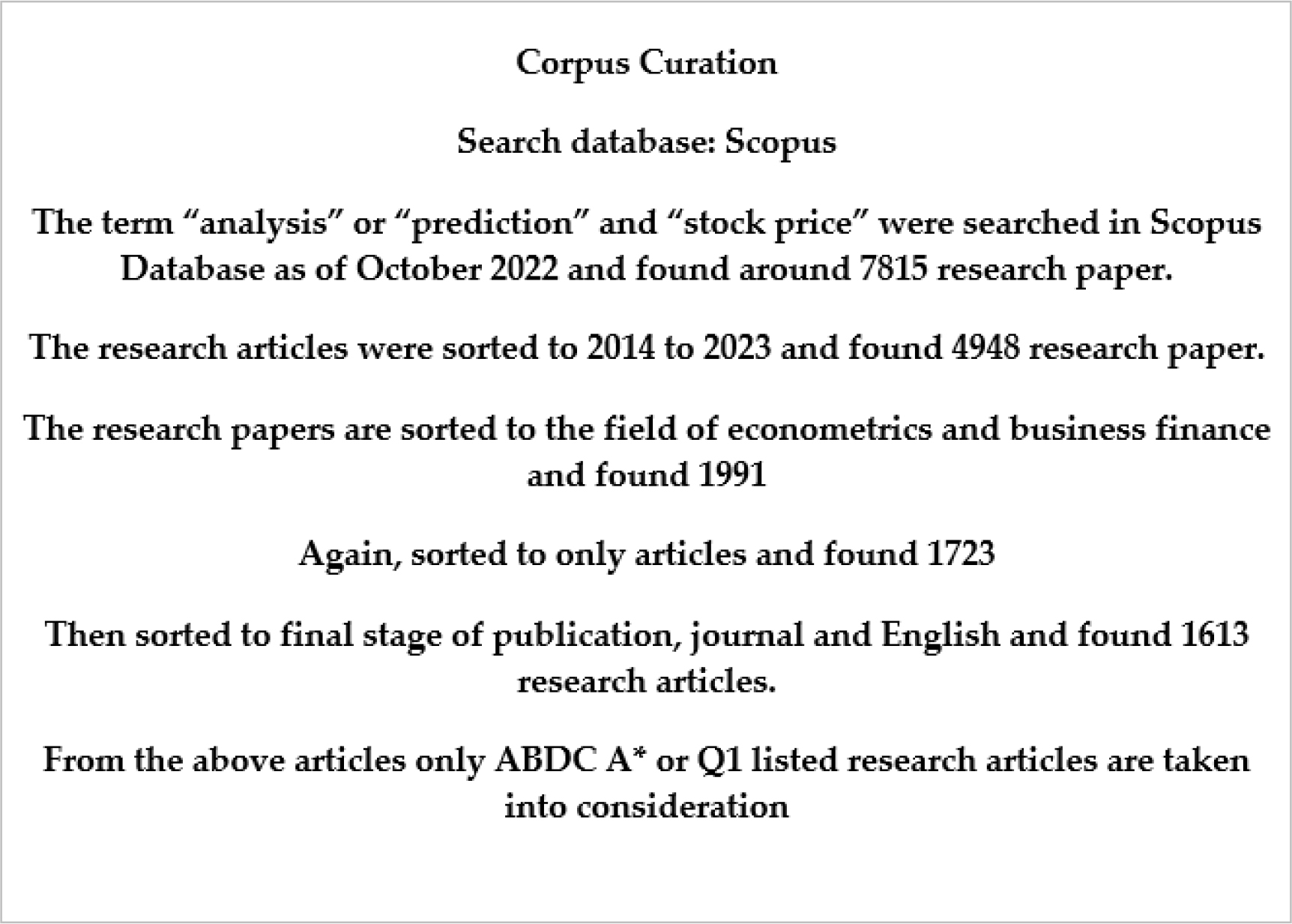
ABSTRACT
Stock market prediction and analysis is an important subject of discussion for stock market prediction and analysis. The aim of the study is to explore literature with respect to stock market and its prediction. The paper focuses on the theme of evolution and conceptual cluster of stock market analysis and prediction based on Bibliometric Analysis. The study mainly derives on three keywords, i.e. stock market, market prediction, as well as stock analysis. For this study, 160 Scopus indexed research papers has been used for analysis. In our analysis the journal of prominence, the most cited authors, most cited articles, co-authorship network analysis, keyword occurrences, clustering and visualization. The outcome demonstrates the trends of research within the specified field and will assist the research community in addressing challenges pertaining to each core concept.
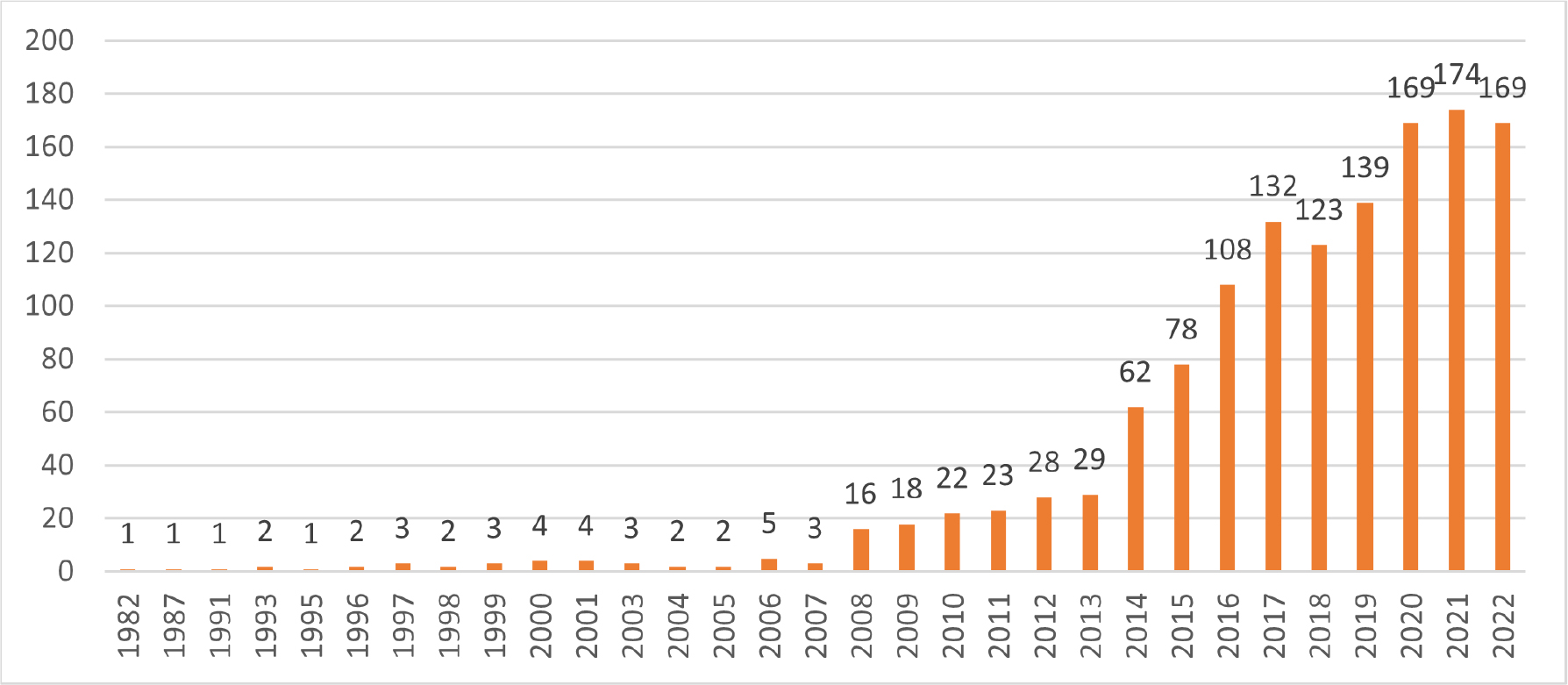
ABSTRACT
Islamic capital market is one of the supporting pillars towards the fast growing of Islamic finance industry. Scholars paid high attention to it, resulting enormous studies being published to accelerate the development of Islamic capital market. This research tries to synthesize those published literatures and mapping the intellectual structure that has been formed and developed around the topic by focusing on the publication trends, key areas of the research, most contributing journals, most influential authors, and collaboration among countries. A bibliometric approach was conducted to achieve the desired result by using Scopus database where 1,336 documents had been successfully retrieved. The research found that there was an upward surge of publication since 2008 with several trending keywords over the years. In addition, two major publishers which have the most contributing journals towards the topic were discovered. We also found three most influential authors within the area. In addition, Malaysia has been noticed to put the highest effort in contributing to the topic while making the most collaboration. Lastly, there are three key highlights for the direction of future research and four implications based on the statistical findings. All these findings shed light and give a significant foundation for future research.
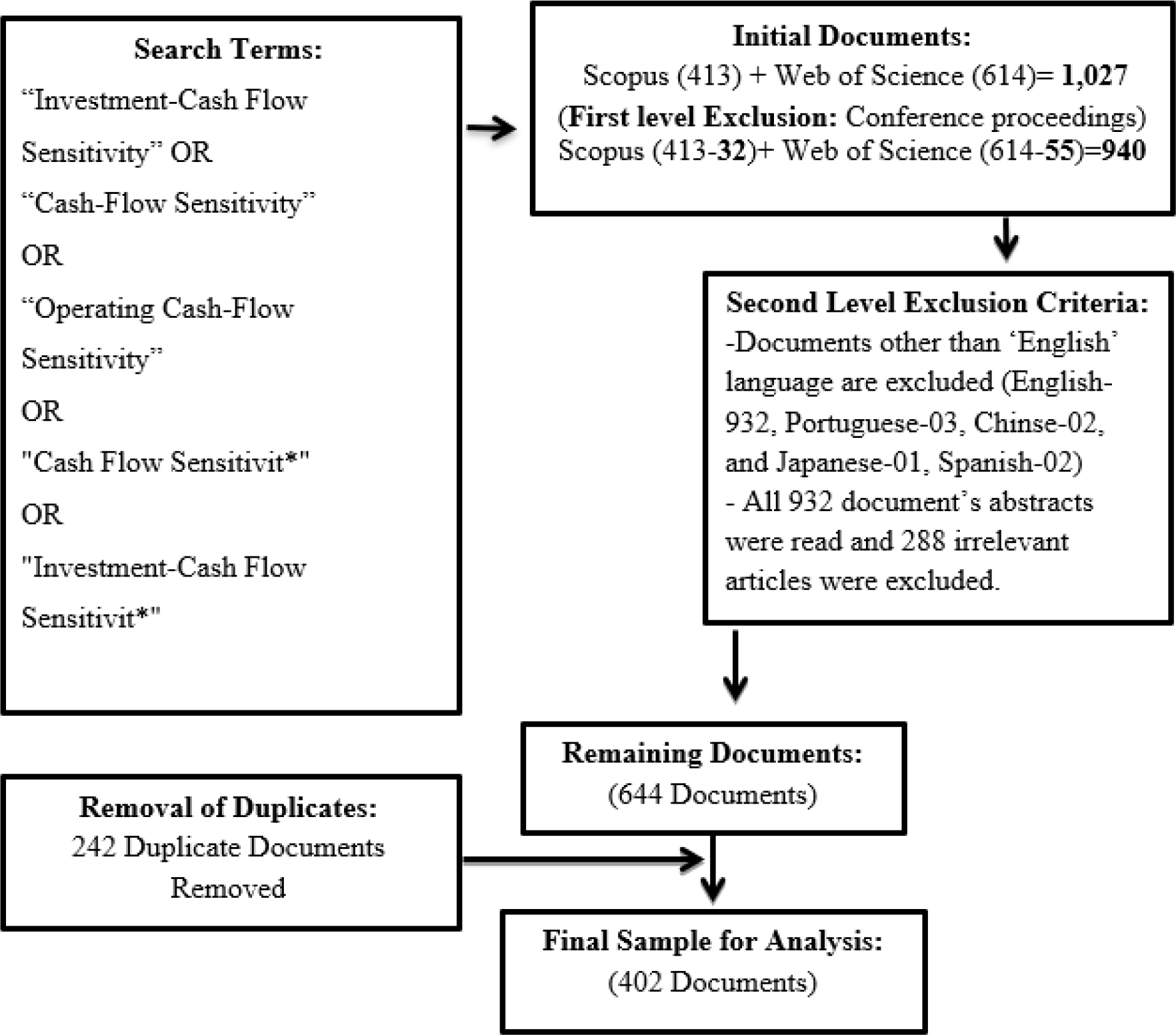
ABSTRACT
This paper undertakes a novel endeavour to bridge the knowledge gap on Investment-Cash Flow Sensitivity (ICFS) through bibliometric analysis, analyzing 402 documents from Scopus and Web of Science databases spanning over three decades (1989-2022). The study not only identifies influential authors and papers but also illuminates major themes, publication trends, active institutions, and countries, thereby delineating the intellectual landscape of ICFS. Moreover, it evaluates current themes, identifies barriers to literature growth, and proposes directions for future research. Furthermore, it is recognized that there is a lack of cross-national collaboration in ICFS research, particularly among researchers from developed and developing nations. Notably, the underrepresentation of short-term investments, macroeconomic factors, and Environmental, Social, and Governance (ESG) considerations in the ICFS research domain is highlighted and can be used in future studies.
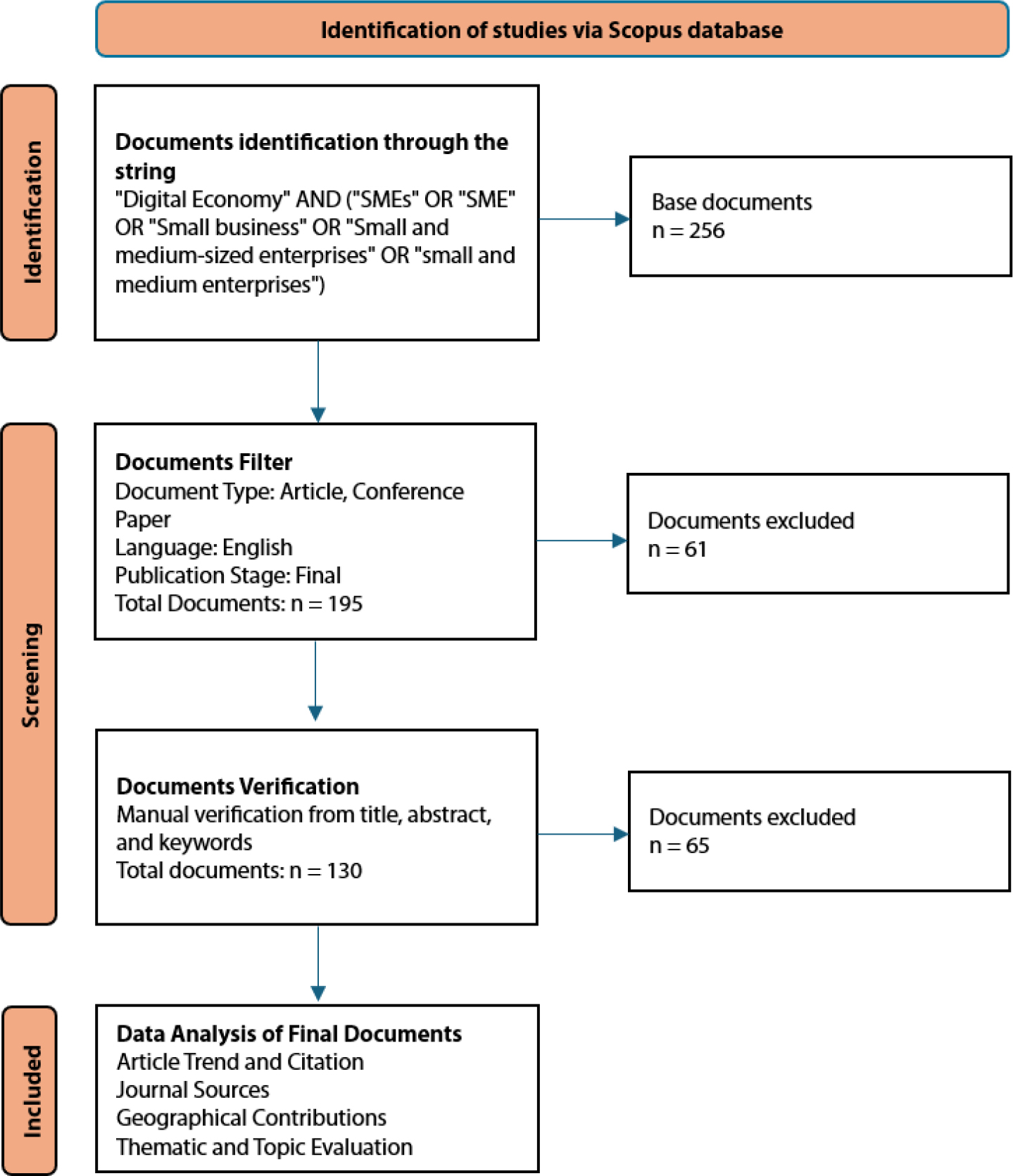
ABSTRACT
This study aims to map the research landscape on the digital economy and Small and Medium-sized Enterprises (SMEs) through bibliometric analysis of 130 Scopus-indexed articles. The study identified publication trends, influential sources, geographical contributions, and the evolution of research themes from 2001 to 2024. Data were collected using a custom query on Scopus and analysed with R Studio software and the bibliometrix package. Results show a significant increase in academic interest in this topic, especially after 2015, which is in line with the wave of global digital transformation. Key findings include the dominance of themes such as "digital economy," "e-commerce," and "digital transformation" as highly developed core themes, while themes such as "small and medium-sized businesses" and "economy and society" were identified as emerging but under-explored areas. The study also found significant geographical disparities, with China leading in number of publications, but the UK and Pakistan showing the highest impact based on average citations per article. This study contributes by filling the literature gap through mapping the evolution of research themes and providing guidance for future research to focus on less developed yet crucial areas. The findings provide valuable insights for researchers, policymakers and practitioners in supporting SMEs to maximise the potential of the digital economy.
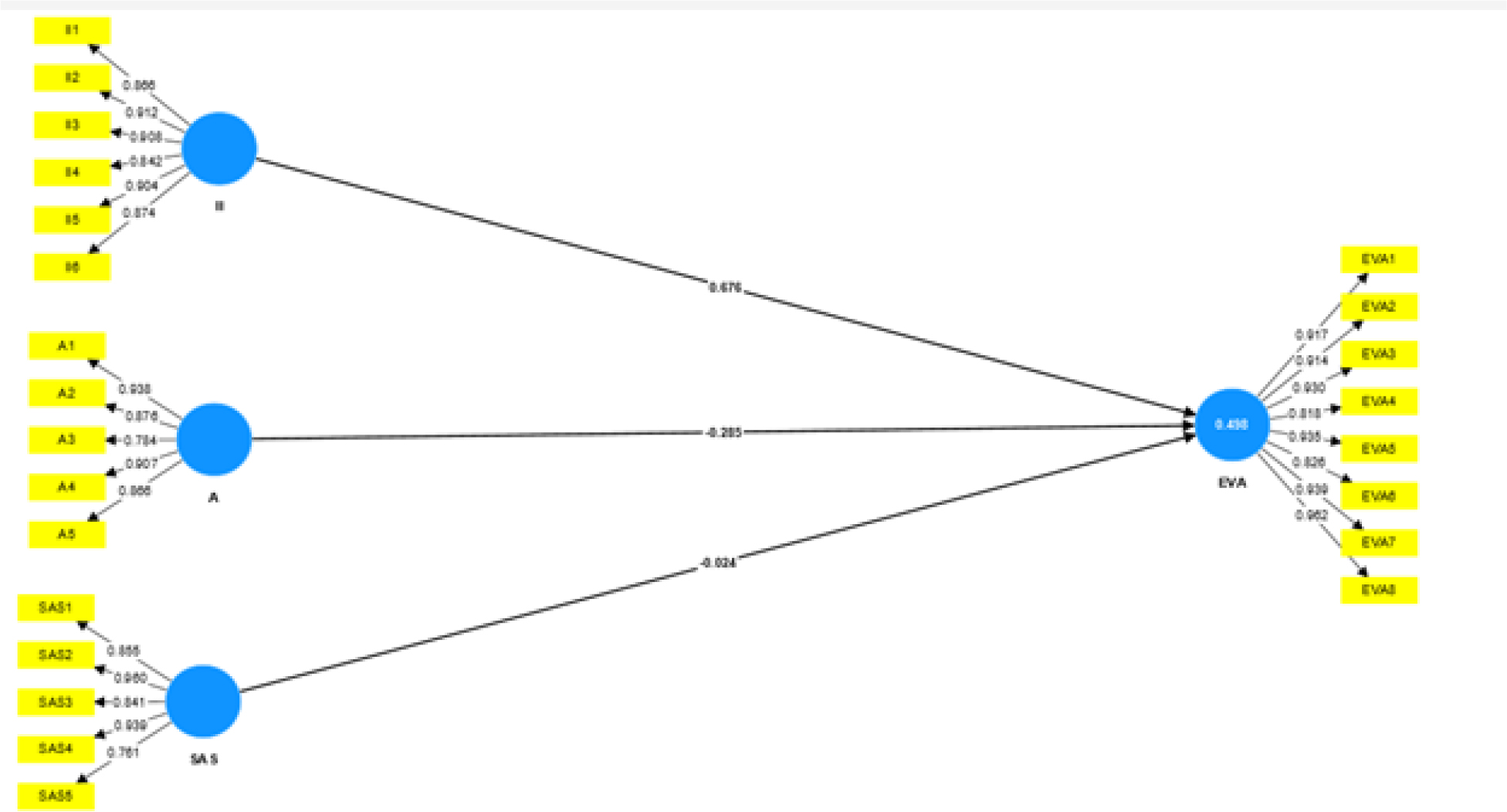
ABSTRACT
Global Electric Vehicle (EV) market growth has garnered attention as an avenue to curb the discharge of greenhouse gases and confront the effects of climate change. Policymakers, industry stakeholders and researchers must comprehend the factors influencing EV adoption. This study investigates the components affecting the introduction of electric vehicles in Bangladesh due to its prominence. The study examines how affordability, insufficient infrastructure and after-sales service affect electric vehicle initiation in Bangladesh through a comprehensive survey of a diverse group. These factors significantly influence EV adoption trends in Bangladesh and the study employs descriptive and inferential statistics to assess their impact. Valid data were analyzed using SmartPLS 4. The study reveals that affordability and infrastructure act as impediments to Bangladesh's adoption of electric vehicles, underscoring the pivotal role of cost in consumer decision-making. To boost EV adoption in the country, there's a need for financial incentives and reduced upfront costs for EVs. Conversely, the study indicates that after-sales service does not significantly affect electric vehicle adoption in Bangladesh. Given these results, policymakers and industry stakeholders should prioritize affordability to accelerate EV adoption and enhance infrastructure and after-sales services to improve the EV ownership experience. These insights can aid policymakers and stakeholders in promoting sustainable transportation and EV adoption in Bangladesh, ultimately contributing to climate change mitigation and global air quality improvement. Additionally, the conclusions derived can serve as a foundation for further investigation, exploring socio-economic, political and macroeconomic variables in greater depth.
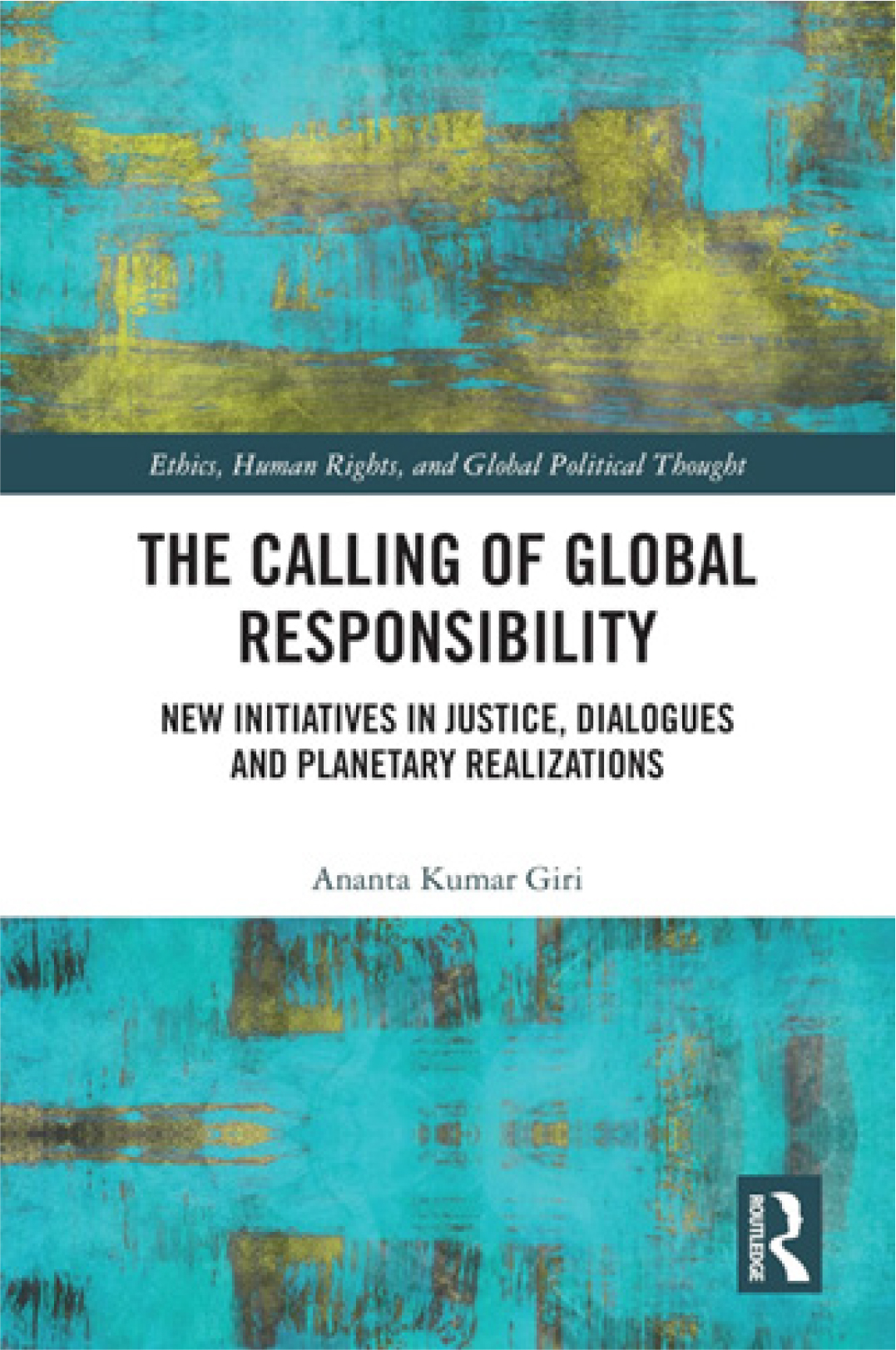
Abstract content not found!
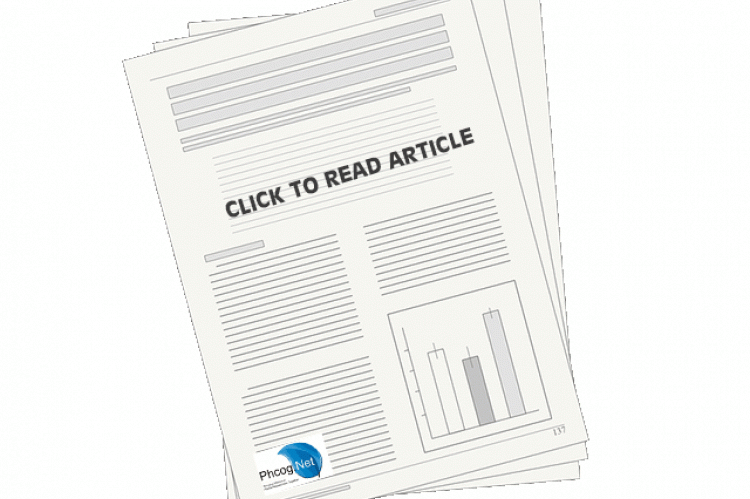
Abstract content not found!
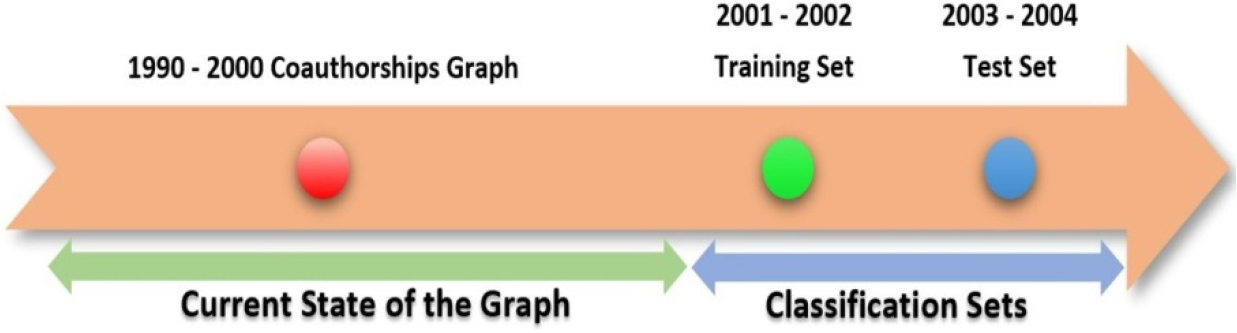
Abstract
This paper presents the challenge of predicting collaborations between research scientists as a datathon experience. The focus of the challenge task is determining whether or not the author of a research paper is keen to collaborate with another author in the future. The main aims of the datathon challenge are: (i) to show the feasibility of automatically identifying potential collaboration in a research network as a link prediction task; (ii) to propose a methodology for the environment configuration that covers data collection, selection and preparation stages required for link prediction in a massive event. (iii) to join the efforts of students from different fields of study in solving the task from a multi-disciplinary perspective. For this purpose, we created a corpus with DBLP data covering publications from 1990 to 2004. The created dataset has been made available for further research. Altogether, the datathon attracted 78 registered students, yielding 13 submissions of teams composed of 6 students each. In this paper, we compare their approaches and analyze their performance.
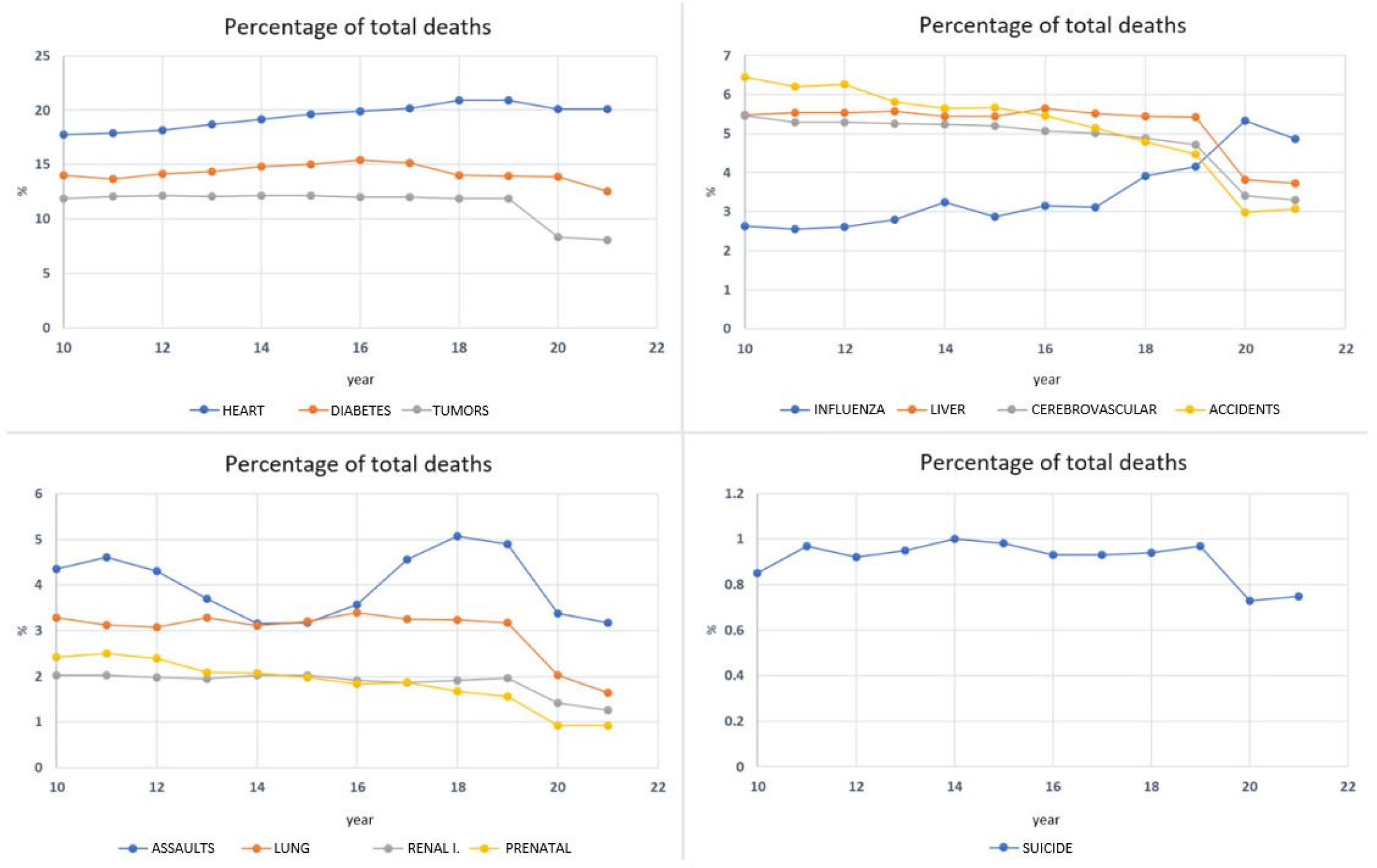
ABSTRACT
Two of the 11 primary causes of death among the Mexican population during the period 2010-2021 were analyzed in its correlation with Mexico's socio-economic situation and the identified patterns are presented. It has been confirmed that the application of learning algorithms, specifically the use of decision trees, is very useful in analyzing and correlating data from various sources, which is evidenced by the obtained results. The constructed decision trees illustrate and explain the patterns found between deaths from heart disease and suicide compared to certain socio-economic attributes from Mexico. This helps to characterize the population that experienced these deaths. Our results can be utilized to aid in the decision-making process for policies aimed at preventing health issues that affect the Mexican population, as well as to define other health public policies.
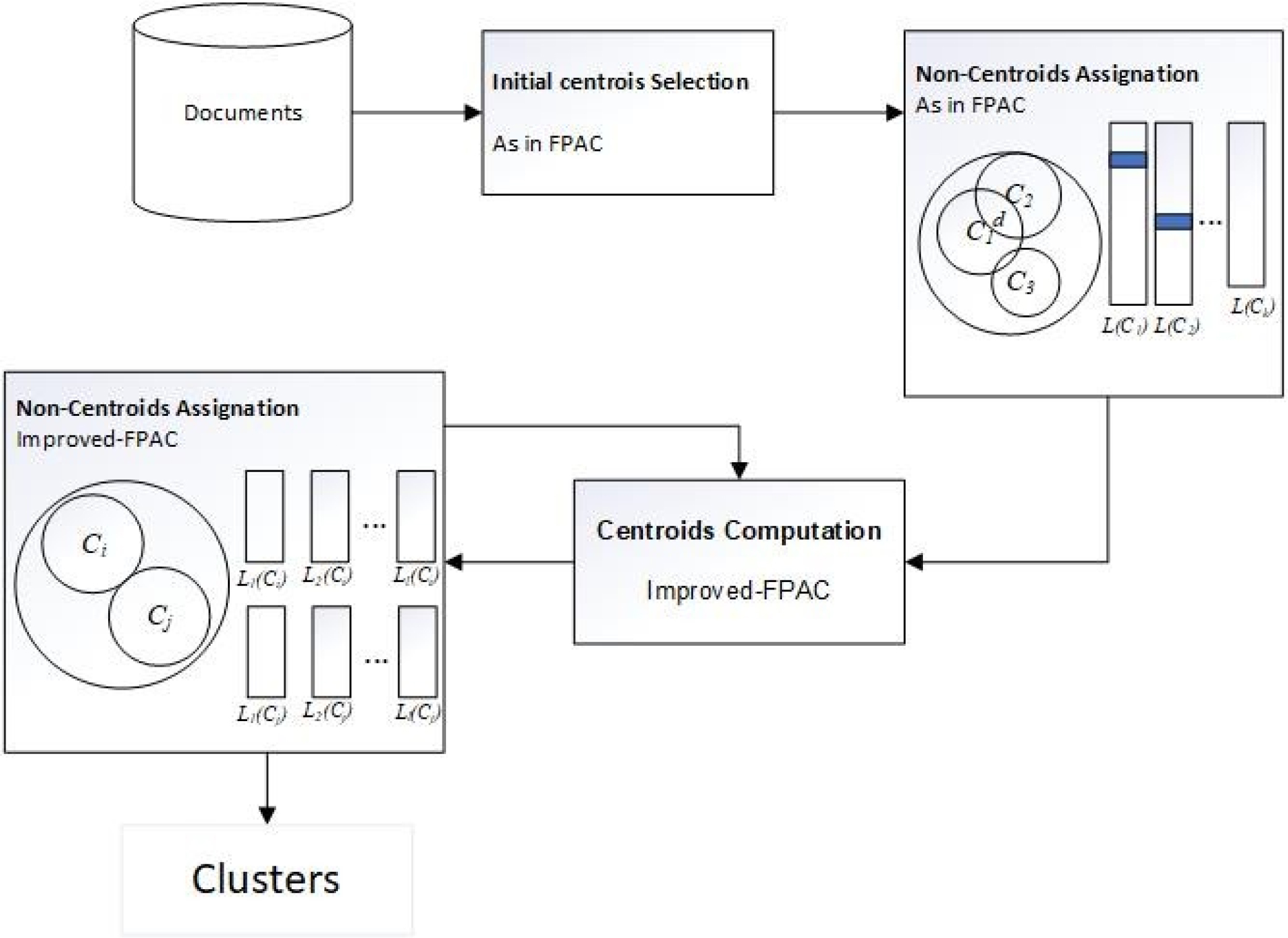
ABSTRACT
Clustering is fundamental for categorizing and exploring information, particularly in written texts. Traditional clustering algorithms such as K-Means generate clusters in which each document is assigned to a cluster based on a centroid or representative of the cluster. Nevertheless, it is common to find situations where a single centroid is not enough to represent a cluster. To solve this problem, some variants of the K-Means algorithm have been introduced by considering more than one centroid per cluster. However, determining the number of centroids per cluster is a challenge; the user must employ trial and error to determine a good value, which is time-consuming. This paper proposes a solution to this problem for Improved-FPAC (Fast Partitional Clustering Algorithm, one of the most recent text document cluster algorithms) by introducing a method to compute the parameter l (number of centroids per cluster) based on the characteristics of the corpus. Based on our experiments on different public standard data sets, the method proposed in this paper, allows Improved-FPAC to obtain better clustering quality than the default value suggested by Improved-FPAC’s authors.
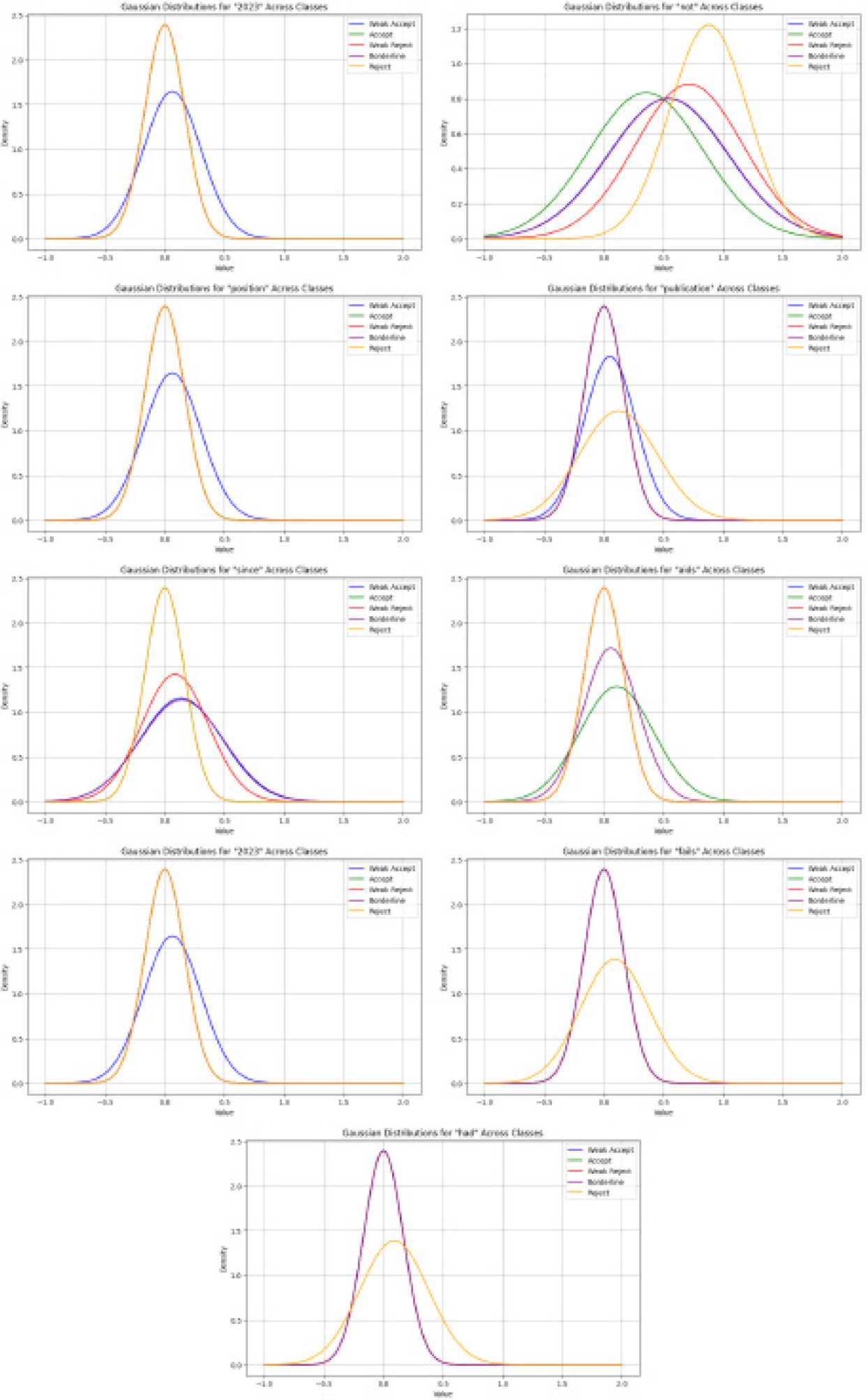
ABSTRACT
This research investigates the efficacy of various computational models and feature sets in the task of classifying scientific text reviews into distinct categories. Utilizing a combination of Word Space Models (WSM) and the Linguistic Inquiry and Word Count (LIWC) dictionary, the study endeavors to categorize reviews initially into five classes before simplifying the classification scheme into a binary system (’accept’ and ’reject’). Despite the relatively straightforward nature of the employed feature sets, the binary classification approach demonstrated a notable improvement over a basic baseline that non-discriminatively assigns reviews to the most populous category. We obtain a recall of 0.758, compared with a baseline of 0.585 to the majority class and 0.62 and 0.66 of BERT and RoBERTa respectively. This performance can be considered significant given the diverse and subjective nature of the review content, contributed by 80 distinct individuals, each with their unique writing style and evaluative criteria. This work contributes to XAI through linguistic analysis revealing, for example that a minimal subset of features, specifically two out of the seventy provided by LIWC, can yield insightful distinctions in review classifications (0.649 recall). The analysis further identifies specific lexemes, such as ‘not’, ‘since’ and ‘had’, which offer deeper insights into the linguistic constructs employed by reviewers.
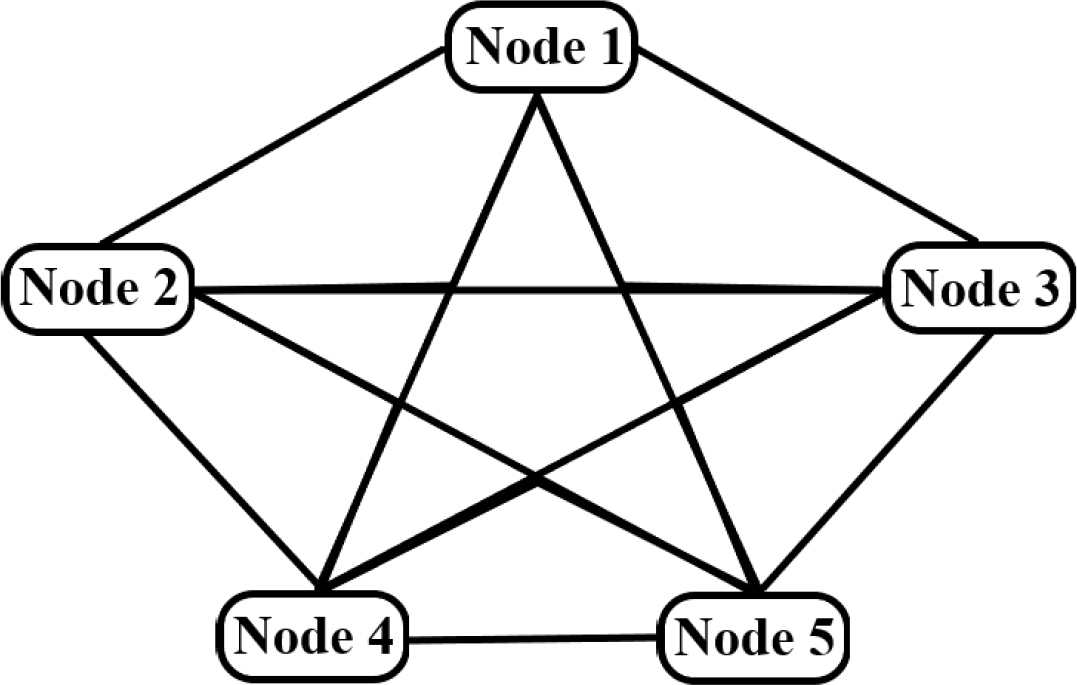
ABSTRACT
Co-reference resolution has been a perennial issue in linguistics and language processing discipline. Over the past more than fifty years, researchers have developed a variety of strategies to solve this problem. The problem is still being researched today and trying to address the challenges of the problem in various languages. We have discussed a Peer-to-Peer (P2P) network-based strategy for developing a co-reference resolution system in this research. This method substitutes global information for local information in the text and hence improves the accuracy. The Assamese data set is created with the necessary tagging to test the system, and the results are pretty encouraging.
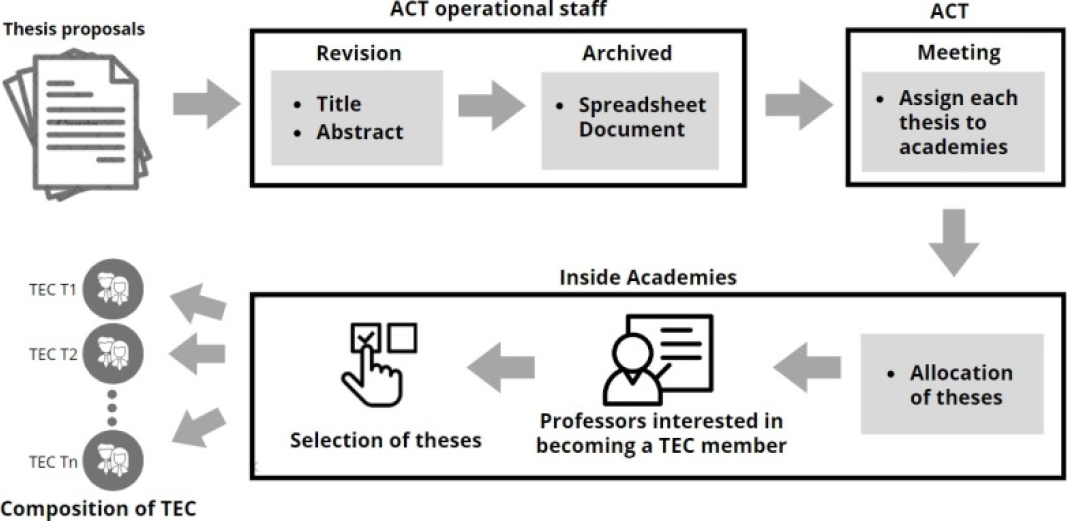
ABSTRACT
This paper presents a recommender system for the assignment of thesis reviewers at ESCOM-IPN Mexico. The system analyzes the thesis proposals, extracting relevant information and comparing it against the profiles of professors and courses given by the academic departments. The system determines the most suitable profiles and departments for the thesis review through a similarity evaluation. The results show that the system can generate accurate recommendations with a high degree of coincidence with the current process carried out by the committee in charge. In addition, the system performs an alternative process by suggesting reviewers with profiles more closely related to the thesis proposals considering additional information such as research papers and areas of expertise.
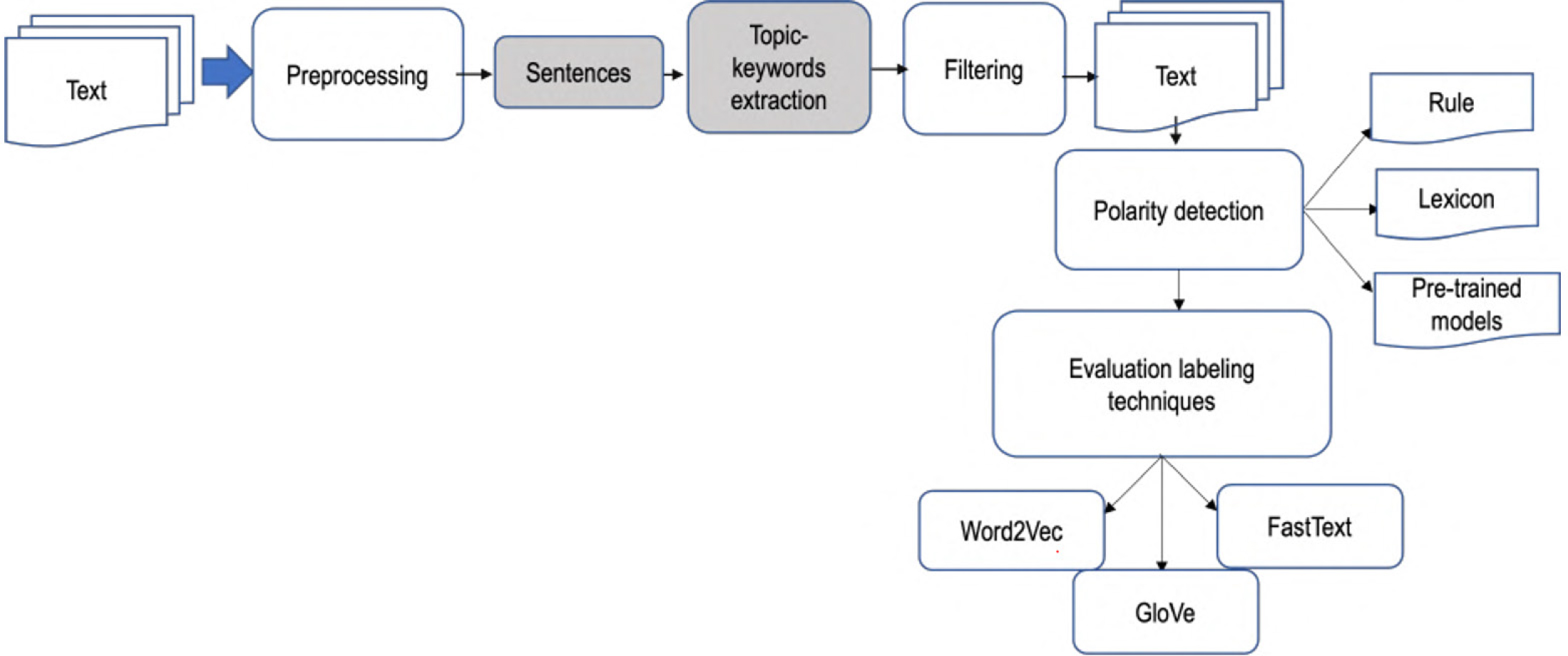
ABSTRACT
In this article, we explore the potential of textual data as an alternative source for economic analysis. We employ a range of data extraction and labeling techniques, including domain expert, rule-based approaches and pre-trained language models to determine the polarity of textual data. By compiling a corpus of Spanish news articles from Mexico spanning 2006 to 2023 and comparing our sentiment index with economic data from official agencies, we demonstrate that news articles can serve a reliable source for economic analysis.
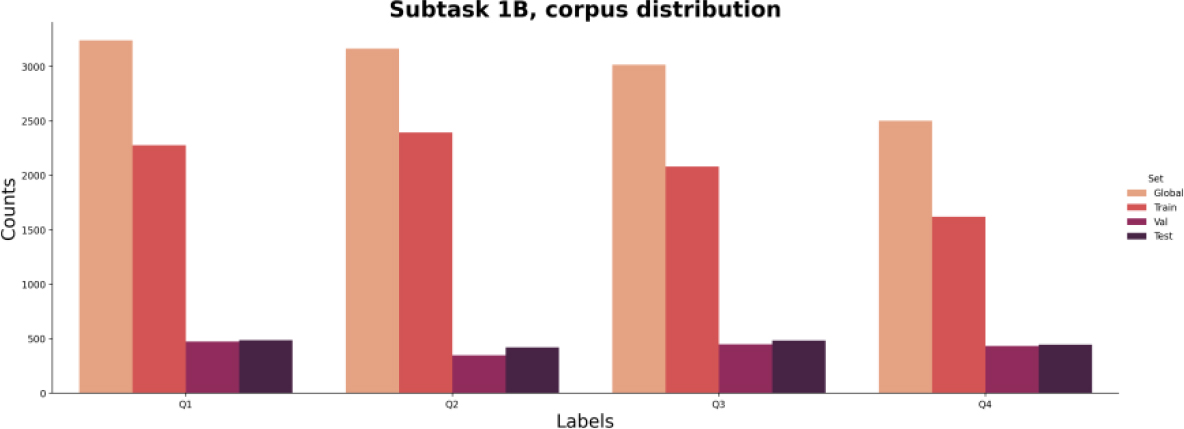
ABSTRACT
Journal recommendation systems serve as valuable tools for researchers, addressing the complex task of multi-class text classification. With the advent of Transformer architectures, there is newfound potential to enhance existing recommendation systems, particularly in the realm of academic journals. While current technologies are capable of classifying journals based on article content, we still lack an algorithm that can predict the quartile ranking of journals. Such a development would be immensely beneficial for researchers to assess their articles before submission. In our study, we tackle both tasks simultaneously. We trained various state-of-the-art Transformer architectures and machine learning algorithms, ranging from BERT to GPT-2. Surprisingly, we achieved better quantitative results with smaller models, especially DistilBERT, as well as classical classifiers. However, when it came to quartile prediction, success was limited to testing within the same journals. Generalization across different journals proved elusive. This observation strongly suggests that quartile prediction currently relies indirectly on journal classification, highlighting the limitations of existing technology, the collected dataset, or the impossibility of solving the task.